Synthetic Intelligence: An In-Depth Exploration
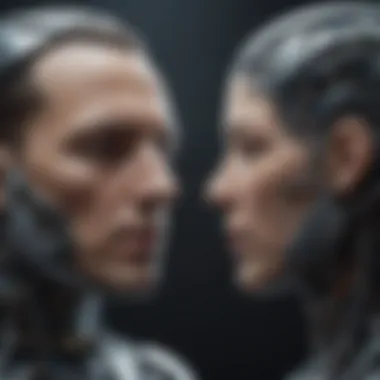
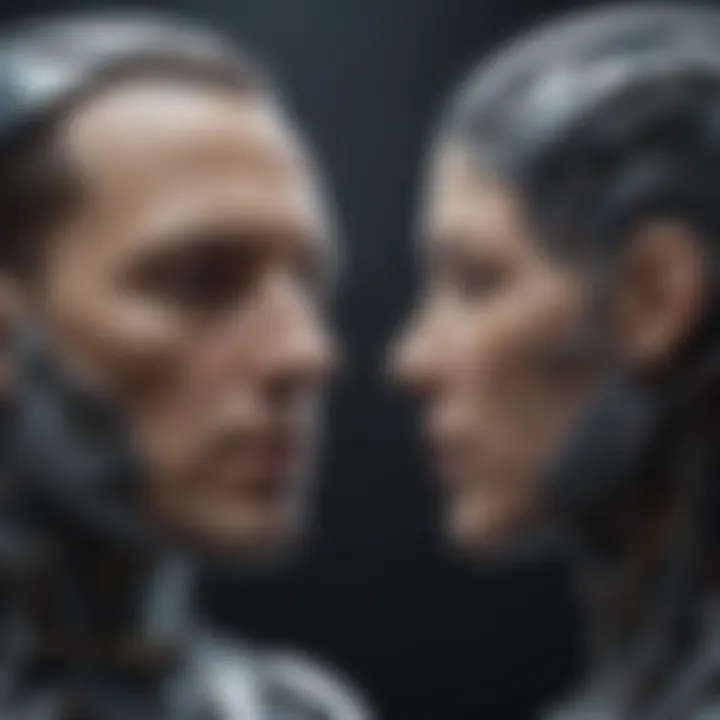
Intro
Synthetic intelligence is a rapidly evolving field, garnering attention due to its potential to transform various sectors. Unlike traditional artificial intelligence, which primarily aims to replicate human-like behavior, synthetic intelligence seeks to create systems that function through unique processes and methodologies. This nuanced difference is crucial for understanding its implications in our technological landscape.
Researchers are making significant strides in this area, applying synthetic intelligence to practical challenges in healthcare, education, and beyond. The focus isn't just on developing smarter algorithms but on conceptualizing an entirely different approach to problem-solving through synthetic paradigms.
In this exploration, we will dissect the core elements that define synthetic intelligence, its foundational principles, essential methodologies, and the ethical considerations that come into play. Understanding these aspects will provide clarity on how synthetic intelligence can be positioned as a disruptive force in both society and the economy.
Key Research Findings
Overview of Recent Discoveries
Recently, several breakthroughs have been identified in synthetic intelligence.
- Data Integration Techniques: Improved methods for unifying diverse data sources are enhancing the intelligence of systems.
- Autonomous Learning Models: Algorithms that allow systems to learn from their environment without human intervention have shown promising results.
- Real-World Applications: Pilot projects in healthcare are integrating synthetic intelligence to optimize patient care and resource management.
These discoveries highlight the rapid advancement in this field, providing a grasp of how synthetic intelligence can outpace conventional AI technologies.
Significance of Findings in the Field
The research outcomes hold significant implications:
- Enhanced Decision-Making: Synthetic intelligence systems can assess complex data sets to boost efficiency and accuracy in decision-making processes.
- Interdisciplinary Benefits: The technologies developed contribute to various fields, blending insights from computer science, neuroscience, and social sciences.
- Ethical Implications: The expansion of synthetic intelligence raises critical questions about moral responsibilities in AI development and application, including accountability and transparency.
These findings suggest a pivotal shift in how we view and utilize machine intelligence, underscoring the necessity for rigorous ethical frameworks to ensure responsible advancement.
Breakdown of Complex Concepts
Simplification of Advanced Theories
Synthetic intelligence revolves around several advanced theories that may appear complex at first. Simplifying these concepts can facilitate better understanding:
- Coactive Learning: This model promotes collaboration between humans and machines, enhancing outcomes by combining strengths.
- Generative Adversarial Networks (GANs): A cutting-edge method where two neural networks compete to improve the realism of outcomes. This is instrumental in creating models that closely resemble real-world scenarios.
Visual Aids and Infographics
Visual representation of data is essential for comprehending complex ideas in synthetic intelligence. Infographics can depict:
- Comparison: A side-by-side examination of synthetic intelligence versus traditional AI.
- Data Flow: How information moves through a synthetic intelligence framework.
- Case Studies: Real-world applications and their outcomes.
Utilizing these visual tools can help engage audiences more effectively, ensuring the information is digestible. Additionally, incorporating relevant links and further reading materials can enhance the learning experience.
"As synthetic intelligence evolves, understanding its foundations and implications is essential for both innovators and ethical guardians of technology."
Through the exploration of these components, one can appreciate the comprehensive landscape of synthetic intelligence and its emerging role in shaping our future.
Prolusion to Synthetic Intelligence
Synthetic intelligence, a term increasingly used in the discourse surrounding advanced computational systems, warrants a closer examination. Understanding this concept is crucial for grasping the broader implications these technologies may have. Unlike traditional artificial intelligence, synthetic intelligence focuses on creating systems that do not merely simulate human-like behavior but rather replicate underlying processes of intelligence itself.
The significance of synthetic intelligence lies in its potential applications across various fields such as healthcare, environmental science, and industrial processes. The benefits are manifold. For instance, in healthcare, synthetic intelligence can facilitate quicker diagnosis and personalized treatment plans. By recognizing the nuances of synthetic intelligence, professionals in diverse fields can leverage its capabilities to enhance productivity, efficiency, and innovative solutions to complex problems.
Several considerations arise within the conversation of synthetic intelligence. These include ethical implications, privacy concerns, and the balance between technological advancement and societal impact. Addressing these considerations enables researchers and practitioners to navigate potential pitfalls while harnessing the transformative power of these intelligent systems.
Defining Synthetic Intelligence
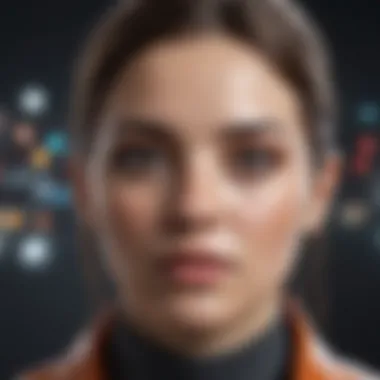
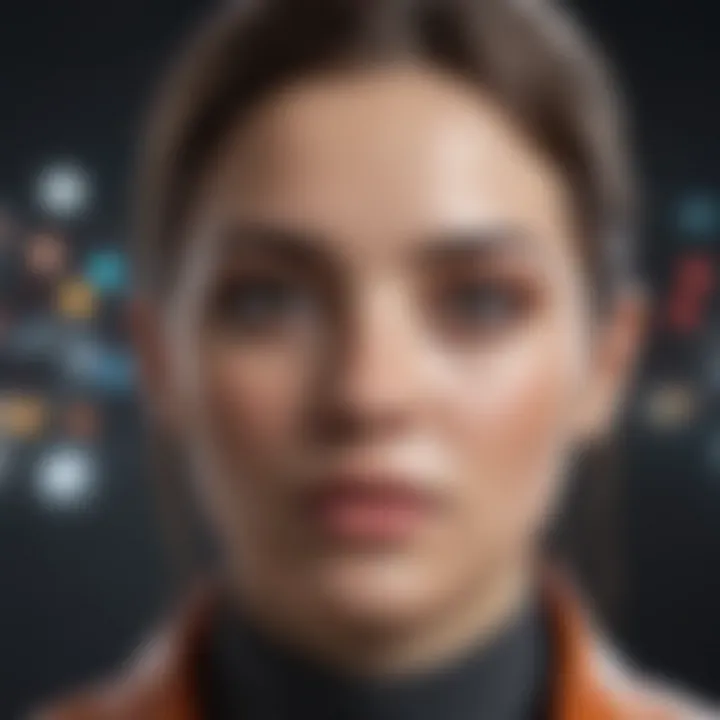
Defining synthetic intelligence involves understanding its core attributes and how it differs from artificial intelligence. Synthetic intelligence encompasses a broader approach to the design of intelligent systems, emphasizing the replication of cognitive processes that characterize human reasoning and learning.
In essence, synthetic intelligence seeks to create systems that can interact with their environment, make decisions, and adapt over time, much like humans do. This definition moves beyond mere algorithmic processing, venturing into domains that require adaptability and real-world interaction.
Historical Context and Evolution
The evolution of synthetic intelligence is marked by a gradual understanding of what intelligence means. Early models of artificial intelligence relied heavily on rule-based systems and simple algorithms, limiting their effectiveness in more complex scenarios. Over time, the field began to embrace more sophisticated approaches, including machine learning and neural networks.
Key milestones in this evolution include the introduction of deep learning techniques in the early 2010s, which allowed for unprecedented advancements in image and language processing. Synthetic intelligence emerged as an extension of these advancements, pushing the boundaries of what machines can achieve.
In summary, the historical trajectory of synthetic intelligence highlights an ongoing quest to emulate human-like cognitive processes. This lays the groundwork for more integrated and responsive systems that can potentially transform numerous sectors. The continued exploration and understanding of synthetic intelligence remain vital as we advance into a future where these systems can coexist with human intelligence.
Foundational Principles
Understanding the foundational principles of synthetic intelligence is key to grasping its essence. This section will explore the core theoretical framework, modeling of intelligence structures, and the components that are vital for building effective synthetic intelligence systems. Comprehending these principles provides a solid base for future applications and ethical considerations. Key elements include the interdependencies of different methodologies and technologies that drive synthetic intelligence forward.
Core Theoretical Framework
The core theoretical framework of synthetic intelligence encompasses the scientific theories and academic research that underpin its development. It integrates diverse disciplines, including cognitive science, computer science, and information theory. This framework elucidates how synthetic intelligence systems are designed to mimic certain cognitive functions, aiding in problem-solving and decision-making tasks. The research in this field consistently emphasizes the importance of structured thinking and systematic approaches to emulate human-like intelligence.
Modeling of Intelligence Structures
Modeling intelligence structures is essential for simulating the cognitive processes that synthetic intelligence aims to replicate. This involves creating conceptual models that represent how intelligence operates, from basic perception to higher-order reasoning. These models serve as mimetic constructs, guiding programmers and researchers to create systems that can interpret data similarly to humans. The effectiveness of these models lays the groundwork for the functionality and sophistication of future synthetic intelligence applications.
Key Components of Synthetic Intelligence Systems
Key components of synthetic intelligence systems must be understood to appreciate their functionality fully. These components are intricately linked and serve distinct roles:
Data Acquisition and Processing
Data acquisition and processing form the backbone of synthetic intelligence systems. This aspect involves gathering raw data from various sources and converting it into a usable format. The key characteristic here is the ability to handle large volumes of data efficiently, which is crucial for the system's performance. Data acquisition techniques may vary, yet they consistently emphasize the importance of accuracy and relevance. However, a challenge lies in ensuring data privacy and compliance with regulations.
Decision-Making Algorithms
Decision-making algorithms are essential for enabling synthetic intelligence to make choices based on the processed data. These algorithms analyze data inputs and generate outcomes, providing a systematic approach to problem-solving. Their primary advantage is efficiency in processing complex datasets, allowing for quick and informed decisions. However, there is an inherent risk of blind reliance on algorithms, which can lead to outcomes that may lack human judgment and intuition.
Learning Mechanisms
Learning mechanisms in synthetic intelligence allow systems to adapt and evolve through experience. Utilizing various forms of machine learning, these mechanisms enhance the system's capability to learn from data patterns. The significant characteristic of learning mechanisms is their ability to improve over time. This adaptability is a huge benefit, as it promotes originality in responses and solutions. On the downside, the need for vast amounts of data for training can present challenges, particularly in sensitive applications.
Methodologies in Synthetic Intelligence
Understanding the methodologies in synthetic intelligence is vital for grasping how these systems are developed and implemented. Methodologies refer to the various methods and techniques employed to create synthetic intelligence systems. They emphasize systematic approaches that ensure these systems are efficient, ethical, and effective. The significance of this section lies in its capacity to reveal not only how synthetic intelligence can be built but also the considerations that must come into play when developing it.
Techniques for Development
Simulations and Modelling
Simulations and modelling form a cornerstone in the domain of synthetic intelligence. They allow researchers and developers to create high-fidelity representations of real-world systems. This process helps to predict outcomes, test hypotheses, and identify faults in an environment that mimics reality without the risks associated with real-world applications.
The key characteristic of simulations and modelling is their flexibility. They can model various scenarios, from simple decision-making trees to complex simulations of environments impacted by multiple variables. This makes simulations an advantageous choice in the iterative process of method development and system refinement.
However, a unique feature of this approach is its dependency on the accuracy and richness of the input data. While simulations can provide precise predictions when designed correctly, poor data can lead to misleading results. Understanding these parameters is crucial for developers as they navigate the intricacies of creating synthetic intelligence systems.
Machine Learning Applications
Machine learning applications are pivotal in advancing synthetic intelligence. This approach provides the ability to learn from vast amounts of data, enabling systems to improve their performance over time without explicit programming for each task.
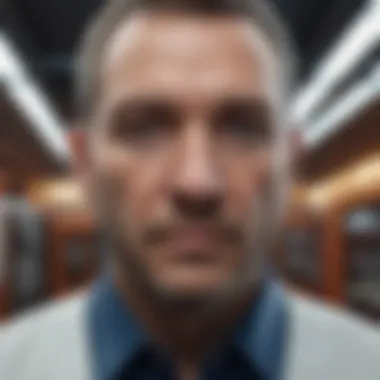
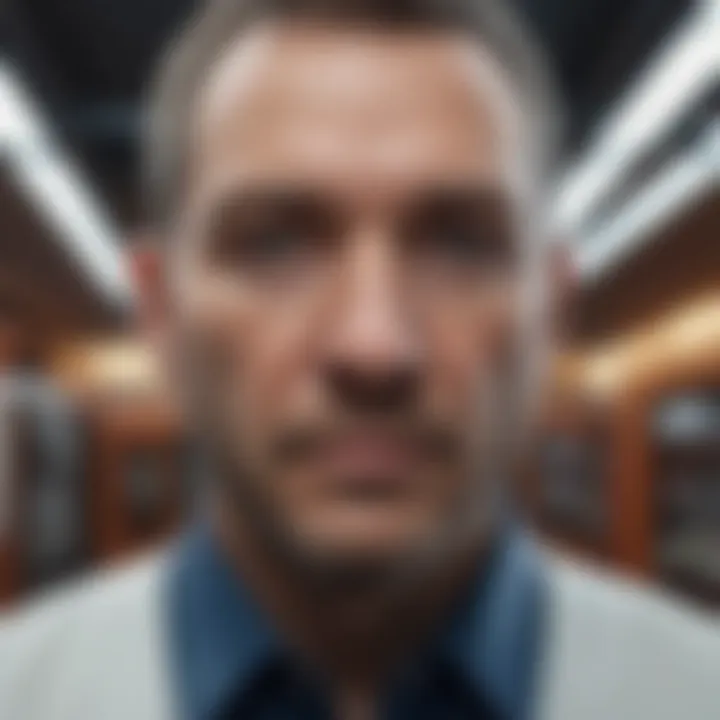
A significant characteristic of machine learning is its adaptability. It allows synthetic intelligence systems to fine-tune themselves based on feedback and new information from the environment. This adaptability makes machine learning a popular choice in the development of synthetic intelligence, as it aligns well with the dynamic nature of data interactions in real-world scenarios.
One unique feature of machine learning is the use of algorithms. Algorithms, like neural networks or decision trees, can handle various tasks ranging from image recognition to natural language processing. However, the potential for overfitting is a notable disadvantage. Overfitting occurs when a model learns too much from the training data, causing it to perform poorly on unseen data.
Hybrid Intelligence Systems
Hybrid intelligence systems represent a synthesis between various forms of intelligence, such as human and artificial. This methodology blends machine learning, expert systems, and other forms of intelligence to create robust decision-making frameworks.
The key characteristic of hybrid systems is their multi-faceted approach, combining strengths from different methodologies. This confers numerous benefits, including enhanced flexibility and efficiency in problem-solving. Such systems can react to a wider array of inputs, leading to better outcomes than solely relying on one method alone.
One unique aspect of hybrid intelligence is its ability to incorporate human intuition within machine capability. This integration can lead to improved outcomes in complex scenarios. However, the challenge lies in the balance between human input and machine processing. If this balance is not maintained, it can lead to inefficiencies or diluted decision-making processes.
Role of Big Data
In an era characterized by unparalleled data generation, the role of big data in synthetic intelligence cannot be overstated. Big data encompasses vast datasets that are too large or complex for traditional data-processing software. The implications of this trend are substantial, influencing how synthetic intelligence systems operate and evolve.
Big data allows synthetic intelligence systems to harness information from diverse sources. By analyzing these data sets, systems can detect patterns and correlations that would not be evident otherwise. This capability enhances the decision-making and learning processes inherent in these systems, driving innovations across various fields.
Additionally, the ability to leverage big data presents opportunities for continuous improvement. Synthetic intelligence systems can perpetually update and refine their models by accessing new information, leading to increasingly sophisticated applications. However, the challenges of data privacy and integrity must be acknowledged, requiring careful management to ensure ethical implications are addressed.
Overall, methodologies in synthetic intelligence shape its development and application. Understanding these methodologies enables stakeholders to evaluate and anticipate the impact of synthetic intelligence across multiple sectors.
Applications of Synthetic Intelligence
The applications of synthetic intelligence are vital to understanding its transformative potential across various sectors. This segment highlights how synthetic intelligence not only enhances existing systems but also creates new opportunities for innovation. Unlike artificial intelligence, which often focuses on emulating human cognition, synthetic intelligence aims to create a more adaptive, modular framework that can respond to complex real-world situations with greater effectiveness.
Impact on Healthcare
The healthcare sector stands to benefit enormously from the integration of synthetic intelligence. By utilizing advanced algorithms and modeling techniques, synthetic intelligence systems can assist in predictive analytics, patient management, and personalized treatment plans. For instance, large datasets can be analyzed to identify patterns in patient symptoms, leading to earlier interventions and more accurate diagnoses.
Moreover, synthetic intelligence can streamline administrative tasks, reducing the burden on healthcare professionals. Automating paperwork and other repetitive tasks allows medical personnel to focus more on patient care. Studies have shown that systems powered by synthetic intelligence can decrease wait times in hospitals and improve the quality of service.
"The application of synthetic intelligence in healthcare can lead to improved outcomes and operational efficiencies."
Healthcare providers must consider integrating synthetic intelligence thoughtfully to address challenges, such as data privacy and the reliability of the algorithms used. It becomes crucial to maintain ethical standards to protect patient information while enhancing operational efficiency.
Enhancements in Industrial Processes
In industrial settings, synthetic intelligence plays a crucial role in the optimization of production processes. By analyzing real-time data from machinery and workflows, these systems can identify inefficiencies and suggest improvements. This enhances productivity and ultimately conserves resources, leading to a reduction in operational costs.
Another significant advancement is predictive maintenance. Synthetic intelligence enables industries to monitor equipment health and predict failures before they occur. This proactive approach minimizes downtime and prolongs equipment lifespan, which is essential in sectors like manufacturing and logistics.
Furthermore, synthetic intelligence can facilitate supply chain optimization. By examining data from various components of the supply chain, industries can streamline operations, manage inventories better, and reduce waste. This not only increases profitability but also contributes to sustainability efforts.
Applications in Environmental Science
The realm of environmental science finds promising applications for synthetic intelligence, particularly concerning climate modeling and conservation efforts. Synthetic intelligence systems can simulate environmental scenarios based on vast datasets. This capability enables researchers to predict future conditions and assess the impact of various interventions.
Additionally, synthetic intelligence can be instrumental in monitoring ecosystems. By employing data from remote sensors, these systems can provide real-time feedback on environmental health, allowing for prompt action when issues arise. This could include everything from tracking wildlife populations to analyzing air quality in urban settings.
In agricultural practices, synthetic intelligence enhances precision farming. Farmers can utilize these technologies to optimize irrigation, reduce pesticide use, and boost crop yields. The ability to analyze data on soil conditions and plant health leads to more sustainable agricultural practices, which are vital in addressing food security.
Ethical Considerations
The exploration of synthetic intelligence naturally raises numerous ethical considerations. These considerations are crucial in guiding the development, implementation, and regulation of synthetic intelligence technologies. The importance of ethics in this field stems from the potential consequences that synthetic intelligence can have on society, human behavior, and individual rights. As synthetic intelligence systems become more integrated into everyday life, ensuring that ethical frameworks are in place is essential to safeguard against misuse and unintended side effects.
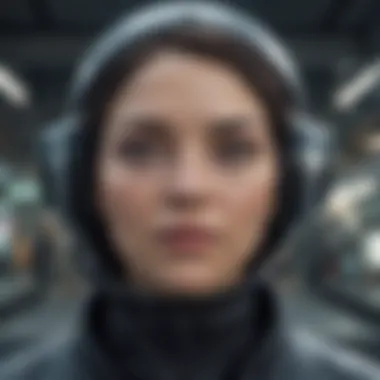
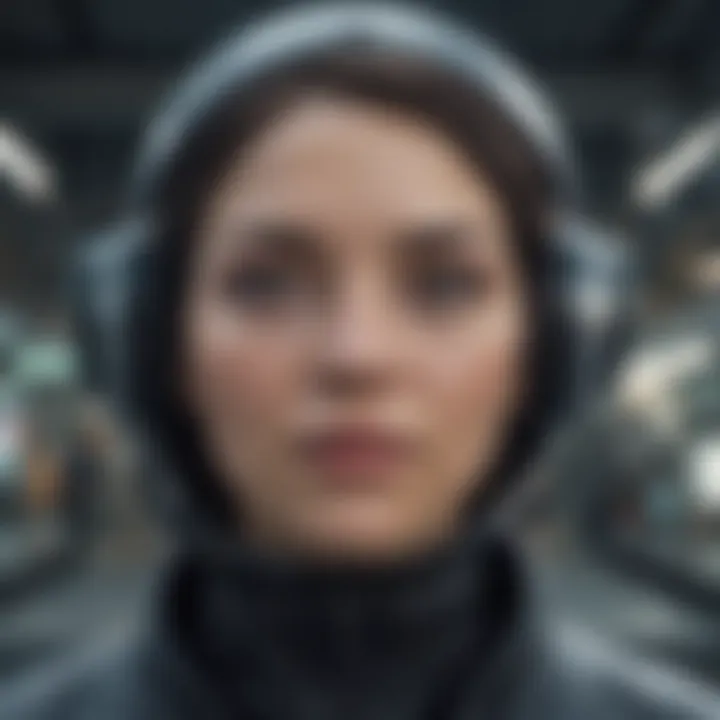
Moral Implications of Synthetic Intelligence
Synthetic intelligence carries significant moral implications. The capacity of these systems to make decisions autonomously raises questions about accountability. If a synthetic intelligence system makes a decision that leads to harm, who is responsible? The designer? The user? Or the system itself? This ambiguity complicates accountability and necessitates clear guidelines.
Moreover, synthetic intelligence can influence societal norms and values. For example, chatbots and recommendation algorithms can alter perceptions and behavior. If these systems are biased or programmed with flawed values, they may perpetuate stereotypes or misinformation. As researchers and developers of synthetic intelligence, there is a moral obligation to ensure that the technology aligns with principles of fairness and respect for all individuals.
"We must strive to understand how synthetic intelligence systems are shaping human interactions and societal structures."
Issues of Privacy and Surveillance
Privacy is another critical ethical consideration. The deployment of synthetic intelligence often involves collecting vast amounts of personal data. This data can enhance the performance and capabilities of these systems. However, the collection and use of personal information pose risks regarding privacy violations and surveillance. The balance between utilizing data for improved service and violating personal privacy is delicate and requires careful navigation.
Surveillance technologies powered by synthetic intelligence can lead to a reduction in civil liberties. When facial recognition systems or other monitoring technologies are applied indiscriminately, it can create an environment of fear and suspicion. It is essential to establish protocols that protect individuals' rights while leveraging the capabilities of synthetic intelligence. Without appropriate measures, the risk of abuse remains a significant concern.
Bias and Fairness in Synthetic Intelligence
Bias in synthetic intelligence systems is a pressing issue that has garnered attention in recent years. Algorithms are only as good as the data on which they are trained. If the training data contains biases, the resultant systems can reinforce these biases, leading to unfair outcomes. For example, biased datasets can lead to discrimination in job recruitment tools, law enforcement algorithms, and even credit scoring.
Ensuring fairness in synthetic intelligence involves several steps:
- Diverse Data Collection: Gathering data from various sources to minimize bias.
- Regular Audits: Conducting assessments to identify and rectify biases in algorithms.
- Inclusive Design: Involving diverse voices in the design process to ensure a broader perspective is considered.
Addressing bias and fairness is not just an ethical requirement but also a practical necessity. Ensuring these elements fosters trust and efficacy in synthetic intelligence systems, ultimately leading to better outcomes for everyone involved.
Future Prospects
The exploration of synthetic intelligence is imperative for understanding its evolving role in the future. This section will unfold the anticipated advancements within the field and how they hold significance across numerous sectors. As society becomes increasingly reliant on intelligent systems, comprehending these future prospects will enable stakeholders to make informed decisions and adapt strategies that align with technological developments. Crucial to this discussion are the predicted trends in synthetic intelligence, alongside its potential integration with other emerging technologies that can amplify its effectiveness.
Predicted Trends in Synthetic Intelligence Development
The landscape of synthetic intelligence is shifting rapidly, and several trends are emerging that will define its future. Notably, there is an ongoing evolution towards more autonomous systems. Researchers are focusing on creating synthetic intelligences that can operate independently while adapting to their environment. This autonomy can lead to significant improvements in efficiency and decision-making processes across various industries.
"The autonomy of synthetic intelligence systems may redefine how we approach problem-solving in complex environments."
Moreover, there is an increasing trend toward customizing intelligent systems. Organizations are seeking tailored solutions that address specific challenges. This means that synthetic intelligence development will likely prioritize modular designs, allowing flexible integration in various applications such as healthcare, finance, and manufacturing.
Another trend is the enhancement of human-machine collaboration. Future developments will emphasize systems that work alongside humans effectively. This collaboration is expected to optimize productivity while maintaining human oversight, particularly in sensitive domains like medicine where the stakes are high.
Integration with Other Emerging Technologies
The integration of synthetic intelligence with other emerging technologies is essential for maximizing its impact. For instance, the fusion of synthetic intelligence and the Internet of Things (IoT) can result in smart environments that learn and react to user behavior. This interconnectedness can support transformative applications ranging from automated home systems to advanced industrial processes.
Furthermore, synthetic intelligence is predicted to synergize with blockchain technology. The decentralized nature of blockchain can provide a secure framework for the data transactions that synthetic intelligence relies on. This integration not only enhances data security but also improves transparency in automated processes.
In addition, advancements in quantum computing will likely bolster the capabilities of synthetic intelligence. The computational power of quantum systems can accelerate data processing and analysis, making it possible for intelligent systems to operate at unprecedented speeds.
The End
The conclusion of this article serves as a vital component in encapsulating the significance of synthetic intelligence. It summarizes the intricate discussions surrounding its foundations, methodologies, applications, and ethical concerns. By clearly delineating the various elements of synthetic intelligence, this section helps to reinforce the overarching principles that have been explored throughout the paper.
Summarizing Key Findings
In reviewing the key findings, we can highlight several pivotal points:
- Definition and Clarity: Synthetic intelligence is distinct from traditional artificial intelligence. It encompasses a wider array of constructs that mimic human-like understanding and reasoning capabilities.
- Core Principles: Understanding the modeling of intelligence structures is essential. These frameworks allow for the development of more sophisticated systems that can learn from both structured and unstructured data.
- Applications Across Domains: The exploration of applications across fields such as healthcare, environmental science, and industrial processes emphasizes the transformative potential of synthetic intelligence.
- Ethical Considerations: The discussion on ethical issues addresses the moral implications, privacy concerns, and biases that may arise from deploying these systems.
The relevance of these findings lies in their ability to highlight the intricate layers of synthetic intelligence and its pervasive influence across multiple domains.
Call for Further Research
The landscape of synthetic intelligence is rapidly evolving. To keep pace with advancements and understand their implications, further research is paramount. Key areas warranting exploration include:
- Improving Learning Mechanisms: More research into adaptive learning algorithms can help create systems that learn more like humans do.
- Ethical Framework Development: As synthetic intelligence grows, establishing robust ethical frameworks becomes critical. This will aid developers and policymakers in guiding the deployment of these technologies responsibly.
- Interdisciplinary Studies: Collaborations between computer scientists, ethicists, and social scientists can foster a broader examination of synthetic intelligence's societal impact.
- Longitudinal Studies: The long-term effects of synthetic intelligence applications need investigation to better understand their lasting implications.