Exploring Big AI: Implications and Future Directions
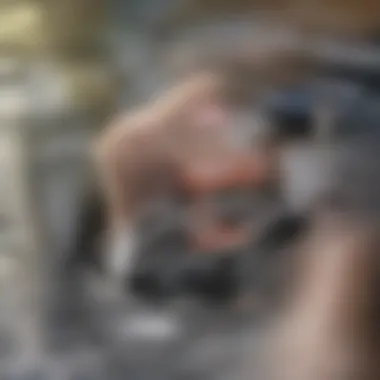
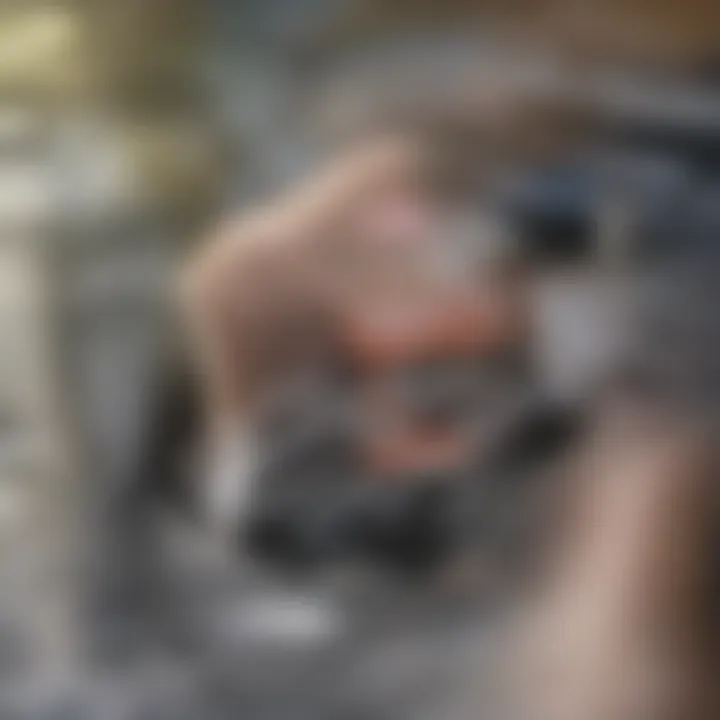
Intro
The landscape of artificial intelligence is rapidly evolving, particularly with the advent of big AI. This term, signifying the utilization of vast datasets and advanced algorithms, prompts new questions and opportunities across various domains. As we venture deeper into this chapter of technology, understanding the implications and challenges that accompany big AI is paramount. The field is not just about the intelligence within machines; it also encompasses ethical dilemmas, regulatory considerations, and potential societal impacts. By dissecting these elements, we can assemble a clearer picture of what the future may hold.
Key Research Findings
Overview of Recent Discoveries
Recent studies highlight that big AI systems can outperform traditional ones in areas like natural language processing, computer vision, and even predictive analytics. For instance, models like OpenAI's GPT-3 demonstrate how AI has progressed, enabling nuanced human-like interactions. Furthermore, developments in deep learning and reinforcement learning have expanded the boundaries of what AI can achieve.
Significance of Findings in the Field
The significance of these findings cannot be overstated. Big AI not only revolutionizes how industries operate but also challenges existing regulatory frameworks. The capability of AI systems to learn and adapt raises pertinent questions about data privacy and bias in algorithmic decisions. As such, researchers and policymakers must work closely to address these challenges, ensuring that technologies benefit society as a whole.
"Big AI has the potential to redefine numerous industries, but ethical considerations are just as crucial to its progression."
Implications of Big AI
Ethical Challenges
The ethical landscape surrounding big AI is complex. Issues such as data sovereignty, algorithmic accountability, and transparency are critical. The more integrated AI becomes in our daily lives, the more profound is its impact on personal privacy and public safety. Addressing these challenges requires a cohesive effort involving technologists, ethicists, and lawmakers.
Economic Impact
The economic implications of big AI are significant. Industries are increasingly adopting AI tools to optimize processes, reduce costs, and innovate products. Yet, there is potential for job displacement as automation becomes more prevalent. Balancing economic growth with workforce transition is a task that demands attention and planning.
Future Directions
Envisioning the future of big AI involves examining emerging technologies, such as quantum computing and advanced robotics. These developments could further enhance AI capabilities and introduce novel applications. Simultaneously, ongoing discussions about ethical AI and equitable access will shape the regulatory landscape that governs its deployment.
The End
Big AI holds tremendous promise but also presents multiple challenges. By thoroughly exploring its implications, researchers, students, and professionals can contribute to a better understanding of how to navigate this intricate domain. Collaboration across disciplines will be essential to ensure that big AI serves the interests of society while fostering innovation.
Intro to Big AI
The dynamic landscape of artificial intelligence continues to expand, epitomizing the significance of Big AI in modern technology. This section frames the discussion for understanding the profound changes that Big AI brings forth. An exploration of Big AI is not just timely; it is essential. Understanding its implications aids in navigating various sectors where these systems proliferate.
Defining Big AI
Big AI refers to the large-scale deployment of artificial intelligence technologies that process vast amounts of data to generate insights, automate tasks, and enhance decision-making. Unlike traditional AI, Big AI is characterized by its capability to learn from diverse datasets on an unprecedented scale. This concept embraces a range of technologies, including machine learning, neural networks, and natural language processing.
A clear definition is crucial, as it helps stakeholders recognize the potential of Big AI in various applications. For instance, companies can leverage Big AI for improved customer experiences or operational efficiencies. The reach and capacity of Big AI carve a pathway for innovative solutions.
Historical Context
Understanding the roots of Big AI is crucial to grasp its current trajectory. AI development dates back to the mid-20th century, but the notion of Big AI emerged in the early 21st century with advances in computing power and the availability of massive datasets. The enhancement of algorithms, particularly deep learning, played a pivotal role in catalyzing this evolution.
The increasing penetration of the internet has facilitated unprecedented access to data. Large organizations began investing heavily in AI technologies to gain insights and drive strategic initiatives. For example, companies like Google and Amazon demonstrated the efficacy of Big AI through personalized recommendations and robust search algorithms. Through these historical lenses, it becomes evident that Big AI is not just an evolution of existing technologies, but a profound shift resonating across industries.
In essence, Big AI represents more than computational advancement; it signifies a revolution in how we understand and interact with technology.
Fundamental Technologies Behind Big AI
The fundamental technologies behind Big AI are core components that enable its functioning and evolution. These technologies lay the groundwork for how artificial intelligence learns, processes information, and ultimately performs tasks at scale. Understanding these technologies is essential not only for practitioners in the field but also for observers who wish to grasp the implications of AI advancements on society and industry.
The significance of these technologies permeates various fields, ranging from healthcare to finance. Each technological element contributes to the system's ability to analyze large data sets, improve efficiency, and provide better insights. Moreover, as we continue to encounter challenges such as data management and ethical issues, the evolution of these technologies remains pivotal.
Machine Learning Algorithms
Machine learning algorithms are at the heart of Big AI. They allow systems to learn from data, identify patterns, and make decisions without being explicitly programmed. There are several types of machine learning algorithms, including supervised learning, unsupervised learning, and reinforcement learning.
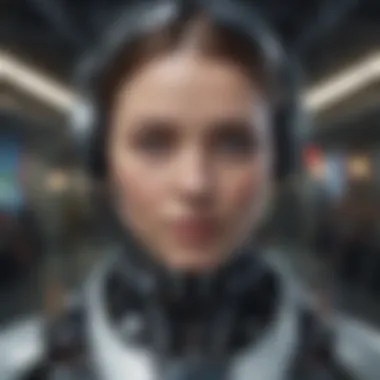
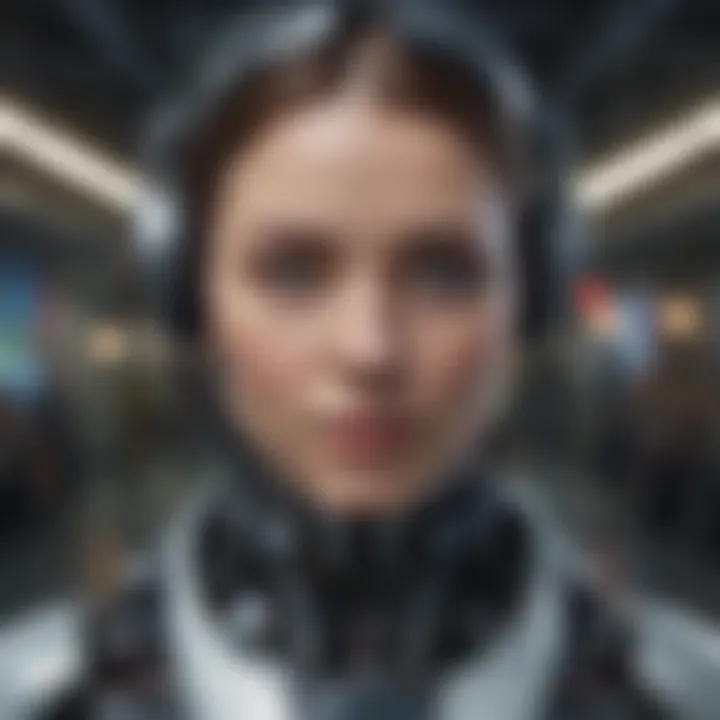
- Supervised Learning: This involves using labeled datasets to train models, where the system learns from examples provided. Applications include image recognition and spam filtering.
- Unsupervised Learning: Here, models work with unlabeled data. The algorithm tries to identify patterns on its own, useful for clustering and association tasks.
- Reinforcement Learning: This focuses on agents that learn to make decisions by receiving rewards or penalties for their actions, applicable in gaming and robotics.
The adaptability of machine learning algorithms makes them crucial for the advancement of Big AI. They continuously improve over time, becoming more accurate as they process more data.
Neural Networks and Deep Learning
Neural networks are inspired by biological brains and consist of interconnected nodes or neurons. Deep learning, a subset of machine learning, employs multi-layered neural networks to analyze vast amounts of data. This technology is essential in tasks such as image and speech recognition.
The depth of these networks allows for complex feature extraction, enabling systems to learn intricate patterns that simpler algorithms cannot capture. For example, in image recognition, deep learning algorithms can discern subtle differences in visual data, leading to higher accuracy rates than traditional methods.
However, deep learning requires significant computational power and large datasets. As such, understanding the infrastructure necessary for such technology is important for effective deployment in practical applications.
Natural Language Processing
Natural language processing (NLP) is vital for facilitating human-computer interaction. This technology enables machines to understand, interpret, and respond to human language. NLP encompasses various tasks, including translation, sentiment analysis, and text generation.
The significance of NLP in Big AI cannot be overstated:
- It bridges the gap between human communication and machine understanding.
- Assists in developing applications like chatbots and virtual assistants, enhancing user experience and accessibility.
- It is essential for processing unstructured data from texts, emails, and social media, offering valuable insights for businesses and organizations.
The intersection of machine learning and NLP has led to remarkable advances, such as the development of sophisticated language models capable of generating human-like text.
Understanding these fundamental technologies allows stakeholders to grasp the core functionalities of Big AI, leading to informed discussions about its future and ethical implications.
Applications of Big AI
The applications of big AI are vast and multifaceted, touching various essential sectors of society. It plays a crucial role in revolutionizing industries by enhancing efficiency, accuracy, and decision-making. The impact of big AI is profound; it helps transform how organizations operate and innovate. This section will elaborate on several significant applications, focusing on their specific merits and practical implications.
Healthcare Innovations
Predictive Analytics
Predictive analytics in healthcare focuses on leveraging data to forecast patient outcomes and trends. It enables healthcare professionals to anticipate disease outbreaks, patient admissions, and treatment responses. This characteristic makes predictive analytics a beneficial tool for proactive healthcare management.
Its unique feature is the use of historical and real-time data to create algorithms that can identify high-risk patients and recommend interventions. One advantage is that it can prevent medical emergencies by allowing preventative measures. However, reliance on data can sometimes lead to overgeneralization, which may not always apply to unique cases.
Diagnostic Tools
Diagnostic tools powered by big AI utilize algorithms to aid in identifying diseases more accurately and speedily. These tools analyze an array of symptoms and test results to assist doctors in their clinical decisions. Their key characteristic is the speed and precision in diagnostics, often surpassing traditional methods.
A notable feature of these tools is their ability to learn and adapt from new data continuously. This ensures that they remain current with the latest medical knowledge. The main advantage is the reduction in diagnostic errors, ultimately leading to better patient care. However, these tools also present a challenge regarding the trust and reliance placed on automated systems by healthcare providers.
Finance and Banking
Fraud Detection
In finance, fraud detection is pivotal for safeguarding consumer assets and corporate integrity. Big AI systems analyze transaction patterns to identify suspicious activities efficiently. This capability is essential for maintaining trust in financial systems.
The key characteristic here is the real-time analysis of large datasets to spot anomalies instantly. Such a method is advantageous because it minimizes the potential losses caused by fraud. Still, reliance on automated fraud detection can lead to false positives, disrupting legitimate transactions.
Algorithmic Trading
Algorithmic trading employs complex algorithms to execute trade orders based on pre-set conditions. This technique enhances market efficiency and boosts profit potential in financial markets. Its main feature is the ability to process vast amounts of data in milliseconds, allowing traders to capitalize on market movements.
One advantage of algorithmic trading is its capacity to remove emotional influences from trading decisions. However, it also presents challenges, such as market volatility driven by algorithms acting on similar data sets simultaneously.
Transportation Advancements
Autonomous Vehicles
Autonomous vehicles represent a significant leap in transportation technology. They integrate AI to navigate without human intervention, aiming to improve road safety and optimize traffic flow. A key characteristic is their use of sensors and AI algorithms to make real-time decisions based on environmental data.
The advantage of autonomous vehicles is their potential to reduce traffic accidents caused by human error. However, ethical concerns and the readiness of infrastructure to support such technology remain prominent issues.
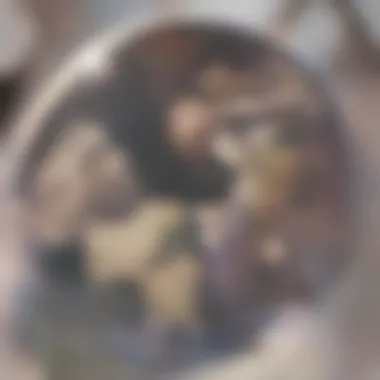
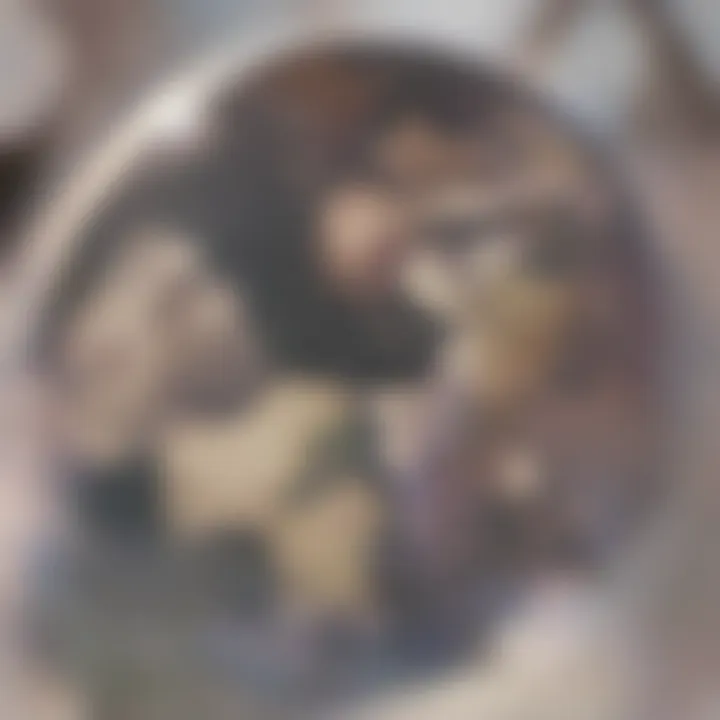
Traffic Management Systems
Traffic management systems utilize big AI to analyze traffic patterns and optimize flow. These systems are essential in urban planning and development, providing solutions for congestion and improving transit efficiency.
Their key feature is the ability to process real-time traffic data for dynamic routing and signal adjustments. The advantages include fewer delays and improved air quality. However, technological dependence also raises concerns about data security and privacy.
Impacts on Communication
Chatbots
Chatbots serve as a primary means of automated communication for businesses. They use AI to engage with customers in real time, providing immediate responses to queries. Their ease of deployment is a critical characteristic, making them a popular choice for enhancing customer service.
A unique feature of chatbots is their ability to handle multiple interactions simultaneously. While they improve efficiency, their limitations in understanding nuanced human emotions can hinder the quality of interactions.
Virtual Assistants
Virtual assistants, like Siri and Alexa, represent personal AI tools for managing everyday tasks. They are designed to help users by setting reminders, answering questions, and controlling smart devices. Their flexibility is a key aspect, making them a valuable addition to personal and professional life.
The main advantage of virtual assistants is that they save time and enhance productivity. However, concerns about data privacy and security continue to spark discussions regarding their implementation in daily use.
The integration and application of big AI across various sectors hold the potential to transform industries, enhance decision-making, and foster innovation while also posing critical ethical and operational challenges.
Ethical Considerations in Big AI Development
The rapid advancement of large-scale artificial intelligence brings forth a myriad of ethical considerations that demand careful examination. Ethical considerations in big AI development are crucial because they influence not only the design and deployment of these technologies but also their long-term implications on society. As AI systems become increasingly integrated into everyday life, understanding these implications can guide responsible innovation. The following subsections will explore several key aspects including bias and fairness, privacy concerns, and accountability and transparency.
Bias and Fairness
Bias in AI systems can arise from multiple sources such as data selection, algorithm design, and human intervention. When datasets used to train AI models reflect existing societal prejudices, the systems can perpetuate or even amplify these biases. For instance, facial recognition technologies have faced criticism for misidentifying individuals from underrepresented groups at disproportionately higher rates. Ensuring fairness in AI means acknowledging these biases and actively working to mitigate their effects.
To address bias:
- Diverse datasets must be employed in training AI systems. This helps create models that are representative of various populations.
- Algorithmic audit techniques can be developed to regularly assess and correct biases within AI systems.
- Implementing regulations that mandate fairness evaluations can uphold ethical standards in AI applications.
Privacy Concerns
With the expansion of AI capabilities, privacy has emerged as a significant concern. AI systems often rely on extensive data collection to train models and enhance functionalities. This collection raises questions about consent and the secure handling of personal information. For example, voice-activated virtual assistants gather continuous data to improve user experience, but may inadvertently record sensitive information without user awareness.
Addressing privacy concerns involves:
- Implementing strict data governance policies that dictate how data is collected, stored, and shared.
- Employing anonymization techniques to protect individuals from potential misuse of their data.
- Advocating for user control in data sharing decisions, allowing individuals to opt out easily.
Accountability and Transparency
As AI systems make decisions that affect human lives, accountability and transparency become essential. Who is responsible when an AI's decision leads to a negative outcome? This question can be complex. Transparency in AI processes can demystify how decisions are made, enhancing the trustworthiness of these systems. For instance, explainable AI aims to provide insights into the decision-making processes of complex models, thereby allowing users to understand and challenge outcomes when necessary.
To enhance accountability and transparency, it is critical to:
- Develop clear guidelines that outline the roles and responsibilities of developers, organizations, and stakeholders in AI deployment.
- Promote transparency standards that encourage companies to disclose information about their models and datasets.
"Ethical considerations in AI are not merely technical challenges; they encompass profound questions about society, equity, and human dignity."
Ensuring ethical practices in big AI development not only protects individual rights but also promotes public trust. As we advance further into this technological era, conscientious efforts must be made to navigate the ethical complexities surrounding AI.
Challenges Facing Big AI
The evolution of big AI systems brings diverse challenges that must be addressed to harness their full potential. These challenges are crucial not just for developers but also for policymakers, businesses, and society at large. They range from technical hurdles such as computational limitations to the softer aspects revolving around public perception. Each element carries implications for the broader integration of AI into everyday life.
Computational Limitations
Big AI systems often require extensive computational resources. The demand for high-performance hardware increases as the complexity of algorithms grows. Training advanced models, like deep learning networks, involves impressive processing power, which can lead to scalability issues. Organizations might face difficulties in securing the necessary infrastructure to support their AI initiatives.
This situation poses significant barriers for smaller entities. They may lack the financial capacity to invest in advanced computing power. Additionally, energy consumption is a concern. The environmental impact of running these extensive systems is a growing worry among stakeholders. Developing solutions that balance performance with sustainability is essential for the future of AI.
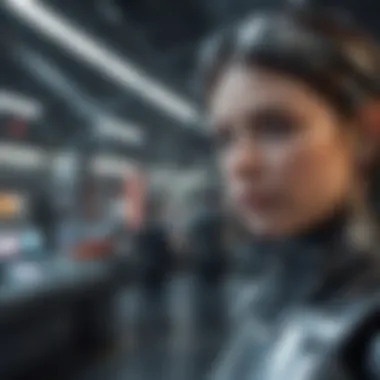
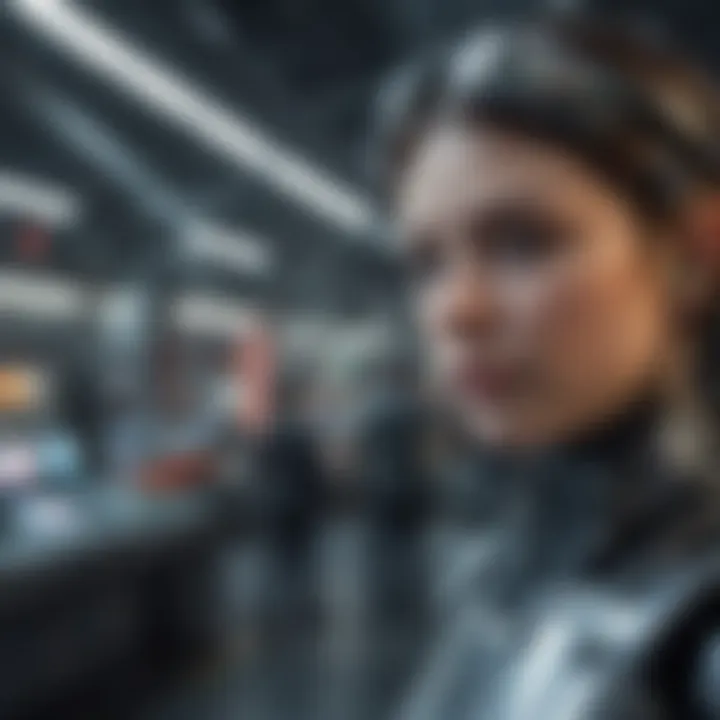
Data Management Issues
Data scarcity and management present another challenge in the big AI landscape. Large datasets are foundational for training effective machine learning models. However, acquiring quality data is not straightforward. Companies often encounter difficulties in obtaining sufficient, relevant, and clean data. Moreover, the sheer volume of data generated raises questions about storage and processing capabilities.
Compliance with regulations, such as GDPR, further complicates data management. Organizations need to ensure that their data practices align with legal frameworks while still leveraging data's full potential for AI-driven insights. Inadequate data governance can lead to ethical issues, including privacy violations and concerns about biased algorithms.
Public Perception
Public perception significantly influences the successful implementation of big AI. Many individuals harbor skepticism or fears about how AI technologies might affect their daily lives. Concerns regarding job displacement, privacy invasions, and the overall trustworthiness of AI systems contribute to resistance against broader AI integration.
Effective communication about AIβs benefits and limitations is crucial for shaping public opinion. Stakeholders must engage in informative dialogues that demystify AI technologies. Educating the public about AI's capabilities can foster understanding and acceptance. Collaborative approaches involving community feedback are essential for addressing concerns and building trust in AI implementations.
"Successfully navigating the challenges of big AI requires transparency and a commitment to ethical practices."
By overcoming these challenges, stakeholders can pave the way for more responsible, effective uses of artificial intelligence, ensuring that its benefits are felt across various sectors.
The Future of Big AI
The exploration of the future of big AI is crucial for understanding its broader implications across multiple sectors. Anticipating how big AI will evolve not only allows for better preparedness but also supports the creation of frameworks that can mitigate potential risks. This future perspective includes examining emerging trends, fostering interdisciplinary collaborations, and aiming for sustainability within AI systems. Each of these facets presents unique opportunities and challenges that influence how society interacts with technology.
Emerging Trends
Big AI is poised to evolve with several emerging trends that merit attention. These trends shape the direction of research, development, and implementation of AI technologies. Some of the most notable trends include:
- Advancements in Explainable AI: There is a sizable push towards creating systems that can explain their decision-making processes. This is important for enhancing trust in AI systems by ensuring users understand how conclusions are reached.
- Federated Learning: This approach allows the training of algorithms across numerous decentralized devices, promoting privacy and security. It minimizes the risk of sensitive data exposure while still producing robust models.
- AI Ethics and Governance: As awareness of ethical challenges increases, organizations are putting a greater emphasis on developing governance structures. Responsible AI practices are becoming a focal point in tech companies and research institutions.
- Human-AI Collaboration: The future will most likely see AI not as a replacement for human labor but as an augmenting tool that enhance productivity across fields. Industries will benefit from human-AI teams that utilize each other's strengths effectively.
These emerging trends signal a resilient and adaptable AI landscape that responds to societal needs while addressing ethical and operational concerns.
Interdisciplinary Collaborations
Fostering interdisciplinary collaborations is vital for the advancement of big AI. No single field has all the answers; instead, it requires input from various domains such as computer science, sociology, psychology, law, and ethics. Collaborative efforts can lead to the following benefits:
- Enhanced Innovation: Different disciplines can provide unique perspectives that fuel innovation. By working together, researchers and developers can create more effective and comprehensive AI solutions.
- Diverse Problem-Solving: Complex challenges associated with AI, like bias and inequity, can be addressed more effectively when experts from differing fields collaborate. A multi-faceted approach can yield solutions that are holistic and pragmatic.
- Broader Knowledge Sharing: Interdisciplinary collaborations foster an environment where knowledge sharing is encouraged. This results in a more informed community that is better prepared to tackle the evolving landscape of AI.
An example of effective interdisciplinary collaboration is the partnership between tech companies and academic institutions, which often leads to groundbreaking research and the development of cutting-edge AI technologies.
Towards Sustainable AI
Sustainability is increasingly recognized as a fundamental principle in the future of big AI. Building AI solutions with sustainability in mind not only enhances their longevity but also minimizes their environmental footprint. Some key aspects of sustainable AI include:
- Energy Efficiency: AI models can be resource-intensive, requiring significant computational power. Developing more efficient algorithms can lessen their environmental impact.
- Responsible Data Use: Data collection and usage should be conducted with sustainability as a priority. This includes developing practices that avoid unnecessary data duplication and promote reuse.
- Long-term Societal Impact: Beyond technology, sustainable AI should account for broader societal consequences. Policymakers must consider how AI systems will influence employment, education, and healthcare in the long run.
The quest for sustainable AI not only aligns technology with ethical practices but also prepares it to address future challenges effectively.
"The future of big AI will unfold in ways we must actively shape through collaboration, ethical considerations, and sustainability initiatives."
Finale
Summary of Insights
In this article, we explored the intricate landscape of big AI, emphasizing its substantial implications and multifaceted challenges. We began by defining big AI, noting its historical roots and the rapid evolution of technologies that have enabled its current capabilities. Through a comprehensive review of fundamental technologies such as machine learning algorithms, neural networks, and natural language processing, we established a foundation for understanding how these systems operate.
The diverse applications of big AI in sectors like healthcare, finance, transportation, and communication were highlighted. Each application carries potential benefits, such as improved diagnostics in healthcare and enhanced efficiency in financial transactions. However, these advancements are not without ethical considerations, including issues related to bias, privacy, and accountability that can arise as these systems are integrated into society.
We also discussed the challenges facing big AI, including computational limitations and data management issues. Public perception poses additional hurdles, often influenced by misunderstandings about the technology. In looking to the future, emerging trends and interdisciplinary collaborations offer paths forward, pushing towards the development of sustainable AI solutions.
To synthesize, understanding big AI requires a balanced view of its benefits and challenges. This perspective is crucial for students, researchers, and professionals alike, as it encourages informed discussions about responsible AI development.
Call for Thoughtful Discourse
As we move forward in this age defined by big AI, it is essential to foster thoughtful discourse surrounding its development and deployment. The intricacies involved call for collaboration across various fieldsβethics, technology, and policy are all critical areas for engagement. Thoughtful discourse can help address the ethical considerations that arise and ensure that big AI serves the interests of society as a whole.
Encouraging conversations among educators, researchers, and industry professionals will deepen collective insights. Discussions should include:
- Effective strategies for mitigating bias in AI systems.
- Regulatory frameworks that protect individual privacy without stifling innovation.
- Collaborative efforts that promote transparency in AI decision-making processes.
By prioritizing such dialogue, we can navigate the complexities of big AI, aiming to maximize its benefits while minimizing its risks. This ongoing conversation serves not only to enhance our understanding but also to shape policies that ensure AI development aligns with societal values.
"Engaging in critical dialogue is essential to harness the true potential of artificial intelligence while safeguarding human interests."