Judea Pearl's Impact on Causal Inference Explained
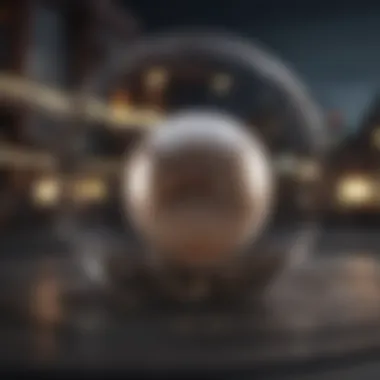
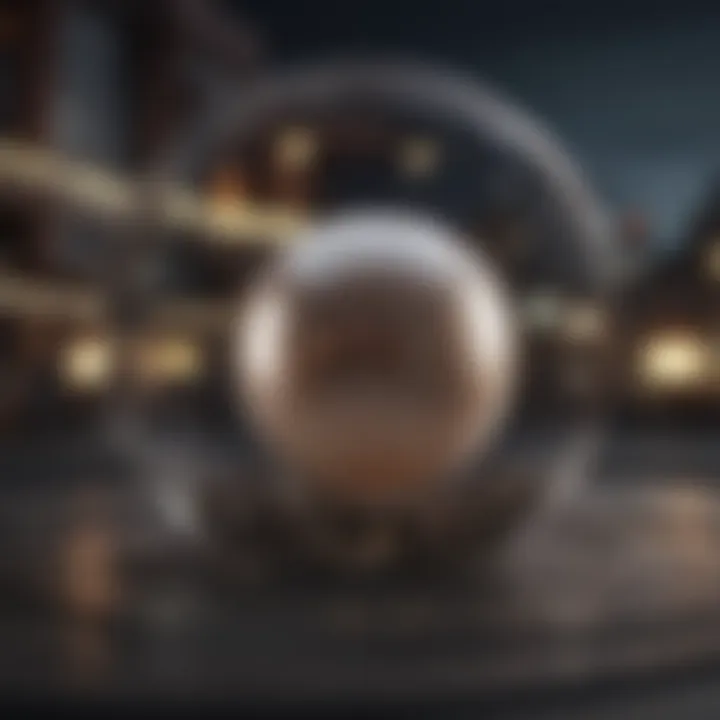
Intro
Causality is like the electric current that powers the engine of scientific inquiry. Got it? Well, Judea Pearl is one of the foremost figures who has lit up that path of understanding. His insights into causal inference have laid bare how relationships between variables can be unraveled, transforming our grasp of everything from medicine to social sciences.
In this journey through Pearl’s contributions, we'll delve into his foundational theories, see how his ideas have been implemented across various fields, and discuss their implications for contemporary research methodologies. This isn’t just about the theory, but also about the real-world applications that prove his work is not confined to dusty textbooks, but rather pulsates with life in experiments and analyses all around us.
Key Research Findings
Overview of Recent Discoveries
Judea Pearl's work has led to several pivotal breakthroughs, particularly in the realm of causal graphical models. His invention of the Bayesian network has allowed researchers to decipher complex systems in fields like genetics, economics, and artificial intelligence, making it much easier to understand the chain of cause and effect. His ability to blend statistical theory with semantics is a remarkable feat that reshaped how causation is perceived in modern research.
Moreover, Pearl's development of the do-calculus provides a framework for reasoning about interventions. By answering the question "What if we do X?", researchers can robustly predict outcomes without falling into the trap of mere correlation, which can mislead if naively interpreted.
Significance of Findings in the Field
The significance of Pearl’s findings cannot be overstated. He propelled a shift in thinking, replacing the older models that relied heavily on correlation with his causality-centric approach. This has influenced the way researchers craft experiments and interpret data, ensuring they not only identify relationships but also understand their depths and nuances.
One cannot overlook the ripple effects of Pearl’s contributions across disciplines. For instance, in medicine, his work has informed clinical trials, guiding how physicians determine the effectiveness of treatments. Similarly, in social science research, his frameworks enable more accurate interpretations of sociocultural dynamics.
"Causality gives us keys to unlock mysteries, where correlation leaves us in a fog," says Edward, a student of Pearl’s work.
Breakdown of Complex Concepts
Simplification of Advanced Theories
While Pearl’s theories may first appear as smoke and mirrors to those unfamiliar with the intricacies of statistics, they stand on a firm foundation of logic and clarity. Take the concept of the counterfactual: it’s about imagining an alternative scenario to understand causation better. When you ponder, "What if I didn’t take that job?" you engage with a basic form of Pearl’s theory. This simplification leads to deeper insights into how decisions impact outcomes, a theme relevant not just in personal reflections but in high-stakes academic research.
Visual Aids and Infographics
Utilizing visual aids can deepen the understanding of Pearl's theories. Diagrams illustrating Bayesian networks, for instance, provide clarity on how various variables interconnect. These infographics can serve as a bridge between complex academic ideas and practical applications, making it easier for both students and professionals to grasp the nature of causal relationships.
In sum, as we explore Judea Pearl’s impact on the science of causality, we uncover a treasure of knowledge that serves not just academics but anyone wishing to better understand the world around them. His legacy is firmly planted in the soil of empirical inquiry and real-world applications, pushing the boundaries of what we can know and understand.
Prolusion to Causality
Understanding causality is akin to peeling an onion; it brings layers of complexity into focus, one layer at a time. In the realm of scientific inquiry, establishing a causal relationship is paramount. It goes beyond mere correlation, unveiling the mechanisms that govern how events influence one another. This distinction is crucial as researchers and practitioners aim to draw accurate and actionable insights from their work. Causality explains not just that A is related to B, but that A indeed causes B, a concept that forms the backbone of scientific methodologies across diverse fields.
The significance of causality extends into various disciplines - from medical research establishing the effects of treatments, to social sciences analyzing the impact of policies on communities. In this article, we will delve into the foundational theories of causality, with a particular focus on the pioneering work by Judea Pearl. His contributions fundamentally altered how researchers approach causal inference, providing a coherent framework that enhances clarity and rigor in investigation.
Causality is a cornerstone for informed decision-making. When causal relationships are established, predictions become possible. This predictive power is not only critical in theoretical research but also in practical applications like public health, economics, and artificial intelligence. It allows policymakers to implement changes based on informed projections of outcomes, ultimately driving effective solutions to complex problems.
The following sections will discuss how Judea Pearl's theories and methodologies have influenced contemporary research practices, illuminating the path from theoretical understanding to real-world applications.
Defining Causality
Causality can be defined as the relationship between causes and effects. In simple terms, it's the 'why' behind actions or events. For instance, if we consider a situation where a person drinks water and subsequently quenches their thirst, we can propose that drinking water caused the thirst to subside. However, establishing this connection requires more than just observation; it calls for a deeper inquiry into underlying variables and the processes that link them.
Pearl emphasizes the need for a rigorous framework to analyze these relationships; this is where his structural causal model shines. This model allows researchers to represent causal relationships graphically, providing clarity to the complex interactions in multivariate systems. A well-defined causal model helps to navigate the intricacies of real-world phenomena by safeguarding against common misconceptions, such as confusing correlation with causation.
Importance of Causal Understanding
Grasping the fundamentals of causality is essential for myriad reasons:
- Informed Decision Making: Causal understanding equips decision-makers with the insights necessary to make evidence-based choices that can lead to desirable outcomes.
- Predictive Analytics: By grasping cause-and-effect relationships, one can forecast the consequences of various actions. This predictive capacity is invaluable in fields like economics and public health, where anticipated results can guide interventions.
- Enhanced Research Rigor: Causality enriches scientific inquiry, helping researchers formulate robust hypotheses and design experiments that more effectively test their ideas.
- Policy Formulation: In social sciences, understanding causal relationships can shape effective policies. This ensures that interventions are not based on assumptions, but on well-established causal connections.
Ultimately, understanding causality goes a long way in reducing uncertainty in a world filled with complexities. As we dive deeper into Judea Pearl's work, it becomes evident that these concepts are not just academic but have real implications that resonate across multiple domains.
Judea Pearl: A Biographical Sketch
This section brings to light the life and achievements of Judea Pearl, a pivotal figure in the realm of causal inference. Understanding Pearl's journey is key to appreciating his groundbreaking contributions to this field. His life experiences frame how his ideas have shaped contemporary theories and applications of causality. Pearl’s insights didn’t just emerge from thin air; they were cultivated through a confluence of education, personal determination, and a deep-rooted curiosity about how the world works. By examining his background and professional milestones, readers can gain clarity on the foundations of his work and the inspiration behind his theories.
Early Life and Education
Judea Pearl was born in 1936 in Tel Aviv, Israel. Growing up in a modest household, he often found himself wondering about the "why" behind various phenomena. This early penchant for questioning would set the stage for his later work in causality. In 1947, the Pearl family migrated to the United States, where young Judea had the opportunity to immerse himself in a different educational culture.
His academic pursuits took him to the City College of New York, where he initially studied electrical engineering. However, his fascination with the underlying principles of intelligent systems led him to switch fields. He completed a degree in mathematics, providing him the analytical skills that would serve him well in his later research. Pearl’s passion for knowledge did not stop after his degree; he pursued a master’s in electrical engineering from the University of California, Los Angeles. Here, he delved into the realm of artificial intelligence, setting him on his path toward the development of causal reasoning.
Career Milestones
Judea Pearl has had a remarkable career, replete with achievements that have significantly influenced the landscape of computer science and statistics. Starting as a professor at UCLA, he was able to channel his dual interests in artificial intelligence and statistics. In the 1980s, he proposed the Structural Causal Model, a framework that helped to formalize causal relationships in scientific inquiry.
One of his most noted contributions is the development of Bayesian networks, which enabled complex reasoning about cause-and-effect relationships through graphical models. This was a game-changer, allowing researchers to visualize and compute probabilistic relationships in a structured manner.
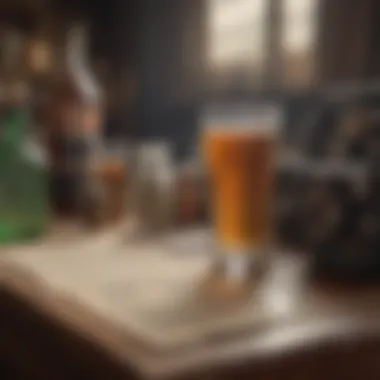
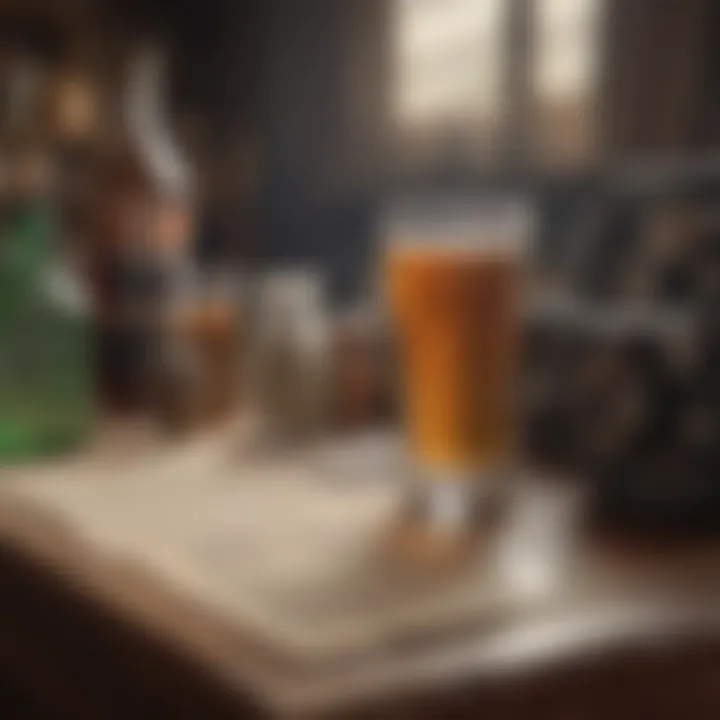
Pearl didn't stop there; he relentlessly worked on expanding the understanding of causal inference, leading to the publication of his notable books, including "Causality: Models, Reasoning, and Inference" in 2000. This work has been foundational in both philosophy and applied sciences.
In later years, his theories have been instrumental in various fields, from economics to public health. His commitment to clarity and rigor in understanding causation has initiated numerous debates and discussions among scholars, contributing to an evolving conversation in the scientific community. Pearl's accolades, like the Marconi Prize in 2011 and the Turing Award in 2011, underscore his international recognition and importance in his field.
In summary, Judea Pearl's biography illustrates not just an individual journey, but a transformative arc that profoundly shaped various disciplines through the exploration of causality. His legacy continues to resonate as more researchers adopt his insights into their work. Through this understanding, we can appreciate the layers of thought and dedication that characterize Pearl’s contributions to the science of causation.
Theoretical Foundations of Causality
The study of causality is a cornerstone of various academic fields, including statistics, philosophy, and artificial intelligence. Judea Pearl's contributions to these foundational theories have reshaped how researchers approach causal relationships. By developing a structured framework, Pearl not only clarified the interplay between cause and effect but also emphasized the significance of understanding the mechanisms behind observed data.
Understanding the theoretical foundations builds a bridge between abstract theories and practical applications. The benefits are profound, as causality enables breakthroughs in testing hypotheses, refining algorithms, and formulating policies that are more effective and data-driven. The discussion here revolves around two pivotal elements: causal graphs and counterfactual reasoning.
Causal Graphs and Diagrams
Causal graphs are a visual representation that elucidates the relationships between variables, serving as a valuable tool in both theoretical research and practical applications.
- Directed Acyclic Graphs (DAGs): Pearl introduced the concept of directed acyclic graphs, which elegantly express causal relationships without any cycles, meaning that you can't return to the same point. This simplicity belies their power, as they allow for a clear guide through complex systems.
- Conditional Independence: Causal graphs help in identifying which variables are conditionally independent, shedding light on potential confounding factors. This can significantly influence the design of studies and models in fields ranging from epidemiology to social sciences.
- Interventions and Predictions: By illustrating how interventions affect outcomes, causal graphs empower researchers to predict the outcomes of changes in a system. This property is particularly beneficial in areas like healthcare, where introducing a new treatment can dramatically alter patient outcomes.
The elegance and utility of causal graphs exemplify why they’re foundational to Pearl's work. Here's a depiction of how a simple causal graph might look:
"Causal diagrams are more than just pictures; they are the fundamental vehicle for understanding causal inference."
Counterfactual Reasoning
Counterfactual reasoning emerges as a pivotal element in Pearl's discourse on causality. At its essence, it considers "what could have been" under different circumstances. The significance of this topic cannot be overstated, as it also allows one to explore alternate realities arising from different actions or interventions.
- Treatment Effects: In public health and social sciences, counterfactual reasoning is instrumental in assessing the effect of treatments. For instance, researchers can estimate the impact of a drug by comparing observed outcomes against a hypothetical situation where the drug wasn't administered.
- Policy Analysis: In the realm of policymaking, counterfactuals inform decisions by allowing analysts to predict the outcomes of various policy interventions. This becomes crucial when determining the most effective measures to address societal issues.
- Historical Analysis: Social scientists often employ counterfactuals to analyze historical events. By hypothesizing alternative outcomes, they gain insights into the causal mechanisms that shaped events.
Through counterfactual reasoning, Pearl's framework pushes scholars to think beyond mere correlation and delves into causation and its implications. This leap in understanding paves the way for a more robust engagement with data, enhancing the validity of conclusions drawn from research.
Pearl's Structural Causal Model
Judea Pearl's structural causal model represents a monumental leap in the way we understand and analyze causality. This approach unpacks the complexities of how variables interrelate, providing a rigorous framework that elevates our thinking about causation beyond mere correlation. Pearl emphasizes that merely observing relationships without considering their underlying causal mechanisms can lead to misleading conclusions. This model underscores the distinction between correlation and causation, an essential differentiation that is often overlooked in everyday discourse.
The significance of Pearl's structural causal model lies in its ability to formalize causal inference. By decomposing systems into components and analyzing them through directed acyclic graphs (DAGs), researchers can visualize and understand the dependencies and influences among different variables. This clarity benefits a range of disciplines—spanning from economics to epidemiology—where understanding the causal structure impacts policy decisions, health interventions, and economic modeling.
Pearl's model provides the tools necessary to both describe and predict the effects of interventions, crucial for making informed decisions in uncertain environments.
Components of the Model
At the heart of Pearl's structural causal model are several foundational components that work in concert to represent causal relationships. Primarily, these components include:
- Variables: These are the building blocks of the model. Each variable represents a distinct entity that can influence other variables, including treatment, outcome, and confounding factors.
- Causal Graphs: Illustrated through directed acyclic graphs (DAGs), these graphs depict how different variables influence each other. Each arrow indicates a causal direction, visually simplifying complex interdependencies.
- Structural Equations: For each variable, these equations define how it is influenced by other variables. By establishing mathematical relationships, researchers can articulate the processes guiding the relationships shown in the graphs.
- Interventions: Pearl's framework distinguishes between observational and interventional analysis, allowing researchers to explore how changes in one variable affect another through controlled interventions, using techniques such as do-calculus.
Together, these elements enable comprehensive modeling of causal relationships, making it easier to infer the likely effects of potential actions in the real world.
Applications of Structural Models
The applications of Pearl's structural causal model can be seen across a broad spectrum of fields, highlighting its versatility and impact. Here are some notable examples:
- Public Health Research: Researchers can assess the impact of health interventions, such as vaccination programs. By modeling causal pathways, they can estimate potential outcomes under various scenarios, aiding in effective decision-making.
- Economics: Structural causal models are increasingly used in economic policy evaluations, helping economists understand how changes in economic conditions or policies might affect employment rates, inflation, and overall economic growth.
- Machine Learning: In AI, understanding causality helps refine algorithms. By incorporating causal models, machine learning can move beyond making predictions based solely on correlation, improving accuracy and reliability in predictions.
- Social Sciences: In sociology and psychology, these models aid in understanding how social policies impact community behavior, helping to formulate more effective measures that account for social dynamics.
The breadth of these applications not only emphasizes the importance of Pearl’s model but also showcases its potential to reshape approaches in various scientific domains, enhancing our understanding of the intricacies of causality.
Causality in Machine Learning
Causality in the realm of machine learning offers an insightful lens through which complex models can be interpreted and improved. The integration of causal inference within machine learning algorithms is not merely an academic exercise; it has real-world implications that shape decision making across various sectors. Understanding the cause-and-effect relationships rather than just correlations leads to better predictions and more effective interventions. This section explores the significance of causality within machine learning, emphasizing its multifaceted benefits and critical considerations.
Incorporating Causal Inference into Algorithms
Incorporating causal inference into algorithms essentially changes the landscape of how machine learning models are constructed and interpreted. Traditional approaches often focus purely on statistical relationships, running the risk of missing the deeper, underlying structures that govern data generation.
To weave causality into machine learning, researchers and practitioners use causal frameworks such as Judea Pearl's causal graphs. These frameworks enable practitioners to delineate between mere correlations and true causative influences.
Some pivotal aspects of this incorporation include:
- Enhanced Model Interpretability: By understanding the causal pathways between features, stakeholders can make sense of machine learning predictions.
- Improved Decision-Making: Models that incorporate causal relationships can support better strategic choices in fields like healthcare or marketing, where outcomes depend on specific actions.
- Robustness to Confounding Variables: When algorithms are speechless about confounding variables, causal understanding helps in adjusting the models. This reads into the core implications of features and their actual influences on the target variable.
Here is a simple snippet that illustrates how a causal inference might be initiated in a Python environment:
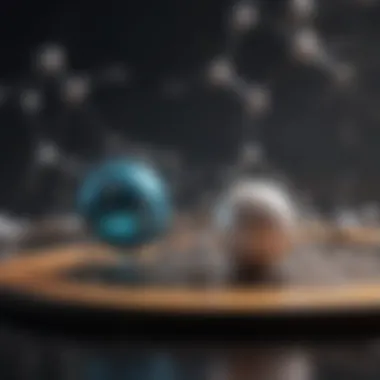
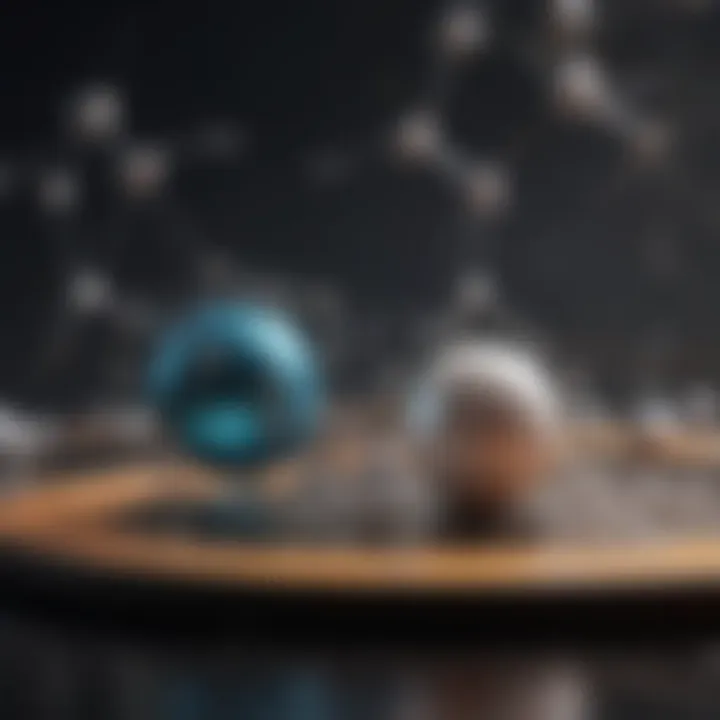
As practitioners cultivate these causal insights, the potential for innovation in real-world applications grows tremendously. The ability to alter inputs in a calculated manner leads to optimized outputs, thus paving the path for advancements across industries.
Real-world Applications
The real-world applications of integrating causality in machine learning run the gamut from healthcare discoveries to marketing optimizations. Some notable examples are:
- Healthcare: In clinical research, causal inference can reveal the effectiveness of treatment plans or identify harmful effects from medication. For instance, causal analysis in randomized trials often leads to better patient outcomes by revealing genuine cause-effect relationships.
- Finance: Causality helps financial institutions in credit scoring and risk assessment. By discerning the factors that truly influence a borrower’s likelihood of defaulting on a loan, banks can refine their models, enhancing both decision accuracy and profitability.
- Marketing Strategies: Companies utilize causal models to understand the effectiveness of their advertising campaigns. They can pinpoint which marketing strategies lead to increased sales rather than merely tracking an uptick in revenue linked temporally to advertising expenditures.
"Causality provides a framework that not only refines machine learning outcomes but also aligns them more closely with real-world phenomena, ensuring practical relevance in applications."
- Policy Making: Government bodies can employ causal inference to evaluate the impact of policies. For instance, by investigating the effects of a new tax law, policymakers could better understand the direct consequences of fiscal changes on the economy.
In summary, the infusion of causal inference into machine learning is more than just a theoretical enhancement. It’s a leap towards understanding the underlying mechanisms that drive outcomes, thereby fostering innovations that lead to tangible benefits across various fields.
The Role of Causality in Social Sciences
Causality plays a fundamental role in the social sciences, serving as the backbone for understanding relationships between variables and the dynamics of societal phenomena. Grasping causal relationships helps researchers make sense of complex social systems, enabling them to draw meaningful conclusions about human behavior, economic trends, and political movements. When applied effectively, causal reasoning can alter how we approach problem-solving and policy formulation in the public domain.
Causal Reasoning in Economics
In economics, causal reasoning is crucial for predicting outcomes and assessing the effects of various interventions. Economists utilize models that hinge on causal inference to identify factors that contribute to economic growth or recession. For example, consider the relationship between education and income levels. An economist might analyze whether rising education rates directly lead to increased earnings or if other factors, like industry demand, play a significant role.
To effectively illustrate causality in economic models, professionals often employ:
- Statistical Analyses: Methods such as regression analysis can help quantify relationships and isolate causal impacts.
- Natural Experiments: Researchers might rely on unexpected events to study their effects on economic indicators. For instance, a sudden tax policy change can serve as a natural experiment to assess shifting consumer behavior.
- Instrumental Variables: This approach helps control for variables that might bias estimates, providing a clearer picture of cause-and-effect relationships.
A real-world example is the economic impact assessments performed after significant policy changes, like the introduction of minimum wage laws in various regions. By observing patterns of employment and income post-implementation, economists can infer whether the rise in wage directly affects job availability or if concurrent economic processes are at play.
Implications for Policy Making
Understanding causality also holds immense implications for policy making. Policymakers, guided by empirical evidence of causal relationships, can design more effective interventions. The challenge lies not only in establishing causation but also in translating these findings into actionable policies.
For instance, if studies indicate that increased funding for early childhood education results in improved long-term academic performance, policymakers are more likely to prioritize such funding. Furthermore, causality aids in identifying unintended consequences. By understanding causality deeply, lawmakers can anticipate negative effects of proposed legislation, thus fostering more rounded strategies.
Some critical considerations in this context include:
- Data Quality: Reliable, high-quality data is pivotal for accurate causal inference.
- Longitudinal Studies: Continuous observation over time helps track changes and reinforces causal understanding.
- Interdisciplinary Cooperation: Collaborations between economists, sociologists, and psychologists can enrich causal insights, creating a more holistic view of social dynamics.
"Establishing clear cause-effect relationships can transform how we formulate and implement public policies, making approaches not just reactive but predictive."
In summary, the role of causality in the social sciences cannot be overstated. By integrating rigorous causal reasoning into economic models and policy frameworks, researchers and practitioners can navigate the complexities of human society more effectively, achieving outcomes grounded in evidence and enhanced understanding.
Causal Inference and Public Health
Causal inference plays a pivotal role in public health, as it enables researchers and practitioners to make informed decisions that can ultimately save lives. Understanding the cause-and-effect relationships between various health interventions and outcomes allows for targeted strategies aimed at maximizing benefits and minimizing adverse effects. Causal inference provides a structured approach to dissect complex systems inherent in public health issues, leading to more effective policies and interventions. By integrating Judea Pearl's pioneering work in causality, this section explores how his contributions illuminate the pathways through which health determinants exert influence on health outcomes.
Assessing Treatment Effects
When evaluating the effectiveness of public health interventions, accurately assessing treatment effects is crucial. This process entails determining whether a particular treatment improves health outcomes and, if so, to what extent. Pearl's structural causal model lends itself well to this process, allowing researchers to model the effect of a treatment while accounting for confounding variables, ensuring a clearer picture of its true efficacy.
For instance, consider a study evaluating the impact of a vaccination program on reducing disease incidence. Using causal diagrams, researchers can visualize relationships between vaccination uptake, incidence of disease, and potential confounders like age or socioeconomic status. This visual representation facilitates a systematic examination of how vaccination directly influences disease rates, helping to isolate the treatment's effect from other influencing factors. A real-world example can be found in the application of causal inference in analyzing randomized controlled trials, which are heralded as the gold standard in treatment evaluation.
"An understanding of causal relationships is integral to public health, as it guides effective interventions and policies."
Public Health Interventions
Public health interventions encompass a broad array of measures aimed at improving health outcomes at population levels. These can range from vaccination campaigns, health education initiatives, nutritional programs, to measures that address social determinants of health. An essential consideration in the design and implementation of these interventions is the ability to predict their impact using causal inference methods.
Causal inference not only helps in assessing the impact of existing public health initiatives but can also inform the design of new ones. For instance, by leveraging Pearl's framework, public health officials can evaluate the potential impacts of policies designed to increase access to healthcare in underserved communities. The insights gained from such analyses can lead to evidence-based decisions that promote health equity and maximize the overall efficacy of interventions.
In summary, the employment of causal inference in public health allows for a deeper understanding of the effectiveness and direct impact of interventions. It empowers decision-makers by providing clarity amidst the complexities of health data, ultimately supporting more strategic and justified health policies.
Pearl's Contribution to Artificial Intelligence
Judea Pearl’s influence on artificial intelligence (AI) is profound and far-reaching, encapsulating theories and methodologies that effectively merge the realms of causality with machine learning. By introducing a framework that emphasizes causal relationships, Pearl provides AI researchers and practitioners with essential tools to navigate complexities inherent in data interpretation. His work fosters an understanding of how various factors interact, ultimately leading to more robust and reliable AI systems.
Causality in AI Models
Incorporating causal inference into AI models represents more than a mere mathematical tweak; it signifies a paradigm shift. Traditional AI techniques often focus purely on correlations, leaving researchers with a multitude of patterns without insight into their origins. Pearl's methodologies, particularly through causal diagrams, empower researchers to visualize and manipulate these relationships. For example, consider a healthcare AI model that predicts patient outcomes based solely on statistical correlations between symptoms and diagnoses. Without a causal framework, the model might miss crucial underlying factors, leading to misleading predictions. Pearl’s causal models foster a deeper understanding by enabling researchers to probe these connections.
- Causal Relationships: Instead of the usual black-box algorithms, Pearl’s models help explain why certain outcomes happen based on specific inputs, enhancing transparency.
- Actionable Insights: By calculating the probabilities of various outcomes, AI systems can provide actionable recommendations. For instance, a marketing AI might determine not only which ads work but also why they resonate differently across demographics.
- Increased Robustness: When systems can identify and account for confounding variables, the reliability of predictions significantly improves.
As AI continues to evolve, integrating these causal approaches allows for models that are not just reactive but proactive, anticipating outcomes based on strategic manipulations of variables. The emphasis on causality thus catapults AI into a realm where understanding nuances and complexities become possible rather than merely guessing outcomes.
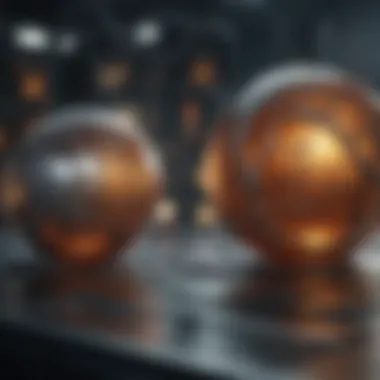
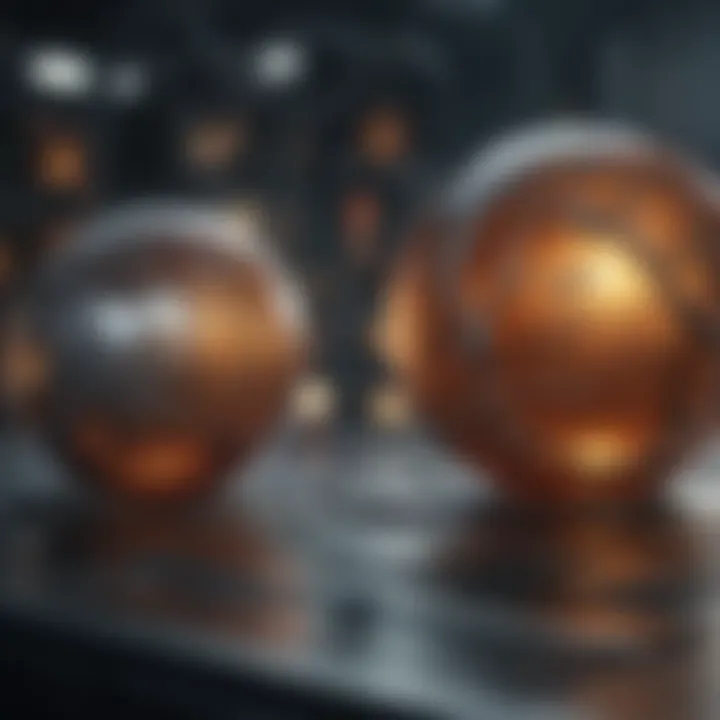
Challenges and Future Directions
While Pearl’s contributions to AI are invaluable, they come with a distinct set of challenges.
- Complexity in Modeling: The intricacies of real-world data can lead to overly complex causal diagrams that are difficult to interpret. Simplifying these diagrams without sacrificing Valuable information remains a significant hurdle.
- Computational Limitations: Causal inference, especially in large datasets, demands substantial computational resources. Current systems may struggle with the demands of these advanced algorithms, requiring a balance between complexity and utility.
- Integration with Existing Systems: Merging causal frameworks with established machine learning systems can be cumbersome. There’s often resistance towards adopting new methodologies, especially in industries accustomed to traditional correlation-based approaches.
Looking ahead, the future of AI enriched by causal inference seems promising. By investing in advanced algorithms that automatically detect causal relationships, researchers are likely to pave the way for breakthroughs we have yet to imagine. Interdisciplinary collaborations that blend knowledge from statistics, computer science, and domain-specific expertise also stand to enrich this field significantly.
"Causality might be the key to unlocking a deeper understanding of intelligence in machines and humans alike. By grasping the why behind the data, we pave the way for more meaningful applications of AI."
As technology progresses, the lessons learned from Judea Pearl's work will inform new directions in AI, ultimately leading to systems that aren't just efficient, but also intelligent in a deep, causative sense.
By embedding causality into AI, we are not only enhancing analytical competence but also building trust in AI systems that fundamentally improve decision-making processes across a broad spectrum of fields.
Critiques and Controversies
The discourse surrounding Judea Pearl’s contributions is not devoid of contention. Critiques and controversies around causality and its applications often serve as a catalyst for deeper investigations in the field. By engaging with these debates, we grasp the significance and potential shortcomings in Pearl's framework. This section will lay out the pivotal conversations within the scientific community that challenge Pearl's assertions and theories.
Debates in the Scientific Community
Engaging with debates in the scientific community is crucial for a comprehensive understanding of any field, and causality is no exception. Numerous scholars have brought forth diverse perspectives on Pearl's methodology, often honing in on the assumptions underlying his structural causal models. For instance, while Pearl’s approach seeks to establish a clear demarcation between correlation and causation, critics argue that the reliance on graphical models can oversimplify complex interactions.
In one such debate, researchers have countered that while causal diagrams offer clarity, they can also obscure nuances in data. These critiques are not merely academic exercises; they have real-world implications, particularly in fields such as epidemiology or economics, where decisions driven by causal inferences can affect public policy or health interventions.
Moreover, some critiques underscore the limitations of counterfactual reasoning. Detractors suggest that imagining alternate scenarios can be fraught with biases and speculations. This skepticism is not to dismiss Pearl’s contributions but rather to enrich the conversation around the boundaries of what causality can explain.
Counter-arguments to Pearl's Theories
Challenging established theories is an essential aspect of scientific progress, and Pearl's work is no exception. Critics have nuanced arguments that question the interpretations and implications of his work on causality. One prevalent counter-argument proposes that Pearl’s emphasis on certain variable relationships may lead to overfitting in statistical models. In situations where there are many variables, isolating the influence of one can potentially mislead researchers.
Furthermore, the dependency on specific causal structures can face critique. There are scholars who contend that assumptions about the independence of certain variables can introduce errors if the real-world dynamics display intricate dependencies.
A notable discussion stems from the contrasting viewpoints on the do-calculus that Pearl promotes. Critics argue that while this calculus provides a robust framework for some scenarios, it may lack flexibility in cases with unobserved confounding factors. The complexity of human behavior and societal systems often resists simplification into neat causal diagrams.
"Understanding the critiques of Pearl's work not only illuminates its limitations but also inspires new avenues of research that might lead to more comprehensive models of causality."
In summary, these critiques and controversies are not roadblocks but milestones on the path to refining causal theories. By diving into these constructive debates, the field of causality can evolve, paving the way for more sophisticated models that reflect the intricacies of our world.
Future of Causality Research
The realm of causality research, particularly in light of Judea Pearl's contributions, presents a fertile ground for exploration and innovation. As advancements in technology, methods, and strategies flow in, the significance of understanding causal relationships remains crucial across various domains, from social sciences to artificial intelligence. In this section, we dive into the emerging trends and technologies reshaping the landscape of causal research and the interdisciplinary approaches fostering growth in this field.
Emerging Trends and Technologies
Causality research is not stagnant; it's a dynamic field that contends with new-age challenges and possibilities. Several key trends are emerging that warrant attention:
- Machine Learning Integration: The blending of causal inference with machine learning algorithms is making waves. This fusion aids in better decision-making by accounting for causal relationships rather than mere correlations.
- Big Data Approaches: As we amass a mountain of data, the capability to harness big data analytics for causal reasoning is becoming paramount. Techniques such as causal discovery, which unveil causal structures from vast datasets, are gaining traction.
- Software Tools and Frameworks: Innovations like the DoWhy library and the CausalML framework are simplifying the implementation of Pearl’s causal models in practical scenarios. More researchers can now freely test and explore causal assumptions.
- Policy Simulation Models: Simulating public policy choices with causal models is becoming routine in social policy research. Such models allow for prospective analysis—enabling researchers and policymakers to anticipate outcomes in various scenarios before implementation.
"The future of causality research will hinge not only on theoretical advancements but on practical applications that can directly inform real-world decision-making."
With these trends in motion, researchers are better equipped to elucidate the intricate webs of cause and effect that govern our world.
Interdisciplinary Approaches
As the landscape evolves, the importance of interdisciplinary collaboration cannot be overstated. Fields such as psychology, economics, epidemiology, and computer science are now intersecting in fascinating ways. Some aspects of this interdisciplinary push include:
- Collaboration Across Fields: By engaging researchers from different disciplines, causal inference benefits from varied perspectives and methodologies, enriching analysis and expanding its applicability.
- Shared Languages and Frameworks: Initiatives aimed at standardizing terminologies and frameworks across disciplines promote clearer communication, thus facilitating better understanding among practitioners in diverse fields.
- Education and Training Programs: As causality grows in importance within various academic contexts, tailored educational programs are emerging. These programs aim to equip students with skills that bridge gaps between theories and practices in their respective disciplines.
As we peer into the future, it's evident that the journey of causality research is about collaboration, integration, and continual evolution. Positive advancements will not only refine our understanding of causality but will also empower researchers to tackle complex societal issues with confidence and precision.
Finale
The conclusion of an article serves as a critical reflection point that encapsulates the essence of the entire discussion. In this article, the examination of Judea Pearl's contributions to the realm of causality is not only insightful but pivotal, as it sheds light on how his work reshapes our understanding of causal relations in complex systems. A well-articulated conclusion does the heavy lifting by summarizing key insights and reaffirming their relevance in contemporary research.
Summary of Key Insights
The exploration of Judea Pearl's theories of causality reveals several pivotal insights:
- Causal Models: Pearl introduced structural causal models that articulate causal relationships through directed graphs. This framework is not merely theoretical; it lays the groundwork for practical applications in data science and other fields.
- Counterfactuals: His emphasis on counterfactual reasoning alters how researchers approach causal inquiries, moving from simple correlations to deep, meaningful interpretations of data.
- Interdisciplinary Impact: Pearl’s work transcends the boundaries of computer science, impacting disciplines such as economics and public health. This underscores the versatility and applicability of his contributions.
- Durable Legacy: The tools and concepts developed by Pearl continue to influence new generations of researchers, pushing boundaries in fields like artificial intelligence and machine learning.
The Lasting Impact of Pearl's Work
Judea Pearl's legacy is not just in the theories he proposed but in their transformative effects on scientific inquiry. The importance of his work lies in its ability to provide clarity and structure to the often murky waters of causality. By advocating for a rigorous approach to causal inference, he has equipped researchers with vital frameworks for understanding complex systems.
"Pearl's work teaches us that without a clear causal framework, we risk making decisions based on mere correlation, which can lead to misguided conclusions."
The ramifications of Pearl’s contributions are manifold:
- Educational Shifts: His theories are now integral in data science curricula, shaping how future generations think about data and its implications.
- Enhanced Decision-Making: Researchers and policymakers have access to more precise tools for impact evaluation, enhancing the efficacy of interventions and policies.
- Expanded Research Horizons: The focus on causal structures encourages interdisciplinary collaboration, fostering innovations that might not occur in isolated academic silos.
- Ethics in AI: Pearl's insights challenge the ethical dimensions of artificial intelligence applications, urging developers to consider causal reasoning to mitigate biases and incorrect predictions.
In sum, Judea Pearl’s contributions offer a robust framework that enhances our understanding of causality across disciplines. The conclusion not only summarizes essential insights but also hints at the endless possibilities that lie ahead in applying his groundbreaking theories. The pursuit of understanding causality continues, inspired by Pearl's legacy, promising to yield rich fields of inquiry for those willing to explore.