Quantum Computing and AI: Unleashing New Potential
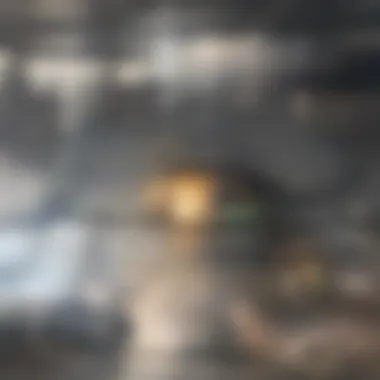
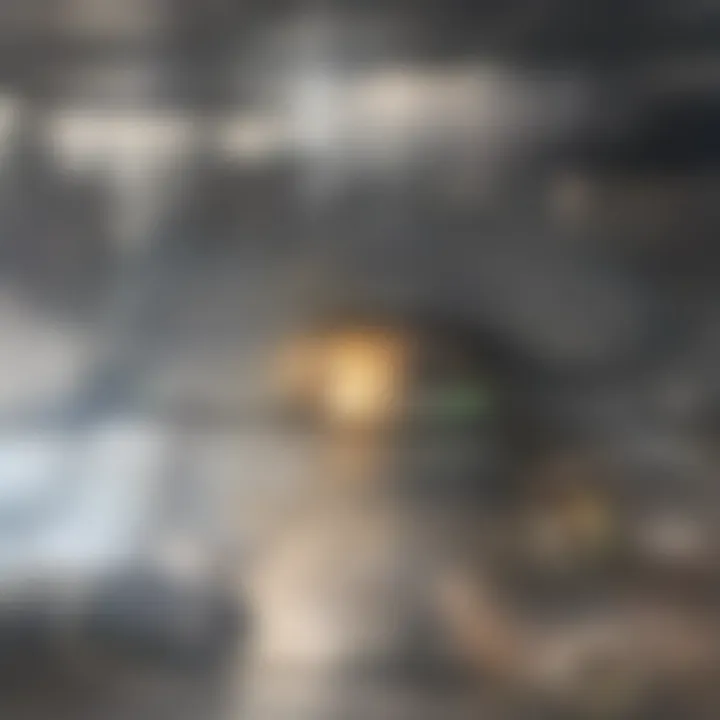
Intro
The convergence of quantum computing and artificial intelligence (AI) presents a paradigm shift in technology and innovation. Each field independently pushes the boundaries of what is possible, but their integration holds immense promise. Quantum computing leverages the principles of quantum mechanics to perform computations that are impractical for classical computers. On the other hand, artificial intelligence seeks to mold machines into entities capable of learning, reasoning, and making decisions. Together, they may redefine capabilities across a range of sectors.
This article investigates how these two powerful realms intersect. It examines significant advancements in both quantum computing and AI. Additionally, it highlights challenges and future prospects, providing a comprehensive overview of their potential synergy.
Key Research Findings
Overview of Recent Discoveries
Recent discoveries in quantum computing have set the stage for breakthroughs in artificial intelligence. Quantum algorithms, such as Grover's and Shor's algorithms, demonstrate the potential for drastically improving data processing speeds and efficiency. These advancements enable handling of complex data sets and optimization problems that traditional systems struggle with.
In AI, research has focused on enhancing machine learning models and neural networks. Incorporating quantum methodologies can enhance these systemsβ learning capabilities. For example, quantum machine learning offers the promise of faster training times and improved pattern recognition.
Significance of Findings in the Field
The significance of these findings is reflected in several domains. For instance, in healthcare, quantum-enhanced AI models could catalyze drug discovery, enabling researchers to analyze molecular structures at lightning speed. Such applications can accelerate innovation and lead to breakthroughs in treatments for diseases.
In finance, the integration of quantum computing can optimize trading strategies, risk assessment, and fraud detection. The ability to compute vast amounts of data instantaneously allows for informed decision-making in real-time market dynamics.
Breakdown of Complex Concepts
Simplification of Advanced Theories
Many concepts in quantum mechanics can be daunting. However, a simplified explanation often helps contextualize their relevance to AI. Quantum bits, or qubits, differ from classical bits by existing in multiple states simultaneously. This property, known as superposition, allows quantum systems to process information at unprecedented speeds.
These characteristics can enhance AIβs capabilities by allowing for more sophisticated computations, leading to better outcomes in machine learning applications.
Visual Aids and Infographics
Visual representation can greatly aid understanding. Infographics illustrating the differences between classical and quantum computing can be beneficial for learners and professionals alike. Here are notable comparisons:
- Classical computing operates on binary bits (0 or 1), while quantum computing uses qubits, leveraging both 0 and 1.
- Data processing in classical systems is linear, whereas quantum systems use parallelism due to superposition, resulting in faster computations.
"In essence, quantum computing is not just another step in computational powerβit is a new way of thinking about information itself."
This statement encapsulates the revolutionary nature of quantum technologies in the context of AI and beyond.
Applications in Various Industries
From cybersecurity to logistics, the applications are vast:
- Pharmaceuticals: Speeding up the process of drug discovery.
- Telecommunications: Enhancing secure communication channels.
- Transportation: Optimizing routes and schedules using complex algorithms.
As we continue to explore this synergistic frontier, the impact of quantum computing on artificial intelligence remains a domain of significant interest and opportunity.
Preamble to Quantum Computing and Artificial Intelligence
The convergence of quantum computing and artificial intelligence represents a frontier with transformative potential across multiple fields. Understanding this topic is essential because both technologies, while distinct, offer unique benefits that can enhance each other when integrated. Quantum computing provides significant computational power that surpasses traditional computing. This ability to process vast amounts of data can lead to enhanced learning algorithms in AI, enabling machines to draw deeper insights from large datasets.
Moreover, the implications are significant for various sectors including healthcare, finance, and logistics. By merging quantum mechanics with AI's learning capabilities, we can tackle complex problems that are currently beyond reach. However, this union also presents various considerations such as ethical concerns, technical limitations, and scalability issues. The following sections delve deeper into defining these technologies and examining their implications in modern research and development.
Defining Quantum Computing
Quantum computing is a paradigm of computation that employs the principles of quantum mechanics. Unlike classical computers, which use bits as the smallest unit of data, quantum computers utilize quantum bits or qubits. Qubits can exist simultaneously in multiple states, thanks to a property called superposition. This means quantum computers can perform complex calculations at unprecedented speeds, especially for tasks that involve large datasets or optimization problems.
Entanglement, another key aspect of quantum computing, allows qubits that are entangled to be correlated with one another. This facilitates the rapid transfer of information across qubits, enabling quantum systems to solve certain computational problems more efficiently than classical counterparts. The capabilities of quantum computing can accelerate machine learning processes and improve algorithmic performance in AI applications.
Understanding Artificial Intelligence
Artificial Intelligence, commonly referred to as AI, encompasses systems that are capable of performing tasks that typically require human intelligence. These tasks include learning, reasoning, problem-solving, and understanding natural language. AI technology is largely rooted in machine learning and neural networks, which enable systems to analyze data and improve over time.
The significance of AI lies in its ability to automate processes, enhance decision-making, and create predictive models. Applications range from image recognition in healthcare to customer service chatbots in finance. As AI continues to evolve, its integration with quantum computing holds promise for even more advanced capabilities, fostering innovation in diverse fields.
"The integration of quantum computing and artificial intelligence could redefine problem-solving and decision-making processes across industries, presenting new opportunities and challenges."
Understanding these foundational concepts is crucial for grasping the broader impact of quantum computing and artificial intelligence in today's technology landscape. Their intersection not only offers advancements in computational efficiency but also poses significant challenges and ethical considerations that must be addressed.
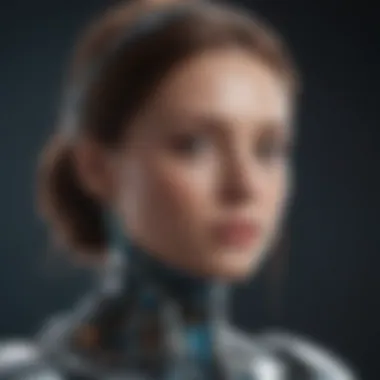
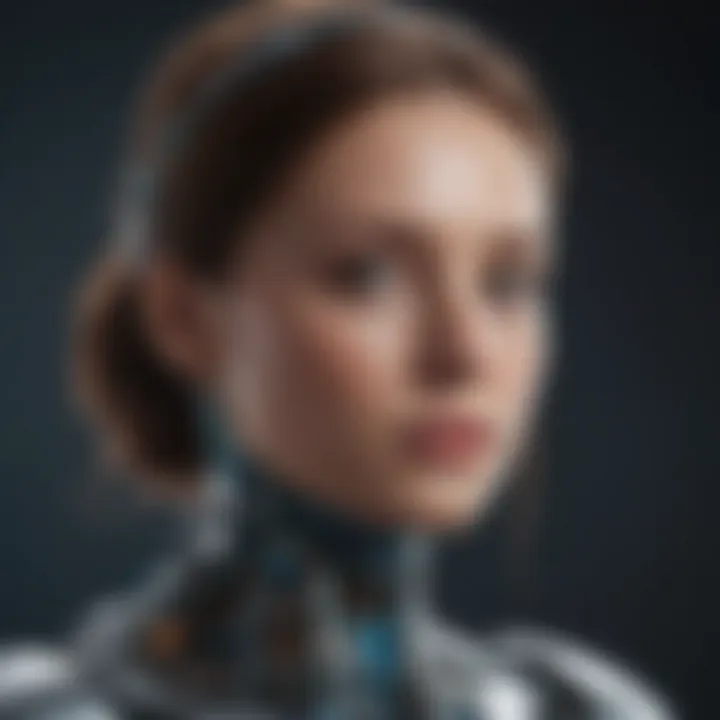
Fundamental Principles of Quantum Computing
Understanding the fundamental principles of quantum computing is crucial for appreciating its potential impact on various fields, particularly artificial intelligence. At its core, quantum computing offers different approaches to processing information, which diverges significantly from classical computing. The two main components that define quantum systems are quantum bits and quantum gates. By grasping these elements, one can begin to explore how they may enhance computational capacities beyond conventional limits.
Quantum Bits and Entanglement
Quantum bits, or qubits, are the building blocks of quantum computing. Unlike classical bits, which can be either 0 or 1, qubits can exist in a state of superposition. Superposition allows qubits to represent multiple combinations of 0 and 1 simultaneously. This characteristic enables quantum computers to perform computations in parallel, vastly increasing their processing power for certain types of calculations.
Moreover, the phenomenon of entanglement connects qubits in a way that the states of one qubit can depend on the state of another, even when separated by large distances. This interdependence means that manipulating one qubit can instantly affect its entangled partner. Such behavior is vital for developing quantum algorithms that efficiently solve complex problems.
Some key advantages of quantum bits and entanglement include:
- Increased computational power: Quantum computers can process vast amounts of data much faster than classical computers.
- Parallel processing: Performing multiple calculations at once leads to improved efficiencies in solving problems.
- Enhanced problem-solving: Quantum systems are particularly adept at certain tasks such as cryptography, optimization, and simulations of quantum physical systems.
"Quantum computing leverages the strange properties of quantum mechanics to enable new computational paradigms that can outperform classical systems in specific domains."
Quantum Gates and Circuits
Quantum gates are the operational components that manipulate qubits. They function similarly to classical logic gates but with the unique advantage of working across multiple qubits due to quantum superposition and entanglement. Each gate performs a specific unitary operation and can transform the state of qubits in various ways through combinations.
A quantum circuit is a sequence of these gates applied to qubits. The design of quantum circuits is crucial, as it determines how effectively a quantum computation will be executed. An important aspect of designing quantum circuits is the consideration of coherence times, which refer to how long qubits can maintain their quantum state before interacting with their environment leads to decoherence.
Key points about quantum gates and circuits include:
- Versatile Operations: Quantum gates can perform a variety of operations, including rotations, measurements, and conditional logic.
- Circuit Design: Crafting efficient circuits is integral for maximizing a quantum computerβs performance, which involves minimizing errors and optimizing gate usage.
- Promise for AI: The design and implementation of quantum circuits can lead to innovative approaches in machine learning, improving the speed and accuracy of data analysis tasks.
Key Techniques in Artificial Intelligence
The significance of Key Techniques in Artificial Intelligence (AI) stems from their ability to extract insights, automate processes, and enhance decision-making across multiple sectors. This article delves into two fundamental components: Machine Learning Models and Neural Networks, both essential in advancing AI capabilities. Understanding these techniques is critical, as they form the backbone for developing applications that can learn from data, adapt, and improve over time.
Machine Learning Models
Machine Learning models are algorithms that facilitate the understanding and analysis of large sets of data. They allow systems to learn without being explicitly programmed for every single task. A few common types of models include supervised, unsupervised, and reinforcement learning.
- Supervised Learning: This involves training a model on labeled datasets. The algorithm learns to predict outcomes based on input data and can achieve impressive accuracy. For example, email filtering is often powered by supervised learning that identifies spam.
- Unsupervised Learning: In this approach, models analyze data without prior labeling. They identify patterns or groupings, which can be beneficial in market segmentation and recommendation systems.
- Reinforcement Learning: Here, agents learn optimal actions through trial and error. Used frequently in robotics and game-playing AI, this approach supports development in environments where the consequences of actions are not immediate.
Machine Learning Models provide the ability to process vast amounts of information quickly and derive patterns that might not be evident to humans. Their impact is felt across various industries, influencing everything from predicting consumer behavior to enhancing operational efficiency.
Neural Networks and Deep Learning
Neural Networks are a pivotal component of AI, inspired by the human brain's architecture. They consist of interconnected nodes (or neurons), allowing them to process input data across multiple layers. This structure is fundamental in Deep Learning, which has gained significant traction due to its ability to handle unstructured data such as images and text.
Interconnected layers enable neural networks to learn increasingly complex features from raw data. For instance:
- Input Layer: Receives the initial data, such as images or audio.
- Hidden Layers: These intermediate layers transform and refine the input, extracting relevant features through weights adjusted during training.
- Output Layer: Produces a final result, such as a classification or prediction.
Deep Learning has led to revolutionary advancements in fields like computer vision, natural language processing, and speech recognition. Applications such as self-driving cars rely heavily on this technology for accurate environmental understanding.
Current Landscape of Research
The intersection of quantum computing and artificial intelligence is becoming a vibrant area of study. Researchers are exploring how these two domains can complement each other. This synergy could strengthen the capabilities of artificial intelligence while pushing quantum computing towards practical applications. Understanding the current landscape helps in identifying which research areas are gaining traction and highlights potential impacts on various industries.
Symbiotic Research Developments
Recent advancements in both quantum computing and artificial intelligence have led to exciting research developments.
- Quantum Machine Learning: This field is gaining attention. Quantum computers possess the potential to process information in ways that classical computers cannot. This advantage can improve machine learning algorithms, leading to faster processing and more accurate models.
- Optimization Processes: New approaches to optimization problems, often faced in AI, are being studied. Quantum algorithms can tackle such issues more efficiently than traditional methods. For instance, quantum algorithms like the Quantum Approximate Optimization Algorithm (QAOA) are being investigated.
- Data Analysis Techniques: Researchers are exploring how quantum computing can enhance big data analytics. Quantum data analytics could unveil patterns and insights that are difficult to achieve with classical computing systems.
These developments underscore the promise that arises from the union of quantum computing and artificial intelligence, reflecting a growing body of work that seeks to understand their interdependencies.
Leading Institutions and Laboratories
Various institutions are at the forefront of exploring the symbiotic relationship between quantum computing and artificial intelligence.
- MIT: The Massachusetts Institute of Technology is deeply engaged in quantum research. They have established the MIT-IBM Watson AI Lab, which focuses on AI's integration with quantum technologies.
- IBM Quantum: The IBM Quantum team is pioneering developments in quantum computing. They provide tools for developers to explore quantum algorithms related to AI applications.
- Google AI Quantum: Googleβs elite researchers are blending their expertise in quantum physics and AI. Their projects aim for breakthroughs in both fields and have the global tech community paying attention.
- University Research Institutes: Many universities have dedicated research groups focusing on quantum AI, such as Stanford and University of California, Berkeley. These institutions are making strides in educational outreach and collaboration among students and researchers.
These institutions exemplify efforts that are critical in shaping the current research landscape. Their dedication signifies a broader commitment to harnessing the advantages of both quantum computing and artificial intelligence.
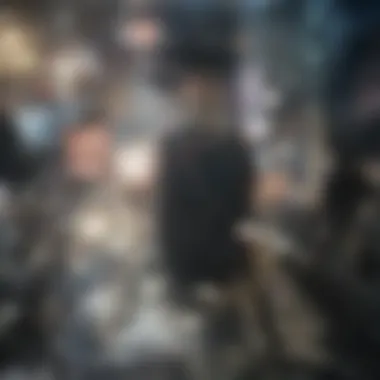
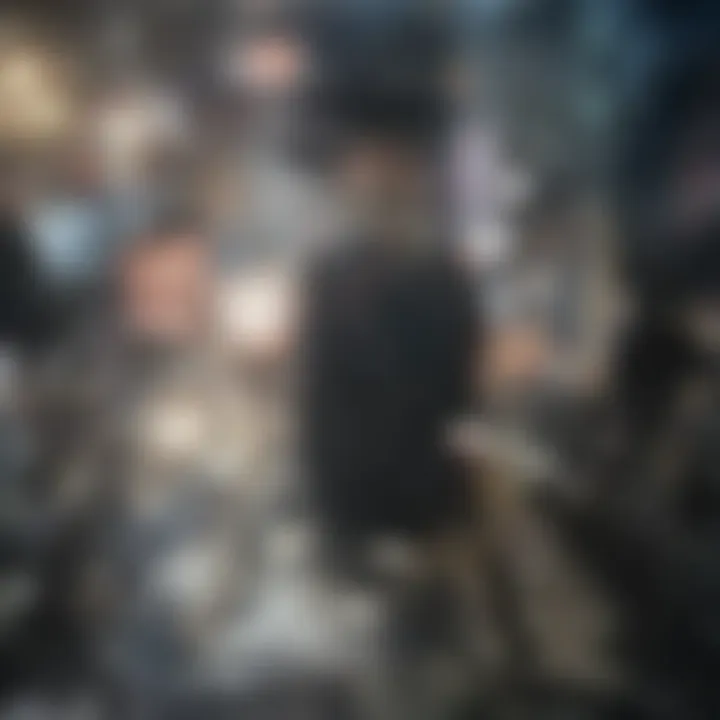
"The integration of quantum computing in AI can potentially reshape industries, providing tools that augment human capabilities."
Overall, the current landscape of research shows a promising path forward as experts from different disciplines come together to investigate how quantum computing can enhance artificial intelligence.
Applications of Quantum Computing in AI
Applications of quantum computing in artificial intelligence stand as a fascinating confluence of two cutting-edge research domains. The integration of quantum technologies into AI processes can offer remarkable enhancements in both efficiency and capability. This section explores the vital ways in which these two fields intersect, highlighting specific applications that can profoundly transform industries and research.
Enhanced Data Processing
Quantum computing promises to revolutionize data processing by leveraging the principles of superposition and entanglement. Classical computers process data in bits that represent either 0 or 1. In contrast, quantum bits or qubits can exist in multiple states simultaneously. This capability allows quantum computers to assess numerous possibilities at once, significantly accelerating the data processing time.
In the context of AI, enhanced data processing enables faster training of machine learning models. For example, quantum computing can manage vast datasets more efficiently. This is especially crucial in areas where data is either too massive or diverse for classical systems to handle promptly. Industries such as healthcare or climate modeling can benefit immensely from such advancements, as they rely heavily on processing complex data patterns.
Another important aspect is the improved performance in optimization problems. Many AI tasks, from neural network training to resource allocation, can be framed as optimization challenges. Quantum algorithms, like the Quantum Approximate Optimization Algorithm (QAOA), can provide solutions much quicker than classical methods. This efficiency can lead to much faster decision-making processes across various industries.
Improving Algorithms
Quantum computing can also enhance existing AI algorithms or even create entirely new ones. Classical AI algorithms often face challenges when dealing with unstructured data or dynamic environments. Quantum-enhanced algorithms can potentially surpass traditional capabilities.
For instance, quantum versions of algorithms such as Grover's search algorithm offer quadratic speedup for database searching tasks. This ability allows for quicker retrieval of relevant information, making it more efficient in training AI models. Furthermore, quantum algorithms can improve neural network architectures, making them more efficient and precise in tasks like image and speech recognition.
Another consideration is the development of hybrid quantum-classical models. These models can utilize quantum computations to optimize certain processes while retaining classical components for other tasks. This dual approach could result in more robust AI solutions, combining the best of both paradigms. Researchers are actively investigating such integrations, with promising initial results reported in several studies.
"Quantum AI has the potential not only to accelerate existing computations but also to open new approaches to learning and decision-making."
In summary, the applications of quantum computing in artificial intelligence hold significant promise. Enhanced data processing and improved algorithms showcase just a fraction of what this integration can achieve. The ongoing research into these applications underscores the transformative potential that this synergistic frontier holds for various sectors, suggesting a fruitful path ahead.
Industry Applications and Case Studies
The intersection of quantum computing and artificial intelligence is revealing transformational possibilities. In various sectors, these technologies exhibit unique capabilities that can lead to significant advancements. Understanding real-world applications and case studies is essential to grasp their potential impact on industries. Here, we focus on two prominent fields: healthcare and finance, illustrating how quantum computing can shape innovative solutions within these domains.
Healthcare Innovations
Quantum computing holds promise for the healthcare industry, particularly in drug discovery and personalized medicine. One critical area is the simulation of molecular interactions. Traditional computing methods struggle with the complexity of quantum systems, but quantum computers can accurately model these interactions, vastly speeding up the discovery of new drugs.
- Drug Discovery: The process of finding new medications is often lengthy and expensive. By leveraging quantum algorithms, researchers can reduce the time it takes to analyze molecular structures, potentially lowering costs significantly.
- Genomic Data Analysis: The ability to process large sets of genetic data is crucial for developing targeted therapies. Quantum computing enables efficient analysis of vast genomic datasets, facilitating personalized medicine tailored to individual genetic profiles.
- Clinical Trials: Optimizing clinical trial designs using quantum algorithms can improve patient selection and outcome predictions. This leads to more effective testing phases, thus accelerating the time to bring new treatments to market.
"Quantum computing stands to revolutionize drug discovery, making it quicker and less costly, ultimately saving lives."
Finance and Risk Assessment
In the finance sector, the integration of quantum computing with artificial intelligence can greatly enhance decision-making processes and risk management strategies. Financial markets exhibit vast complexity that traditional models may not adequately capture. Quantum computing provides a new lens through which to analyze market dynamics.
- Portfolio Optimization: Managing investment portfolios efficiently is crucial for maximizing returns while minimizing risks. Quantum algorithms excel at this task, allowing financial analysts to explore multiple variables in real time and simulate numerous market conditions.
- Risk Analysis: Accurately assessing the risk associated with investment decisions is a perennial challenge. Quantum computing enables financial institutions to compute risk more precisely. By simulating a wide range of scenarios, firms can gain insights into potential losses or gains.
- Fraud Detection: The speed of quantum processing can improve models used for detecting fraudulent transactions. By analyzing patterns and anomalies in real time, financial institutions can react swiftly to potential threats, significantly reducing losses.
Integrating quantum computing with artificial intelligence can yield unprecedented advancements across various sectors. The applications in healthcare and finance are just the beginning. As the technology evolves, further case studies will illuminate additional avenues where quantum AI can drive value, innovate practices, and transform industries.
Challenges of Integrating AI and Quantum Computing
The integration of artificial intelligence (AI) with quantum computing presents a multitude of challenges. These challenges are not only technical but also ethical and societal. Understanding these hurdles is essential for researchers and professionals involved in this rapidly evolving field, as they can hinder or facilitate progress in real-world applications.
Technical Limitations and Scalability
One of the primary concerns in integrating quantum computing with AI relates to technical limitations. Quantum computers are still largely experimental, and their capabilities are limited compared to classical computers. For instance, while quantum bits (qubits) can represent multiple states simultaneously, maintaining coherence among qubits during computation is challenging. This results in error rates that significantly impede large-scale computations necessary for AI applications.
Furthermore, scalability remains a critical issue. Currently, quantum systems are usually designed for small-scale problems. As the problems become more complex, the hardware and software must evolve accordingly. Most quantum algorithms developed so far are theoretical. They require practical implementations that can handle real-world data sizes. Without significant advancements in scalability, the promise of quantum-enhanced AI remains largely untested.
More specifically:
- Error Correction: The need for error correction in quantum systems adds another layer of complexity in designs. Classical systems have well-established error correction protocols, while quantum systems are still figuring out effective methods.
- Hybrid Models: Many researchers propose hybrid models that combine classical and quantum approaches. While promising, these models require intricate designs and the integration can be non-trivial.
Ethical Considerations
As we advance toward integrating AI with quantum computing, ethical concerns cannot be ignored. The potential power of quantum computing raises crucial questions about responsibility and decision-making capabilities of AI systems. The implications of quantum-enhanced AI go beyond technical efficiency; they touch societal norms and values.
For instance:
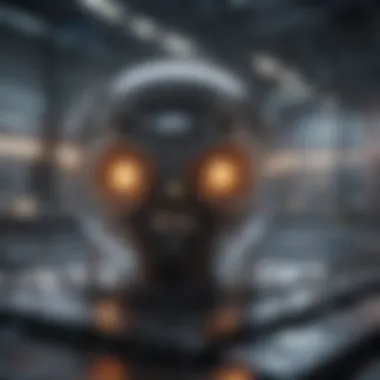
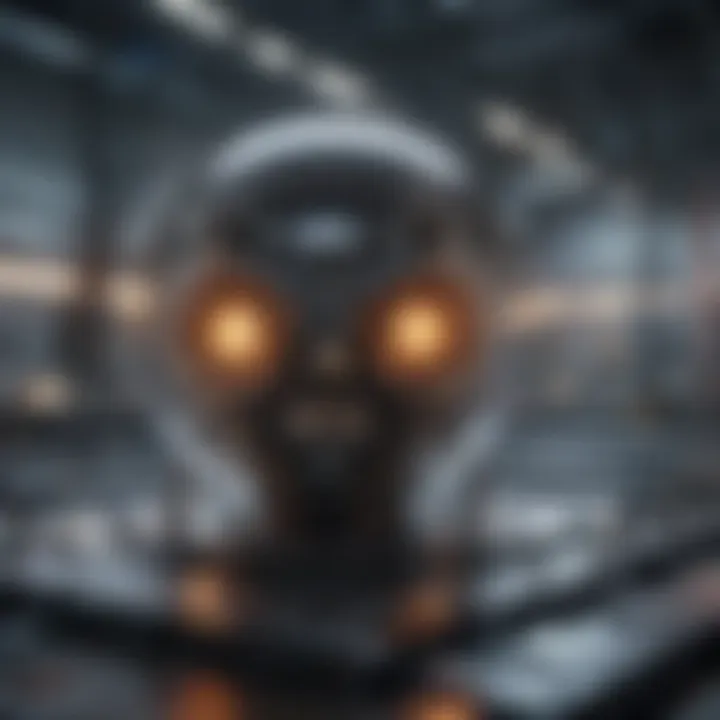
- Data Privacy: With quantum computing, there could be capabilities to break conventional encryption methods. Ensuring data privacy and security will require new frameworks and regulations.
- Bias in AI Algorithms: AI algorithms are only as good as the data they are trained on. Quantum computing may amplify existing biases in AI models if not designed responsibly. Ensuring that quantum AI systems operate fairly and equitably is a pressing concern.
- Job Displacement: Just as AI has raised fears about job loss, the integration of quantum computing could accelerate this trend. Preparing social structures to address potential changes in job markets will be crucial.
Effective frameworks addressing both technical and ethical considerations will be essential for the successful integration of AI and quantum computing.
In summary, the challenges of integrating AI with quantum computing are multifaceted. They require a balanced approach that combines technical innovation with ethical foresight. Engaging with these challenges is not merely a technical exercise but a necessary step towards unlocking the full potential of this powerful synergy.
Future Prospects in Quantum AI
The future prospects of quantum computing in the field of artificial intelligence (AI) hold significant potential. Understanding this intersection is vital, as it paints a picture of how advancements in one can significantly enhance the other. As quantum computing matures, it is set to unlock capabilities that traditional AI methods are unable to realize. The integration could lead to unprecedented efficiencies, allowing us to tackle problems considered intractable with classical computing.
Quantum AI is not merely an academic endeavor; it has practical implications that could reshape industries and areas of research. Consider the advantages of processing large datasets more swiftly. Traditional AI models can become cumbersome when analyzing big data or complex problems. On the other hand, quantum algorithms could provide speedups, making it feasible to derive insights from colossal datasets swiftly. This capability has far-reaching consequences, influencing sectors such as healthcare, finance, and information technology.
Potential Breakthroughs
Research into quantum AI is paving the way for numerous breakthroughs. One area of focus is quantum machine learning, which integrates quantum computing principles with machine learning frameworks. Techniques like quantum support vector machines and quantum-enhanced reinforcement learning are being explored. These methods hold potential to improve existing machine learning models by performing complex calculations that classical systems struggle with.
The algorithmic advancements that could come from this are profound. For instance, imagine training neural networks that can learn from data exponentially faster. This speed could usher in a new age of predictive analytics, where AI systems better anticipate trends, behaviors, and needs.
Moreover, initiatives such as quantum optimization algorithms show promise in logistics and supply chain management. These algorithms could drastically reduce computation times and provide optimal solutions faster than classical counterparts.
"As quantum technology continues to develop, the synergy between AI and quantum computing could revolutionize multiple sectors fundamentally."
Impact on Society and Economy
The integration of quantum computing into AI will undoubtedly bring about significant societal and economic impacts. First and foremost, it could enhance decision-making processes in sectors like healthcare, finance, and even public policy. For example, personalized medicine could benefit greatly from quantum-enhanced data analysis. This could lead to tailored treatment plans based on unique patient data much quicker than current methodologies allow.
The economic implications are equally profound. Embracing these technologies might drive efficiency and productivity across industries. This efficiency could lead to greater innovation, possibly creating new markets and jobs in the quantum technology sector, while also leading to cost reductions in various processes.
However, there are challenges to consider. The rapid development of quantum systems might render existing skills obsolete. Thus, it is essential to focus on education and training programs to prepare the workforce for future demands. Moreover, the ethical considerations regarding data privacy and access to these advanced technologies must not be overlooked. The potential for misuse or inequality in access could perpetuate social divides.
In summary, the future of quantum AI is bright but calls for thorough planning and responsible innovation. As we explore these frontiers, a balanced approach that considers both benefits and ethical implications will be critical.
Global Initiatives and Collaborations
The intersection of quantum computing and artificial intelligence is rapidly evolving, driven significantly by global initiatives and collaborations. These ventures are crucial in leveraging resources, knowledge, and expertise across borders. They foster innovation, enabling the integration of quantum technologies into AI frameworks that were previously unimaginable. Collaborative efforts not only amplify the pace of research but also ensure that the advancements are accessible to a broader audience.
International Projects and Studies
Across the globe, numerous international projects strive to advance the integration of quantum computing with artificial intelligence. One prominent initiative is the Quantum Computer Science and Artificial Intelligence Research Initiative, which connects researchers from various institutions. This collaboration focuses on developing new quantum algorithms specifically designed to improve machine learning tasks. The exchange of ideas among researchers from different countries allows for a more robust exploration of theoretical and practical applications.
Another notable project is the Quantum AI Network, a consortium of universities and research organizations. This network aims to conduct interdisciplinary studies that evaluate how quantum systems can improve the efficiency of AI models. By pooling resources, participants can run large-scale experiments that would be impossible for a single entity. The results from these studies contribute to academic literature and the development of cutting-edge technologies.
Corporate Partnerships
In addition to academic collaborations, corporate partnerships play a vital role in advancing quantum AI. Tech giants like IBM and Google are dedicating significant resources to quantum research, recognizing its potential in revolutionizing artificial intelligence. IBMβs Quantum Experience platform is a prime example of how corporate investment drives accessibility to quantum computing resources. This platform allows researchers and developers to experiment with quantum algorithms, enhancing their understanding of how these systems can interact with AI.
Similarly, Googleβs efforts in quantum supremacy have paved the way for AI applications that utilize quantum processing power. By establishing partnerships with educational institutions, these companies ensure the continuous flow of knowledge and skills between industry and academia.
Within this context, corporate collaborations also extend to startups focused on quantum AI. These small yet nimble companies often bring innovative ideas to the table. The synergy between established corporations and startups can lead to new solutions and applications in various fields, including healthcare, finance, and logistics.
"The future of AI fundamentally relies on breakthroughs in quantum computing, and this is only possible through global collaboration."
The collaboration efforts mentioned are vital not only for technological advancement but also for setting ethical standards and practices. Joint initiatives help define guidelines that govern the responsible use of quantum AI, ensuring that its development aligns with societal needs and concerns.
In summary, global initiatives and collaborations are essential to the progression of quantum computing and its integration with artificial intelligence. Through international projects, corporate partnerships, and interdisciplinary research, the potential of this synergistic relationship can be fully realized, bringing us closer to groundbreaking applications that could redefine various industries.
End
In closing, the intersection of quantum computing and artificial intelligence represents a pivotal frontier in technology. It is essential to recognize how these domains can complement and enhance each other. The unique capabilities of quantum computing, particularly in speed and parallelism, can significantly bolster AI algorithms, leading to more advanced machine learning models.
Summary of Insights
Throughout this article, we have explored several key insights regarding quantum computing and AI. The foundational principles of quantum mechanics set the stage for breakthroughs in data processing, enabling AI to handle larger datasets more efficiently. Enhanced algorithms derived from quantum computations not only improve accuracy but can also reduce training times considerably for deep learning models. The application landscape is vast, ranging from healthcare innovations that optimize diagnostics to financial models that better assess risks.
"Quantum AI could potentially redefine what's possible in computing and data analysis, creating opportunities that we have yet to fully imagine."
Moreover, the challenges of integrating these technologies cannot be overlooked. Issues like technical limitations and ethical considerations pose significant hurdles that must be addressed. Collaborative efforts across institutions and industries play a crucial role in navigating these complexities.
Call for Further Research
Given the rapid evolution of both fields, further research is necessary to uncover the full potential of combining quantum computing with AI. There is a critical need for more studies focusing on scalable quantum algorithms tailored specifically for use in AI. In addition, the implications of these innovations on society and the economy should be continuously analyzed. Addressing ethical concerns surrounding AI development within the quantum realm must also become a priority.
Ultimately, the journey toward a harmonious integration of quantum computing and AI is just beginning. As we look ahead, sustained investment in research and development will be vital to realize the profound possibilities that lie in their merger.