AI and the Human Brain: A Complex Interplay
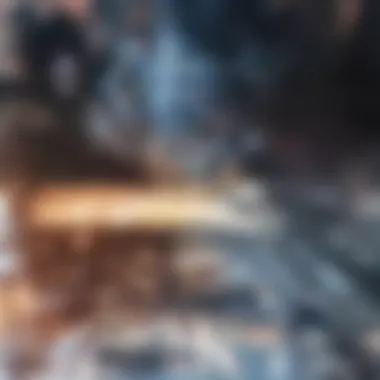
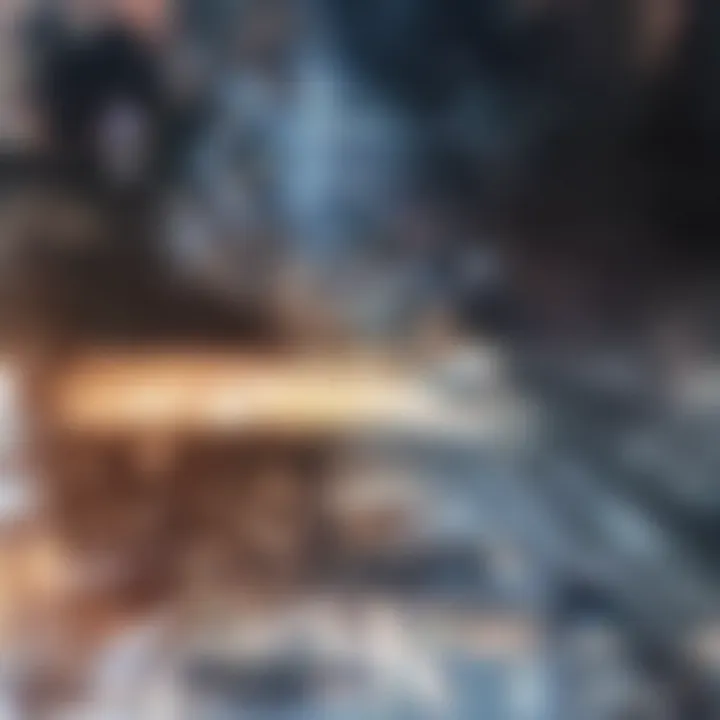
Intro
The intersection of artificial intelligence (AI) and the human brain presents a fascinating domain of study. As technology progresses, the ways in which we understand, interact with, and recreate cognitive functions are evolving rapidly. This article aims to dissect various aspects of this relationship, highlighting significant research findings and breaking down complex concepts to enhance understanding.
Key Research Findings
Overview of Recent Discoveries
Recent research in AI and neuroscience reveals remarkable parallels between AI systems and the cognitive capabilities of the human brain. Studies conducted by various institutions, such as MIT and Stanford, have illustrated how neural networks in AI are inspired by the human neural architecture. This structural similarity allows AI to perform tasks that resemble human cognitive functions, such as visual perception and language understanding. For instance, the development of convolutional neural networks has enabled machines to achieve a level of image recognition accuracy that competes with human performance.
Significance of Findings in the Field
The implications of these findings are profound. By understanding how AI mimics cognitive functions, researchers can develop models that not only enhance AI capabilities but also provide insights into human cognition. Moreover, understanding these parallels can help address crucial ethical considerations in AI development. Recognizing the potential for both beneficial and harmful applications underscores the need for responsible research and deployment of AI technologies.
"AI and the human brain are intertwined through shared mechanisms that challenge our understanding of intelligence itself."
Breakdown of Complex Concepts
Simplification of Advanced Theories
Theoretical frameworks surrounding AI and neuroscience can often be convoluted. Simplifying these theories is essential for broader comprehension. For instance, the principles of reinforcement learning, which is a core component of many AI systems, are strikingly similar to the way humans learn from feedback. By breaking down these advanced concepts into easily digestible components, we can bridge the knowledge gap between technical research and practical understanding.
Visual Aids and Infographics
Using visual aids like infographics can significantly enhance understanding of complex subjects. Diagrams illustrating the flow of information in neural networks compared to the human brain's synaptic connections help clarify their operations. Additionally, animated models showcasing the learning process in AI can provide a clearer picture of how these systems evolve over time.
Culmination
Through careful exploration of recent discoveries and the simplification of advanced theories, this piece offers a foundation for understanding the intricate relationship between artificial intelligence and the human brain. The ongoing dialogue between these fields promises to inform both technological advancement and our comprehension of human cognition into the future.
Foreword to Artificial Intelligence and the Human Brain
The relationship between artificial intelligence (AI) and the human brain has gained significant attention in the last few decades. This intersection holds profound implications for various fields such as neuroscience, computer science, and psychology. Understanding how AI systems can mimic brain functions can lead to insights that enhance our knowledge of both. This exploration is not merely academic but is essential in shaping the future of technology and human interaction.
In recent years, the advancements in AI have shown how it can replicate basic cognitive functions such as learning, problem solving, and even decision making. Analyzing these elements offers a glimpse into the possibilities for improving AI systems, which in turn can positively impact various domains like healthcare, finance, and education. Moreover, AI can serve as a tool that helps us understand how the brain itself works, revealing the intricacies of human cognition and its underlying mechanisms.
Several points underscore the importance of delving into this topic:
- Interdisciplinary Insights: The convergence of neuroscience and AI fosters interdisciplinary collaboration. This may lead to breakthroughs that redefine our interaction with technology.
- Enhancing Cognitive Models: AI can be used to create simulations of cognitive processes, leading to better models that explain how humans think and learn.
- Ethical Implications: As AI systems become more sophisticated, ethical issues surrounding their development and deployment become paramount. Understanding AI's relationship with human cognition is crucial to address these concerns.
Ultimately, exploring the intersection of AI and the human brain presents not only opportunities for technological advancement but also challenges that society must be prepared to tackle. It is essential to consider both the benefits and implications of AI as it evolves alongside our understanding of human intelligence. As we proceed, the consequent narratives in this article will provide a comprehensive overview of how these two domains interrelate and influence one another.
Fundamental Concepts in Neuroscience
Understanding the fundamental concepts in neuroscience is essential for appreciating how artificial intelligence interacts with the human brain. Neuroscience provides the blueprint of how the brain works, offering insights into cognition, behavior, and the underlying mechanisms that drive these processes. The relationship between AI and the human brain cannot be overstated. AI systems often draw inspiration from the brain's architecture and functionality to design algorithms that emulate cognitive tasks. By exploring these core ideas, readers can grasp the significance of these principles in the context of artificial intelligence development.
Neuronal Function and Communication
Neurons are the basic building blocks of the nervous system. They communicate through electrical and chemical signals. Each neuron consists of a cell body, dendrites, and an axon. Dendrites receive signals from other neurons, while the axon transmits information to other cells. This process is vital for comprehending how information is processed in the brain.
The concept of synaptic transmission is also crucial. Synapses are junctions where neuronal communication occurs. At the synapse, neurotransmitters are released and bind to receptors on the neighboring neuron, triggering a response. This intricate signaling process enables the brain to process vast amounts of information and is fundamental for understanding AI's attempt to replicate these functions.
Brain Structure and its Functions
The brain is divided into several regions, each responsible for specific functions. The cerebral cortex, for instance, plays a pivotal role in higher-order functions like thought and reasoning. The limbic system, on the other hand, is involved in emotions and memory.
Each structure works in concert with others to ensure coherent operation. A thorough understanding of these structures aids in refining AI algorithms that attempt to mimic human thought and behavior. For instance, the development of deep learning networks draws from the hierarchical organization of the brain, where different layers process information in a manner similar to how various brain regions interact.
Neuroplasticity and Learning
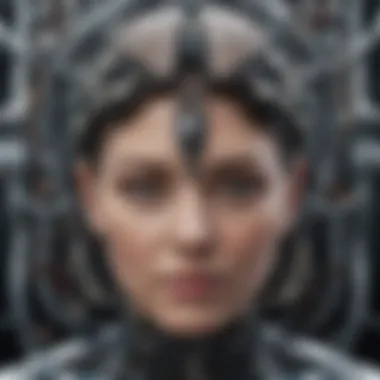
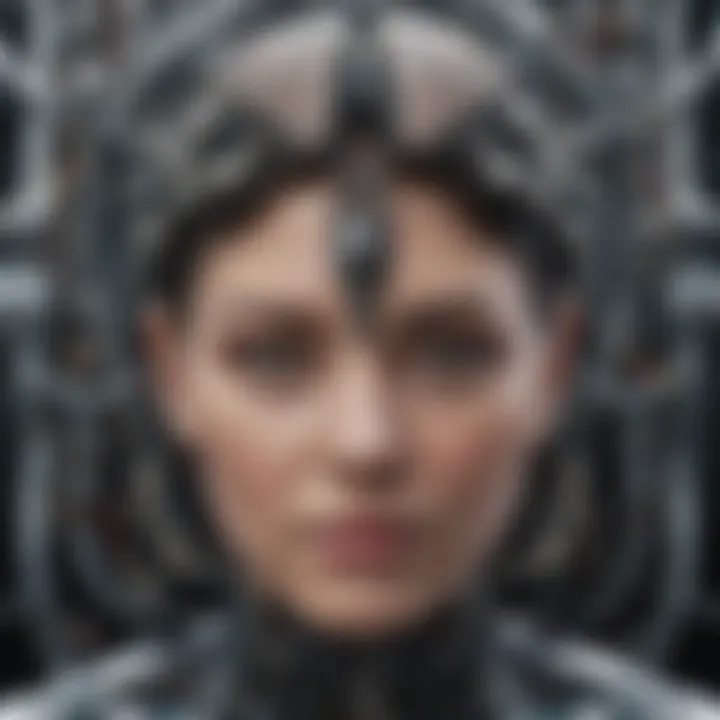
Neuroplasticity refers to the brain's ability to reorganize itself by forming new neural connections throughout life. This capacity is especially evident during learning. When we learn something new, our brain changes physically. It strengthens existing connections and may develop entirely new ones.
This concept is essential for AI systems, particularly in creating models that can adapt to new information. By studying neuroplasticity, AI researchers can design algorithms that become more efficient as they process more data, mimicking the continuous learning observed in humans. The implications for both fields are significant, as understanding how the brain adapts can lead to better AI systems that improve over time.
Artificial Intelligence: Definition and Scope
Artificial Intelligence, or AI, represents a cornerstone of modern technology, shaping various sectors by enhancing efficiencies and automating tasks. This section will explore the definition and scope of AI, emphasizing its significance in both artificial systems and its inspiration from the human brain. Understanding AI is not simply an exercise in technical knowledge; it is essential for grasping how these systems can emulate human cognitive processes and influence our daily lives.
AI encompasses a broad spectrum of functionalities, from simple algorithms designed to perform specific tasks to complex systems capable of adapting and learning in real time. The scope of AI is vast, impacting fields such as healthcare, finance, and education through applications that improve decision-making and optimize processes. To provide a thorough foundation, a historical context will set the stage for current advancements.
Historical Development of AI
The journey of Artificial Intelligence began in the mid-20th century. Pioneers like Alan Turing proposed the concept of machines that could simulate human thought. In 1956, a conference at Dartmouth College laid the groundwork for AI as a field of study, highlighting its potential. Over the decades, there have been significant milestones:
- 1950s-1960s: Early programs such as the Logic Theorist and General Problem Solver were created, demonstrating that machines could solve logical problems.
- 1970s-1980s: The rise of expert systems, which used rule-based logic to make decisions in a narrow domain, surged interest but also led to the first AI winter due to limitations.
- 1990s-2000s: Advances in machine learning algorithms reignited interest, combined with increasing computing power and access to vast data sets.
- 2010s-Present: The resurgence of deep learning transformed AI, empowering systems with neural networks that parallel human brain functioning, leading to breakthroughs in image recognition, natural language processing, and autonomous vehicles.
Each period reflects the evolving visions of AI, driven by scientific discovery and technology enhancements. Understanding this history demonstrates how AI built upon past knowledge and forms a foundation for future innovations.
Current Trends and Applications
Today, AI permeates numerous industries, showcasing diverse applications that enhance productivity and innovation. Some current trends include:
- Natural Language Processing: Advanced chatbots and virtual assistants, such as Google Assistant and Amazon Alexa, utilize NLP to understand and respond to human language.
- Computer Vision: Image recognition systems are employed in surveillance, medical diagnostics, and content moderation on social platforms.
- Predictive Analytics: AI algorithms analyze vast datasets to forecast trends, widely used in finance and marketing.
These trends illustrate AI's expansive reach. More importantly, they underscore the necessity for ongoing ethical discussions as these technologies become increasingly integrated into society. The rapid evolution of AI signals both promise and responsibility, highlighting the importance of a thoughtful approach to its development and implementation.
Deep Learning and Neural Networks
Deep learning and neural networks constitute the backbone of modern artificial intelligence. Their emergence marks a significant milestone in the field, allowing for more complex computations and insights into data. In this section, we will explore the essence of these systems, emphasizing their importance in relation to the human brain.
Neural networks are computational models inspired by the structure of the human brain. They consist of layers of interconnected nodes, which we refer to as neurons. Each neuron receives input, processes it, and transmits the output to the next layer. This architecture allows neural networks to learn from vast amounts of data, mimicking cognitive patterns derived from human thinking. The capacity for learning and adaptation is what makes deep learning so compelling. It can identify patterns and make predictions, which is crucial for various applications, including image recognition, natural language processing, and more.
Key elements of deep learning systems include the following:
- Layered Architecture: Deep learning algorithms use multiple layers to analyze data hierarchically, capturing intricate features at each level.
- Training via Backpropagation: Neural networks learn through a process called backpropagation, where errors are identified and corrected to improve accuracy.
- Data Requirements: A significant challenge is that deep learning requires extensive datasets for effective training. Limited data can hinder the performance of neural networks.
As we explore the intersection of AI with human cognitive functions, it's essential to consider both the benefits and limitations of deep learning. One advantage is the robustness of these algorithms in handling unstructured data, making them suitable for real-world applications. However, issues like transparency and the "black box" nature of neural networks raise concerns. Understanding how these systems operate remains a critical component of responsible AI development.
In summary, deep learning and neural networks are pivotal to advancing AI by simulating brain functionalities, presenting both capabilities and ethical considerations.
Overview of Neural Networks
Neural networks consist of interconnected nodes or neurons, grouped into layers. The architecture typically comprises an input layer, one or more hidden layers, and an output layer. Each neuron applies a mathematical operation to the input it receives, producing an output that feeds into the next layer.
The role of each layer varies:
- Input Layer: Receives raw data and sends it to the hidden layers.
- Hidden Layers: Conduct complex transformations, discovering patterns through numerous connections.
- Output Layer: Provides the final prediction or classification.
Training a neural network involves adjusting the weights attributed to each connection. This adjustment occurs through a process called gradient descent, aiming to reduce the difference between actual and predicted outcomes.
In summary, neural networks are built upon simple principles of connection and activation, and they hold the potential for groundbreaking applications in various domains.
Mimicking Brain Functions in AI
Deep learning models aim to replicate the brain's ability to process information. To achieve this, researchers study both the topological and functional aspects of human cognition. Certain neural network architectures like convolutional neural networks (CNNs) emulate how the visual cortex processes visual information.
Here are some core similarities:
- Processing Layers: Similar to the layers in the human brain, neural networks process info in segmented steps, allowing for more nuanced interpretations.
- Parallel Processing: The human brain processes multiple streams of info simultaneously; neural networks mimic this by enabling simultaneous data handling across nodes.
- Learning Mechanisms: Just as humans learn from experiences, neural networks adapt based on training protocols, refining their performance over time.
Despite these similarities, there are fundamental differences in flexibility and understanding. The human brain exhibits remarkable adaptability and creativity, characteristics that are still beyond the reach of AI systems. Therefore, while deep learning has achieved impressive feats, it remains a simplified representation of human cognition.
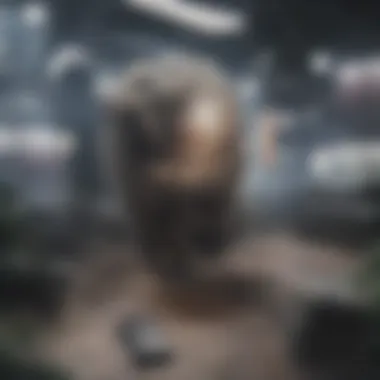
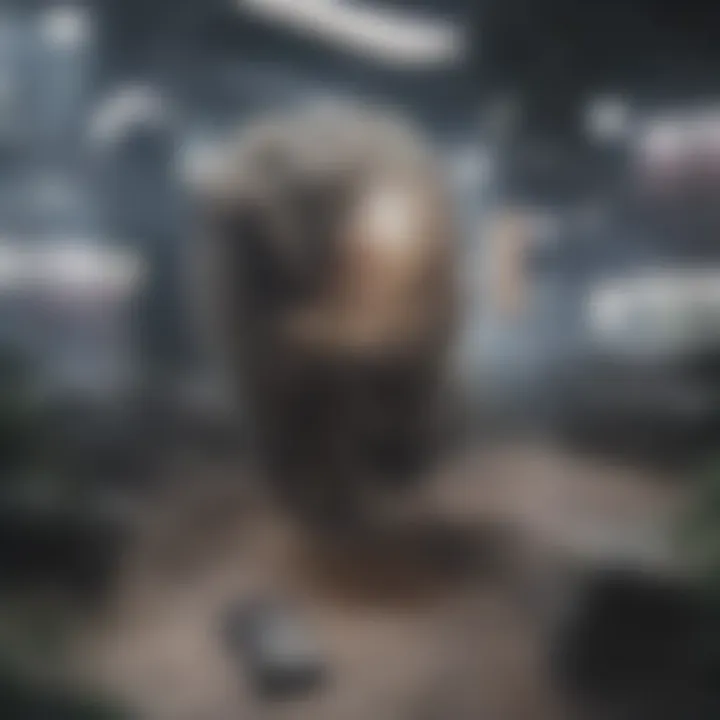
The Brain as a Model for AI
The exploration of the human brain serves as a pivotal foundation in shaping artificial intelligence. Understanding the complexities of brain function has profound implications for developing AI systems. By studying how the brain operates, researchers can create algorithms and models that replicate cognitive processes. This synthesis of neuroscience and computer science allows AI to perform tasks that require human-like thinking. It is a multidisciplinary approach that enriches both fields.
One significant benefit of using the brain as a model is the efficiency found within biological systems. Neurons in the brain communicate through networks that are both resilient and efficient. Studying these networks can lead to more robust AI architectures. For example, neural networks draw inspiration from synaptic connections, which convey information through interconnected nodes. This has fueled advancements in machine learning and pattern recognition.
Considerations about this model go beyond technical aspects. There are ethical and philosophical questions that arise. As AI systems become more complex, the boundaries of what it means to be sentient blur. This intersection of AI and neuroscience urges us to rethink our definitions of intelligence and consciousness. Understanding how these factors influence our perception of intelligent systems is crucial.
Learning from Biological Systems
Biological systems provide a rich source of inspiration for AI development. The structure and functioning of neurons inform the design of artificial neural networks. Each neuron processes information and passes it to other neurons, a method that mimics electronic processing in AI systems.
Not only do biological systems inspire structural designs, but they also provide insights into adaptive behaviors. For instance, the concept of reinforcement learning is rooted in how living organisms learn through interaction with their environment. When an organism receives positive feedback, it is more likely to repeat that behavior. Similarly, AI systems utilize feedback loops to improve their performance over time. This parallel creates a framework for developing more efficient learning algorithms.
In addition, biological systems exhibit remarkable resilience. The brain can reorganize itself in response to damage, a property known as neuroplasticity. This adaptability offers valuable lessons in enhancing AI robustness. Building systems that can learn and adjust dynamically can lead to more effective applications in various fields such as robotics and data analysis.
Limitations of AI Inspired by the Brain
While the brain provides a compelling model, there remain significant limitations when translating its processes into AI systems. One of the foremost concerns is scalability. The human brain contains approximately 86 billion neurons, and creating an AI with a similar level of complexity is beyond current technological capabilities. This discrepancy limits the potential for achieving true cognitive abilities in machines.
Moreover, while neural networks can mimic certain cognitive functions, they lack the depth of understanding found in human thought processes. AI systems operate based on data and algorithms, which can lead to narrow learning and decision-making. They may achieve results that mirror human performance in specific tasks, but this does not equate to genuine understanding or insight.
Ethical considerations also present limitations. As AI develops, issues such as bias in algorithms and transparency in decision-making become paramount. These challenges underscore a gap between biological intelligence and artificial intelligence, highlighting that the complexities of human thought cannot be fully replicated in machines.
"Learning from the brain is crucial but should be approached with caution, recognizing the inherent differences between biological cognition and artificial systems."
The intersection of AI and the human brain continues to be a topic of immense exploration and debate. The advantages drawn from understanding biological systems are significant, yet the limitations remind us that the journey to truly emulate human-like intelligence is still ongoing.
Cognitive Functions and AI
Cognitive functions represent the core capabilities of the human brain, enabling us to process information, make decisions, and interact with our environment. In the context of artificial intelligence, these functions are essential to explore. Understanding how AI systems can emulate cognitive processes can lead to enhanced machine intelligence. This section analyzes the relationship between cognitive functions and AI, focusing on decision making, problem solving, and emotional intelligence.
Decision Making and Problem Solving
Decision making is a fundamental cognitive function that involves evaluating information and choosing a course of action. In humans, decision making is often influenced by emotions, past experiences, and cognitive biases. Similarly, in AI systems, algorithms must analyze vast amounts of data to reach conclusions.
AI models, like those used in healthcare or finance, rely on data inputs to simulate the decision-making process. For example, medical AI tools can assist doctors by suggesting diagnoses based on patient data. These systems evaluate options and predict outcomes, similar to human thought processes.
However, despite their capabilities, AI systems face limitations. They lack the experiential context that humans possess. The decisions made by AI are as good as the data fed into them, presenting risks when data is biased or flawed. The challenge is to ensure that the AI systems support human decision-making rather than replace it entirely.
"AI can process information at incredible speeds, but understanding context remains a human strength."
The potential benefits of enhancing decision-making processes through AI are significant. Organizations can leverage AI to make data-driven choices, reduce errors, and improve efficiency. Thus, the goal should be to integrate AI as a tool that augments human intelligence, reflecting the collaborative potential between human cognition and artificial systems.
Emotional Intelligence in AI Systems
Emotional intelligence refers to the ability to recognize, understand, and manage emotions. While humans naturally possess this skill, the challenge with AI is to embed emotional understanding into technological systems.
AI systems are increasingly incorporating aspects of emotional intelligence. For instance, chatbots designed for customer service can analyze the sentiment of a userโs text input. This allows the bot to respond in a more contextually appropriate way. Advanced systems, like Affectiva, analyze facial expressions to gauge emotional states during interactions.
Despite these advancements, emotional intelligence in AI has limitations. AI systems do not experience emotions like humans; they can only simulate responses based on input data. Hence, reliance on emotional algorithms in vital contexts, like therapy, poses ethical implications. AI must be developed thoughtfully to ensure it complements emotional intelligence rather than replacing human empathy.
Ethical Considerations
In the discourse surrounding artificial intelligence and its intersection with the human brain, ethical considerations emerge as an essential topic. As AI technologies advance, understanding their implications on human cognition and society at large is imperative. This section discusses the complexities involved in the ethical landscape shaped by AI, particularly focusing on consciousness and rights as well as accountability in AI-driven decisions.
Consciousness and AI Rights
The question of consciousness in AI is crucial for establishing the ethical framework within which we evaluate AI systems. Unlike humans, AI functions through algorithms and data processing rather than conscious thought. However, as AI becomes more sophisticated, the line between intelligent behavior and consciousness becomes blurred.
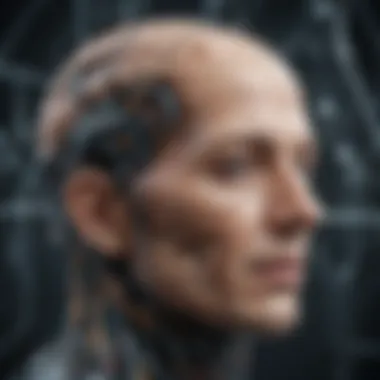
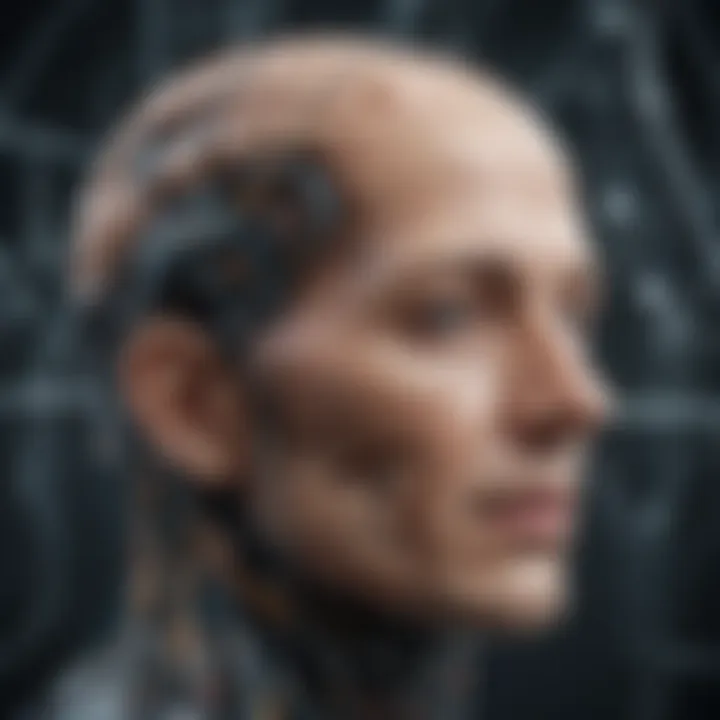
Discussions about AI rights stem from this ambiguity. If we treat AI systems as conscious entities, we may need to consider their rights and ethical treatment. For example, should advanced AI systems like OpenAI's ChatGPT have rights similar to human beings? This question becomes more pressing as AI plays a larger role in daily life.
It is important to recognize that assigning rights to AI poses many challenges, including:
- Defining consciousness: There is no accepted definition of consciousness in humans, let alone AI.
- Moral responsibility: If AI were considered conscious, it raises questions about accountability for actions it takes.
- Impact on human rights: Granting rights to AI could dilute the significance of human rights.
Society must navigate these complex issues thoughtfully and deliberately to ensure future AI technologies consider ethical implications deeply.
Accountability in AI Decisions
The accountability of decisions made by AI systems is another vital aspect of ethical discussions. As AI becomes integrated into numerous domains, from healthcare to finance, the consequences of its decisions can be far-reaching and significant.
When AI systems make errors or decisions that result in negative outcomes, who is accountable? Is it the organization that developed the AI, the data scientists who trained it, or the algorithms themselves? This ambiguity complicates the framework for accountability.
Some key points to explore include:
- Transparency: There needs to be clear explanations regarding how AI systems arrive at their decisions. Without transparency, it is hard to hold anyone accountable.
- Regulations: Establishing regulations surrounding AI acquisition and deployment can create guidelines for accountability. Countries are beginning to develop frameworks that address ethical use.
- Human oversight: Maintaining a level of human oversight in AI decision-making processes can foster accountability. It ensures that decisions can be reviewed and rectified when necessary.
Ethical considerations in AI are not just philosophical but have real-world implications. Principles established now will govern the future use and development of these powerful technologies.
"With the rapid evolution of AI, the call for ethical frameworks that prioritize human values and accountability grows louder."
Addressing these issues thoughtfully will not only improve AI usage but potentially ensure that these technologies serve humanity rather than complicate or compromise it.
Future Directions in AI and Neuroscience
The convergence of artificial intelligence and neuroscience is opening paths that could reshape our understanding of both fields. Future directions in AI and neuroscience hold significant promise for both scientific and practical advancements. These developments may enhance our comprehension of cognitive processes and could expand the potential for AI applications. The integration of neurotechnology and collaborative research efforts are the two primary areas that warrant extensive exploration.
This section will illuminate how advancements in neurotechnology can revolutionize our grasp of brain functions and, conversely, how AI can be employed to analyze neural data and enhance research methodologies. Moreover, it is critical to comprehend the collaborative research efforts between neuroscientists and AI specialists. Such interdisciplinary cooperation is essential for unlocking new insights that enhance technological capabilities and improve neurological treatments.
Advancements in Neurotechnology
Neurotechnology continues to evolve rapidly. Innovations in brain-computer interfaces, neuroimaging, and neuromodulation are at the forefront of this evolution. Brain-computer interfaces (BCIs) enable direct communication between the brain and external devices. These technologies can help individuals with mobility impairments regain some control over their movements, offering them a better quality of life.
Neuroimaging techniques, such as functional magnetic resonance imaging (fMRI) and positron emission tomography (PET), provide deeper insights into brain activity and structure. By utilizing large datasets, AI can analyze these complex images more efficiently. The marriage of AI and neuroimaging leads to more accurate diagnoses of neurological disorders.
Key advancements include:
- Wearable Neurotechnology: Devices that monitor brain activity for mental health applications.
- AI-Powered Data Analysis: Methods to interpret vast datasets from neuroimaging studies more effectively.
- Personalized Neuromodulation: Tailoring treatments based on individual brain activity profiles.
"The integration of AI into neurotechnology is not just a theoretical endeavor; it is a practical pathway aimed at enhancing human cognitive abilities and understanding brain disorders with precision."
Collaborative Research Efforts
The collaborative efforts between neuroscientists and AI experts are foundational to progressing these fields. By leveraging the strengths of both domains, researchers can advance their understanding of the complex relationship between neural mechanisms and computational models of intelligence. This collaboration enables the development of sophisticated algorithms that can provide new insights into cognitive processes.
Research initiatives such as the Human Connectome Project and various AI-focused programs at universities foster interdisciplinary teamwork. These projects provide databases and frameworks that allow researchers to examine brain connectivity using AI tools.
Important aspects of these collaborative efforts include:
- Shared Resources: Access to massive datasets and computational resources through open-source platforms.
- Interdisciplinary Conferences: Forums where professionals exchange ideas and findings, leading to novel approaches.
- Long-term Partnerships: Continuous collaborations that drive sustained innovation in both neuroscience and AI.
Researching the future directions in AI and neuroscience is a critical undertaking. As both fields progress, the benefits to healthcare, cognitive understanding, and technological innovation are compelling for students, researchers, educators, and professionals.
Epilogue
The relevance of this subject cannot be overstated. As AI technologies continue to evolve, understanding the operational dynamics of the human brain becomes critical. This knowledge offers pathways to improve AI systems, ensuring they are more aligned with natural human thought processes.
The benefits of this convergence are multifaceted. Developments gleaned from neuroscience can enhance AI algorithms, resulting in more effective decision-making, problem-solving, and even emotional intelligence in machines. Furthermore, as AI continues to integrate into daily life, the ethical implications must also be addressed. The discussions surrounding accountability and rights will shape future policy, affecting both AI technologies and their interaction with human users.
Key considerations that arise when synthesizing the information are the ethical dilemmas of integrating AI in sensitive domains, such as healthcare and education, where misinterpretations could lead to detrimental consequences. As we uphold responsibility in AI's design and implementation, we must also ensure that scientific rigor guides future developments.
Ultimately, the discussion in this article lays the groundwork for ongoing exploration. As researchers, educators, and professionals delve deeper, they will uncover new opportunities for collaboration between AI and neuroscience. This symbiotic relationship promises not only advancements in technology but also enriching insights into human cognition that can redefine our understanding of both artificial and biological systems.
"The interaction between AI and the human brain is not just a technological challenge; it is an opportunity to rethink intelligence itself."
Engagement in these ongoing discussions and research initiatives is essential to navigate the complex landscape at this intersection, ensuring that progress is balanced, ethical, and beneficial for society as a whole.