Exploring Prediction Engines: Technologies and Impacts
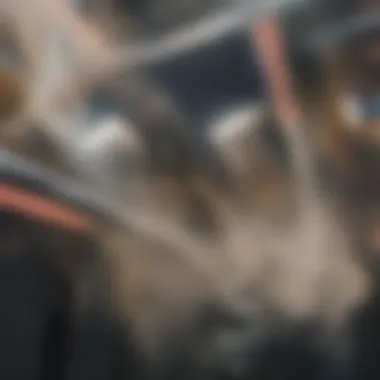
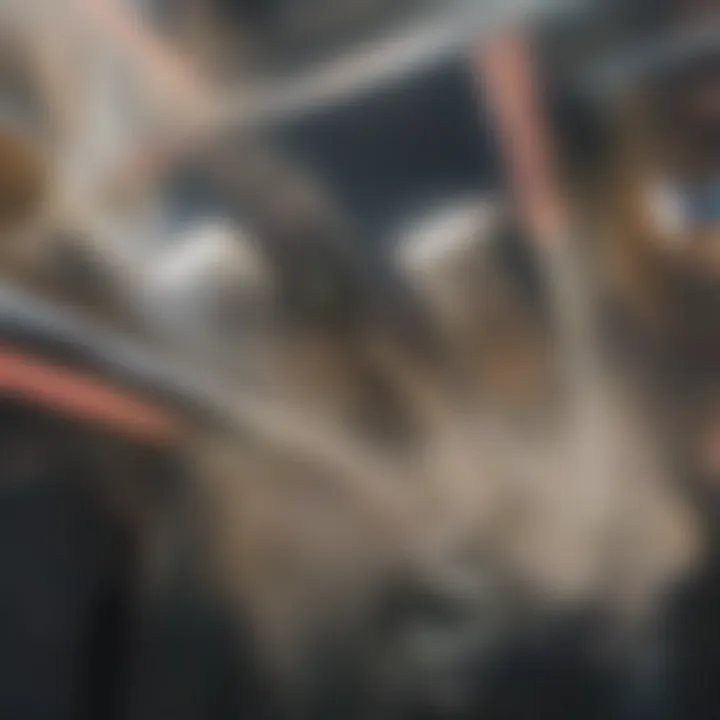
Intro
Prediction engines have emerged as critical tools in various fields, utilizing vast quantities of data to generate insights and forecasts. These engines enable users to anticipate trends, consumer behaviors, and outcomes across different sectors, from finance to healthcare. Understanding their mechanics is essential for students, researchers, and professionals alike, as the implications of these technologies permeate daily operations and strategic decisions.
With a foundation in data science and machine learning, prediction engines operate on complex algorithms and statistical models. The efficiency of these tools relies not only on the sophistication of the underlying technology but also on the quality and breadth of the data fed into them. As businesses and academic institutions increasingly adopt these innovations, it is imperative to unpack the dynamics and applications that make them so powerful.
Key Research Findings
Overview of Recent Discoveries
Recent studies emphasize the role of artificial intelligence and machine learning in refining prediction engines. Techniques such as supervised learning, unsupervised learning, and reinforcement learning have accelerated the development of more efficient models. For instance, advancements in natural language processing enable better context understanding, improving predictions based on textual data.
Moreover, data privacy and ethical considerations have gained prominence. Researchers are exploring methods to enhance transparency and mitigate bias in predictive analytics. This attention on ethics can transform the perception of prediction engines from mere tools to pivotal instruments of responsible decision-making.
Significance of Findings in the Field
The findings illustrate a shift towards more inclusive and nuanced application of prediction engines. In commercial settings, companies use tailored models to predict sales, assess customer loyalty, and manage inventory more effectively. In academia, these models contribute to research in social sciences, epidemiology, and environmental studies, offering quantifiable estimates that can influence policy and practice.
"The integration of predictive analytics is no longer optional; it has become fundamental in understanding complex systems and making informed decisions in real time."
Applications of Prediction Engines
Prediction engines find their relevance across diverse sectors:
- Finance: Risk assessment and portfolio management depend on accurate predictions.
- Healthcare: Patient outcomes can be forecasted to optimize treatment plans and resource allocation.
- Retail: Businesses leverage customer behavior predictions to fine-tune marketing approaches and boost sales.
- Transportation: Traffic flow predictions improve route planning and reduce congestion.
Understanding these applications reveals the versatile nature of prediction engines. Each industry tailors the technology to suit unique challenges and objectives.
Ethical Considerations and Challenges
As the reliance on prediction engines grows, so does the importance of addressing ethical concerns. Data bias can lead to skewed predictions, impacting trust and fairness. Therefore, developing frameworks for accountability and transparency is essential in fostering public confidence.
Moreover, data security poses another challenge. Protecting the information used in predictions is vital to maintain user privacy and comply with regulations. Organizations must implement robust security measures while harnessing the power of prediction technology.
Intro to Prediction Engines
Prediction engines are vital tools in todayβs data-driven world. They harness vast amounts of data to make informed predictions and recommendations across different sectors. Understanding prediction engines is essential. They influence decision-making processes in areas like healthcare, finance, and marketing. Their relevance grows as more domains rely on data analytics for better outcomes and competitive advantages.
Defining Prediction Engines
A prediction engine is a system designed to analyze data and produce predictions about future events or behaviors based on historical patterns. These engines integrate various technologies to process data. At the core, they rely on algorithms to interpret information and make predictions. They can forecast sales, recommend products, or assess risks.
The term encompasses several models and approaches, including machine learning and statistical techniques. For example, a retail prediction engine may analyze purchasing behavior to suggest products to customers. Such engines not only reflect trends but also anticipate future actions, achieving a foresight that is increasingly valuable in many industries.
Historical Context and Evolution
The evolution of prediction engines dates back several decades. Initial models relied on basic statistical methods and were limited by the available data. As computational power increased, more complex algorithms were developed. The rise of the internet greatly expanded data accessibility, enabling a shift toward big data analytics.
In the 1990s, advancements in machine learning and data mining began to reshape the field. New techniques, such as decision trees and neural networks, gained popularity. These advancements broadened the scope of prediction engines dramatically. Today, they are an integral part of business strategies across the globe, from healthcare diagnostics to stock market predictions. Their progress continues, promising even greater capabilities in the future.
Core Components of Prediction Engines
The core components of prediction engines are essential for understanding how they function effectively across different applications. These components work synergistically to enable predictive capabilities. Understanding the intricacies of these components, such as data acquisition, data processing techniques, and algorithms, is vital for utilizing prediction engines to their fullest potential.
Data Acquisition
Data acquisition is the first step in the lifecycle of prediction engines. It involves gathering relevant data from various sources, which can include databases, APIs, sensors, or user-generated content. The quality and quantity of data directly impact the predictive accuracy. Inadequate data can lead to unreliable predictions, while comprehensive datasets can enhance model performance. Thus, establishing efficient data pipelines is crucial for sustained success.
Considerations include:
- Diversity of sources: Data should be collected from multiple sources to ensure robustness.
- Real-time capabilities: The ability to acquire data in real-time allows for timely predictions that are highly relevant in dynamic environments like financial markets.
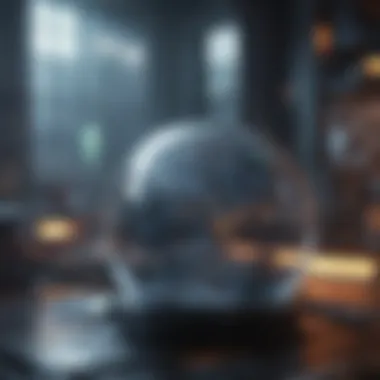
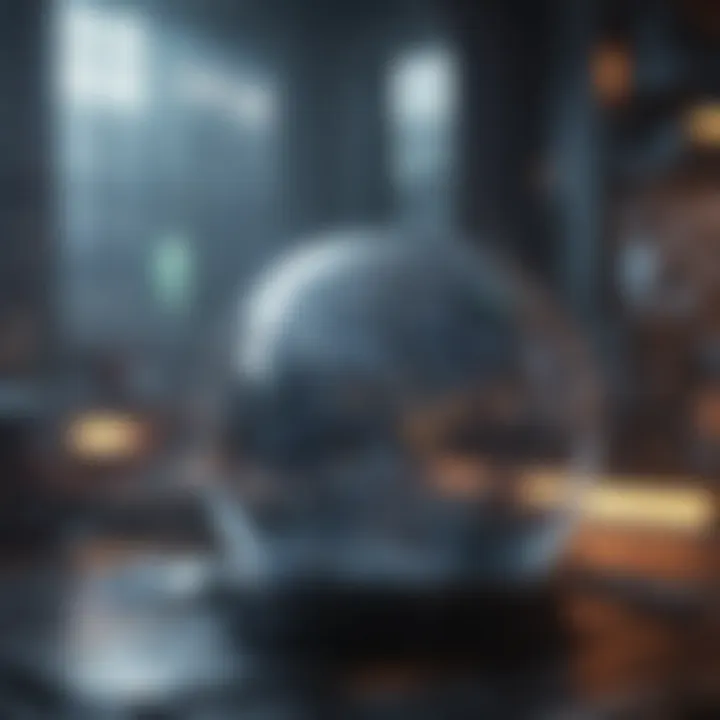
Data Processing Techniques
Once data is acquired, it must be processed to derive meaningful insights. Data processing techniques are fundamental in cleaning and transforming data into a usable format. This step helps in identifying patterns, outliers, and trends that are significant for prediction. Several techniques can be utilized during this phase, including data normalization, aggregation, and transformation.
The primary goals are:
- Improving data quality: Cleaning data eliminates inaccuracies that can skew predictions.
- Enhancing data usability: Processes like normalization make datasets comparable across different scales.
Algorithms and Models
Algorithms and models form the backbone of prediction engines. They are the tools that take processed data and apply mathematical procedures to produce predictions. Different algorithms can be utilized depending on the specific predictive task.
Machine Learning Algorithms
Machine learning algorithms have gained a lot of traction in recent years. They excel in finding patterns within large datasets without explicit programming. This ability makes them particularly well-suited for a variety of prediction tasks, from finance to healthcare.
A key characteristic of machine learning algorithms is their adaptability. They improve over time as they process more data, making them a popular choice in dynamic environments. It allows victory against static statistical models which might fail to capture evolving trends.
One unique feature of machine learning algorithms is their capability for non-linearity. This means they can model complex relationships between variables that traditional models might miss. However, they can also lead to challenges such as overfitting, where a model performs exceptionally well on training data but poorly on unseen data.
Statistical Models
Statistical models, on the other hand, rely on mathematical theories and statistical methods to make predictions. They are grounded in rigorous mathematical foundations, typically providing higher interpretability than machine learning algorithms.
These models are valuable due to their predictive validity in controlled contexts. They allow users to test hypotheses about relationships within the data in a straightforward manner. A distinct advantage of statistical models is their computational simplicity, which can be a crucial factor in scenarios with limited available data resources.
Yet, they may not capture complexity as well as machine learning approaches. Consequently, understanding both types provides a comprehensive view in prediction engine development.
Key Takeaway: The choice between machine learning and statistical models should align with the specific requirements of the prediction task, as both offer unique strengths and weaknesses.
Types of Prediction Engines
Predictive engines are essential tools in various industries, allowing organizations to leverage vast amounts of data for better decision-making. Understanding the different types of prediction engines is crucial. Each type serves unique purposes and utilizes distinct methodologies, affecting their effectiveness and applicability.
Predictive Analytics Engines
Predictive analytics engines utilize historical data to forecast future outcomes. These engines provide valuable insights that help organizations make informed decisions. They are commonly used in industries like finance, healthcare, and retail.
The functioning of predictive analytics engines involves several key processes:
- Data collection: Gathering data from multiple sources is vital. This can include customer interactions, sales data, or even social media activity.
- Modeling: Once data is collected, various statistical and machine learning methods are applied to extract patterns. This may involve regression analysis or classification techniques.
- Scoring: After creating a model, the predictive analytics engine scores or ranks the likelihood of certain outcomes based on new data inputs.
By enabling companies to anticipate future trends and behaviors, predictive analytics engines contribute significantly to strategic planning.
Recommendation Systems
Recommendation systems are specialized prediction engines tailored to suggest products or services to users. They are pivotal in enhancing user experience and driving sales in e-commerce and content platforms. These engines analyze user behavior, preferences, and historical interactions to generate recommendations.
Key components of recommendation systems include:
- Collaborative Filtering: This method relies on the behavior of similar users to recommend items or content. For example, if user A and user B have similar tastes, and user A likes a new movie, it is likely that user B will enjoy that movie too.
- Content-Based Filtering: In contrast, this approach focuses on the characteristics of the items themselves. If a user has positively rated action movies, the system might recommend more action movies based on their features.
Recommendation systems not only provide personalization but also enhance user engagement and retention.
Forecasting Tools
Forecasting tools are designed to predict future events based on historical data. These tools are critical in various sectors, such as supply chain management and weather prediction. They utilize various statistical methods to project future trends and behaviors.
The significant aspects of forecasting tools include:
- Time Series Analysis: This technique involves analyzing data points collected over time. Time series forecasting is often used in financial markets to predict stock prices.
- Scenario Planning: Forecasting tools can also model different scenarios based on varying inputs to prepare for potential outcomes. This is crucial for strategic planning in uncertain environments.
Overall, forecasting tools enable organizations to be proactive rather than reactive, improving their ability to navigate future uncertainties.
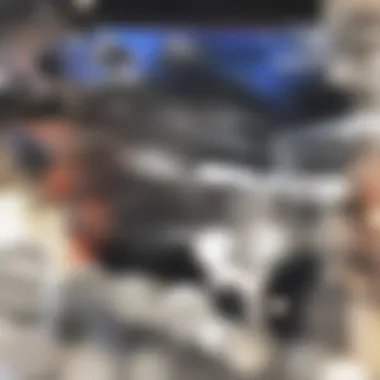
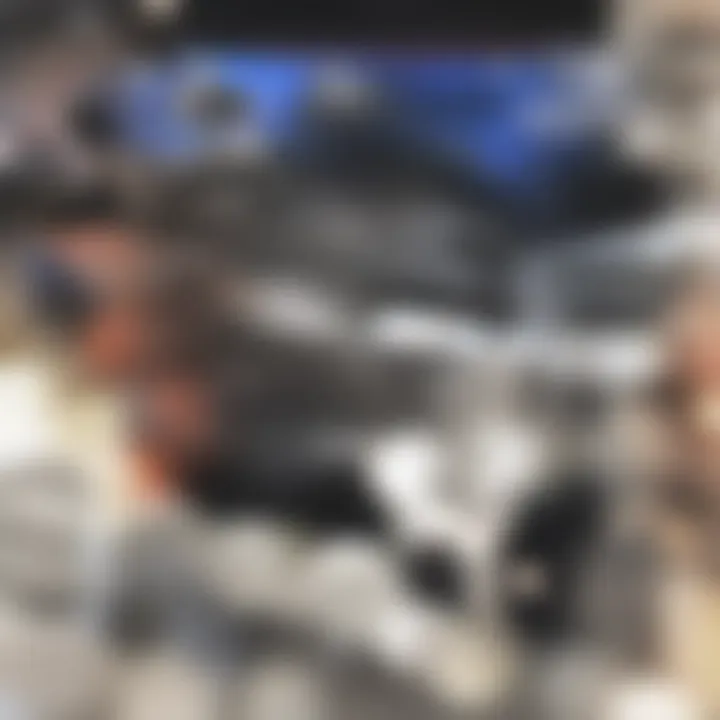
Applications in Various Domains
The applications of prediction engines span numerous sectors, illustrating their significance in shaping decision-making processes. By leveraging data-driven insights, businesses and organizations can enhance their operations, improve services, and ultimately boost profitability. Prediction engines facilitate the transformation of raw data into actionable information, enabling a variety of applications that address complex problems in real-time.
The key areas where prediction engines prove valuable include healthcare, finance, marketing, and supply chain management. Each domain presents unique challenges and opportunities for utilizing advanced models to generate accurate forecasts. This section discusses the influence and implications of prediction engines across these critical fields.
Healthcare and Medicine
In healthcare, prediction engines have a profound impact on patient care and treatment outcomes. They analyze large volumes of clinical data to help identify potential health risks before they escalate. Use cases include predicting disease outbreaks, personalizing treatment plans, and optimizing hospital resource management. For instance, machine learning algorithms process historical patient data to forecast future health trends, allowing healthcare providers to allocate interventions more effectively.
Moreover, predictive analytics is also utilized in operational management within medical facilities. By forecasting patient admission rates, hospitals can better manage their staffing and inventory, resulting in lower costs and improved professionalism in service delivery.
Finance and Investment
The finance sector leverages prediction engines to mitigate risk and identify investment opportunities. Financial institutions deploy these tools to predict stock price movements, assess credit risk, and enhance fraud detection. Complex algorithms analyze historical market data and economic indicators to generate insights that inform investment strategies.
For example, hedge funds and investment firms utilize predictive models to make data-backed decisions regarding asset allocation. This use not only aids in maximizing returns but also helps in minimizing potential losses, a crucial aspect in today's volatile markets. Additionally, algorithmic trading practices have revolutionized how trades are executed, relying on real-time data processing for rapid decisions.
Marketing and Consumer Insights
In the realm of marketing, prediction engines are essential for understanding consumer behavior. They analyze purchasing patterns, preferences, and engagement metrics to develop tailored campaigns. By forecasting future buying trends, businesses can align their marketing strategies to meet consumer needs efficiently.
Moreover, recommendation systems, part of many prediction engines, enhance customer experiences by suggesting products that align with individual preferences. For instance, Amazon uses recommendation algorithms to suggest items based on past purchases, increasing customer satisfaction and sales conversions.
Supply Chain and Inventory Management
Supply chain dynamics benefit significantly from prediction engines through enhanced inventory control and demand forecasting. Businesses utilize predictive analytics to optimize stock levels, minimizing excess inventory while avoiding stockouts. By analyzing past sales data and current market trends, predictions help suppliers plan and adjust their production schedules accordingly.
For example, companies like Walmart employ predictive analytics to ensure their inventories align with anticipated customer demand, allowing for a more efficient supply chain. Such insights contribute to reduced operational costs and improved service levels, critical components of maintaining a competitive edge in todayβs marketplace.
"Prediction engines allow organizations to convert data into strategies that address real-world challenges across various domains."
Understanding these applications not only highlights the versatility of prediction engines but also underscores their role as pivotal tools in driving innovation and efficiency across multiple sectors.
Ethical Considerations
When discussing prediction engines, ethical considerations emerge as a crucial topic. These considerations cover a range of aspects that need careful thought and action as the technology evolves. Ethical implications are increasingly important to ensure that systems built on prediction engines function fairly and do not perpetuate existing societal biases or inequities.
Data Privacy and Security
Data privacy is one of the most pressing ethical concerns regarding prediction engines. As these systems rely heavily on user data for model training and analysis, safeguarding this data becomes paramount. Users must have confidence that their private information is not being misused or inadequately protected. Legal frameworks, such as the General Data Protection Regulation (GDPR), emphasize the necessity for companies to handle personal data transparently. Security measures must be put in place to prevent data breaches, which can lead to severe repercussions for individuals and organizations alike. Ensuring secure storage and limited access to sensitive data is essential.
Bias in Algorithms
Bias in algorithms poses another significant ethical dilemma. Prediction engines often inherit biases present in the training data. If historical data contains discrimination based on race, gender, or socioeconomic status, the models may produce biased outputs that reinforce these inequalities. This issue highlights the need for researchers and developers to critically assess and audit datasets before utilizing them. Implementing real-time monitoring tools can also help identify and mitigate bias as it arises. This process is relevant not only to create fair systems but also to build trust among users that the predictions made are unbiased and reflect an accurate representation of reality.
Accountability and Transparency
Accountability and transparency are fundamental ethical principles necessary in the deployment of prediction engines. Stakeholders involved in designing and implementing these systems must be aware of their responsibility in ensuring ethical outcomes. Users need insight into how predictions are made, especially in high-stakes scenarios like healthcare, finance, and justice. This understanding requires organizations to provide clear documentation on how algorithms function, including their strengths and limitations. > "Transparency builds trust and enables users to make informed decisions about reliance on prediction engines." Moreover, establishing accountability mechanisms, such as auditing processes, can ensure that any problematic outcomes can be addressed and corrected swiftly.
Engaging with these ethical considerations is essential for the responsible use of prediction engines. By prioritizing data privacy, addressing algorithmic bias, and committing to accountability and transparency, developers can create systems that generate positive and fair outcomes for all stakeholders.
Challenges in Implementing Prediction Engines
The implementation of prediction engines is not without its own set of hurdles. Various factors contribute to the complexity and difficulty of this process. Understanding these challenges is essential as they directly affect the effectiveness and reliability of prediction models. Below, we delve into three principal challenges: data quality issues, integration with existing systems, and performance limitations.
Data Quality Issues
Data quality is a fundamental aspect of developing effective prediction engines. Poor quality data can negatively influence the accuracy and reliability of predictions. Several elements determine data quality, including accuracy, completeness, consistency, and timeliness.
When data is incorrect or incomplete, the predictions generated by the engine can lead to misguided business decisions.

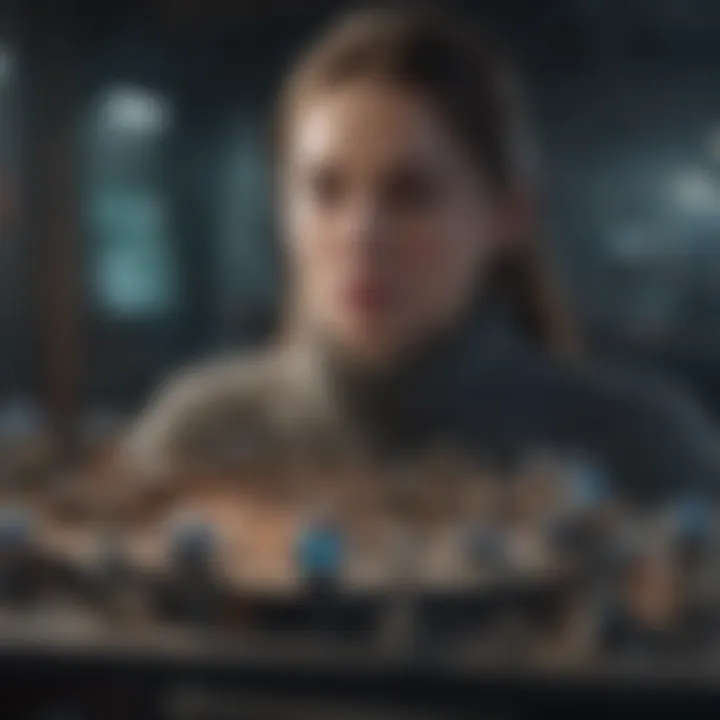
Furthermore, data may come from disparate sources, which can introduce inconsistencies. To address these issues, organizations must invest in robust data cleansing processes. Effective data management protocols can help ensure that the data used is high-quality and suitable for analysis.
Integration with Existing Systems
Integrating prediction engines with existing systems poses another set of challenges. Many organizations use legacy systems that may not be designed to accommodate modern prediction technologies. This misalignment can result in technical difficulties and inefficiencies.
Organizations must carefully evaluate their current technical infrastructure before implementing a prediction engine. The process often requires significant modifications to existing systems or even complete replacements. In addition, ensuring seamless communication between different systems is necessary. This integration challenge highlights the necessity for a comprehensive strategy during the initial planning phases.
Performance Limitations
The performance of prediction engines can be limited by various factors, including computational efficiency and scalability. High volumes of data require significant computational power to analyze effectively, which can lead to delays in generating predictions.
Moreover, as the complexity of models increases, so does the need for more processing power. Organizations often face the challenge of balancing performance and resource utilization. If the engine cannot scale effectively with growing data needs, it will be less effective in delivering timely insights.
Addressing performance limitations requires not only technical solutions but also strategic planning to meet the evolving demands of the application space.
Future Trends in Prediction Engine Technology
As prediction engines continue to evolve rapidly, understanding their future trends is essential. These developments promise to reshape how industries operate, enhance decision-making processes, and leverage data more effectively.
Advancements in AI and Machine Learning
The next wave in prediction engine technology primarily hinges on advancements in artificial intelligence and machine learning. These technologies provide a robust foundation for enhancing the capabilities of prediction engines. By employing deeper and more complex algorithms, prediction engines can improve their accuracy by learning from larger datasets.
Deep Learning is a significant factor in this evolution. It allows for the handling of unstructured data, such as images and text, offering insights that traditional methods often miss. Utilizing neural networks, machines can extract patterns and trends from this data more efficiently, leading to more informed predictions.
Moreover, natural language processing (NLP) plays an important role. By analyzing how humans communicate, NLP can enhance user interfaces, making interactions with prediction engines more intuitive and effective. As more industries acknowledge the potential of AI and machine learning, the integration of these technologies into prediction engines will likely accelerate.
Real-time Prediction Capabilities
Real-time prediction capabilities mark another significant trend in the realm of prediction engines. With the advent of big data and better processing technologies, organizations demand instant insights. This need fuels the push for systems that can process and analyze data simultaneously, allowing for immediate predictive outputs.
This move towards real-time prediction has clear benefits:
- Operational Efficiency: Real-time data processing enables quicker decision-making, ultimately improving productivity.
- Enhanced Customer Experience: Businesses can tailor services and products based on live data, significantly increasing customer satisfaction.
- Risk Mitigation: Immediate insights allow companies to anticipate challenges swiftly, enabling them to act decisively.
To capitalize on this trend, companies often incorporate powerful cloud services that offer high-frequency data processing, further enhancing their ability to operate in dynamic environments.
Interdisciplinary Approaches
As prediction engine technology matures, interdisciplinary approaches will become more prominent. Fields like sociology, psychology, and economics will increasingly inform the development of prediction models. By integrating diverse methodological frameworks, prediction engines can refine their predictive capabilities, drawing on a more holistic understanding of complex systems.
For example:
- Market Analysis: Combining economic theories with advanced analytics to better understand consumer behaviors.
- Healthcare Insights: Using sociological research to develop better models for patient prediction, addressing social determinants of health.
- Environmental Predictions: Engaging with ecological data to predict climate-related impacts more accurately.
Such approaches emphasize the importance of collaboration across disciplines, enriching the framework within which prediction engines operate.
The future of prediction engines is poised for remarkable innovation, with AI, real-time capabilities, and interdisciplinary strategies paving the way for their evolution.
These trends signify a profound shift in how organizations can leverage prediction engines, enhancing both their efficacy and societal impact. Understanding these dynamics will be crucial for those looking to navigate the future landscape of technology.
Epilogue
In exploring the realm of prediction engines, it becomes clear that their significance extends beyond mere technological innovation; they are pivotal in shaping modern decision-making processes across various fields. This conclusion synthesizes key insights while elucidating the importance of continued research in this dynamic area.
Summary of Key Insights
Prediction engines serve as sophisticated systems that harness data to generate insights and forecasts. With applications ranging from healthcare to finance, they optimize various processes by analyzing patterns and predicting outcomes. The main takeaways from our discussion include:
- Data Centrality: The efficacy of prediction engines hinges on the quality and volume of the data they utilize. Reliable data sources enhance accuracy and reliability in predictions.
- Algorithm Diversity: Different domains may require diverse algorithms to achieve optimal predictions. Machine learning techniques and statistical models each have unique advantages applicable in specific contexts.
- Ethical Implications: As these technologies become more integrated into daily functions, ethical concerns, including data privacy and algorithmic bias, warrant serious consideration. Implementing transparent practices can help mitigate potential harms.
It is important to recognize these elements as foundational to the understanding and successful deployment of prediction engines.
The Importance of Continued Research
As the landscape of prediction engines continues evolving, ongoing research is essential. Here are several reasons why:
- Adaptation to New Challenges: The rapid pace of technological advancement necessitates constant refinement of existing algorithms and development of new ones to address emerging challenges, particularly in areas like AI maturity.
- Mitigation of Bias: Research can guide the identification and reduction of inherent biases in algorithms. This is especially critical as prediction engines become more prevalent in sensitive areas such as criminal justice and healthcare.
- Interdisciplinary Insights: By integrating knowledge from various fields, researchers can develop more robust prediction models. Interdisciplinary approaches can foster innovation and enhance the practical applicability of these engines.