The Landscape of Emerging AI: New Frontiers Ahead
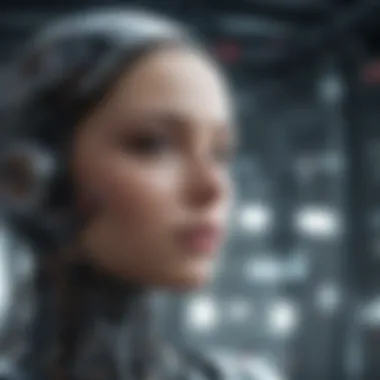
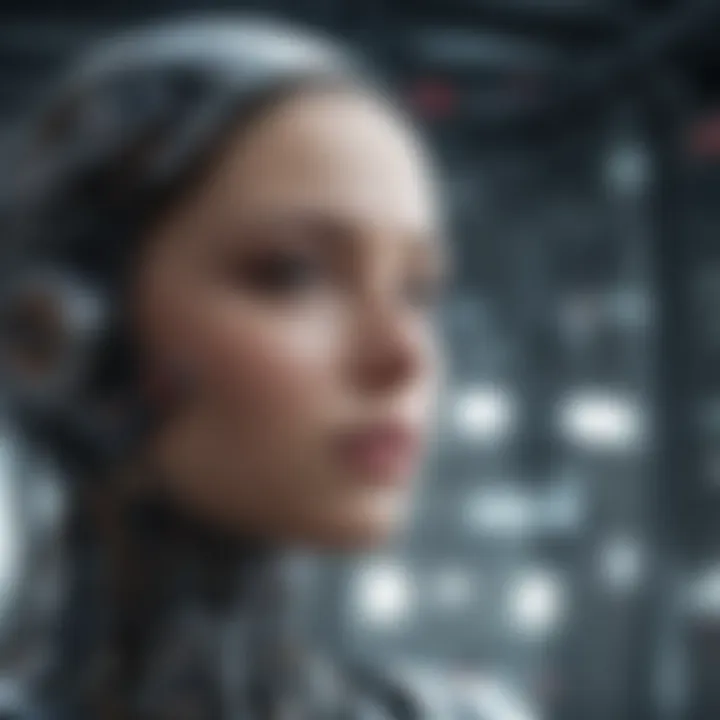
Intro
The domain of artificial intelligence (AI) is rapidly evolving, marked by significant advancements that alter the way businesses operate, scientists research, and individuals interact with technology. As we venture into this territory, it becomes essential to parse the current developments to understand their implications. With AI permeating various sectors, including healthcare, finance, and education, the urgency to grasp its fundamentals intensifies. This article aims to deliver an insightful exploration of the state of emerging AI technologies, their key players, ethical ramifications, and the long-term effects on society and governance.
Key Research Findings
Overview of Recent Discoveries
Recent research has uncovered numerous breakthroughs within AI. Notable developments include the advent of generative models like OpenAI’s ChatGPT, which has transformed natural language processing capabilities. Moreover, advancements in computer vision have paved the way for real-time facial recognition and object identification, impacting security systems and user experiences alike. Machine learning frameworks, such as Google's TensorFlow and Facebook's PyTorch, have become widely adopted tools for researchers and developers, facilitating the creation of sophisticated AI models.
In addition, studies indicate a surge in routine applications. For instance, AI-driven diagnostic tools in healthcare have shown promise in areas like radiology and pathology, where they assist professionals in identifying diseases more efficiently. Further research has emphasized the intersection between AI and big data, illustrating how businesses utilize predictive analytics to refine decision-making processes.
Significance of Findings in the Field
The implications of these discoveries are profound. They not only enhance operational efficiency across sectors but also challenge conventional paradigms.
- Healthcare: AI applications can lead to cost reductions and improved patient outcomes through faster diagnosis and personalized treatment plans.
- Finance: Algorithms improve risk assessment and fraud detection, enabling financial institutions to protect assets effectively.
- Education: Adaptive learning platforms harness AI to provide tailored educational experiences, catering to individual student needs.
These findings point toward a future where AI technologies continually reshape our professional landscapes and everyday experiences.
Ethical Considerations
As these technologies become integrated into society, ethical concerns arise. Issues of bias in AI algorithms, privacy, and transparency in decision-making processes are thus crucial topics within this discussion. The risk of unintended consequences warrants careful examination.
"AI is a tool, not a decision-maker. Its effectiveness is limited by the quality of its inputs and the integrity of its operators."
Societal stakeholders must engage in interdisciplinary dialogues to forge responsible AI governance. This collaborative approach is vital to align technological capabilities with ethical boundaries.
Future Outlook
Looking ahead, the trajectory of AI research indicates an increasing focus on hybrid models that combine multiple AI approaches. By doing so, researchers aim to enhance stability and adaptability, ensuring that systems can respond intelligently to real-world variables.
The role of interdisciplinary collaboration can’t be overstated. Experts from fields like ethics, sociology, and technology must come together to shape AI applications that prioritize human welfare.
Understanding Emerging AI
The realm of artificial intelligence is rapidly evolving, demanding attention and scrutiny from various sectors. Understanding emerging AI is crucial as it lays the groundwork for recognizing how these technologies will shape our future. This section will discuss the significance of defining emerging AI and the key technologies that are driving this field forward.
Experts view emerging AI as a crucial area of study for its potential to revolutionize industries, enhance human capabilities, and solve complex global challenges. The current trajectory indicates that investments in AI can lead to substantial benefits, especially in productivity and efficiency. This exploration will help us grasp not only the technological aspects but also the ethical implications and societal impacts these developments may have.
Defining Emerging AI
Emerging AI refers to computer systems that exhibit advanced capabilities such as learning from experience, understanding human language, and recognizing patterns in data. Unlike traditional AI, which relied heavily on predetermined rules, emerging AI systems adapt and evolve based on vast datasets and real-time information.
This definition encapsulates several underlying technologies and approaches, including machine learning, natural language processing, and computer vision. Each of these plays a significant role in making AI systems smarter and more efficient. Defining these terms accurately is key to discussing their implications in various industries.
Key Technologies That Underpin AI
Machine Learning
Machine learning stands as a foundational element of emerging AI. This technology allows systems to learn from data without explicit programming. The key characteristic of machine learning is its ability to find patterns and make predictions based on input data. This flexibility positions machine learning as a beneficial choice for many applications, especially in areas like finance and healthcare.
A unique feature of machine learning is its scalability, which allows it to analyze large datasets to improve outcomes. This is advantageous, but it can also lead to biases if the data is not carefully curated. Thus, understanding machine learning is essential for comprehending the broader AI landscape.
Natural Language Processing
Natural language processing (NLP) focuses on enabling machines to understand and interpret human language. One critical aspect of NLP is its capability to enhance human-computer interaction. This technology is vital for applications such as chatbots and virtual assistants.
The key characteristic of NLP is its capacity to analyze context and sentiment, making interactions more meaningful. Its growing popularity stems from the demand for efficient communication across platforms. However, challenges exist, as NLP must deal with the nuances and complexities of human language, which can lead to misinterpretations if not implemented correctly.
Computer Vision
Computer vision gives machines the ability to interpret and understand visual information from the world. This technology is pivotal in sectors ranging from autonomous vehicles to image recognition systems. The defining aspect of computer vision is its ability to analyze images and videos to identify objects and their relationships.
Computer vision is a beneficial and transformative technology, providing utility in countless applications, including security and surveillance. However, it faces challenges regarding the accuracy of recognition systems, particularly in diverse settings. Addressing these limitations is crucial for the continued development of effective computer vision solutions.
Understanding these technologies is not just about knowing their capabilities. It's about recognizing their implications on privacy, employment, and ethics in a broader context.
By comprehending these foundational technologies, one can appreciate how emerging AI is reshaping our world and what considerations must be taken into account as we advance.
The Evolution of AI Technologies
The evolution of AI technologies marks a pivotal transformation in how systems learn, adapt, and interact with the world. Understanding this evolution is crucial as it provides insights into past developments, recent advancements, and the trajectory of future innovations. The advancements in AI technologies are not just technical achievements; they represent a shifting paradigm in various sectors, profoundly impacting industry practices, societal norms, and even individual daily life.
Historical Context of AI Development
The roots of artificial intelligence trace back to the mid-20th century. Early work in this field focused on mimicking human reasoning and problem-solving. Pioneers like Alan Turing laid foundational concepts, notably encapsulated in the Turing Test, which sought to measure a machine's ability to exhibit intelligent behavior. Significant milestones include the development of symbolic AI in the 1960s, which used human-like reasoning through rule-based systems. However, these early systems struggled with complex tasks due to their reliance on explicit programming.
In the late 20th century, the advent of machine learning began to change the landscape. Instead of relying on hard-coded rules, machine learning allowed systems to learn from data. This shift enabled more reliable performance across various tasks, ultimately leading to the AI boom we observe today.
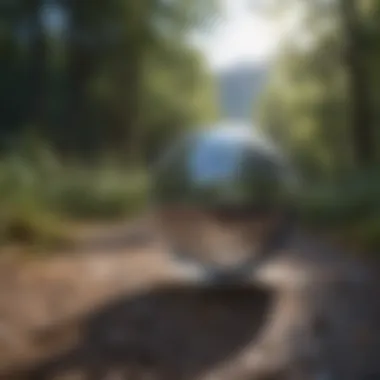
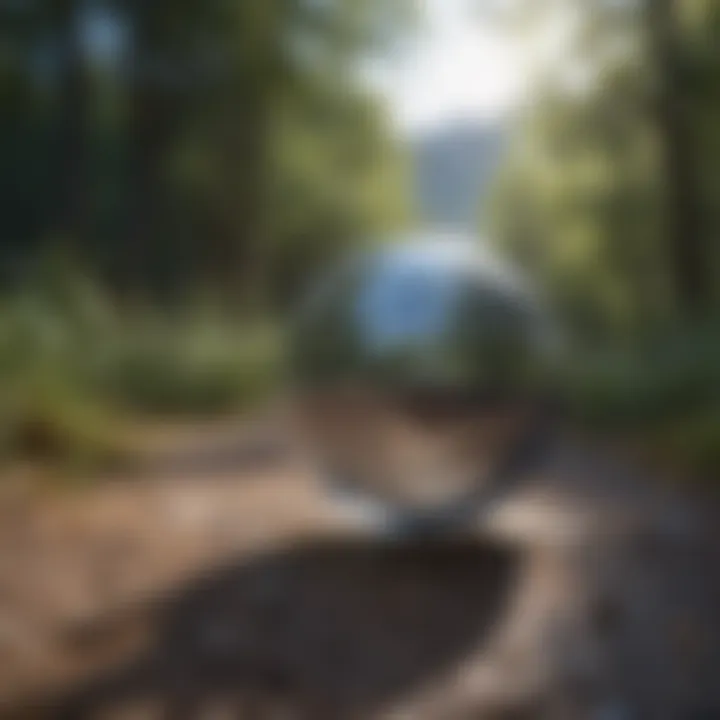
Recent Breakthroughs in AI
The past few years have witnessed significant breakthroughs that have reshaped the field of AI. These breakthroughs not only reveal the sophistication of current technologies but also emphasize their practical applications in real-world scenarios.
Deep Learning Advancements
Deep learning has emerged as a cornerstone of modern AI. A specific aspect of deep learning advancements is the utilization of neural networks that can learn and make intelligent decisions. This approach is characterized by its ability to process vast amounts of data, uncover patterns, and improve over time through training.
The key characteristic of deep learning is its hierarchical structure, which separates the processing of information into multiple layers. This enables enhanced feature detection, making it particularly effective in complex tasks like image and speech recognition. Such capabilities make deep learning a popular choice for various applications, especially in sectors like healthcare, where accurate diagnostics are critical.
However, deep learning also has challenges. It requires substantial computational power and large datasets, making accessibility a concern for smaller organizations. Nevertheless, its unique feature of making sense of unstructured data gives it advantages often unmatched by traditional AI methods.
Generative AI Models
Generative AI has made waves across various domains, producing new content from existing datasets. A pertinent aspect of generative AI models is their ability to create realistic and diverse outputs, including images, texts, or sound. This capability has sparked creativity in art, music, and even automated report generation.
The key characteristic of generative AI is its use of models such as Generative Adversarial Networks (GANs) that pit two networks against each other, improving the quality of generated content over iterations. This aspect makes generative AI increasingly popular, as it opens up myriad possibilities for content creation, personalization, and innovation.
However, the unique feature of generative models is coupled with ethical considerations and potential misuse. Issues like copyright infringement and the creation of fake content pose significant challenges. The advantages of generative AI, such as enhancing creativity and efficiency, must be weighed against these important concerns.
The evolution of AI technologies is defined not only by technical capabilities but also by the ethical discussions they provoke and the societal changes they invoke.
Applications of Emerging AI
The applications of emerging artificial intelligence technologies are critical for understanding how AI is reshaping various sectors of society. These applications not only showcase the capabilities of AI but also provide tangible benefits in fields such as healthcare, finance, education, and transportation. Each application illustrates the unique ways in which AI enhances efficiency, improves decision-making, and influences outcomes.
AI's integration into diverse industries signifies a shift towards automated systems that learn from data. It is essential to consider how these technologies not only allow for innovative solutions but also bring challenges such as ethical concerns and potential biases. Understanding applications of emerging AI helps stakeholders make informed decisions.
AI in Healthcare
AI in healthcare represents a transformative force in medical practice. Technologies from predictive analytics to diagnostic tools are emblematic of AI's potential in improving health outcomes and streamlining operations within medical institutions.
Predictive Analytics
Predictive analytics in healthcare involves using data analysis techniques to assess the risk of patient conditions and outcomes. This application integrates numerous data sources like electronic health records, genetic information, and lifestyle factors to foresee health issues before they arise.
The key characteristic of predictive analytics is its ability to leverage vast amounts of data to identify patterns that can lead to preventive care. The benefit of this application is significant; it can enhance patient care by allowing for timely interventions. A unique feature of predictive analytics is its adaptability across various healthcare scenarios, from chronic disease management to patient readmission risks. However, it also has disadvantages, such as potential inaccuracies in predictive models if data is biased or insufficient.
Diagnostic Tools
Diagnostic tools powered by AI significantly enhance the speed and accuracy of medical diagnoses. These tools can analyze images, sound, and other data to identify diseases more efficiently than traditional methods.
The key characteristic of diagnostic tools is their capacity for image recognition and pattern detection at a level that often exceeds human capabilities. It is a popular choice in this article due to its direct impact on patient outcomes and medical efficiency. A unique feature is the continuous learning process of these tools, enabling them to improve over time. Nonetheless, the reliance on AI for diagnostics may raise concerns regarding overdependence on technology and the eventual replacement of human expertise.
AI in Finance
In finance, AI plays a pivotal role in analytical capabilities and decision-making processes. The use of technologies like algorithmic trading and risk assessment demonstrates how AI optimizes operations and enhances financial strategies.
Algorithmic Trading
Algorithmic trading uses AI algorithms to execute trades at speeds and volumes that are impossible for human traders. By analyzing market data and trends, these algorithms can make real-time decisions that maximize investment returns.
The key characteristic of algorithmic trading is its efficiency in processing and acting on vast financial data sets. It is a favorable topic in this article due to its capacity for reducing costs and risks associated with trading. A unique feature is the customizable nature of algorithms, allowing firms to tailor them to specific investment strategies. However, the volatility induced by algorithmic trading might lead to market instability during sudden shifts.
Risk Assessment
Risk assessment in finance employs AI to evaluate the potential financial exposure an organization might face. This application analyzes historical data and market conditions to predict risk levels across various portfolios.
The key characteristic is its capability to process more data than traditional risk assessment methods, providing more nuanced insights. It is a beneficial topic in this article due to its impact on informed decision-making and compliance with regulations. A unique feature is the enhancement of risk models through continuous learning, which aids in maintaining accuracy. On the downside, reliance on algorithms raises issues about transparency and accountability in decision-making.
AI in Education
In education, AI supports personalized experiences and assessment automation, thereby fostering a more effective learning environment. Applications such as personalized learning and assessment automation are reshaping how educational institutions deliver content and measure learning outcomes.
Personalized Learning
Personalized learning facilitates tailored educational experiences based on individual learner's needs, objectives, and pace. AI analyzes data from student interactions and performance to customize lesson plans.
The key characteristic is its adaptability, ensuring that education fits the learner rather than the other way around. This is a popular application in this article due to its potential for maximizing student engagement and achievement. A unique feature of personalized learning is the implementation of feedback loops that allow for real-time adjustments. However, it might lead to concerns regarding the equity of access to technology and personalized resources.
Assessment Automation
Assessment automation uses AI systems to evaluate student performance, reducing the time educators spend on administrative tasks. These systems can grade tests and assignments, providing quick feedback to students.
The key characteristic is its efficiency in processing large volumes of assessments, making it a beneficial choice for educational systems. This article includes it as it exemplifies how AI can optimize educational workflows. A unique feature is the capability of such tools to provide analytics on student performance over time. Nonetheless, there is the risk that automated assessments may lack the nuance of human judgment, leading to unfair evaluations.
AI in Transportation
Transportation is another area where AI enables considerable advancements through applications like autonomous vehicles and traffic management systems. These innovations have the potential to revolutionize how we move people and goods.
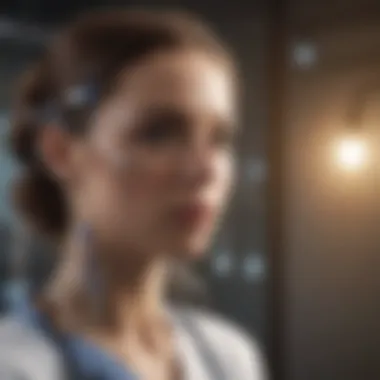
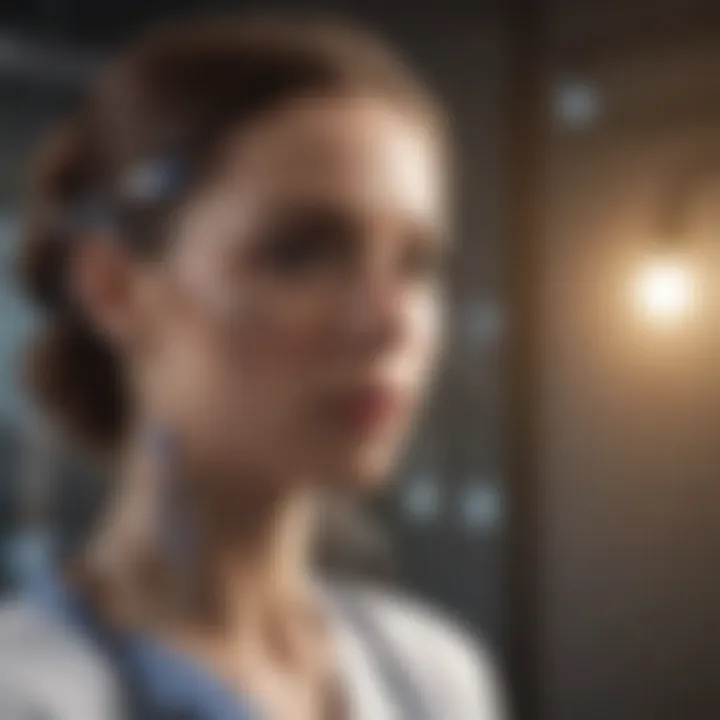
Autonomous Vehicles
Autonomous vehicles utilize AI to operate without human intervention. These vehicles incorporate various technologies like computer vision and sensor data to navigate and respond to their surroundings.
The key characteristic of autonomous vehicles is their potential to reduce human error in road safety. AI’s role in this sector is crucial as it highlights safety benefits as well as efficiency in transport logistics. A unique feature is the continuous improvement of AI algorithms based on real-world driving data. However, public acceptance and regulatory concerns remain major challenges to widespread implementation.
Traffic Management Systems
Traffic management systems employ AI to optimize traffic flow and reduce congestion in urban areas. These systems analyze real-time traffic data, enabling them to adjust signals and manage vehicle distribution.
The key characteristic is its dynamic response to traffic conditions, allowing for more efficient use of infrastructure. It is a beneficial inclusion in this article because improved traffic management contributes to overall urban productivity and safety. A unique feature is integration with other smart city technologies, facilitating a holistic approach to urban planning. Nonetheless, there can be challenges related to the cost of implementation and interoperability with existing systems.
The applications of emerging AI extend far beyond individual sectors. They illustrate the technology’s capacity to enhance operational efficiency, improve decision making, and address complex challenges, shaping the future of industries across the globe.
Ethical Implications of Emerging AI
The rise of emerging AI technologies brings profound ethical questions that can not be ignored. Addressing these questions is crucial for ensuring that AI systems are developed and utilized responsibly. As these technologies permeate sectors such as healthcare, finance, and education, they carry the potential to impact lives significantly. A thorough exploration of the ethical implications surrounding emerging AI is necessary for fostering trust and accountability among stakeholders.
Privacy Concerns
Privacy is a fundamental right that can be jeopardized by advances in AI. Emerging AI systems often rely on large datasets, which can include personal information. This increases the risk of unauthorized access and misuse. For instance, consider AI applications in healthcare, where sensitive patient data is used to improve diagnostic accuracy. While the intention is to enhance patient care, the trade-off includes potential exposure of private health information.
Maintaining privacy requires establishing robust data governance frameworks. These frameworks should outline how data is collected, used, and stored. Organizations need to implement strict security measures to protect personal information. Transparency about data practices is also vital. When people understand how their data is being used, they are more likely to trust AI technologies.
Bias and Fairness in AI
Bias in AI is another pressing ethical issue. AI systems learn from historical data, which can contain societal biases. If not addressed, these biases can be perpetuated and even amplified in AI outcomes. For example, facial recognition technology has faced criticism for lower accuracy rates among certain demographic groups. This raises concerns about fairness in law enforcement and hiring practices.
To mitigate bias, it is essential to adopt inclusive data practices. This includes diversifying datasets to ensure representation across various demographic categories. Regular auditing of AI systems for bias is also necessary. This includes evaluating algorithms based on their performance across different groups. Research in fairness and accountability can play a significant role in developing equitable AI systems.
Accountability and Responsibility
With great power comes great responsibility. The accountability of AI systems is a critical aspect of ethical AI development. Stakeholders must understand who is responsible for the outcomes generated by AI systems. As AI takes on more decision-making roles, it is essential to establish clear guidelines regarding accountability.
For instance, if an autonomous vehicle is involved in an accident, determining liability can be complex. Should the manufacturer be held accountable? Or is it the responsibility of the software developers? Establishing legal frameworks that define accountability is necessary to address these questions.
Moreover, organizations must embrace a culture of ethical responsibility. This includes training their teams to understand the ethical implications of their work. From developers to decision-makers, everyone should be aware of the impact their actions can have.
"Addressing ethical implications right from the development phase can prevent longer-term societal issues and foster a better relationship between AI technologies and the public."
The Future of AI Research
The future of AI research holds significant implications for both technological advancement and societal change. As AI technologies become increasingly integrated into various facets of life, researchers and practitioners must consider how these innovations can contribute positively. Emerging AI research aims to not only advance applications but also to address challenges. The following subsections outline emerging trends and potential challenges in this domain.
Emerging Trends in AI Research
Interdisciplinary Approaches
Interdisciplinary approaches stand out as a critical trend in AI research. Combining insights from fields like computer science, sociology, and cognitive sciences fosters innovation and encourages diverse perspectives. This blending of expertise enhances problem-solving capabilities. One key characteristic of this method is its adaptability in addressing complex problems that cannot be solved within the confines of a single discipline.
The unique feature of interdisciplinary approaches is their ability to produce holistic solutions. By acknowledging the multifaceted nature of real-world issues, researchers can create AI models that are more robust and socially relevant. However, a challenge often faced is the communication gap between different fields. Effective collaboration is paramount, yet it often requires additional effort to align methodologies and terminologies.
Collaboration Between Sectors
Collaboration between sectors, including academia, industry, and government, significantly propels AI research forward. This synergy results in shared resources and data, leading to enhanced innovations. A notable characteristic of this collaboration is the merging of theoretical insights with practical applications. Such partnerships often yield technological advancements that are both viable and scalable, which aligns perfectly with the goals of this article.
The unique feature of cross-sector collaboration lies in its diverse vantage points. Each sector offers distinct expertise and resources, which can enrich the research process. Nevertheless, potential disadvantages include differing priorities, which can complicate joint projects. Successful collaboration necessitates clearly defined goals and transparent communication to mitigate such issues.
Potential Challenges in AI Development
Regulatory Frameworks
Regulatory frameworks represent a critical aspect of overseeing AI development. These frameworks aim to ensure ethical standards and protect citizens' rights in a rapidly evolving digital landscape. The essential characteristic of effective regulatory frameworks is their balance between innovation and consumer protection. In this article, they play a beneficial role by providing guidance to researchers and practitioners.
A significant advantage of robust regulatory frameworks is that they can instill public trust in AI technologies. When stakeholders know that there are strict guidelines in place, they become more open to adopting new technologies. However, there can be disadvantages. Overly stringent regulations may stifle innovation and slow down the pace at which new solutions are developed.
Technological Limitations
Technological limitations continue to be a pervasive challenge in AI research and development. These limitations include issues like data quality, processing power, and algorithmic biases, each affecting the reliability and applicability of AI systems. One key characteristic of this topic is its ability to highlight areas needing further research. Identifying these gaps is crucial for moving the field forward.
A unique feature of understanding technological limitations is its role in fostering realistic expectations. By acknowledging what current technology can and cannot achieve, researchers can set actionable goals and timelines. However, this can also limit ambition, leading some to feel discouraged rather than motivated by these insights.
"The intersection of diverse fields enhances our understanding and application of AI technologies, paving the way for innovative solutions that resonate with societal needs."
This approach will not only advance practical applications but also ensure that they align with ethical standards.
Key Players in Emerging AI
Understanding the key players in emerging AI is crucial for grasping how this technology evolves and impacts various sectors. These players drive innovation, shape research agendas, and influence application areas that affect society at large. Their contributions help lay the groundwork for how AI technologies are developed, governed, and utilized.
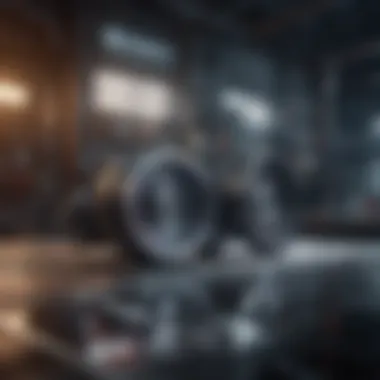
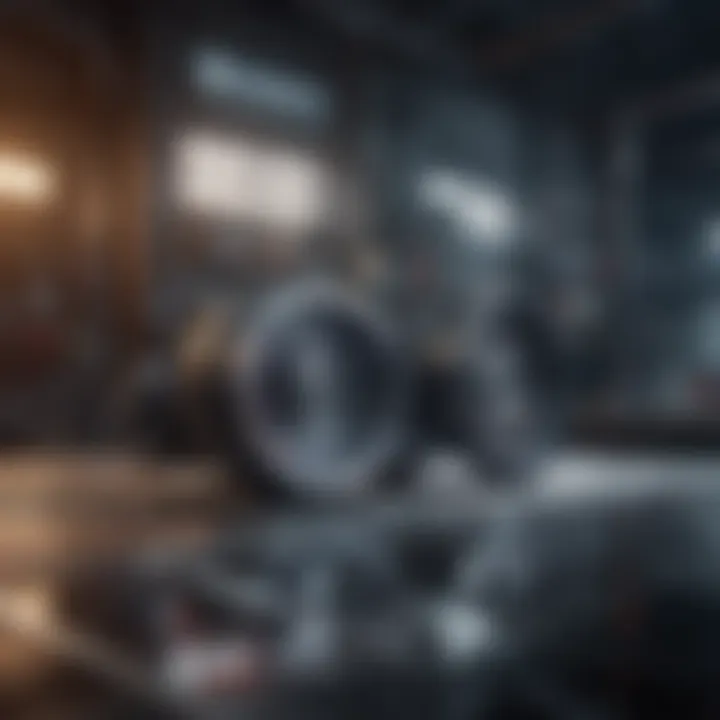
Leading Tech Companies
Google is a leader in AI development, leveraging its vast data resources and advanced algorithms. The company's investments in deep learning and neural networks have significantly contributed to breakthroughs in natural language processing and image recognition. Google's AI research focuses on enhancing user experience and enabling smarter products, like Google Assistant. The key characteristic of Google is its emphasis on scalability, allowing it to deploy AI across its products effectively. A unique feature is Google's TensorFlow, an open-source library that supports machine learning projects, enabling developers globally to innovate. However, concerns exist about user privacy and data handling.
Microsoft
Microsoft has made substantial advancements in the AI arena, particularly with the integration of AI into its cloud services through Azure. The company prioritizes building AI tools that are accessible and beneficial for businesses. A key characteristic of Microsoft is its commitment to ethical AI, promoting fairness and transparency in AI algorithms. The unique feature is its focus on enhancing workforce productivity through solutions like Microsoft 365, which incorporate AI functionalities. This makes Microsoft a popular choice in enterprise solutions. However, there are discussions about the competition it faces from other tech giants in AI innovation.
IBM
IBM stands out with its longstanding history in AI, particularly with IBM Watson. The company has positioned itself as a leader in enterprise AI solutions, focusing on industry-specific applications. A key characteristic of IBM is its strong emphasis on responsible AI practices, potentially giving it an edge in sectors like healthcare and finance. IBM's Watson has a unique feature that allows it to analyze vast datasets and provide insights across multiple domains. This capability can greatly enhance decision-making processes. The challenge lies in the complexity of its systems, which may deter smaller organizations from adopting the technology.
Academic Contributions
Notable Research Institutions
Academic institutions play a vital role in pushing the boundaries of AI research. These institutions often collaborate with tech companies, fostering innovation through a blend of theoretical and applied research. A key characteristic of notable research institutions is their focus on foundational algorithms and ethical considerations in AI development. Their unique feature is the capacity to publish peer-reviewed studies, which advance public knowledge on AI. This collaboration can lead to breakthroughs but may face limitations due to funding constraints and the slow pace of academia compared to industry-driven research.
Influential Scholars
Influential scholars in the field of AI provide critical insights and theoretical frameworks that guide AI advancements. They often engage in interdisciplinary research, exploring how AI intersects with ethics, policy, and technology. A key characteristic of these scholars is their ability to influence regulatory discussions and public perception of AI technologies. Their unique feature is the opportunity to shape curricula at educational institutions, preparing the next generation of AI researchers and practitioners. Nonetheless, they may face challenges in translating their findings into practical applications in the fast-paced tech environment.
Startups and Innovators
Emerging startups and innovators represent the agility and creativity in the AI landscape. They often explore niche applications of AI, challenging established players by providing unique solutions. These companies can move quickly, adapting to market needs and technological developments. A key characteristic of startups is their focus on disruptive technologies, addressing gaps in existing services. Their unique feature often lies in specialized applications, fostering creativity and innovation. However, they usually encounter challenges with scaling their technologies and securing funding in a competitive market.
Governance of Emerging AI Technologies
Governance in the context of emerging artificial intelligence technologies holds a crucial role. It encompasses the systems, policies, and practices that guide AI development and deployment. As AI increasingly influences various sectors of society, establishing strong governance is essential to ensure ethical use and societal benefit. This section will explore the significance of governance in AI, alongside international regulatory efforts and ethical frameworks that are shaping the future of AI.
International Regulatory Efforts
The rise of artificial intelligence technologies has prompted governments and international bodies to consider regulatory frameworks that manage its use and impact. International regulatory efforts aim to establish guidelines that govern AI deployment across borders, ensuring consistency and ethical standards. The European Union has led with its proposals for AI regulations, which emphasize accountability, transparency, and risk management.
Some key points in international regulatory efforts include:
- Compliance and Standards: Agencies are establishing compliance benchmarks for organizations developing AI technologies. This includes guidelines on data protection, algorithmic fairness, and transparency in AI systems.
- Cross-Border Considerations: Regulations must consider the global nature of AI development. Countries are encouraged to collaborate, sharing information and best practices to address cross-border challenges.
- Public Involvement: Engaging with the public is critical in regulatory processes. Stakeholders, including AI developers and civil society, must have a voice in shaping regulations to ensure they align with the public good.
Governance frameworks strive to balance innovation with safety, preventing misuse while still encouraging technological advancement. The importance of comprehensive regulation cannot be overstated as AI continues to evolve, presenting new challenges and ethical dilemmas.
Ethical Frameworks for AI Development
The establishment of ethical frameworks is vital to navigating the complexities of AI technologies. These frameworks are designed to guide AI development in a way that prioritizes human rights, fairness, and accountability. As AI systems infiltrate daily life, defining ethical standards helps mitigate risks associated with AI decision-making.
Some ethical considerations include:
- Transparency: AI algorithms should be understandable. Users need to know how decisions are made and which data is utilized. Transparency fosters trust among users and stakeholders.
- Fairness and Bias Mitigation: AI systems must be designed to minimize biases. Developers should actively work to ensure that their algorithms are fair across diverse populations, reducing disparities in outcomes.
- Accountability Mechanisms: Clear accountability structures should be established. Organizations should be responsible for AI outcomes, with mechanisms to address grievances and rectify mistakes.
"Establishing ethical frameworks is as important as technological innovation in the realm of AI. Without ethical guidelines, even the most advanced systems can lead to societal harm."
Governance and ethical frameworks together form the backbone of responsible AI development. As the landscape of AI technologies continues to evolve, these structures will play an integral role in shaping the future of AI, ensuring it aligns with societal values and serves the collective good.
End: The Road Ahead for AI
The topic of the road ahead for artificial intelligence holds significant relevance in today's discourse on technological advancement. As AI continues to permeate various sectors, understanding its potential trajectory becomes essential for students, researchers, educators, and professionals alike. This article unpacks multiple dimensions of emerging AI technologies and captures a glimpse into future developments.
The landscape of AI is dynamic. Reflecting on current trends provides clarity on how we have arrived at this juncture. The innovations and challenges we face can often serve as indicators for future directions in AI research, application, and governance. Recognizing these elements is crucial for those engaged in the field, as they balance opportunities with the responsibilities that come with powerful technologies.
In contemplating future directions, it is evident that significant strides are needed across various fronts. Not only should stakeholders prioritize ethical frameworks to govern AI usage, but they must also invest in interdisciplinary research. This would ensure that the technology does not merely evolve in isolation but instead benefits a broader context of human endeavor.
Throughout this article, we explored various themes. We considered key players, relevant applications, and the ethical challenges presented by emerging AI systems. Understanding these facets allows for a more profound insight into the implications of artificial intelligence as it continues to advance.
"The future of AI is not just about technology; it’s about how we integrate it within the fabric of society, ensuring that its benefits are equitably distributed."
In summary, as we draw conclusions regarding the road ahead for AI, it is crucial to acknowledge the responsibility we hold. Keeping ethical considerations at the forefront, actively engaging with technological advancements, and remaining vigilant against potential pitfalls will shape the impact of artificial intelligence in the times to come. \n By fostering a landscape where innovation thrives alongside ethical practices, we can chart a promising pathway into the future of AI.
Reflections on Current Trends
Current trends in AI reveal a complex interplay between technological advancements and the societal implications they generate. The growth of deep learning and natural language processing has led to significant breakthroughs, yet challenges persist regarding bias and accountability. For instance, the adoption of AI tools in healthcare has shown promising results in patient care, but the issues surrounding data privacy cannot be overlooked.
Moreover, an increasing emphasis on responsible AI development showcases a collective awareness that extends beyond mere functionality.
- Ethical Guidelines: There is a burgeoning focus on establishing robust ethical guidelines that govern AI applications. This is especially significant in sectors such as finance, where algorithmic decision-making can have far-reaching consequences.
- Interdisciplinary Research: A noticeable trend is the shift towards interdisciplinary approaches, where fields like ethics, sociology, and law converge with AI technology to shape meaningful research agendas.
As these trends unfold, it becomes necessary for stakeholders to be proactive. Keeping abreast of advancements while remaining cognizant of their implications is vital for shaping an AI landscape that serves all of humanity.
Future Directions for Research and Implementation
Future research in AI necessitates a multi-faceted approach. The next wave of advancements must incorporate ethical considerations, interdisciplinary collaboration, and regulatory frameworks.
- Regulatory Frameworks: With the rapid deployment of AI technologies, comprehensive regulatory frameworks will be essential. These frameworks will provide guidance, ensuring that AI does not operate outside the bounds of societal norms and values.
- Interdisciplinary Collaboration: Researchers from varied disciplines must work together to address the complexities surrounding AI. This can include collaborations between technologists, ethicists, social scientists, and policymakers.
- Continuous Learning: Implementing AI systems will require ongoing research to adapt to new developments or challenges. Emphasizing continuous learning will ensure that AI systems remain relevant and effective in an ever-changing landscape.
Additions to existing research, coupled with a focus on the ethical implementation of AI technologies, will foster an environment where innovation can thrive responsibly.