Exploring the Nexus of Science and AI Innovations
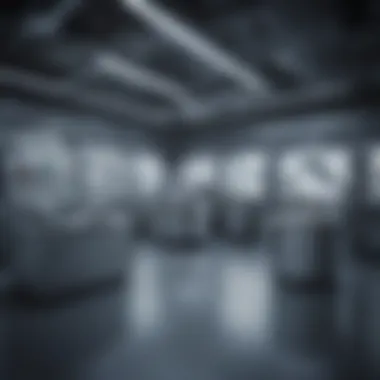
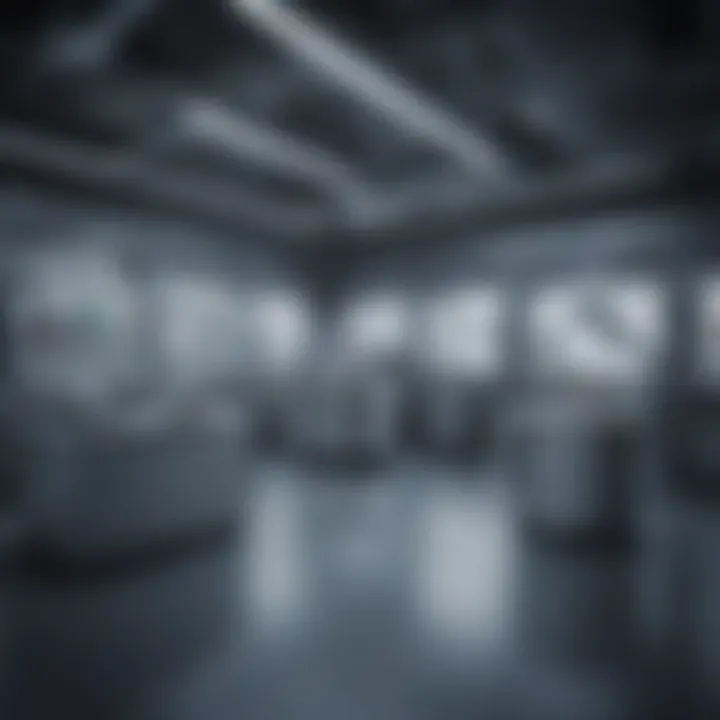
Intro
In recent years, the synthesis of science and artificial intelligence (AI) has emerged as a transformative force across various academic and industrial landscapes. The rapid advancements in AI technology provide innovative tools that augment scientific research, facilitating new discoveries and enhancing analytical capabilities. This intersection not only fosters exploration within established disciplines but also encourages interdisciplinary collaborations that drive innovation.
This overview aims to unpack the relationships between AI and numerous scientific fields, including biology, physics, and computer science. By analyzing relevant case studies and theoretical frameworks, we will assess how AI technologies shape research methodologies and lead to significant breakthroughs. Moreover, we will address the ethical dilemmas involved, emphasizing the importance of thoughtful integration of AI in science.
Key Research Findings
Overview of Recent Discoveries
The past decade has witnessed groundbreaking research that showcases the impact of AI across different scientific arenas. Fundamental discoveries are notably found in genomics, where AI algorithms have improved the accuracy of gene prediction. In particle physics, AI plays a crucial role in data analysis, allowing scientists to better understand complex phenomena such as the Higgs boson. Such advancements are not limited to the natural sciences; social sciences have also benefited from AI, with machine learning techniques analyzing vast datasets to identify trends and inform policy decisions.
- Genomics: AI enhances predictive accuracy in gene identification.
- Physics: Effective data analysis techniques unveil fundamental particles.
- Social Sciences: Machine learning aids trend analysis and policy formulation.
Significance of Findings in the Field
The implications of these discoveries are multifold. Enhanced prediction capabilities enable researchers to accelerate the pace of research and development. This efficiency can lead to health advancements through precision medicine and improved models for climate change, creating sustainable solutions. The significance lies in the ability to address complex challenges that were previously considered insurmountable. Such breakthroughs demonstrate that AI is not merely a tool but a fundamental component of the scientific process.
"Artificial intelligence is revolutionizing the scientific endeavor, allowing researchers to traverse previously uncharted territories."
Breakdown of Complex Concepts
Simplification of Advanced Theories
Many theories within science can be complex and difficult for even seasoned researchers to understand. AI helps simplify these concepts by providing models and tools that transform intricate data into accessible insights. For instance, AI-driven simulations in neuroscience offer clearer representations of brain function, making advanced theories more graspable. Additionally, AI facilitates the visualization of molecular interactions, simplifying the study of biochemical processes.
- Neuroscience: Simulation tools clarify brain activities.
- Biochemistry: Molecular visualization enhances comprehension of interactions.
Visual Aids and Infographics
To communicate complex data effectively, visual aids and infographics can be invaluable. They allow for the succinct presentation of vast amounts of data, making it digestible for a broader audience. Tools such as Microsoft Power BI and Tableau are increasingly employed to visualize research outcomes, thereby enhancing the interpretability of scientific results.
Intro to Science and Artificial Intelligence
The convergence of science and artificial intelligence (AI) represents a significant evolution in how research is conducted and knowledge is created. Understanding this intersection is crucial because it provides insights into both the capabilities and limitations of AI technologies as they relate to different scientific fields. The importance of exploring this topic lies not only in acknowledging AI as a tool but also in recognizing its role as a catalyst for innovation in scientific inquiry.
AI encompasses various subfields including machine learning, natural language processing, and deep learning. These technologies enable researchers to handle massive datasets with greater efficiency and accuracy. As a result, scientists can uncover patterns and derive insights that were previously elusive. This incorporation of AI is becoming indispensable for advancing many scientific disciplines, leading to enhanced research outcomes and increased productivity.
In examining the role of AI in science, it becomes evident that the benefits are multi-dimensional. Improved data analysis and predictive modeling have streamlined experimental procedures and enriched our comprehension of complex biological systems, physical phenomena, and even computational processes. Furthermore, the integration of AI prompts a reevaluation of traditional methodologies, promoting interdisciplinary collaboration among scientists, engineers, and technologists.
However, along with the advantages, several considerations must be addressed when discussing AIβs integration into science. Issues such as data bias, the need for human expertise, and the clarity of interdisciplinary communication present potential challenges to the effective use of AI. As such, understanding the implications of these factors is essential for fostering a responsible and innovative scientific community.
Ultimately, the relationship between science and AI is a dynamic one, characterized by continuous evolution. By delving into the definitions, historical context, challenges, and applications of AI in scientific research, we can better comprehend and shape the future landscape of this intersection.
Defining Artificial Intelligence
Artificial Intelligence refers to the simulation of human intelligence in machines that are programmed to think and learn like humans. These systems utilize algorithms to analyze data, identify patterns, and make decisions. The definition of AI encompasses a broad range of technologies including machine learning, where computers improve their performance based on experience, and natural language processing, which allows machines to understand and interact using human language.
AI is not just about replicating human intelligence; it also involves enhancing specific capabilities beyond natural human potential. In fields such as genomics or astrophysics, AI can process vast datasets at speeds unmatchable by human researchers, enabling unprecedented discoveries.
Historical Context of AI in Science
The roots of AI in scientific research can be traced back to the mid-20th century when the term "artificial intelligence" was first coined. Visionaries in computer science began to explore how machines could replicate cognitive functions. In the following decades, numerous milestones shaped AIβs role in science.
In the 1970s and 1980s, advancements in expert systems allowed for the computerization of decision-making processes in specific domains, such as medical diagnosis. This period marked a significant transition where AI could assist in solving complex problems within scientific inquiry.
The explosion of computational power in the early 2000s, coupled with the rise of big data, facilitated a more substantial integration of AI into scientific research. Researchers began employing sophisticated algorithms for data analysis, leading to breakthroughs in fields ranging from climate science to drug discovery. Today, AI continues to evolve, driving innovations and offering new tools that shape various scientific disciplines.
"Understanding AI's historical context is essential to grasp its current applications and future potential in science."
Through these historical developments, it becomes clear that AI has not merely entered scientific research; it has transformed it, reshaping the landscape of knowledge creation and discovery.
The Role of AI in Scientific Research
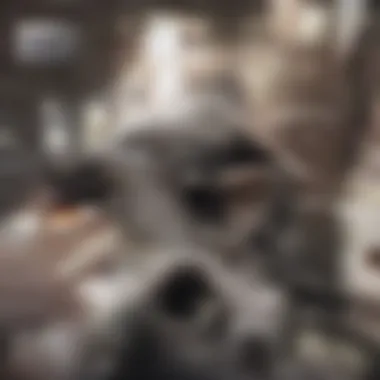
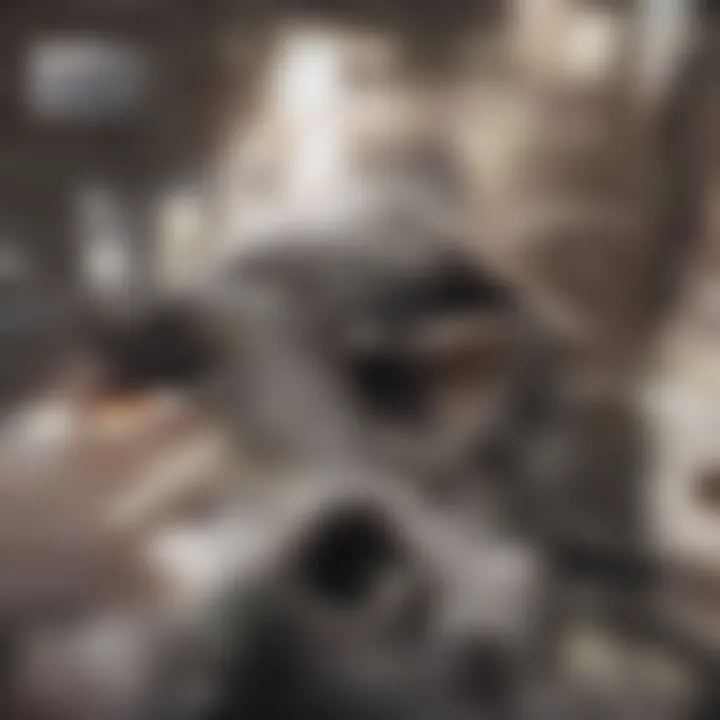
The integration of AI into scientific research represents a pivotal advancement that can dramatically transform current methodologies. AI techniques facilitate the handling of vast datasets, enhance analytical capabilities, and contribute to innovative solutions across various scientific domains. Its importance cannot be overstated; AI is not just an aid but a powerful ally that enhances the efficiency and effectiveness of research.
AI's potential in scientific exploration includes several elements, such as improving the accuracy of data analysis, optimizing experiments, and enabling previously unattainable insights. The incorporation of AI also helps to manage the complexity and volume of data produced in modern research, allowing scientists to focus on interpreting results rather than managing raw inputs.
Data Analysis and Interpretation
Data analysis forms the backbone of scientific inquiry. The advent of AI techniques, particularly machine learning, provides researchers with tools to analyze complex datasets swiftly and accurately. AI algorithms can identify patterns that may not be visible to the human eye, enabling researchers to extract valuable insights.
- Improved accuracy: AI models can minimize human error in data interpretation.
- Increased speed: Algorithms can process large volumes of data at previously unimaginable speeds.
- Real-time insights: Researchers can benefit from immediate feedback, allowing for adjustments on-the-go.
Utilizing AI in data analysis not only saves time but also allows for deeper exploration of scientific questions.
Predictive Modeling and Simulations
Predictive modeling is a significant area where AI demonstrates its capabilities. Researchers use predictive models to simulate various outcomes based on input parameters, aiding in hypothesis generation and testing. This methodology is particularly useful in fields such as climate modeling or epidemiology, where predicting future scenarios can inform effective strategy and intervention.
AI enhances predictive modeling by:
- Leveraging historical data: AI can learn from past data to forecast future occurrences.
- Increasing the precision of models: Advanced algorithms can refine and improve model accuracy over iterations.
These simulations can offer a clearer understanding of potential risks and opportunities, guiding decision-making effectively.
Automation of Experimental Procedures
Automation is another critical application of AI in scientific research. By automating experimental procedures, researchers can increase productivity and consistency while reducing the likelihood of errors.
AI systems can:
- Handle repetitive tasks: Automating routine tasks allows researchers to allocate their time and resources more efficiently.
- Monitor experiments: AI can facilitate real-time monitoring and adjustments, ensuring processes remain optimal.
The shift toward automation not only accelerates research but also fosters environments where creativity can flourish. Researchers can focus on innovative aspects of their work rather than mundane activities.
"As AI continues to evolve, its integration into scientific research will likely unlock new avenues of inquiry and innovation."
In summary, the role of AI in scientific research is multifaceted and transformative. By enhancing data analysis, facilitating predictive modeling, and automating experimental procedures, researchers can navigate complexities and propel their fields forward.
AI Applications Across Scientific Disciplines
The intersection of artificial intelligence and scientific disciplines is a transformative area of research. AI has become crucial in enhancing the efficiency and effectiveness of various scientific fields. This section aims to provide an overview of how AI applications revolutionize life sciences, physics, and computer science. By examining several key areas, we can understand the broad benefits AI brings to these disciplines.
Artificial Intelligence in Life Sciences
Genomic Research
Genomic research plays an important role in understanding biological processes. It often involves handling massive datasets, which can be challenging to analyze manually. AI algorithms can identify patterns and insights that might be overlooked by human researchers. One key characteristic of genomic research is the vast amount of sequential data generated by technologies such as next-generation sequencing. This makes it a beneficial choice for applying AI, as machine learning models enhance data interpretation.
AI can greatly improve genomics through its capabilities in predictive analytics and data mining. However, one disadvantage is the potential for overfitting in models, leading to inaccuracies in results.
Drug Discovery
Drug discovery is another essential application of AI in the life sciences. The process of finding new pharmaceuticals is long and expensive, often taking years. AI can streamline this process by predicting how different compounds will behave in the body. This predictive power is crucial for identifying promising candidates more quickly than traditional methods.
A unique feature of drug discovery with AI lies in its ability to analyze complex biological interactions. It becomes easier to screen vast libraries of compounds. Despite its advantages, there are still challenges, such as regulatory hurdles and the need for extensive clinical trials.
Medical Diagnosis
In medical diagnosis, AI technologies like machine learning and deep learning can assist healthcare professionals in identifying diseases more accurately. This supports the idea of precision medicine by tailoring treatment based on individual characteristics. The ability of AI systems to process and analyze medical images is particularly notable. Algorithms can enhance diagnostic accuracy, leading to earlier and more effective interventions.
However, reliance on AI poses some concerns. The potential for bias in the data used to train algorithms can lead to unequal healthcare outcomes. These issues must be addressed to ensure equitable access to AI-driven diagnostics.
The Influence of AI in Physics
Quantum Computing
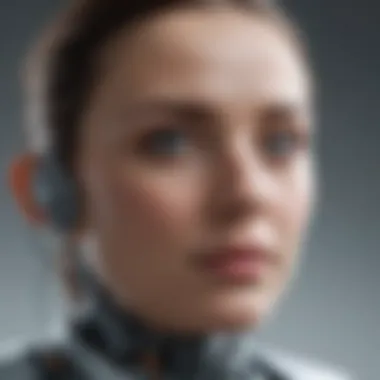
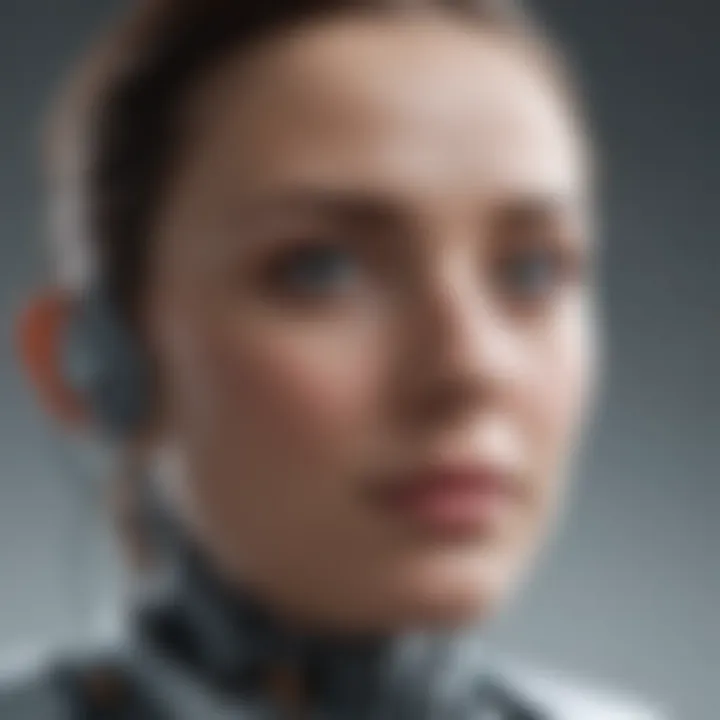
Quantum computing represents a revolutionary approach fundamentally different from classical computing. It harnesses the principles of quantum mechanics to perform calculations at unprecedented speeds. This unique characteristic allows it to tackle complex problems beyond the reach of conventional computers. AI can assist in optimizing algorithms and quantum systems, enhancing computational efficiency.
Despite its revolutionary potential, quantum computing faces significant challenges. The technology is still in its infancy, limited by the need for robust error correction and stable qubit states. AI integration is essential yet complex, making it a fascinating area of study.
Particle Research
Particle research, particularly in high-energy physics, benefits from AI through data analysis and pattern recognition. Facilities like CERN generate immense amounts of data from particle collisions. AI can streamline the analysis of this data, helping researchers identify significant events. The capacity to process and extract information quickly is a key characteristic that makes AI valuable in this area.
One notable advantage is the potential to discover new particles or phenomena that traditional methods may miss. However, the reliance on algorithms brings challenges, such as the need for transparency in AI decision-making processes.
Astrophysics
Astrophysics relies heavily on data analysis from telescopes and other detection systems. AI assists in filtering and processing this data, which can involve terabytes of information. One key characteristic of astrophysics is the focus on both large-scale structures and cosmic events, which can benefit from AIβs ability to identify cosmic phenomena.
Nonetheless, there are disadvantages. The vast scales involved can make it challenging for models to predict smaller details accurately. With continued advancements, however, AI is likely to play an increasingly important role in understanding the universe.
Applications in Computer Science
Machine Learning Algorithms
Machine learning plays a critical role in computer science, with applications ranging from image recognition to recommendation systems. These algorithms can learn from data inputs and improve over time, providing personalization in user experiences. A unique feature of these algorithms is their capacity to process large datasets efficiently, making them a favorable choice for this article.
The main advantage lies in their scalability and adaptability across various sectors. However, the challenge remains how to mitigate biases present in training data.
Robotics
Robotics integrates AI to enhance automation and efficiency. Robots equipped with AI can perform tasks ranging from manufacturing to autonomous navigation. One key aspect of robotics is its ability to interact with the environment in real-time, making decisions based on sensory input.
Despite its advantages in efficiency and precision, robotic systems can also face limitations. The complexity of human environments poses challenges. Ensuring robots operate safely alongside humans remains a critical concern.
Natural Language Processing
Natural language processing (NLP) enables computers to understand and respond to human language. This involves tasks such as translation, sentiment analysis, and chatbots. The capacity to interpret language nuances is a vital characteristic of NLP.
AIβs application in NLP streamlines communication processes, facilitating greater accessibility. Yet, one significant challenge remains the variability of human language and cultural context, leading to potential misunderstandings.
AI continues to shape various scientific disciplines distinctly. Its capabilities can enhance research efficiency, data management, and understanding of complexities within each field. Nevertheless, it is crucial to address associated challenges and limitations as we move forward.
Challenges and Limitations of AI in Science
Artificial intelligence offers exciting opportunities for innovation in science. However, several challenges arise when integrating AI into research disciplines. Understanding these limitations is crucial for maximizing the benefits of AI and fostering responsible practices.
Data Quality and Bias
The effectiveness of AI systems relies heavily on the quality of the data used for training and validation. Poor data quality can lead to misleading results and conclusions. Bias in data can accentuate disparities by skewing outputs, potentially resulting in unequal treatment or flawed scientific models.
Examples of bias may include datasets that are not representative of the entire population or contain historical prejudices. Such issues not only compromise the credibility of AI-driven insights but can also lead to ethical dilemmas. For instance, an AI model developed for medical diagnosis may perform poorly on certain demographic groups if the training data mostly represents another group.
Researchers must prioritize methods to identify, quantify, and mitigate bias in data collection to ensure accurate AI deployment. This effort involves:
- Data auditing: Regular checks of datasets to identify any biases or inaccuracies.
- Diverse Datasets: Incorporating data from varied sources, ensuring broad representation across different demographics.
- Continuous Monitoring: AI performance must be regularly assessed to recognize emerging issues over time.
The Dependence on Human Expertise
AI systems cannot function in a vacuum. Significant human expertise is still required to interpret AI outputs meaningfully. Human scientists need to refine models, choose appropriate algorithms, and adjust parameters to align with ongoing research objectives. AI often lacks the intuitive understanding and contextual insight humans bring to their work.
Experts play a vital role in ensuring that the AI system adheres to scientific rigor. If AI outputs are misused or misinterpreted, the resulting conclusions can be detrimental. Thus, its implementation should complement rather than replace human expertise.
It is essential for organizations to foster collaboration between AI systems and skilled professionals by promoting:
- Training Programs: Educating researchers to effectively use AI tools and derive meaningful insights.
- Integrative Approaches: Encouraging teamwork between AI specialists and domain scientists to build comprehensive solutions.
Interdisciplinary Communication Barriers
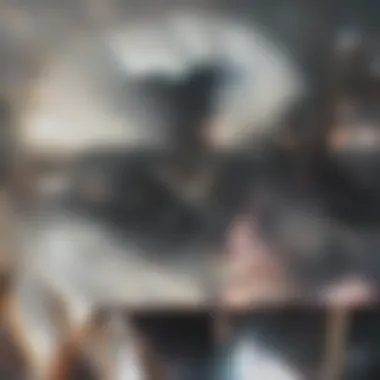
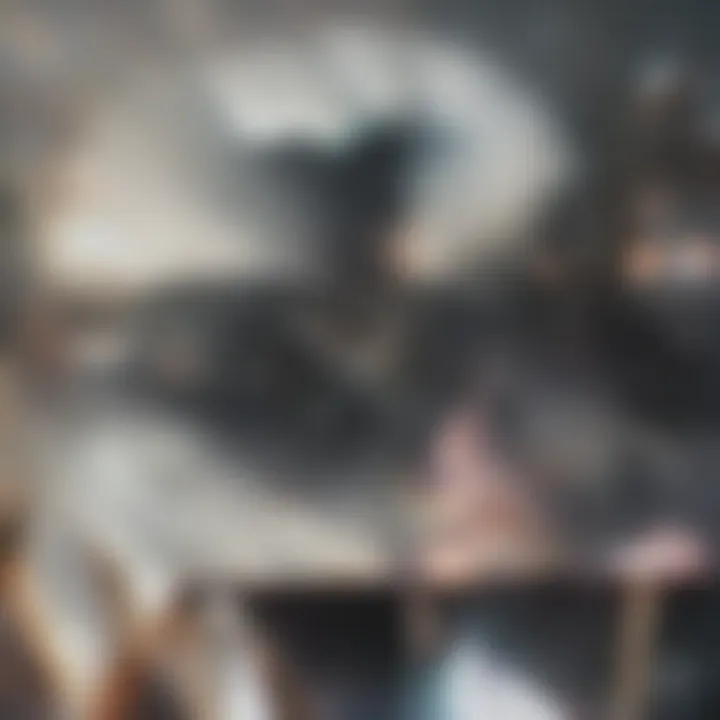
AI research often spans multiple disciplines, creating a necessity for effective communication. Scientists from different backgrounds may struggle to collaborate due to disparate terminologies, methodologies, and perspectives. This barrier can hinder progress, as valuable insights can be lost in translation.
To tackle these communication challenges, several strategies can be employed:
- Common Frameworks: Establishing standardized terms and practices across disciplines to enhance understanding.
- Workshops and Conferences: Organizing interdisciplinary events to facilitate knowledge exchange and collaboration.
- Collaborative Platforms: Utilizing digital tools to bridge gaps between specialties, making resources accessible.
Overall, by addressing these challenges, researchers can harness the power of AI responsibly and effectively, leading to more impactful scientific discoveries.
Ethical Considerations in AI Research
The integration of artificial intelligence (AI) into scientific research yields significant benefits, but it also raises crucial ethical considerations. As AI becomes more embedded in various scientific fields, it is essential to analyze the implications of its use. Ethical discussions guide researchers in ensuring that AI applications uphold integrity, fairness, and accountability.
AI in Biomedical Research
Biomedical research stands out as one of the most impacted areas by AI advancements. Machine learning algorithms analyze vast datasets, enabling researchers to identify patterns and make predictions about diseases. For instance, AI systems, such as DeepMind's AlphaFold, have shown extraordinary capabilities in predicting protein folding, a task that helps in drug development and understanding genetic diseases.
However, the use of AI in this area is fraught with ethical dilemmas. Researchers must navigate issues like consent in using patient data. The reliance on AI can lead to a phenomenon known as "algorithmic bias," where biased training data results in skewed outcomes. It is vital for researchers to prioritize the representation of diverse populations in datasets to ensure equitable healthcare outcomes.
Consequences of AI Decision-Making
The decisions made by AI systems can have profound implications in various research contexts. For instance, when AI algorithms inform clinical decisions, biased outputs can adversely affect patient treatment. This suggests a pressing need to maintain transparency in AI algorithms.
Moreover, the responsibility for AI-generated decisions is also a crucial issue. When an AI model suggests a treatment plan, who is held accountable if the outcome is negative? As the technology evolves, it is essential to create a framework that clearly defines accountability between human researchers and machine-generated recommendations. This will help maintain trust between the public and scientific communities.
Privacy and Data Protection Issues
Privacy remains a paramount concern with the increasing use of AI in research. The data collected for training AI systems often includes sensitive personal information. Ensuring robust data protection measures is critical to safeguarding individual rights.
Regulations such as the General Data Protection Regulation (GDPR) provide a legal framework for data protection, but researchers must actively implement these controls within their work.
- Researchers should adopt anonymization techniques during data collection.
- Access to datasets must be regulated strictly to prevent misuse.
- Continuous assessment of ethical implications is necessary as technology evolves.
The Future of Science and Artificial Intelligence
The future of science and artificial intelligence is a pivotal topic in todayβs research landscape. As AI technologies advance, they are increasingly interwoven with scientific inquiry, leading to greater innovations and efficiencies. Understanding this relationship is essential for leveraging the full potential of AI. By examining emerging technologies, the evolution of interdisciplinary research, and public perception, we can gain insights into how the future will be shaped by these developments.
Emerging Technologies and Innovations
Emerging technologies driven by AI are set to transform many scientific fields. For example, algorithms designed for machine learning are refining data analytics and interpretation. These tools can analyze vast datasets at speeds unattainable by humans, discovering patterns and insights that would otherwise go unnoticed. Furthermore, advancements in neural networks are enhancing image recognition, impacting fields like medical imaging and environmental monitoring.
Innovations such as quantum computing are also relevant. They promise to solve complex scientific problems much faster than classical computers. This potential can significantly expedite research in chemistry, physics, and materials science.
Ultimately, emerging technologies create new opportunities for exploration and understanding, already reshaping how research is conducted across disciplines.
The Evolution of Interdisciplinary Research
The fusion of science and artificial intelligence encourages a paradigm shift toward interdisciplinary research offerings. Researchers are now collaborating across disciplines to tackle complex questions. For instance, a biologist might work with a computer scientist to model biological systems using AI. This creates a new approach to understanding life sciences, leading to breakthroughs in areas like genetics and biotechnology.
AI also facilitates communication between diverse fields. Tools for data sharing and simulation make it easier for researchers from different backgrounds to engage and exchange ideas. As such, interdisciplinary research not only fosters innovation but also produces more holistic solutions to pressing global challenges, such as climate change and public health crises.
Public Perception and Acceptance of AI
A critical factor shaping the future of AI in science is public perception. Trust in AI technologies impacts collaboration between scientists and the general public. There is a growing concern regarding how AI influences decision-making in critical scenarios, including healthcare and environmental policy. Studies indicate that transparency in AI operations is essential to foster this trust.
Educating the public about AI's benefits and limitations is vital for acceptance. People require clarity on how their data is used and how AI systems make decisions. Open discussions and community engagement can help demystify AI and reduce fears surrounding its applications.
In summary, the future of science and artificial intelligence is contingent upon advancements in technology, collaborative research efforts, and public sentiment. By addressing these elements, we can optimize the integration of AI into scientific endeavors, ultimately ensuring its responsible and effective use.
Ending
The conclusion of this article serves as a essential capstone, synthesizing the insights delved into in previous sections. It underscores the significance of understanding the intertwining of science and artificial intelligence. It is increasingly evident that AI is not simply a tool but a transformative force in scientific inquiry, reshaping methodologies and outcomes across various disciplines.
Summary of Key Insights
At the heart of our discussion are critical insights articulating how AI is shaping scientific research. Key points include the substantial role of AI in data analysis, predictive modeling, and the automation of essential processes. Additionally, the examination of distinct applications in life sciences, physics, and computer science highlights the breadth of AI's impact.
Moreover, ethical considerations surrounding AI use in science were thoroughly addressed. Key insights suggest that while AI offers tremendous potential for advancements, it also necessitates a careful approach towards data quality, human oversight, and interdisciplinarity. As scientific inquiry becomes increasingly complex, those insights remain vital for responsible integration of AI technologies.
The Continuing Dialogue Between Science and AI
The relationship between science and AI is not static; it is an evolving discourse. Continuous engagement from researchers, policymakers, and the public is crucial to ensure that AI advancements align with ethical standards and societal needs. Ongoing dialogue is imperative to refine the methodologies, address unforeseen challenges, and harness AI's capabilities responsibly.