Exploring Self-Taught AI: The Future of Learning
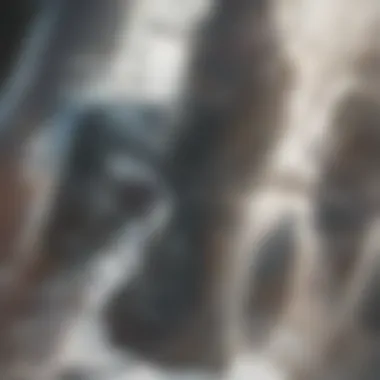
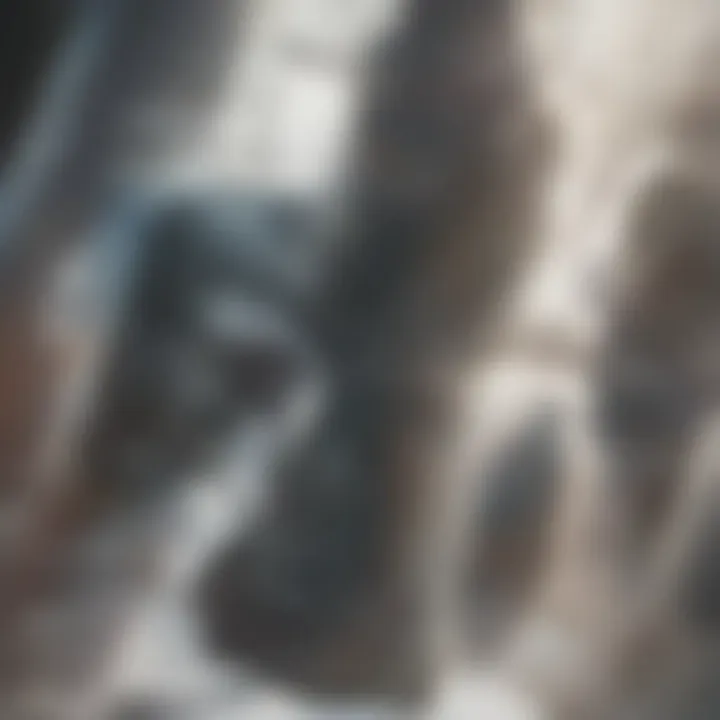
Intro
Artificial intelligence has embarked on a paradigm shift that invites considerable attention and scrutiny. As research develops, the emergence of self-taught AI emerges as a key area of exploration. This concept refers to systems that can learn independently, without direct human instruction. This capability significantly changes the way we perceive AI's potential, making it a vital aspect of ongoing technological conversation.
Understanding how self-taught AI operates is not merely an academic exercise. It has actual implications for industries around the globe. It leads us to consider how these systems could enhance efficiency and innovation. Moreover, ethical questions arise when AI does not follow traditional learning routes. The consequences could be far-reaching, touching everything from employment to data privacy, driving the urgency for comprehensive analysis in this field.
In this article, we aim to dissect the mechanisms of self-taught AI, emphasizing its learning processes and implications for various sectors. We will also explore past research findings and the current landscape, enabling a deeper understanding of this emerging technology.
Prelude to Self-Taught Artificial Intelligence
The exploration of self-taught artificial intelligence (AI) represents a significant leap in how machines interact with information and learn over time. This section delves into the essentials of self-taught AI, emphasizing its importance in today's technological ecosystem. Unlike traditional AI, where algorithms are explicitly programmed to perform tasks, self-taught AI systems learn autonomously by analyzing vast amounts of data. This paradigm shift not only enhances the efficiency of AI applications but also introduces complexities in understanding their decision-making processes.
Understanding self-taught AI requires a nuanced understanding of its definition and conceptual framework, as well as the historical developments that have shaped its evolution. The capabilities of self-taught systems arise from their ability to adapt and improve continually, making them a focal point of modern AI research. As organizations across various sectors harness this technology, it becomes crucial to grasp the mechanisms and implications of self-learning.
Moreover, the benefits of self-taught AI extend far beyond mere data processing. They include increased operational efficiency, cost reduction, and the potential for innovation across many domains. However, with these benefits come considerations regarding ethical implications and the societal impact of such powerful technologies.
Definition and Concept
Self-taught artificial intelligence refers to AI systems that have the capacity to learn and improve from their experiences, often without direct human guidance. This self-directed learning process implies that these AI models can analyze data inputs, identify patterns, and make informed decisions. Key characteristics of self-taught AI include:
- Autonomy: The ability to learn independently from data interactions.
- Adaptability: Changes in performance based on new experiences or information.
- Improvement Over Time: Continuous enhancement of capabilities as more data becomes available.
These characteristics not only redefine how we understand intelligence in machines but also raise pertinent questions about automation, creativity, and the future role of humans in relation to AI.
Historical Context
The concept of self-taught AI is not entirely new; it can be traced back to early machine learning approaches that aimed to mimic human cognitive processes. The development of algorithms capable of learning from data has evolved over decades, layered upon foundational ideas in neural networks and theories of cognition. Key milestones include:
- 1950s: Initial exploration of machine learning concepts.
- 1980s: Resurgence of neural networks with backpropagation algorithms.
- 2010s: Breakthroughs in deep learning techniques that enable more sophisticated models to learn directly from unstructured data.
These historical advances have laid the groundwork for modern self-taught AI systems. The transition from supervised to self-supervised learning marked a turning point, where machines exploit expansive datasets to discern meaningful relationships without explicit labels.
To encapsulate, understanding self-taught AI involves grasping its definition and historical backdrop. This understanding serves as a springboard to dive deeper into the mechanisms that drive self-learning and explore the practical applications emerging from this paradigm shift.
Mechanisms of Self-Taught Learning
The mechanisms of self-taught learning are central to understanding how artificial intelligence evolves without extensive human intervention. Self-taught AI systems harness data exposure to enhance their training, allowing them to identify patterns and make decisions autonomously. This approach not only accelerates learning but also reduces dependency on labeled datasets. The importance of these mechanisms lies in their implications for efficiency and scalability, making self-taught AI a transformative element in today's technological landscape.
Data Exposure and Learning Processes
Data exposure is crucial for self-taught AI systems. These algorithms consume vast amounts of unstructured data from various sources. As they process this data, they extract features and patterns that are not explicitly outlined by humans. This ability to learn directly from data drives innovation in numerous applications, from autonomous vehicles to predictive analytics in healthcare.
The learning process involves algorithms that adjust their parameters based on feedback received from the data. Techniques like unsupervised learning allow these systems to group similar data points, while semi-supervised learning can utilize a small amount of labeled data alongside a larger pool of unlabelled data. This method is valuable in situations where gathering labeled data is costly or time-consuming.
In summary, the ongoing exposure to a diverse dataset allows self-taught AI systems to become more robust and accurate in their tasks. Hence, an understanding of data exposure mechanisms helps in designing more efficient learning environments for these algorithms.
Neural Networks and Their Role
Neural networks play a pivotal role in the framework of self-taught learning. They simulate the human brain's structure and functioning, comprising layers of interconnected nodes, known as neurons. These networks can learn complex functions through training, making them essential in processing intricate patterns within large datasets.
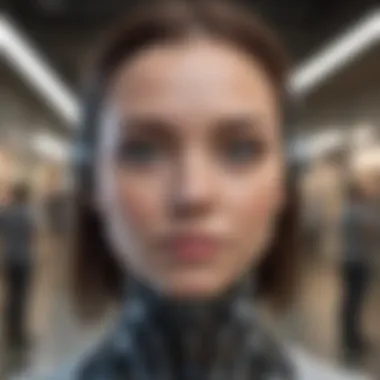
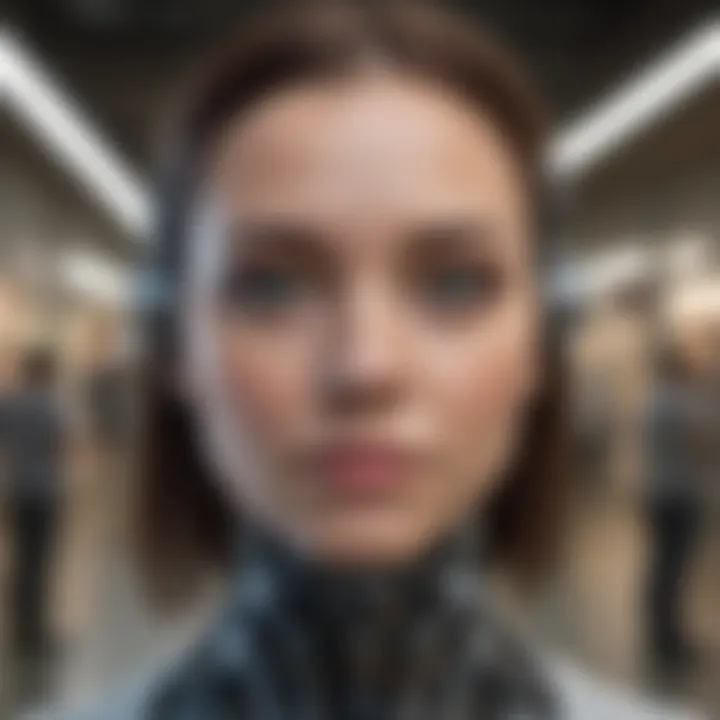
In the context of self-taught AI, neural networks excel in feature extraction and representation learning. For instance, convolutional neural networks (CNNs) are particularly effective in image and video recognition by identifying spatial hierarchies in visual data. Likewise, recurrent neural networks (RNNs) are utilized for sequential data, such as time-series forecasting or natural language processing, enabling AI to learn context and meaning over time.
Through their adaptability, neural networks can significantly enhance the learning process. They continuously refine their weights and biases based on the data processed, which improves prediction accuracy. Understanding their operation is crucial for implementing effective self-taught AI systems, allowing them to leverage large datasets effectively.
Reinforcement Learning Techniques
Reinforcement learning (RL) introduces a different dynamic to self-taught learning mechanisms. In this framework, AI agents learn by interacting with their environment and receiving feedback in the form of rewards or penalties. This trial-and-error approach allows them to develop optimal strategies for various tasks.
For example, in a gaming environment, an AI agent may be rewarded for making moves that lead to victory while penalized for moves that result in defeat. Over time, the agent learns to maximize its rewards through experience. This technique has seen significant success in domains such as robotics, where machines learn to navigate and perform tasks without human guidance.
Reinforcement learning promotes adaptive learning and can handle complex scenarios with multiple variables. Its principles are being increasingly applied across sectors, including finance, healthcare, and autonomous systems.
In summary, the mechanisms of self-taught learning underscore the AI's capacity to evolve independently, making significant strides in accuracy and efficiency across various fields. Understanding these concepts not only enhances our technological capabilities but also prompts deeper reflections on the future of AI.
Applications of Self-Taught AI
The applications of self-taught artificial intelligence are vast and varied, covering multiple industries and areas of human endeavor. This section explores how these technologies are transforming sectors such as healthcare, finance, and content creation. Emphasizing the practical implications of self-taught AI, we can recognize the significant benefits and challenges these innovations bring to the table.
Healthcare Innovations
Self-taught AI plays a crucial role in revolutionizing healthcare. By analyzing extensive medical datasets, these systems can detect patterns that might elude even the most experienced professionals. For instance, AI algorithms are increasingly utilized for diagnostic purposes, identifying conditions like diabetes or cancer at earlier stages. This can lead to better treatment outcomes and lower healthcare costs.
Moreover, self-taught AI systems can personalize medicine by correlating patient data with vast biomedical knowledge. Precision medicine, where treatment is tailored to individual characteristics, relies heavily on these advanced machine learning techniques. The ability to continuously learn from new data means these systems adapt to emerging trends in patient care. This was particularly evident during the COVID-19 pandemic, where self-learning algorithms rapidly integrated data on transmission and treatment to enhance public health responses.
Financial Sector Advancements
The financial sector has seen remarkable advancements owing to self-taught artificial intelligence. Self-learning models are deployed to identify fraudulent transactions effectively. With traditional methods, spotting suspicious activity can be time-consuming. However, self-taught AI can process and analyze transaction data in real-time, flagging anomalies that may indicate fraud. This timely intervention is essential in mitigating financial losses.
Investment management is another domain benefiting from self-taught AI. These systems can analyze market trends by examining historical data and predicting future movements. Algorithms from companies like BlackRock and Bridgewater leverage AI to assess risk and optimize portfolios. The insights generated aid financial professionals in making informed decisions, reducing human error in strategies.
The implications for lenders are also profound. Self-taught AI evaluates credit risk more efficiently by analyzing diverse data points, leading to faster loan approval processes. This opens avenues for financial inclusivity, as underserved populations may gain easier access to credit.
In essence, self-taught AI transforms the financial landscape, increasing safety, efficiency, and accessibility.
Automated Content Creation
Another exciting application of self-taught AI is in automated content creation. This involves using advanced AI tools that can generate written text, graphics, and more. With natural language processing, systems can produce articles, reports, and even creative writing almost indistinguishable from that of a human.
For businesses, automated content creation saves time and resources. Companies often use self-taught AI to manage social media posts, generate marketing content, or create product descriptions. This not only increases productivity but also allows for A/B testing to optimize content strategies based on audience engagement.
However, there are ethical implications. While AI-generated content can enhance efficiency, it raises questions about authenticity and originality. There is a delicate balance between leveraging technology to aid in content creation and ensuring that human creativity is not overshadowed.
Additionally, tools like OpenAIโs GPT-3 set a precedent for how much self-taught AI can simulate human writing. Despite the advantages, care must be taken to ensure quality and integrity in content. In summary, while automated content creation harnesses the power of self-taught AI to streamline production, it calls for mindful use to preserve the essence of creative expression.
Ethical Considerations
The emergence of self-taught artificial intelligence brings forth numerous ethical concerns that warrant thorough examination. As AI systems learn and adapt autonomously, they inherit data biases, which can lead to undesired consequences. Understanding these ethical implications is crucial for developers and stakeholders in facilitating responsible AI deployment.
Bias in Data and Its Consequences
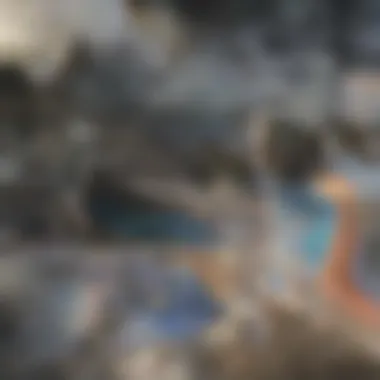
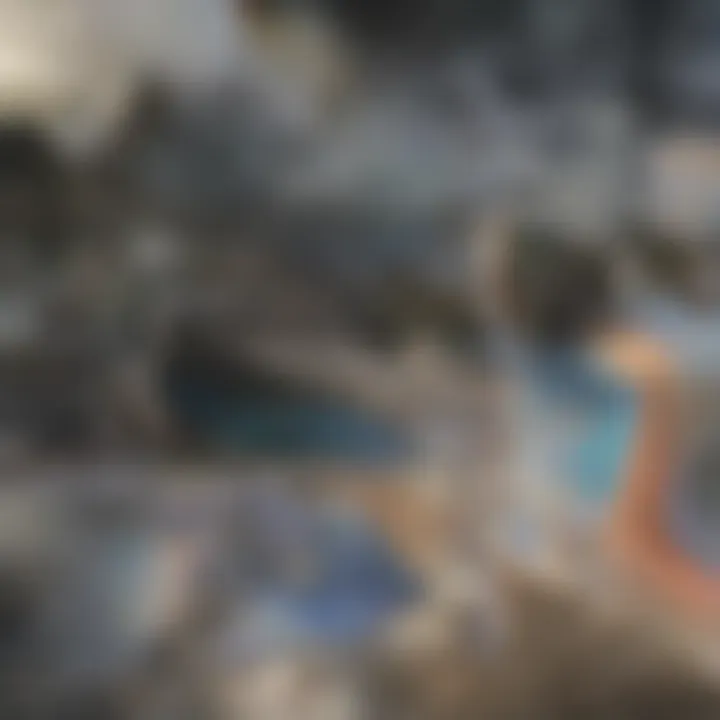
Bias in data is a prominent issue in the realm of self-taught AI. Algorithms learn from the datasets they are exposed to. If these datasets contain biased information, the AI will likely replicate and amplify these biases in its outputs. Social inequalities can be reflected in the data, ultimately skewing the AIโs decision-making processes.
For instance, facial recognition technologies have faced scrutiny for misidentifying individuals from underrepresented racial groups. This highlights the risk of perpetuating stereotypes and discrimination. The consequences of biased data extend beyond technical inaccuracies; they can shape societal perceptions and reinforce existing inequalities.
"Data bias is not just a technical problem; it reflects our societal values and choices."
To mitigate these biases, it is vital to curate diverse datasets that represent all demographics fairly. Developers must adopt rigorous evaluation frameworks to constantly assess and adjust AI behavior. Focusing on transparency in data collection can also aid in identifying inherent biases.
Transparency and Accountability
Transparency is essential for building trust in self-taught AI systems. When AI makes decisions based on data patterns, users deserve clarity about how these decisions are made. Lack of transparency can lead to skepticism or fear regarding AI technologies, particularly in critical applications such as healthcare or law enforcement.
Accountability goes hand in hand with transparency. Who is responsible for the decisions made by an AI system? If an AI mishandles data or causes harm, finding accountability is complex. Is it the developer, the organization using the AI, or the data provider?
Establishing clear accountability structures can help ensure responsible usage. The introduction of regulatory frameworks may be needed to hold stakeholders accountable. Sharing methodologies and training data can enhance transparency, allowing for scrutiny and public confidence.
Impact on Employment and Society
The impact of self-taught AI on employment is a topic that evokes significant concern. As AI systems gain capability, there is a fear of widespread job displacement. Certain labor sectors may become obsolete as manual tasks are automated. Jobs requiring routine decision-making may also dwindle over time.
However, self-taught AI may also create new opportunities. Emerging roles in AI management, ethical oversight, and data analysis may begin to surface alongside the technology. It is important to recognize that, while the landscape of employment may shift, it does not inherently lead to loss. Rather, it paves the way for a transformation in skills needed for the workforce.
Societally, the integration of self-taught AI could influence behavioral norms and interactions among individuals. For example, personalized content generation can shape public opinion and impact democratic participation. While AI offers efficiency and optimization, it can also lead to fragmentation in society if not carefully monitored. Education on the responsible usage of AI can mitigate these effects.
In summary, addressing ethical considerations related to self-taught AI is paramount. By acknowledging biases in data, promoting transparency, and evaluating employment impacts, stakeholders can foster a responsible AI landscape ensuring that technological advancements benefit society as a whole.
Challenges in Self-Taught AI Development
The field of self-taught artificial intelligence presents both opportunities and hurdles. Understanding these challenges is essential for unraveling the complexities behind self-learning AI systems. The effectiveness of self-taught AI hinges largely on the ability to process vast amounts of data without human supervision. Therefore, addressing these challenges not only enhances the performance of AI systems but also ensures ethical and responsible deployment across various industries.
Data Quality and Availability
To thrive, self-taught AI requires high-quality data. Inaccurate or incomplete data leads to ineffective learning, resulting in biased or unreliable outcomes. Data must be diverse, representative, and properly labeled. The challenge of data availability poses a significant barrier, especially in niche areas where collecting data can be difficult or cost-prohibitive. Additionally, reliance on large datasets raises concerns regarding privacy and consent, which further complicates data management.
Some factors affecting data quality include:
- Relevance: Data must reflect the current conditions for effective model training.
- Diversity: Models trained on homogeneous data can perpetuate existing biases.
- Volume: Self-taught systems typically require vast datasets to improve their accuracy and reliability.
In summary, ensuring data quality and managing availability is a crucial element in the successful development of self-taught AI.
Computational Limitations
Computational resources are another substantial challenge in self-taught AI development. Self-learning algorithms consume considerable processing power. Systems must not only handle large datasets but also perform complex calculations in real-time. This requirement often necessitates advanced hardware and optimization techniques.
Some limitations include:
- Processing Speed: As datasets grow, the time required to process these amounts increases, which can inhibit real-time applications.
- Infrastructure Costs: Setting up the necessary computational infrastructure can be financially taxing, limiting the access of smaller organizations.
- Scalability: Ensuring that models remain effective as they scale can be a technical challenge.
Programming techniques like parallel processing or cloud computing emerge as potential solutions. Nevertheless, the challenge remains to efficiently utilize resources within budget constraints.
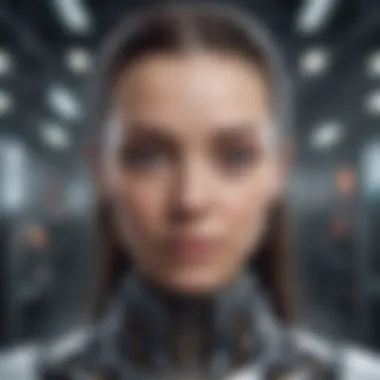
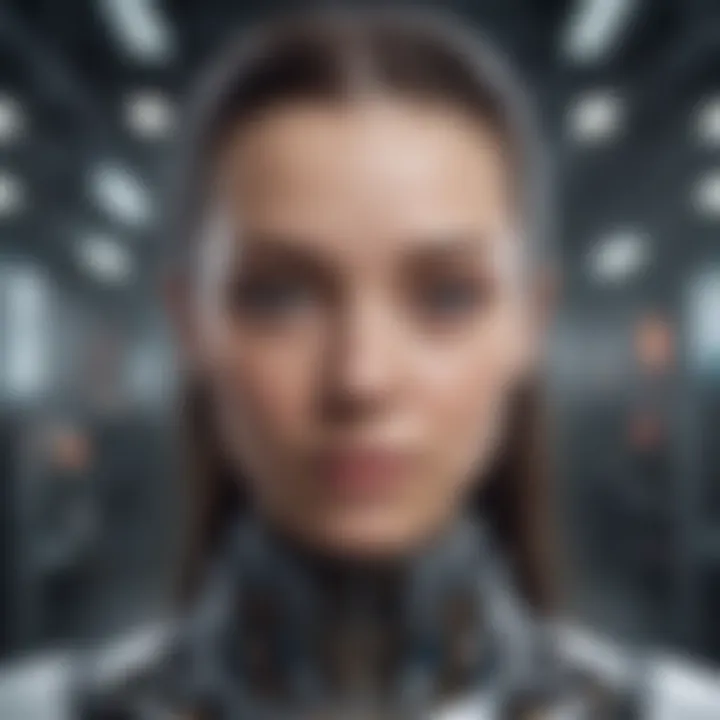
Ethical Dilemmas in Implementation
Implementing self-taught AI solutions often raises ethical dilemmas that must be carefully navigated. The autonomy of AI systems in learning leads to concerns regarding accountability and transparency. If a self-learning model makes erroneous predictions or is involved in a harmful incident, the question arises: who is responsible?
Additionally, ethical dilemmas can emerge from:
- Data Usage: The manner in which data is sourced and used impacts ethical considerations. Unethical data use can lead to breaches of privacy and trust.
- Bias in Learning: As noted previously, biased datasets can lead to discriminatory outputs. Understanding and mitigating this risk is crucial.
- Decision-making Autonomy: Relying on AI for critical decisions such as healthcare or law enforcement invites risks. These decisions must remain subjected to human oversight.
Future Perspectives
Understanding the future perspectives of self-taught artificial intelligence is crucial in grasping its potential impact on technology and society. As self-taught AI systems evolve, assessing their trajectory helps identify trends, opportunities, and challenges that will shape various industries. The explorations in this section will offer insights into how these innovations will function, their benefits, and the considerations that must be addressed as we advance.
Innovative Trends in AI Learning
The landscape of AI learning continuously shifts as technology develops. Among the notable trends is transfer learning, which allows AI models to adapt knowledge gained from one task to new and related tasks. This demonstrates a fundamental efficiency in training methods as fewer data points are needed.
Another significant trend is unsupervised and semi-supervised learning. These approaches enable models to learn patterns without extensive labeled data. They take advantage of large volumes of unstructured data, making learning more accessible and scalable.
Advancements in modular AI systems are also noteworthy. These systems allow for more flexible architectures. Components can be interchanged based on requirements, fostering innovation. The success of these trends is evident in various applications, from autonomous systems to efficient content generation.
Predictions for AI Integration Across Industries
Self-taught AI has implications across diverse sectors. In healthcare, integration can lead to enhanced diagnostics and personalized treatments. AI systems may analyze patient data efficiently, thereby improving outcomes and reducing costs.
In finance, self-taught AI shows potential for risk management and fraud detection. Algorithms that learn continuously from transaction patterns can adapt to emerging threats, making systems smarter and more resilient.
Moreover, the educational field could experience a significant evolution. AI tutors could personalize learning experiences based on student engagement and performance, resulting in improved learning curves.
Integration strategies will vary by industry but consistent preconditions include investment in infrastructure and a focus on ethical deployment. Without these, the potential benefits may not be realized.
Potential for Enhanced Human-AI Collaboration
The trajectory of self-taught AI does not suggest purely automated systems; rather, it indicates a future where humans and AI collaborate effectively. This collaboration can lead to enhanced decision-making processes. AI could manage data analysis, leaving humans to focus on strategic thinking and creativity.
Blending human intuition with AI precision could foster innovation. For instance, in research, AI can process thousands of articles in mere seconds, helping researchers identify trends and gaps in literature, thus saving time.
Furthermore, developing explainable AI can build trust in collaborative processes. If AI systems can clarify their reasoning and accountability, human decision-makers will be more confident in their insights.
In summary, the future of self-taught AI looks promising. Its integration into existing frameworks can facilitate unprecedented advancements across industries while nurturing a cooperative relationship between humans and machines. As we continue to explore this evolving landscape, considerations around ethical implications and transparency will be paramount.
Ending
The conclusion of this article serves as a vital component in synthesizing the complexities of self-taught artificial intelligence. As we have explored, self-taught AI represents a significant paradigm shift in how machines learn and adapt to new information without extensive human intervention. This discussion highlights the necessity of understanding not only the technology itself but also its implications across various sectors.
Summary of Key Insights
A deeper look into the subject reveals several key insights:
- Independent Learning Models: Self-taught AI can adapt and learn from diverse datasets. This grants it a dynamic capability that traditional AI methods lack, making it more flexible in real-world environments.
- Influence on Industries: The impact on sectors such as healthcare, finance, and content creation underlines the transformative nature of this technology. Enhanced diagnostics, better investment predictions, and more creative content generation are but a few examples.
- Ethical Dimensions: Important ethical considerations arise regarding data bias, transparency, and accountability. These aspects must be addressed to harness the true potential of self-taught AI responsibly.
- Future Collaborations: The possible integration of self-taught AI with human expertise opens avenues for unprecedented collaboration. Understanding this interplay is essential as we look forward to a future rich in innovation.
Final Thoughts on the Future of Self-Taught AI
Looking ahead, the future of self-taught artificial intelligence is both promising and challenging. As organizations invest more in AI systems that can learn and autonomously improve, we should prepare for rapid advancements in technology.
- Increased Adoption Across Sectors: Industries will likely see a growing reliance on self-taught AI solutions, prompting a need for skill development in the workforce.
- Regulatory Frameworks: Policymakers must consider regulations that ensure ethical compliance and hold systems accountable for their decisions.
- Sustained Research and Development: Continuous research into improving self-learning mechanisms will drive innovation. Potential breakthroughs could redefine what AI can achieve.