AI on a Chip: Revolutionizing Technology and Industries
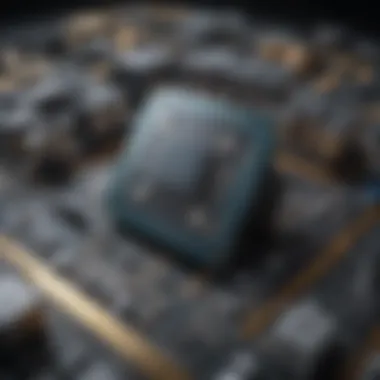
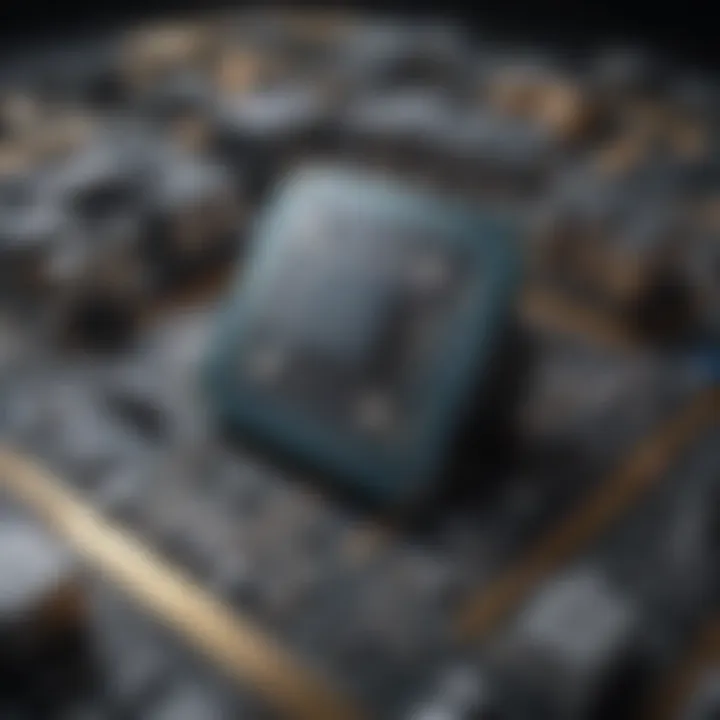
Intro
The surge in artificial intelligence (AI) capabilities has paved the way for innovative hardware, particularly through the concept of AI on a chip. This fascinating intersection of software and hardware isn’t just for tech enthusiasts; it has profound implications for industries ranging from automotive to healthcare, and beyond. By embedding AI directly onto semiconductor chips, we’re witnessing a shift in processing efficiency that redefines what is possible today, and hints at a much smarter future.
AI chips can often operate with a remarkable blend of power and efficiency, allowing complex tasks to be performed at lightning speed. This capability is reshaping how we interact with technology in our daily lives. Imagine a world where your smartphone not only responds to commands but intuitively anticipates your needs based on prior interactions. This is not the stuff of science fiction; it's rapidly becoming our reality as AI chips gain traction.
At the core of this advancement lies a wealth of research that unveils exciting technologies and methodologies, which we will unpack in detail throughout this article. Concepts that may once appeared mind-boggling are now integral to our technological fabric, making it essential to illuminate their significance.
In this discussion, we aim to break down complex subjects, ensuring that even a non-expert can grasp the relevance of AI chips in reshaping our social and professional environments.
Prelims to AI on a Chip
The emergence of artificial intelligence has greatly influenced numerous sectors, driving the need for specialized hardware solutions. Among these innovations, AI on a chip has captured significant attention. This technology merges processing power with advanced AI algorithms directly onto semiconductor chips. By doing so, it enhances the speed and efficiency of devices, making them smart enough to learn and adapt to user behavior.
Understanding AI on a chip is crucial because of its transformative implications across various applications, from smartphones to industrial automation. The goal is to reduce latency and improve real-time decision-making, enabling devices to perform complex tasks much quicker than traditional processing methods.
Benefits of AI on a Chip
To grasp the criticality of this technology, one must consider a few notable benefits:
- Performance Efficiency: Integrating AI processing onto chips streamlines operations, eliminating the lag associated with sending data to cloud servers for processing.
- Energy Savings: Chips designed for AI typically consume less power than conventional CPUs or GPUs, making them ideal for battery-operated devices.
- Scalability: AI chips can adapt to various applications, whether it's a smartphone optimizing camera settings or a factory machine enhancing predictive maintenance.
These benefits showcase how AI on a chip not only revolutionizes device functionality but also enables a level of intelligence that was previously out of reach.
Considerations Regarding AI on a Chip
While the advantages are substantial, the integration of AI on a chip also invites a set of challenges and considerations:
- Cost of Development: Developing dedicated chips often involves significant investment in research and production. This can impact pricing models in the tech industry.
- Security Risks: As with any AI technology, embedding intelligence on chip level doesn't sidestep potential security vulnerabilities.
- Compatibility Issues: Existing software may not seamlessly transition to leverage the unique capabilities of new AI chips.
By understanding not only the significance but also the intricacies surrounding AI on a chip, one can better appreciate its potential to reshape technology as we know it.
Understanding Chip Architecture
Chip architecture serves as the blueprint for how AI integrates processing capabilities within hardware. Understanding this realm is crucial for grasping how AI on a chip fundamentally alters computation efficiency and performance in various fields. The relevance of chip architecture expands beyond theoretical concepts; it manifests in tangible benefits such as optimized resource usage, reduced latency, and an overall boost to processing power.
Basic Components of AI Chips
At its core, AI chips consist of several essential components that work in harmony. These components include:
- Processor Cores: These are the engines that execute tasks, whether they are simple calculations or complex algorithms.
- Memory Units: Rapid data access is crucial for AI processing; thus, specialized memory units like HBM (High Bandwidth Memory) are often integrated.
- Interconnects: They facilitate communication between different chip components, ensuring data flows efficiently.
- Power Management Units: Effective AI chips must handle heat and power consumption efficiently to maintain performance without overheating.
Recognizing these components enables a deeper understanding of how AI chips can leverage architectures tailored to specific workloads.
Comparative Analysis: CPUs vs. GPUs vs. AI Chips
Performance Metrics
When analyzing performance metrics, one can look at various indicators such as throughput, latency, and processing speed. These metrics distinctly show how AI chips outperform traditional CPUs and GPUs in tasks tailored for artificial intelligence. The specialized nature of AI chips allows them to handle simultaneous tasks effectively, making them a go-to choice for intensive processing needs. A unique feature of performance metrics for AI chips is their ability to reach higher levels of parallel processing, compared to CPUs that often rely on sequential processing methods.
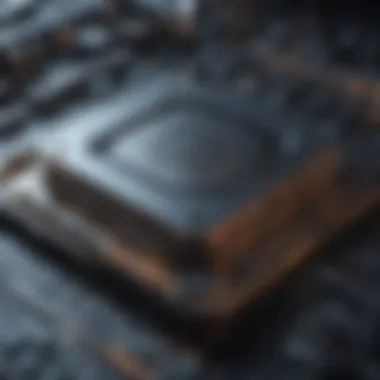
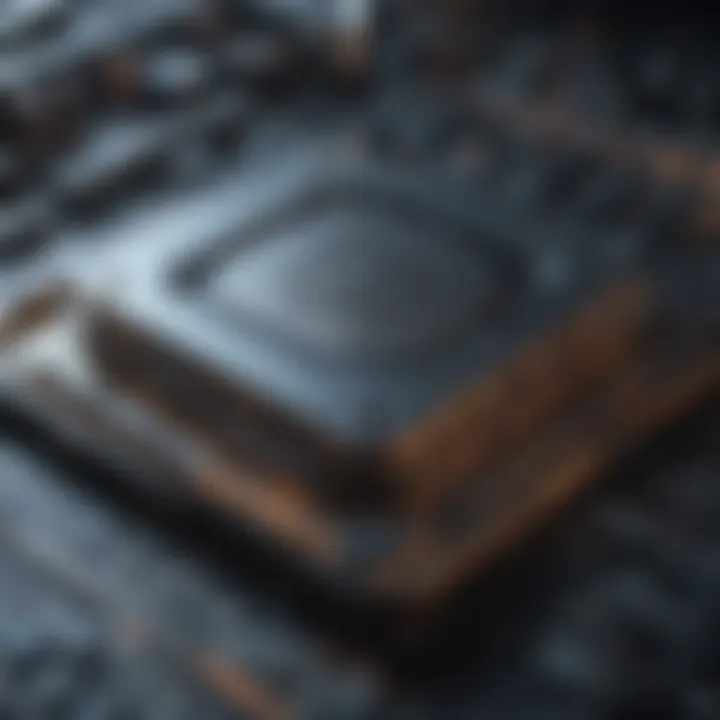
Power Consumption
Power consumption is another vital aspect of chip design, especially in mobile technologies. AI chips often consume power differently than CPUs and GPUs, which can lead to significant operational costs. AI chips are typically more energy-efficient for specific tasks, enabling longer operational times for devices powered by AI. The ability to manage power gracefully while delivering high performance is a critical advantage of AI chips over their conventional counterparts. However, utilizing these chips often requires an investment in appropriate cooling solutions to handle potential thermal outputs.
Use Cases
The use cases for AI chips vary widely, but they shine particularly in applications involving complex data processing. From powering digital assistants to enhancing machine learning algorithms in autonomous vehicles, the versatility of AI chips is remarkable. One key characteristic of these use cases is their tendency to lean toward industries requiring real-time data processing and instant feedback. The unique feature of using AI chips in these contexts is their ability to adapt rapidly to changes in data input, unlike standard processors which may falter under such conditions.
"AI chips transform the framework of computing, driving industries towards efficiency and innovation like never before."
Key Technologies in AI Chips
AI chips represent a cornerstone in the burgeoning field of artificial intelligence, significantly boosting processing power and efficiency. Understanding the key technologies that drive these chips is fundamental for grasping how they enhance both everyday tech and industrial applications. Each technology brings unique strengths and considerations that inform their use in various contexts.
Neural Processing Units (NPUs)
Neural Processing Units, or NPUs, are crafted specifically for the tasks of running AI algorithms. These chips mimic the way the human brain processes information, aiming to optimize tasks such as image and voice recognition. The importance of NPUs lies in their ability to handle vast amounts of data swiftly while maintaining low power consumption.
One of the standout features of NPUs is their parallel processing capability. Rather than executing tasks sequentially, NPUs allow for simultaneous computations, making them ideal for deep learning applications. This characteristic not only speeds up processing but also contributes to scalability; as AI models grow in complexity, NPUs can adapt efficiently.
For instance, in smartphone technology, NPUs enable features like real-time facial recognition, enriching user experience. With AI algorithms becoming more sophisticated, NPUs stand out as essential components for future innovations, ensuring that devices remain responsive and intelligent.
Field Programmable Gate Arrays (FPGAs)
FPGAs are chips that can be programmed after manufacturing, providing incredible versatility. This adaptability allows engineers to customize circuits for specific tasks, making FPGAs a strong option for diverse AI applications. The ability to reconfigure them as needs change is one major advantage, especially in research and development scenarios where requirements can evolve rapidly.
The flexibility of FPGAs offers significant benefits when it comes to prototyping and experimentation. Instead of waiting for new hardware to be manufactured, developers can quickly modify existing designs. This iterative process speeds up innovation cycles, allowing for faster testing of AI models and algorithms.
Additionally, FPGAs strike a balance between performance and power consumption. They can be finely tuned for specific tasks, and when optimized correctly, they provide compelling efficiencies that often beat traditional general-purpose processors. This makes them an attractive option for applications such as robotics, where real-time processing is critical.
Application-Specific Integrated Circuits (ASICs)
ASICs are bespoke chips designed for a particular application. Unlike NPUs and FPGAs, which can be repurposed, ASICs are one-and-done solutions. This specificity allows them to achieve peak performance for designated tasks, making them incredibly efficient at what they do.
The most common application for ASICs in AI is in training neural networks. Their design is streamlined for specific algorithms, allowing for impressive speed and efficiency in processing tasks that would otherwise take considerable time on conventional chips. For example, companies like Google have developed ASICs, such as the Tensor Processing Unit (TPU), to accelerate machine learning applications. These dedicated chips significantly reduce training times, which can lead to quicker deployments and iterations of AI models.
ASICs also have a tangible impact on hardware costs long-term. Because they consume less power than their more generalized counterparts, they help to lower operational expenses. Thus, industries pushing the boundaries of AI—be it in deep learning or data analysis—often rely on ASICs to gain a competitive edge.
"The race for better processing power in AI is not solely about raw speed, but also about efficiency, adaptability, and dedicated performance. Each technology shapes the landscape of AI capabilities."
In summary, the core technologies of NPUs, FPGAs, and ASICs each play vital roles in the evolution of AI chips. Their unique strengths contribute to a richer, more efficient environment for artificial intelligence, pushing boundaries in various sectors such as consumer electronics, automotive tech, and industrial automation.
Applications of AI on a Chip
In the contemporary landscape of technology, microchips that host AI capabilities are becoming the backbone of innovation. The applications of AI on a chip stretch across various domains, each proving essential in elevating efficiency and performance. This convergence of AI and chip technology is transforming the way industries operate and how consumers interact with devices. In this section, we elaborate on the significance of AI-chip applications for consumer electronics, automotive technology, and industrial automation.
In Consumer Electronics
Smartphones
Smartphones have adopted AI on a chip to enhance user experience significantly. With the increasing reliance on mobile devices, the integration of AI enables personal assistants like Apple's Siri or Google Assistant to function seamlessly. The key characteristic of smartphones incorporating AI is their real-time processing capabilities, which allow for speech recognition and facial recognition that feels almost instantaneous. This responsiveness makes smartphones a popular choice for users demanding not just computing power but an intuitive experience.
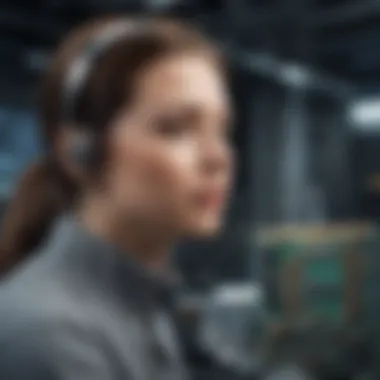
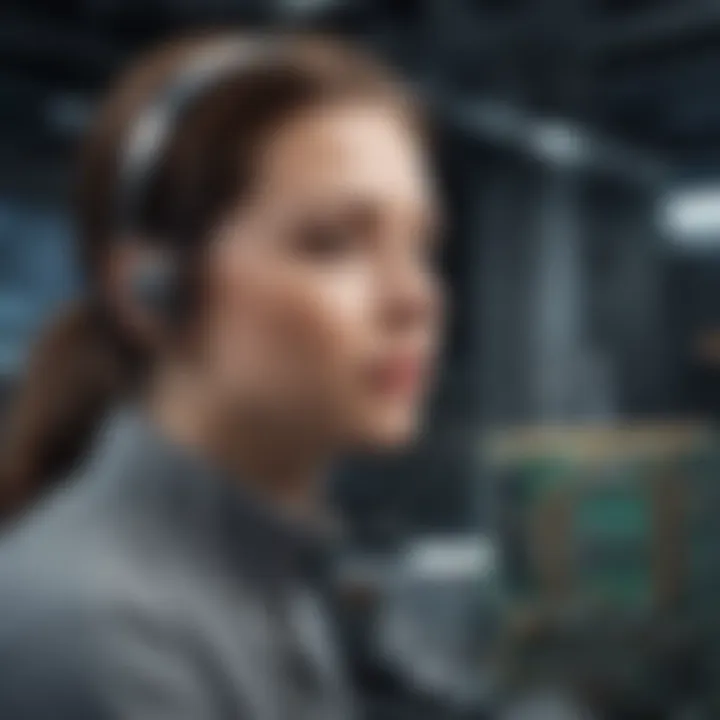
However, there are unique features that come with these advancements. For instance, AI in smartphones helps reduce battery consumption by optimizing app usage, which in turn extends the device's life without needing a charge several times a day. On the other hand, the complexity of AI algorithms can sometimes lead to occasional system slowdowns, particularly during intensive tasks.
Smart Home Devices
Smart home devices have become essential fixtures in modern living spaces, all thanks to AI on a chip. These devices, equipped with AI capabilities, can learn user preferences over time, making them not just reactive but proactive. Their ability to analyze data allows them to optimize home energy use, which is an alluring aspect for environmentally conscious consumers.
The standout characteristic here is automation. Smart home devices can manage everything from lighting to climate control based on learned behaviors. This automation, notable in products like Amazon Echo and Google Nest, provides unparalleled convenience. Yet, there is a flip side; they can raise concerns about privacy and data security as they consistently collect and process personal information to function effectively.
In Automotive Technology
Autonomous Vehicles
Self-driving cars exemplify a groundbreaking application of AI on a chip, representing a significant leap forward in automotive technology. These vehicles utilize a combination of sensors and AI chips to interpret vast amounts of data from their environment, which allows for real-time navigation and safety enhancements. The key characteristic is the ability to make split-second decisions—with precision that far surpasses human reaction times.
One unique feature of autonomous vehicles is their capacity for continuous learning through data aggregation. They can improve their performance through exposure to different driving situations over time. However, full autonomy still faces challenges such as regulatory approval and ethical considerations around decision-making in critical scenarios.
Driver Assistance Systems
Driver Assistance Systems (ADAS) are another sector where AI on a chip plays a critical role. These systems include features like adaptive cruise control, lane-keeping assistance, and automatic emergency braking. The value of ADAS lies in its potential to reduce accidents and improve driving outcomes, making vehicles safer for everyone on the road.
A key aspect of ADAS is its ability to analyze driver behavior using AI algorithms. These can predict and mitigate potential hazards even before they occur. Nonetheless, while these systems can enhance safety, they may foster a false sense of security in drivers, who might neglect their responsibilities behind the wheel, leading to overreliance on technology.
In Industrial Automation
Predictive Maintenance
Predictive maintenance embeds AI chips into machinery to forecast potential failures before they occur. This application can save companies significant amounts in downtime costs and repairs while enhancing productivity. The strength of this approach is its reliance on real-time data analysis to inform maintenance schedules, thus minimizing unexpected machine breakdowns.
The unique aspect of predictive maintenance is its integration with IoT devices, providing a comprehensive overview of equipment health. By using AI to analyze patterns, industries can act swiftly to rectify issues. Nevertheless, setting this system up requires investment in both hardware and training for staff to interpret AI-generated insights properly.
Quality Control
In manufacturing, quality control systems benefit immensely from AI on a chip. These systems leverage AI to automate inspections, ensuring that products meet stringent quality standards. The key characteristic of AI in this context is its precision and speed, as it can detect defects that the human eye might miss.
Quality control mechanisms powered by AI can lead to significant cost savings due to reduced wastage and enhanced customer satisfaction. However, integrating advanced AI systems can be a complex process, often requiring existing processes to be re-evaluated and realigned to maximize the benefits of these transformative technologies.
Challenges Facing AI on a Chip
The journey of integrating artificial intelligence directly onto semiconductor chips has not been without its stumbling blocks. Each step forward in this technology introduces a set of challenges that need to be addressed thoughtfully. Understanding these hurdles is crucial for both developers and industry stakeholders who hope to harness the full potential of AI on a chip. This section presents a closer look at three significant challenges: thermal management issues, scalability concerns, and security vulnerabilities. These challenges not only impact performance but also have broader implications for the future of AI technology.
Thermal Management Issues
Heat management is a critical aspect when it comes to AI chips. As these chips perform increasingly complex calculations at remarkable speeds, they generate significant thermal energy. This rise in temperature can lead to a range of issues from reduced performance to potential chip failure. Efficient cooling solutions are vital to ensure the longevity and reliability of AI chips. Techniques such as liquid cooling or utilizing advanced thermal interfaces are commonly employed.
Moreover, the thermal design must balance efficiency with physical size. Integrating high-performance computing with effective cooling in compact designs can feel like trying to fit a square peg into a round hole.
"Effective thermal management is not just a matter of convenience but a crucial aspect of ensuring that AI chips can deliver on their promises without infrastructural failures."
Scalability Concerns
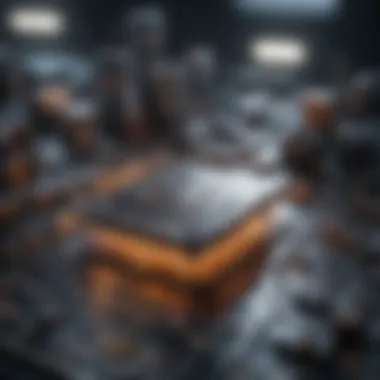
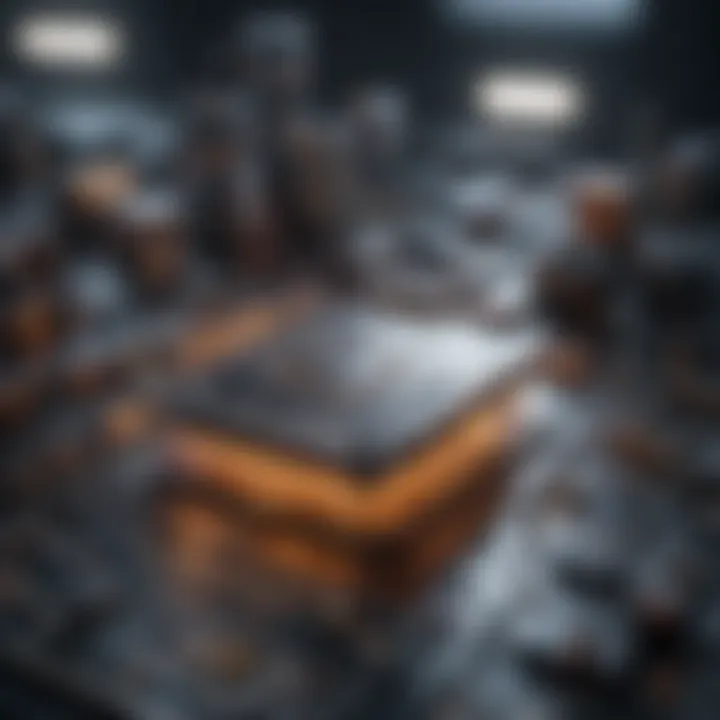
As worldwide demand for AI capabilities continues to soar, scalability presents its own set of challenges. Many manufacturers find themselves facing a race against time to keep up with this demand. AI chips must be able to support an increasing number of simultaneous processes without suffering from performance degradation. The design of the chip architecture plays an essential role here; a poorly designed chip can become bottlenecked as more tasks are loaded onto it.
Furthermore, scaling does not only mean increasing the number of chips produced. It also involves adapting the technology across various applications. A chip that works well for consumer electronics may not perform adequately in automotive contexts, or vice versa. Each application can have different requirements, making the task of creating adaptable and scalable solutions quite complex.
Security Vulnerabilities
As AI chips become more prevalent, so too do the security challenges associated with them. With their interconnected nature, AI chips can be vulnerable to various types of cyber threats. For instance, an adversary could potentially manipulate the learning algorithms, causing the AI to misinterpret data and make reckless decisions.
Additionally, securing the development tools used to create these AI applications is just as important as securing the chips themselves. As companies rush to innovate, corners might be cut, leaving potential backdoors open for exploitation. It's vital that both hardware and software security measures are taken seriously to ensure that trust is maintained in AI systems.
By addressing these three challenges—thermal management, scalability concerns, and security vulnerabilities—stakeholders can make informed decisions that help pave the way for a more reliable and robust future for AI on a chip. Understanding these potential pitfalls not only aids in the design and production of better hardware but also enhances the technology's overall functionality and public perception.
The Future of AI on a Chip
As technology races forward, the discussion surrounding the future of AI on a chip becomes paramount. The integration of intelligence at the silicon level opens up a world of potential that could redefine not just how we compute, but how we engage with several aspects of our lives. We're not merely talking about faster processors; it’s about enhanced capabilities that allow machines to learn, adapt, and respond in real time.
The Role of Quantum Computing
Quantum computing is poised to play an instrumental role in this AI evolution. Unlike classical computers, which encode information in bits, quantum devices use qubits. This means they can process complex calculations at rates that make our current technologies seem sluggish in comparison. For instance, tasks that would take traditional AI on chips weeks could potentially be completed in minutes or even seconds using quantum processing.
Moreover, the energy efficiency of quantum systems could profoundly reduce the carbon footprint of large data centers, making AI applications more sustainable. As these quantum chips are developed, companies are exploring how to integrate them with existing AI frameworks, leading to hybrid solutions that combine the best of both worlds.
Evolving Standards and Protocols
With rare exceptions, the tech world often grapples with standards. AI on a chip is no different. As various companies and open-source communities vie for a place in this burgeoning field, standards become crucial to ensuring compatibility and interoperability. The development of uniform protocols can make or break entire ecosystems.
- Collaborative Efforts: Organizations like IEEE and ISO are keenly focused on establishing these norms. This would facilitate a seamless integration of AI chips into current infrastructures.
- Security Considerations: Furthermore, as more devices become interconnected, the security surrounding AI chips needs equally rigorous attention. Developing standardized security protocols will be essential to safeguard against potential vulnerabilities.
Potential Breakthroughs in Processing Power
As innovations unfold, the landscape for AI on a chip continues to evolve. The next few years could see breakthroughs that promise to escalate the processing capabilities to levels we’ve never seen before. One potential area of growth is the implementation of neuromorphic computing, where chips mimic the way human brains process information. Such architecture could lead to remarkable advancements in problem-solving and pattern recognition.
- Enhanced Performance: As the performance of these chips improves, the applications will expand into fields previously thought unattainable, including more effective healthcare diagnostics and predictive analytics for disaster management.
- Infinity of Applications: Many industries stand to benefit, from smarter manufacturing processes to real-time language translation on-the-go. The significant uplift in processing power means AI can do more, faster, and more effectively than ever.
The future holds a promise where intelligence can be distributed and embedded at every corner of technology - a stepping stone toward smarter societies.
In sum, as we peer into the future of AI on a chip, the horizon looks incredibly bright. The convergence of quantum computing innovations, evolving protocols, and unprecedented processing power will undoubtedly catalyze a transformation across multiple domains. As researchers, educators, and industry professionals navigate this landscape, the ability to adapt and integrate these advancements will determine success.
The End
In wrapping up our discussion on the transformative power of AI on a chip, it is crucial to recognize the significance of this technology in shaping the future of various industries and everyday applications. As detailed earlier, AI chips represent a significant leap forward in computing efficiency and performance, integrating specialized processing power directly onto hardware. This warrants consideration across sectors such as automotive, healthcare, and smart devices, where the demands for rapid, intelligent processing are increasing.
Summary of Key Findings
The exploration of AI on a chip has revealed several pivotal insights:
- Efficiency Gains: Integrating AI capabilities directly onto chips facilitates faster processing and reduced latency, essential in real-time applications like autonomous driving and predictive analytics.
- Cost Effectiveness: By optimizing hardware for AI workloads, businesses can reduce operational costs with energy-efficient solutions, making advanced technology more accessible.
- Wider Adoption: The rise of consumer electronics embedded with AI chips indicates a clear trend towards widespread tech adoption, enhancing user experiences in smartphones, smart speakers, and home devices.
"AI chips not only enhance processing speeds, but they also create a more responsive interaction with technology, pushing boundaries of what is possible."
Final Thoughts on AI Hardware Trends
As we venture into the future, the integration of AI on a chip will undoubtedly progress in tandem with advancements in machine learning and data processing. Future trends suggest:
- The increasing importance of quantum computing as a complementary technology for enhanced processing power.
- The development of evolving standards and protocols will refine how chips interact and communicate, fostering better synergy between devices.
- Anticipating breakthroughs in architecture could lead to the emergence of more sophisticated AI capabilities, positioning AI chips at the forefront of the tech industry.
Ultimately, the conversation around AI on a chip is not just about enhancing existing systems but also about paving the way for innovative solutions that we have yet to imagine.