Exploring Neural Network Biology and AI Intersections
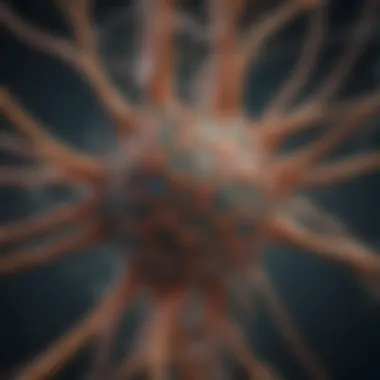
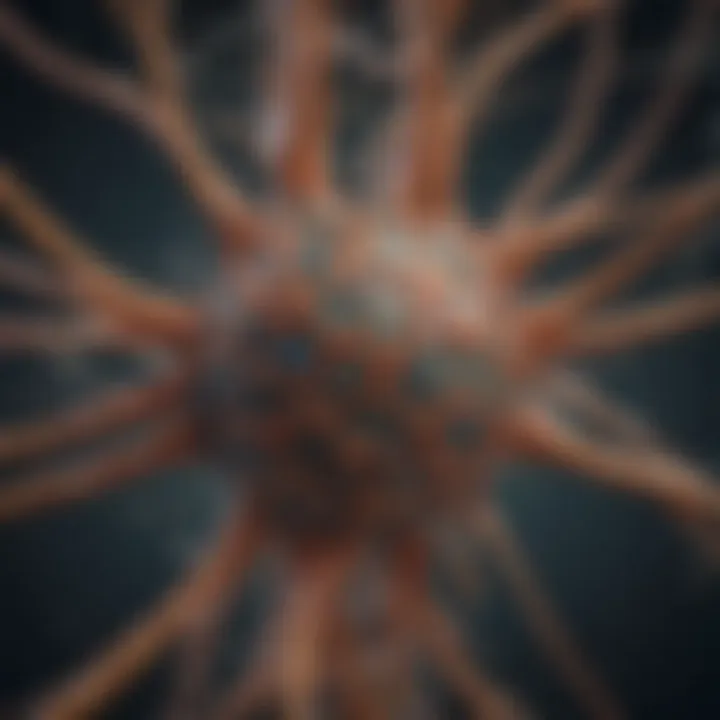
Intro
The interface between biological systems and artificial intelligence has garnered significant attention in recent years. This developing field, often referred to as neural network biology, delves into how biological neural networks can inspire the design of artificial neural networks. Understanding this intersection is crucial as it can lead to advancements in both biology and artificial intelligence. By outlining foundational concepts and exploring current research, this article aims to equip readers with essential insights.
Connecting artificial neural networks to biological processes provides a framework for understanding cognition and behavior. Researchers discover that biological structures inform technological designs. This provides a rich area for exploration across disciplines. The findings in this field not only enhance artificial intelligence but also deepen knowledge of neurological functions.
In exploring this topic, several key sections will provide a comprehensive perspective. From primary research findings to simplifications of complex theories, readers will encounter a variety of information. The narrative will be bolstered with visual aids and breakdowns as necessary, reflecting the dual importance of both biological systems and artificial intelligence.
Foreword to Neural Network Biology
Understanding the principles of neural network biology is crucial in the realm of both biological and artificial systems. This intersection offers insights that can enhance the design of artificial intelligence while also elucidating the complexities of biological neural networks. Neural network biology not only combines disciplines but also invites a deeper exploration of how intelligence arises and functions in different systems.
A dual focus on biological systems and artificial implementations allows for a more nuanced perspective. Researchers can gain valuable lessons from biological processes, which inform the approaches to constructing artificial neural networks. This relationship prompts an examination of the benefits that emerge from studying one field in light of the other.
This article aims to assess key elements, such as the similarities in structures between biological and artificial neurons, and how this knowledge can advance current technology. Additionally, understanding biological neural connectivity can lead to more sophisticated neural networks that mimic the brain's efficiency. Such explorations can bridge gaps between neuroscience and computer science, yielding innovations that impact diverse areas including healthcare and robotics.
In the pages that follow, topics will span foundational neural network concepts, the functionality of biological systems, and the implications for intelligence research. By exploring these connections, we can appreciate the dynamic relationship between biology and artificial intelligence.
Defining Neural Networks
Neural networks are computational models inspired by the biological neural networks present in the human brain and other organisms. They consist of interconnected layers of nodes or "neurons," which are designed to process information in complex ways. Each connection between the neurons has a weight associated with it, which adjusts as learning occurs. This adjustment enables the network to recognize patterns and make decisions based on input data.
In artificial neural networks, the basic structure involves an input layer that receives data, one or more hidden layers that perform computations, and an output layer that delivers results. The process of training these networks typically involves backpropagation, where the network learns from its errors by adjusting the weights based on the difference between predicted and actual outcomes.
Significance in Modern Science
The significance of neural network biology cannot be understated. As our reliance on artificial intelligence grows, understanding the intricacies of both biological and artificial networks becomes increasingly vital.
- Interdisciplinary Research:
- Healthcare Advances:
- Robotics Development:
- Facilitates collaboration between biologists and computer scientists, leading to shared breakthroughs.
- Techniques from neural networks are applied in medical diagnostics and imaging analysis, improving patient outcomes.
- Insights into how biological organisms move and respond to stimuli inform robotic designs.
This synthesis of knowledge promotes not only scientific progress but also the ethical considerations surrounding the application of artificial intelligence in society. As we continue to innovate, understanding the intersection of biological and artificial neural networks will be key to addressing the challenges of the future.
Biological Neural Networks
Biological neural networks represent a foundational element in understanding both the complexity of the human brain and the structures that inspire artificial intelligence design. This article emphasizes the significance of these networks, particularly their architecture and operational mechanisms, which serve as a blueprint for the development of artificial neural systems. By studying biological neural networks, researchers can glean insights into optimizing artificial intelligence in ways that mimic biological processes. This comparative approach allows a deeper comprehension of both biological intelligence and artificial constructs, fostering advancements that respect and utilize the intricacies of brain function.
Structure of Biological Neurons
Understanding the structure of biological neurons is vital because it outlines the fundamental building blocks of neural networks. Each component plays a unique role in facilitating communication and processing information. A clearer grasp of these structures aids in connecting their biological roots to artificial intelligence applications.
Cell Body
The cell body, or soma, acts as the central hub of a neuron. It houses the nucleus and is responsible for maintaining the cell's health. The key characteristic of the cell body is its metabolic functions, supporting the neuronโs overall viability. Its role is crucial in sustaining the networks, making it a central focus in this discussion. The cell bodyโs unique features, such as the presence of organelles required for protein synthesis, give neurons the capacity to manage biochemical processes essential for signaling. However, while robust, its vulnerability to damage makes it a consideration when exploring neural health in both biological and artificial contexts.
Dendrites
Dendrites are branched extensions that receive signals from other neurons. Their enlarged surface area is significant as it allows for a greater number of synaptic connections. This property enables neurons to integrate information from multiple sources, thus highlighting their importance in neural communication. The unique feature of dendrites is their ability to change shape and grow in response to activity. This plasticity supports learning processes, contributing to the adaptability of neural networks. However, excessive dendritic growth can lead to compartmentalization of activity, which may hinder communication.
Axon
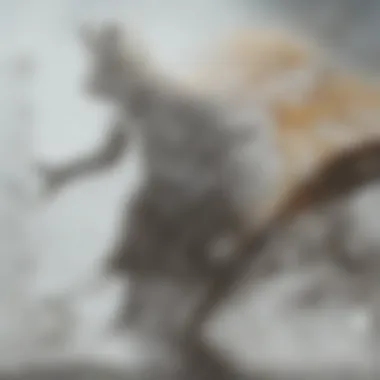
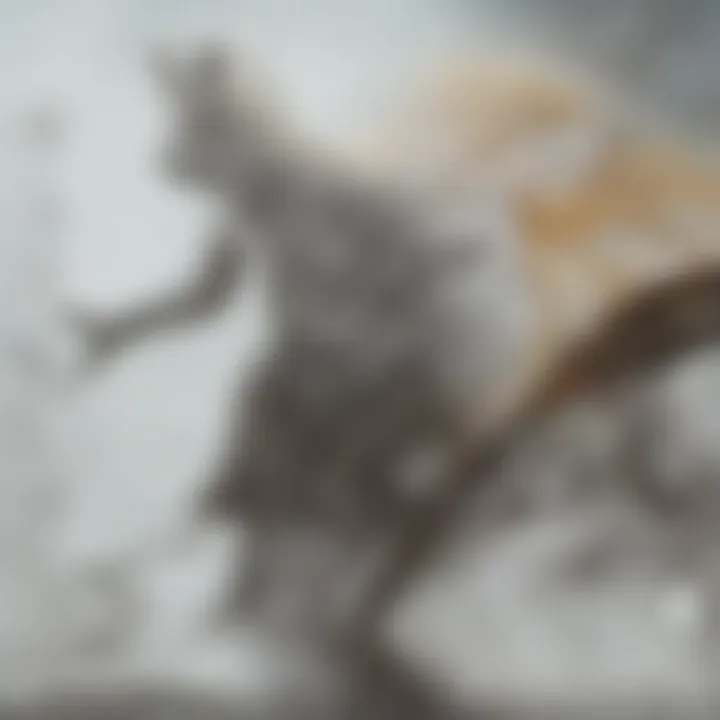
The axon serves as the transmission line of the neuron, facilitating the relay of signals away from the cell body toward other neurons or muscles. Its defining characteristic is the presence of myelin sheaths that enhance signal conduction speed. This makes the axon a crucial element in the quick and efficient transfer of information. The myelination also allows for saltatory conduction, where action potentials jump between nodes of Ranvier. The downside is that if an axon is damaged, it can disrupt communication within the entire network, illustrating the fragility of these connections.
Synapses
Synapses are the junctions where neurons communicate with each other. They play a pivotal role in determining the strength and efficacy of neural signals. The distinguishing feature of synapses is their ability to modulate neurotransmitter release, which can strengthen or weaken signals based on various factors, including signaling activity. This variability offers advantages for learning and memory processes. However, the complexity of synaptic interactions can lead to unpredictable outcomes, making it a challenging area of study in both neuroscience and artificial intelligence.
Neural Network Functionality
The functionality of biological neural networks showcases how information is processed and transmitted within the brain. Understanding these functions is key to developing artificial systems that can replicate some aspects of human intelligence. Every functional aspect interacts synergistically and ultimately determines how biological networks adapt and learn.
Signal Transmission
Signal transmission refers to the process of transferring information through electrical impulses and chemical signals. The efficiency of this process is a mainstay of neural functionality, making it a critical area of interest. A distinctive characteristic of signal transmission in neurons is its reliance on action potentials, highlighting a highly organized sequence of events underpinning neural communication. The robust nature of this mechanism allows rapid responses; however, disruptions can lead to significant impairments in neural function.
Neurotransmitter Action
Neurotransmitter action involves the release and binding of chemical messengers that facilitate communication between neurons at synapses. This aspect is essential for the modulation of signal transmission and greatly affects overall neural activity and behavior. The key characteristic of neurotransmitters is their selective action on specific receptors, allowing for targeted communication pathways. This specificity offers the advantage of finely tuned responses but can also limit the influence of poorly functioning neurotransmitters, illustrating the delicate balance in neural interactions.
Plasticity
Plasticity refers to the brain's ability to change and adapt in response to experience. It is a crucial mechanism underlying learning and memory. The key feature of plasticity is the ability of synapses to strengthen or weaken over time, influencing how information is processed. This adaptability creates advantages for overall cognitive functions, enabling efficient learning processes. However, excessive plasticity can lead to chaotic signaling patterns that may cause dysfunction in neural networks.
Artificial Neural Networks Overview
Artificial neural networks (ANNs) serve as the backbone of many artificial intelligence applications today. Their significance lies in their ability to model complex relationships and make predictions based on data. By mimicking the human brain, ANNs offer various benefits that enhance machine learning and data analysis. From pattern recognition to decision-making processes, the structure and dynamics of neural networks reflect biological systems, which makes them relevant in this discourse.
Basic Architecture
Input Layer
The input layer is crucial for feeding data into the network. It serves as the entry point where raw information is processed. Each node in this layer corresponds to a feature in the dataset. One key characteristic of the input layer is its simplicity; it merely receives data without performing any transformations on it. This aspect makes it a beneficial choice, as it allows for straightforward integration of different kinds of input data. The unique feature of the input layer is its capacity to handle various formats, whether images, numerical data, or text. However, its disadvantage lies in the fact that it does not perform any computations, which can lead to a saturation of raw input data.
Hidden Layers
Hidden layers are where the real calculations happen. They consist of multiple neurons that apply different mathematical functions to the inputs received from the previous layer. This characteristic allows hidden layers to capture complex patterns in the data. Their versatility makes hidden layers a popular choice for building more sophisticated models, as they enable the network to learn intricate representations. A unique feature is the number of hidden layers can vary based on the complexity of the task, benefiting more complicated problems. However, an increase in hidden layers can lead to longer training times and a risk of overfitting.
Output Layer
The output layer produces the final prediction of the neural network. Each neuron in this layer provides a confidence score for the respective class or outcome that the model is designed to predict. A major strength of the output layer is its ability to represent the final decisions, summarizing the information processed by previous layers. It typically employs activation functions like Softmax for classification tasks, which is advantageous for interpreting probabilities. One drawback is that if the architecture isn't well-structured, the predictions can become less reliable, especially if the training data lacks diversity.
Types of Neural Networks
Feedforward Networks
Feedforward networks are the simplest type of neural network architecture. In this arrangement, information moves only in one direction: from the input layer through the hidden layers to the output layer. The key characteristic of feedforward networks is their straightforward connectivity, making them easy to implement. They are beneficial for tasks requiring clear input-output relationships, such as regression analyses. A unique feature is their inability to utilize temporal data; thus, they are not optimal for sequence tasks, like time series data or natural language processing.
Convolutional Networks
Convolutional networks (CNNs) are specialized for processing grid-like data, such as images. Their architecture includes convolutional layers that automatically detect features such as edges or textures. This ability is a key characteristic that allows CNNs to excel in image analysis and recognition tasks. They are especially beneficial for applications in computer vision due to their efficiency in handling large datasets. A unique feature of CNNs is parameter sharing, which reduces the number of parameters in the model. However, a potential disadvantage is they require substantial data for optimal performance, making them less effective with limited datasets.
Recurrent Networks
Recurrent networks (RNNs) are designed to handle sequential data, making them suitable for tasks like language modeling and time series analysis. The key characteristic is their ability to maintain a memory of previous inputs through feedback loops. This feature enables RNNs to understand context in sequences, which is crucial for many applications involving natural language and acoustics. They are beneficial as they allow for variable-length input sequences. A downside is they can suffer from issues like vanishing gradients, complicating the training process over long sequences.
Artificial neural networks drive innovations across various sectors, making them essential components in the advancement of artificial intelligence.
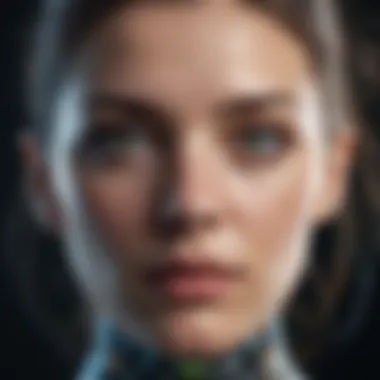
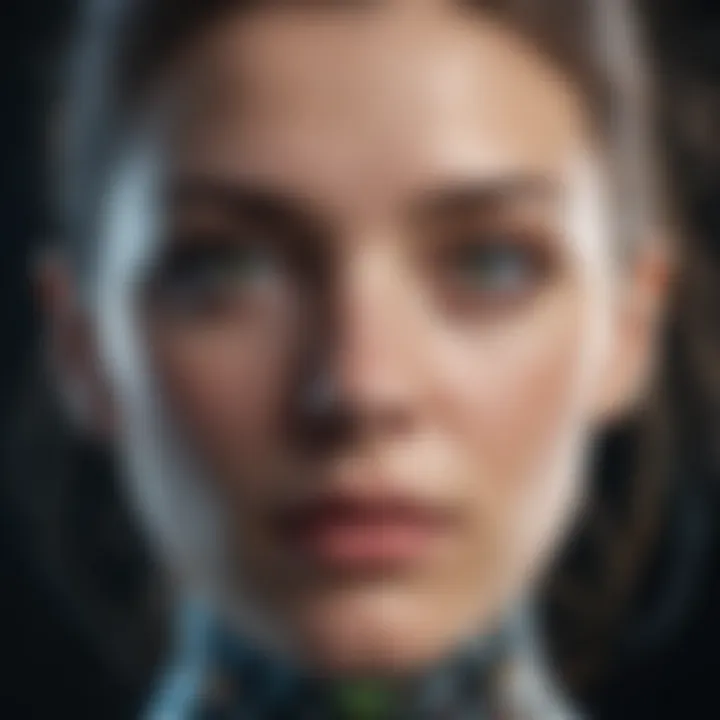
This overview serves to illustrate the importance of understanding artificial neural networks within the broader context of neural network biology. By exploring their architecture and types, we can lay the groundwork for deeper inquiries into their applications and implications in both scientific and practical realms.
The Interdisciplinary Nature of Neural Network Biology
The intersection of biology and artificial intelligence is a crucial area of study, known as neural network biology. This discipline underscores how insights from biological systems can vastly enhance the development and functionality of artificial neural networks. By exploring this interdisciplinary nature, researchers and practitioners gain a broader understanding of both fields, leading to innovative breakthroughs. The scrutiny of interactions between biological neurons and artificial models reveals important principles that guide how we approach problems in AI, particularly in learning and adaptability.
Collaboration Between Fields
Neuroscience and Computer Science
Neuroscience and computer science share a symbiotic relationship essential for advancing neural network biology. Neuroscience provides insights into the mechanisms of biological neurons, helping us to understand how information is processed in the brain. This knowledge is invaluable when creating artificial neural networks that must mimic such processes.
The collaboration allows for a bi-directional flow of information. Computer scientists use biologically inspired algorithms that reflect neural functions, while neuroscientists can test hypotheses using computational simulations. This partnership brings to light new methodologies, enhancing both fields' approaches to complex problems. However, challenges exist, including differing terminologies and conceptual frameworks that can complicate communication between the two disciplines.
Mathematics Applications
Mathematics plays a foundational role in neural network biology, as it offers tools and frameworks necessary for modeling complex biological systems and artificial networks. Mathematical principles underpin algorithms used in machine learning, such as optimization techniques and statistical methods.
One key characteristic of mathematics applications in this field is its ability to quantify and structure data, making it easier to draw valid conclusions from neural interactions. This quantification allows for precise adjustments and developments in both biological understandings and artificial networks.
Yet, the use of mathematics in neural networks can also present drawbacks. It often requires a significant background in advanced mathematics, which may limit accessibility for some researchers. Despite this, the benefits of mathematical applications in guiding research and developing models cannot be overstated.
Implications for Understanding Intelligence
The interdisciplinary exploration of neural network biology has profound implications for our understanding of intelligence. By acknowledging how artificial systems can be designed based on biological principles, researchers can generate more effective models that replicate intelligent behavior. This connection enhances the understanding of cognitive processes, leading to advancements in both AI and neuroscience.
The ongoing dialogue between these disciplines fosters an enriched perspective on what constitutes intelligence, pushing the boundaries of how we define and implement cognitive and artificial systems. As the debate unfolds about biological versus artificial intelligence, the insights gained will likely influence fields ranging from psychology to robotics, enabling a better grasp of how intelligent systems operate and evolve.
Learning Mechanisms in Neurons and Networks
Learning mechanisms are essential to understanding how both biological and artificial neural networks evolve and adapt over time. In biological systems, the process is often driven by experience and interaction with the environment. For artificial systems, learning involves algorithms designed to optimize performance based on data inputs. The link between these two realms offers valuable insights into intelligence and behavior in various contexts.
Biological Learning Processes
Hebbian Learning
Hebbian learning is a foundational concept in neurobiology. It emphasizes that neurons that fire together strengthen their connection. This principle suggests that the synaptic strength between two neurons increases when they activate simultaneously. This characteristic makes Hebbian learning highly relevant to understanding memory formation and associative learning in biological systems. Its contribution is significant because it models how experiences shape neural connections.
The key feature of Hebbian learning is its simplicity and direct connection to natural processes. It does not require external reinforcement. Thus, it can be seen as beneficial for explaining self-organizing learning in neural structures. However, this simplicity can be a double-edged sword. While promoting adaptability, it may also lead to instability if left unchecked, resulting in chaotic neural interactions.
Reinforcement Learning
Reinforcement learning is another pivotal concept. This approach involves learning by interacting with an environment and receiving feedback in the form of rewards or penalties. It is particularly well-suited for tasks where outcomes are uncertain and actions need to be optimized over time. The focus here is on the consequences of actions to drive behavior modification.
A key characteristic of reinforcement learning is its ability to handle complex problem spaces. It is advantageous in applications ranging from robotics to game design, where optimal strategies must be discovered through exploration and exploitation. However, this technique has unique challenges, including the need for substantial data to train effectively and the potential for slow convergence to a stable solution.
Machine Learning Techniques
Machine learning techniques parallel biological learning but operate through different frameworks. They focus on algorithms that improve their predictive performance based on observed data, akin to how biological systems learn from experiences.
Supervised Learning
Supervised learning involves training with labeled data, where the outcome is known. The model learns to map inputs to outputs based on this comprehensive information. It plays a crucial role in tasks such as classification and regression. Its advantage lies in its highly structured approach, providing clarity in model training and evaluation.
The key feature of supervised learning is its reliance on pre-existing data sets. This makes it a popular choice for applications needing accuracy and precision. However, a significant limitation is the need for labeled data, which can be time-consuming and expensive to obtain.
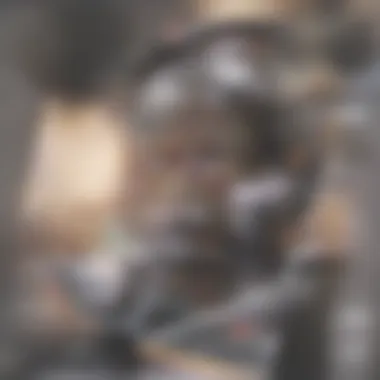
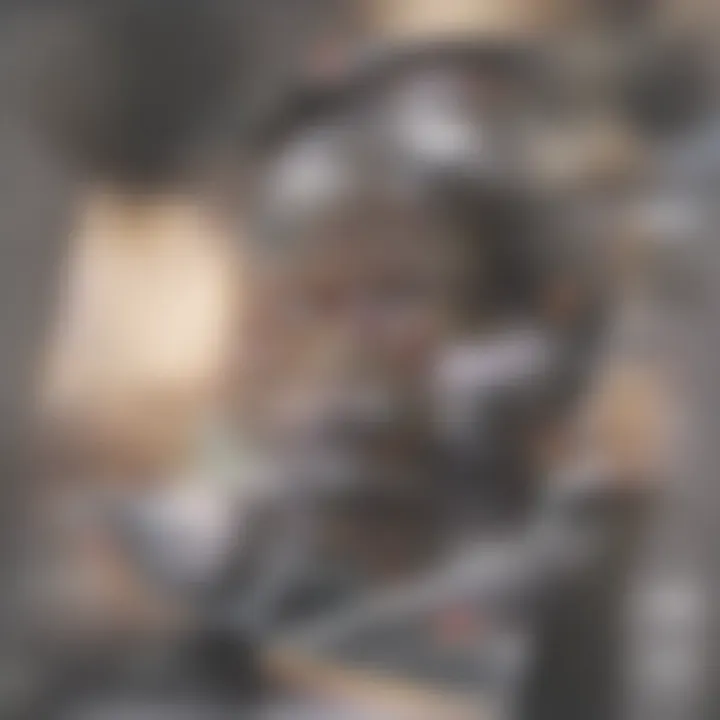
Unsupervised Learning
Unsupervised learning contrasts with its supervised counterpart by working with unlabelled data. Its aim is to identify patterns or structures within the data without prior knowledge of outcomes. This technique helps uncover hidden relationships and groupings, making it useful in exploratory data analysis.
The unique feature of unsupervised learning is its flexibility. It can adapt to various data types and is beneficial for clustering tasks and dimensionality reduction. Nevertheless, the main challenge is the interpretation of outputs, as the absence of labels can lead to subjective conclusions regarding the revealed patterns.
"Understanding the mechanisms of learning in both biological and artificial systems provides insights into the broader implications for cognitive science and artificial intelligence."
Applications of Neural Network Biology
Neural network biology represents a pivotal intersection between biology and artificial intelligence. The applications of this field are vast and transformative, impacting various sectors. Understanding these applications unveils how biological insights can lead to advancements in artificial intelligence and provide practical solutions to complex problems.
One primary area of application is in healthcare innovations. Leveraging neural network principles enhances diagnostics and treatment plans. Machine learning algorithms process patient data, identify patterns, and predict outcomes, which can lead to personalized medicine. Moreover, neural networks are increasingly utilized in medical imaging, improving detection rates of conditions such as cancer through enhanced image analysis. This integration results in timely interventions and better patient care.
Another significant domain is robotics. By applying neural network concepts, robots gain the ability to learn and adapt to various environments. This adaptability allows robotic systems to perform tasks with greater efficiency. Robotics in manufacturing, for example, can utilize artificial neural networks to optimize workflows and reduce errors. As robots evolve to emulate human-like intelligence, their utility in complex operations expands further.
"Neural networks provide the framework needed for machines to learn in ways that mimic human cognitive processes, pushing the boundaries of both artificial intelligence and robotics."
In summary, the continuing exploration of neural network biology holds the promise of major innovations. The integration of biological principles informs artificial systems, enhancing their functionality and effectiveness across diverse applications. This ongoing confluence will likely shape future technological advancements in unprecedented ways.
Ethical Considerations
The intersection of biology and artificial intelligence opens many discussion points. One of the most crucial topics is the ethical considerations surrounding both biological and artificial neural networks. The landscape of AI is rapidly expanding, and as it does, the ethical implications become more pronounced. Understanding these ethical issues enhances our awareness of the responsibilities that come with technological advancements.
Biological vs. Artificial Intelligence Ethics
The ethical frameworks governing biological intelligence and artificial intelligence often overlap but also differ significantly.
- Human Agency and Consent: In biological systems, ethical considerations often focus on human rights and the role of consent in research experiments. For artificial intelligence, the debate centers on how these systems mimic decision-making processes that impact human lives. As AI systems become more integrated in everyday decisions, the stakes increase.
- Accountability: When biological systems, such as human doctors, make mistakes, accountability can be traced back to individuals. In contrast, artificial intelligence systems, like those used in healthcare, complicate accountability. If an AI model fails or causes harm, who is responsible?
- Bias and Discrimination: Biological intelligence can also demonstrate bias, but it is tied closely to human experience. In AI, bias is derived from training data and can be perpetuated across systems. The seriousness of biased AI decisions drives a pressing need for fair data practices.
"As artificial intelligence systems evolve, the ethical implications of their deployment in human contexts cannot be overlooked."
- Impact on Society: The development of AI can lead to significant social changes, particularly in sectors like employment and healthcare. Ethical considerations must include the potential negative impacts these changes may have on communities.
Future Ethical Dilemmas
Looking ahead, several ethical dilemmas may arise as neural networks continue to evolve:
- Autonomy vs. Control: One dilemma is the balance between the autonomy of artificial intelligence and the need for human oversight. As AI grows more complex, deciding how much control humans should retain becomes critical.
- Privacy: With advances in AI, especially in data analytics, privacy concerns are more prevalent. Securing personal data while ensuring the effectiveness of AI systems must be approached delicately.
- Job Displacement: The integration of AI in various sectors raises concerns about job displacement. As machines assume more roles traditionally held by humans, the ethical implications for workers and their communities demand attention.
- Long-term Consequences: The long-term impact of AI decision-making on human life remains unclear. Questions about sustainability and the moral ramifications of AI technology need thorough examination.
In summary, the ethical considerations in neural network biology are complex and multifaceted. By acknowledging these implications, we can navigate the challenges posed by this intersection more effectively.
Future Directions in Neural Network Biology
The convergence of biological systems and artificial intelligence continues to unfold in intriguing ways. Understanding future directions in neural network biology holds significance for various fields. As researchers explore new methodologies, they may discover optimal patterns that mirror the efficiency found in biological neural processing. Emphasizing this intersection can lead to enhanced algorithms and intelligent systems that perform better than current alternatives.
Emerging Research Areas
In recent years, several emerging research areas have gained attention in neural network biology. These areas highlight how we can leverage principles from biology to evolve artificial systems further. Some of these areas include:
- Neuromorphic Computing: This research focuses on designing computer hardware modeled after the neural structure, aiming to optimize processing speed while reducing energy consumption. Neuromorphic chips are setting the stage for mimicking biological processes more accurately.
- Bio-inspired Algorithms: Scientists are developing algorithms that reflect biological concepts such as evolution and natural selection, leading to innovative solutions for complex problems.
- Cross-disciplinary Approaches: Collaborative studies involving neuroscientists, computer scientists, and biologists are pivotal. This enables a holistic perspective, facilitating breakthroughs that would not be achievable within isolated disciplines.
By pursuing these areas, researchers can enrich our understanding of neural network biology and expedite the development of smarter AI systems.
Potential Breakthroughs
The exploration of neural network biology brings potential breakthroughs that could reshape industries. Some key areas to watch include:
- Enhanced Learning Models: Innovations in learning models draw inspiration from synaptic plasticity in biological systems. Greater insights into how neurons adapt might improve machine learning models significantly.
- Personalized Medicine: By applying neural network principles to healthcare, we may see advancements in personalized treatment plans. AI can analyze vast datasets to recommend tailored therapies based on individual responses.
- Advanced Robotics: Both fields are converging to create more adaptable robotic systems. Machines that mimic certain biological functions could lead to more efficient operations in various environments, from manufacturing to healthcare.
"Understanding the potential of integrating biology with artificial intelligence can lead to the next wave of technological innovation."
The future of neural network biology will undoubtedly yield numerous opportunities, affecting technology, healthcare, and our understanding of intelligence itself.