Exploring Hoffman Models in Science and Math
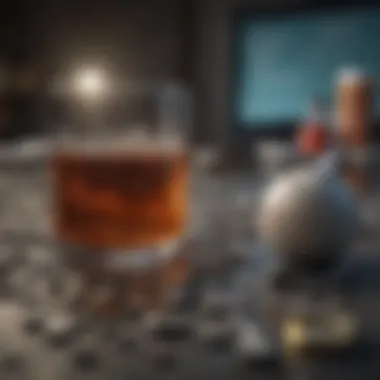
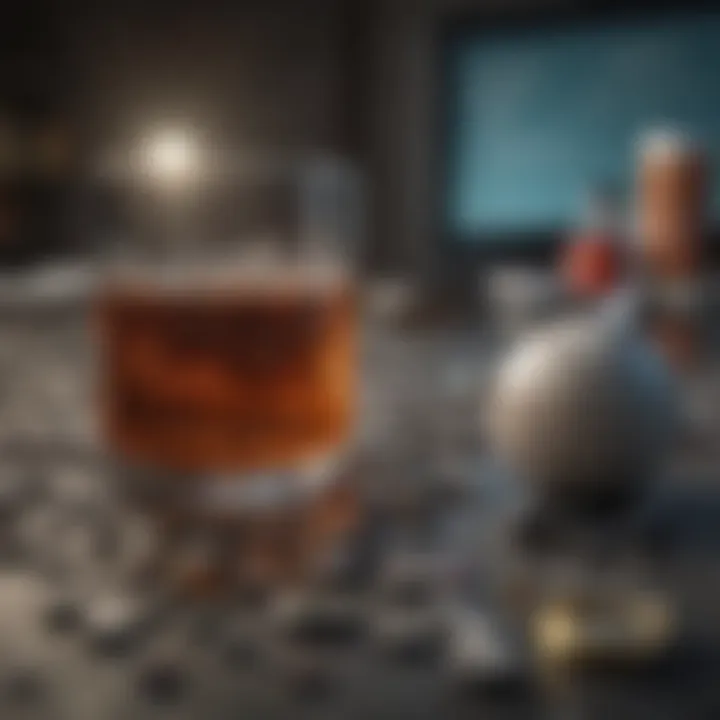
Intro
The Hoffman models represent a significant intersection of diverse fields, particularly mathematics, physics, and computer science. These models are not merely academic constructs; they are tools that aid in the understanding and application of complex ideas. With their roots in foundational mathematics, the evolution of these models illustrates the dynamic relationship between theoretical development and practical application. This exploration seeks to unravel the intricate threads that connect these models to their broader implications in educational contexts and beyond, emphasizing their utility for students, researchers, and educators alike.
Key Research Findings
Overview of Recent Discoveries
Recent studies on the Hoffman models have illuminated diverse applications across various scientific fields. Researchers have made notable advancements in understanding how these models can simplify complex mathematical theories. Importantly, they help bridge the gap between abstract concepts and real-world scenarios. In physics, for instance, Hoffman models provide frameworks for exploring chaotic systems and nonlinear dynamics.
Significance of Findings in the Field
The significance of the findings cannot be understated. The Hoffman models have not only contributed to theoretical discussions but have also facilitated practical scientific advancements. Their implications stretch across computational methods and educational practices. By implementing these models, educators have reported improved comprehension among students, highlighting their potential to motivate and engage learners in science and mathematics.
"The exploration of Hoffman models unveils not just their theoretical robustness but also their practical appeal in educational settings."
Breakdown of Complex Concepts
Simplification of Advanced Theories
One of the most compelling aspects of the Hoffman models is their capacity to distill complex theories into more manageable frameworks. For students and researchers alike, navigating the dense landscape of scientific concepts can often be daunting. The Hoffman models serve as a gateway, providing tools that allow for a clearer understanding of intricate theories, such as those found in topology and statistical mechanics.
Visual Aids and Infographics
The role of visual aids in comprehending the Hoffman models cannot be overlooked. Incorporating infographics can significantly enhance retention and understanding. For example, a visual representation of a Hoffman model in a chaotic system can help clarify relationships between variables, revealing patterns that might remain hidden in textual descriptions. These aids simplify complex data and make learning more interactive, fostering a deeper engagement with the material.
In summary, the Hoffman models offer a multi-faceted approach to understanding advanced scientific concepts, enriching the educational landscape for students and professionals. The ongoing research and applications of these frameworks continue to reshape the discourse in mathematics and science, making them invaluable for anyone pursuing knowledge in these fields.
Prolusion to Hoffman Models
The Hoffman models represent an intricate framework that bridges multiple scientific domains. They are essential in advancing understanding in mathematics, physics, and computer science. This section will explore the historical background, purposes, and overall relevance of these models.
Historical Context and Development
Hoffman models have roots that trace back several decades. Their development is intertwined with the evolution of scientific thought. Early formulations were shaped by mathematicians and scientists who sought to simplify complex phenomena. This simplification often involved abstract models to represent real-world situations.
The pioneering work of Karl Hoffman in the mid-20th century laid the groundwork. He focused on qualitative analysis which helped in understanding systems that exhibit nonlinear behaviors. Researchers expanded on his findings, adapting the models to suit various scientific needs. Over the years, key contributors have emerged, refining the modelsβ applications in diverse areas like chaotic systems and optimization problems. The progression from simple linear representations to complex nonlinear frameworks highlights the growing sophistication in modeling techniques.
In recent years, technological advancements have catalyzed further development. Computational power allows for the simulation of intricate models that were previously intractable. This has opened avenues for real-world applications where Hoffman models can provide insights into intricate systems such as climate change, economic forecasts, and more. Understanding its historical context provides a solid foundation for appreciating its impact today.
Purpose and Relevance
The main purpose of the Hoffman models lies in their ability to provide structured insights into complex scientific situations. They aid researchers in visualizing data, predicting outcomes, and testing hypotheses. Their relevance becomes particularly apparent in three key areas: education, research, and application.
- In education, Hoffman models serve as effective teaching tools. They help students comprehend abstract concepts by providing a clear framework to analyze problems systematically.
- In research, the models facilitate innovative approaches to problem-solving. Scientists are able to experiment with various parameters and see how results change, leading to deeper comprehension of underlying principles.
- In practical applications, such as in physics and computer science, these models are instrumental. They offer streamlined solutions for simulations in fields ranging from algorithm design to thematic research.
Ultimately, the purpose of the Hoffman models is not just theoretical; they enhance our practical understanding of complex systems.
"The significance of Hoffman models transcends academia; they reshape our methodologies in addressing scientific inquiries."
Recognizing their purpose and relevance fosters appreciation for their integration across scientific disciplines. As we delve deeper into the core concepts of Hoffman models, it is crucial to understand the foundations that make them vital to both educational frameworks and scientific innovation.
Core Concepts of Hoffman Models
The core concepts of Hoffman Models serve as the backbone of this exploration. Understanding these elements is critical for grasping how these models function and their significance in various fields such as mathematics, physics, and computer science. The fundamental underpinnings not only reveal the structure of these models but also inform their applications in real-world scenarios. Critical among these are the principles that define the models and their mathematical foundations. By examining these aspects, we can appreciate the complexities and capabilities that the Hoffman models bring to scientific inquiry.
Fundamental Principles
The Hoffman Models rest on several key principles. These principles guide how models are developed and applied. The first principle is modularity. Modular systems can be broken down into smaller, manageable parts. This modular approach makes it easier for researchers to analyze complex phenomena. Each module can be studied independently, which simplifies the overall model.

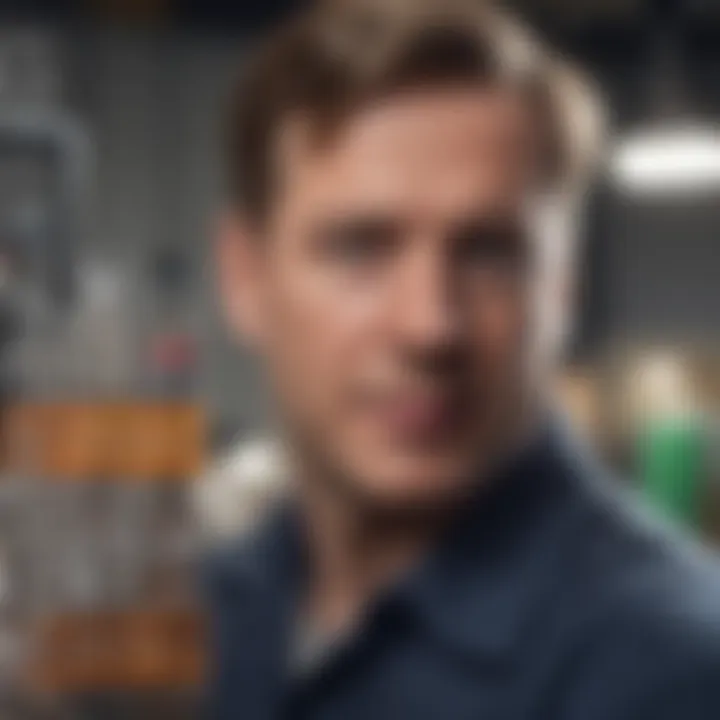
Another crucial principle is scalability. Scalable models can be expanded or contracted without losing functionality. This feature allows researchers to apply the models to both small-scale and large-scale problems. Finally, adaptability is essential. Models must adjust to new information or changing conditions in their respective fields. This adaptability ensures that the models stay relevant over time.
Some benefits of these principles include:
- Easier understanding of complex systems
- Flexibility in application
- Robustness in handling various scientific inquiries
Mathematical Foundations
The mathematical foundations of Hoffman Models are vital for their effectiveness. These models often utilize concepts from linear algebra, calculus, and statistical methods. The reliance on these mathematical areas allows the models to accurately represent relationships and dynamics found in real-world systems.
Linear Algebra plays a significant role in modeling multi-dimensional data. Through techniques like matrix operations, researchers can analyze interactions between various components of a system. For instance, in a physics context, linear equations can describe forces acting on an object.
Calculus is integral in understanding changes within systems. Concepts such as derivatives and integrals help to model rates of change and accumulation. These are commonly found in physical phenomena such as motion and energy transfer.
Statistical methods allow researchers to draw conclusions from data. They help in estimating probabilities, which is crucial in fields like computer science where predictive algorithms are built.
The mathematical structure of the Hoffman Models can be complex but understanding it is key for practical application. This grounding sets the stage for addressing real-world issues practically.
"The mathematical integrity of Hoffman Models ensures their reliability and applicability across scientific disciplines."
In summary, the core concepts of Hoffman Models focus on establishing a framework that is both practical and theoretical. With a firm understanding of these concepts, one can explore the breadth of applications that these models facilitate in scientific inquiry.
Types of Hoffman Models
Understanding the various types of Hoffman Models is crucial for comprehending their applications in science and mathematics. Each type presents distinct characteristics and benefits, addressing specific problems across different fields. The classification encompasses linear, nonlinear, and dynamic models, each vital in their own right.
Linear Hoffman Models
Linear Hoffman Models are based on linear relationships, making them relatively straightforward to analyze. They are defined by linear equations, which imply a constant rate of change. In applications, these models are often used in scenarios where proportionality is expected. For example, they are applied in financial modeling and resource allocation problems where the relationships between variables remain consistent.
The simplicity of linear models makes them ideal for introductory learning in mathematical contexts. Students can grasp fundamental principles without dealing with complexities often found in nonlinear scenarios. Additionally, linear models are less computationally intensive, providing quick results, which is beneficial for both teaching and practical applications.
Nonlinear Hoffman Models
Unlike linear models, nonlinear Hoffman Models present complexities in their equations that can cause variable interactions to change unpredictably. This complexity mimics many real-world situations, where factors do not have a constant relationship. Nonlinear models are crucial in fields such as ecology and economics, where predictions must account for changing rates of interaction.
The challenge of working with nonlinear models lies in their analytical and computational requirements. These models demand a deeper understanding of mathematics and often require the use of numerical methods or simulations for solution. As students and research professionals engage with these models, they gain insights into intricate systems and non-proportional behaviors, enhancing their critical thinking skills.
Dynamic Hoffman Models
Dynamic Hoffman Models extend the concept further by incorporating time as a variable that affects system behavior. The dynamic nature allows for the analysis of systems that evolve, providing insights into transitional phases and time-dependent change. This type of model is invaluable in fields like physics, where understanding the patterns of motion over time is essential.
These models require more sophisticated mathematics, often including calculus or differential equations. As such, they are often employed in advanced educational settings. Engaging with dynamic Hoffman Models enables learners and professionals to analyze trends and predict future states through time-evolving systems.
"Effective application of Hoffman Models provides a solid framework for exploring complex scenarios in mathematics and science."
Applications of Hoffman Models
The applications of Hoffman models are a crucial component of their overall significance in the fields of mathematics, physics, and computer science. These models provide frameworks that help to conceptualize complex processes and systems. By refining mathematical theories or enhancing physical experiments, the applications of Hoffman models demonstrate their value in both theoretical and practical contexts.
In essence, their applications span various domains, including mathematical problem solving, physical experimentation, and computer algorithm development. The benefits of utilizing these models include improved analytical tools, enhanced learning experiences, and expanded avenues for research and innovation.
In Mathematics
In the realm of mathematics, Hoffman models play a transformative role. They provide structured methods for approaching various mathematical problems. For instance, the linear and nonlinear models enable mathematicians to analyze equations and functions more rigorously. These models help in visualizing relationships among variables, culminating in insightful solutions. The integration of Hoffman models in mathematical curricula enhances student comprehension as they facilitate the understanding of abstract concepts.
Hoffman models can also assist in numerical analysis and optimization problems. Researchers and students can use these models to simplify complex calculations or explore high-dimensional spaces. The clear representation of problems leads to a more efficient problem-solving process, ultimately improving mathematical literacy and proficiency.
In Physics
In physics, the applications of Hoffman models are equally valuable. These models assist in describing physical phenomena and analyzing experimental data. By employing dynamic Hoffman models, physicists can better represent systems that change over time, such as fluid dynamics or astrophysical simulations. The ability to model interactions in a cohesive manner allows researchers to generate accurate predictions about physical outcomes.
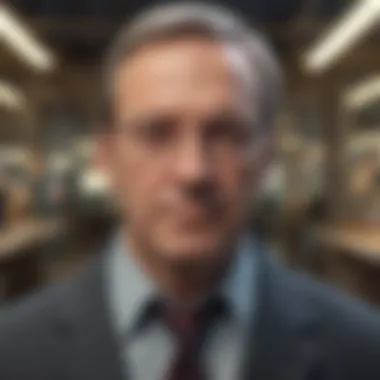
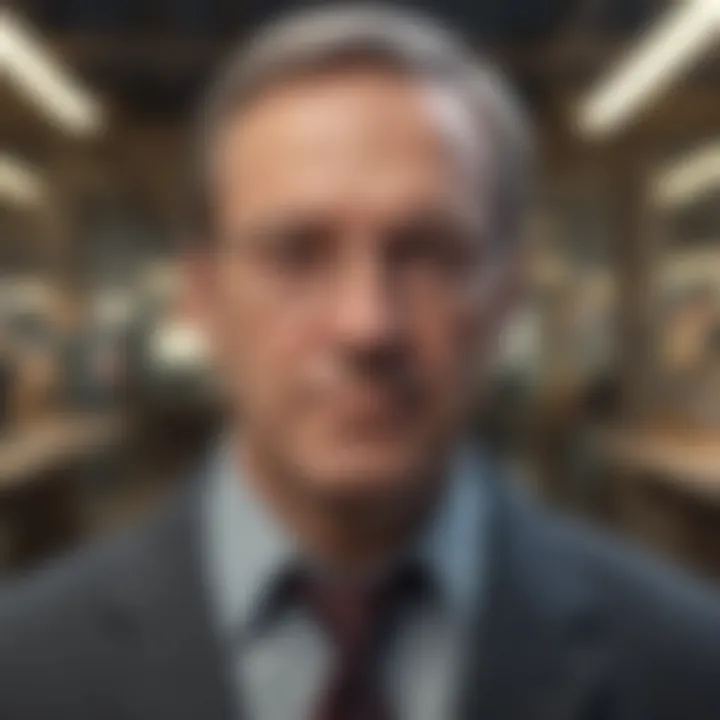
Moreover, Hoffman models contribute to enhancing the design of experiments. By knowing how different variables interact, physicists can refine their methodologies and focus on crucial factors. This leads to more reliable and valid results, important for advancing scientific understanding. The link between theory and experimentation strengthens through these models, providing a solid foundation for future discoveries.
In Computer Science
In computer science, Hoffman models are instrumental in the development of algorithms and computational strategies. These models aid in analyzing the efficiency of algorithms, ensuring optimal performance. For example, using Hoffman models can help developers understand how data flows within complex systems, allowing for the identification of bottlenecks or redundancies. This understanding is critical for optimizing computer programs and enhancing user experience.
Furthermore, Hoffman models facilitate advancements in artificial intelligence and machine learning. They provide frameworks that can be employed to understand neural networks and deep learning processes. By translating real-world complexities into manageable models, researchers can create more accurate and intelligent systems. This ongoing evolution of Hoffman models in computer science signifies their role as an essential tool for navigating the future of technology.
"The applications of Hoffman models reveal their critical role in understanding, teaching, and innovating across multiple fields of study."
Practical Examples of Hoffman Models
The examination of Hoffman models is not merely an abstract endeavor; it finds potent expression through practical examples that illustrate their applicability in varied contexts. Such instances offer not only insight into their theoretical underpinnings but also present tangible benefits in solving real-world issues. Analyzing specific case studies demonstrates how Hoffman models contribute to mathematical problem-solving, physics experimentation, and algorithm development, respectively.
Case Study: Mathematical Problem Solving
In the domain of mathematics, Hoffman models facilitate problem-solving by providing structured frameworks. Consider a situation where students are tasked with tackling complex geometric problems. Using a linear Hoffman model allows for a step-by-step approach, breaking down the problem into manageable parts. This structured pathway encourages critical thinking, guiding students through a logical progression toward the solution.
For instance, a geometrical problem focused on triangle congruence could employ the principles of a linear Hoffman model to draw parallels between known and unknown dimensions. By applying formulas in a systematic manner, students develop a deeper comprehension of geometric relationships. This practical use ensures that theoretical concepts become accessible, enhancing both student confidence and academic performance.
Moreover, the modelβs emphasis on reasoning boosts analytical skills, which are crucial not just in theoretical mathematics but in various disciplines, further emphasizing the importance of Hoffman models in educational contexts.
Case Study: Physics Experimentation
Turning to physics, the implementation of Hoffman models can significantly reshape experimentation methodologies. When conducting experiments involving forces and motion, researchers often rely on nonlinear Hoffman models to duplicate the complex behaviors of physical systems. Nonlinear dynamics can create detailed simulations that present invaluable insights about outcomes based on varying conditions.
For example, consider an experiment focused on projectile motion. When applied, a nonlinear Hoffman model can account for variables like air resistance and gravitational fluctuations. By modeling these factors, physicists can predict how adjustments in velocity and angle influence the trajectory of an object. This detailed analysis leads to more accurate predictions, fostering innovative approaches in experimental design.
Furthermore, this practical example underscores how Hoffman models help streamline intricate experiments, ensuring that hypotheses are rigorously tested and refined based on data gathered from real phenomena.
Case Study: Algorithm Development
Finally, in the field of computer science, Hoffman models find practical applications in algorithm development. Algorithms are foundational to executing processes and solving complex problems efficiently. By employing dynamic Hoffman models, programmers can create algorithms that adapt based on user inputs and operational environments.
Take the instance of machine learning. Here, dynamic Hoffman models allow for the continuous refinement of algorithms based on incoming data. This adaptability enhances the efficiency of data processing and the accuracy of the models developed. For example, an algorithm designed for image recognition can adjust its parameters based on new training data, ultimately leading to improved performance over time.
"The adaptability of dynamic Hoffman models in algorithm development is akin to the evolution of approaches in response to changing data landscapes."
Therefore, examining these practical examples reveals the multifaceted utility of Hoffman models. From enhancing mathematical problem-solving strategies to advancing physics experimentation and optimizing algorithm development, their impact resonates across disciplines. Each case study provides compelling evidence of how these models not only facilitate learning but also drive innovation in scientific research.
Educational Implications of Hoffman Models
The Hoffman models offer more than theoretical frameworks in science and math; they carry significant educational implications. By leveraging these models in classroom settings, educators can enhance students' understanding of complex concepts. The integration of Hoffman models in education promotes deep engagement with material, encourages critical thinking, and supports practical applications of theory.
One major benefit is that these models help in bridging the gap between abstract theories and tangible practices. Students often struggle with mathematical and scientific principles when they see them solely in theoretical contexts. However, Hoffman models provide a structured way to visualize and apply these principles in real-world scenarios. This connection fosters greater interest and comprehension among learners.
Teaching Methodologies
Effective teaching methodologies that incorporate Hoffman models need to focus on interactivity and application. For instance, professors might utilize project-based learning which centers around the use of Hoffman models in real-life situations. This hands-on approach allows students to apply what they learn. They can solve complex problems by engaging directly with the subject matter.
Additionally, the use of collaborative learning techniques can enhance the educational experience. Students working in teams can exchange ideas and insights based on the Hoffman frameworks. This peer collaboration encourages diverse thinking and can often lead to innovative solutions. Furthermore, the variety between linear, nonlinear, and dynamic models can be an excellent starting point for comparative studies. Each type can stimulate discussions about their respective contexts and applications, enhancing critical thinking.
Another approach is using digital tools and simulations that replicate the behavior of these models. Software like Wolfram Alpha can allow students to visualize the models dynamically, bringing theoretical concepts to life. When learners can manipulate variables and immediately see outcomes, they develop a better grasp of the underlying math and science involved.
Curriculum Development
The integration of Hoffman models into curricula calls for thoughtful consideration and planning. Education systems can develop curricula that embed these models in a way that aligns with learning outcomes across mathematics, physics, and computer science. By doing so, they will ensure that students are not only exposed to these frameworks but also understand their relevance.
One essential element in curriculum development is to equip educators with the right resources and training. Teacher workshops focused on the mechanisms and implications of Hoffman models can enhance instructional quality. Moreover, textbooks and digital resources should be refreshed to include recent advancements in these models, making them more relevant to current educational standards.
Additionally, assessments can be developed that emphasize not only the understanding of the Hoffman models but also the application of these concepts in varied contexts. This means evaluating students on their ability to implement model frameworks in solving real-world problems.
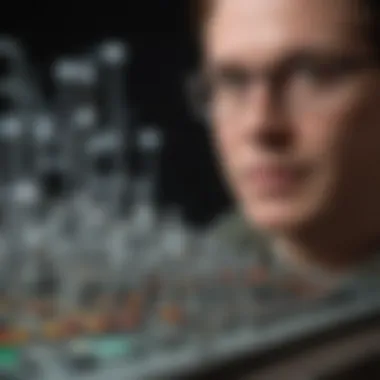
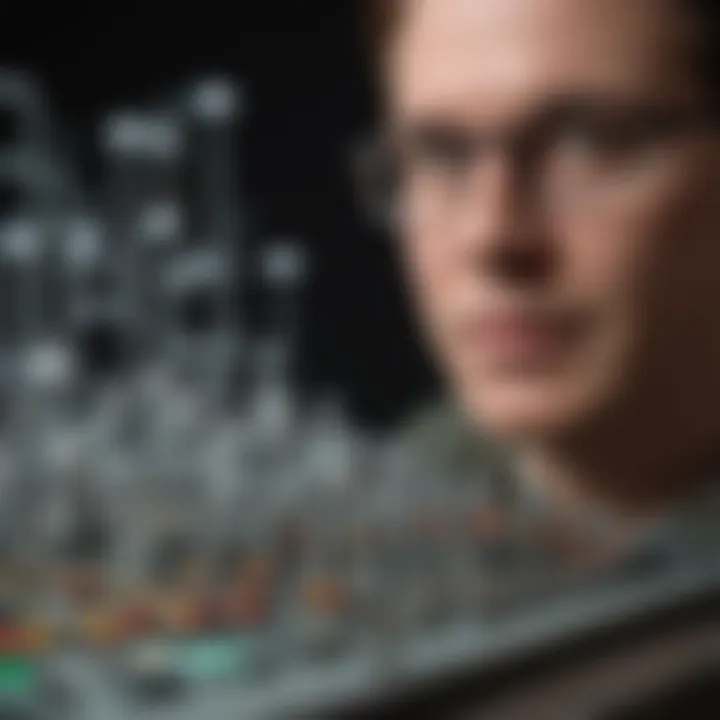
Key Insight: The effective application of Hoffman models in education can significantly enhance learner engagement and comprehension, ultimately preparing students for complex challenges in their academic and professional journeys.
Challenges and Limitations of Hoffman Models
This section deals with the challenges and limitations inherent in the application of Hoffman models. While these frameworks provide valuable insights in various scientific disciplines, their use does come with certain restrictions. Understanding these constraints helps researchers and educators to appreciate the models' scope and their potential misapplications in different contexts.
Mathematical Constraints
Hoffman models are built on specific mathematical foundations that dictate their scope. These constraints can limit their effectiveness in capturing complex phenomena. For example, some models may depend heavily on linear assumptions. In real-world scenarios, phenomena are often nonlinear. In such cases, applying a linear model can lead to significant errors in predictions and analyses.
Additionally, the complexity of the underlying mathematics can pose a barrier for educators and students. The steep learning curve associated with certain mathematical principles used in these models may discourage learners from engaging with the models in a meaningful way. The need for a strong foundational knowledge in both mathematics and theoretical concepts is essential to fully leverage the capabilities of Hoffman models.
Mathematical models are only as good as the assumptions upon which they are built.
Implementation Issues
The implementation of Hoffman models also faces practical challenges. One notable issue is the accessibility of data that is required for these models. Obtaining accurate, high-quality data can be a labor-intensive process. In situations where data is incomplete or noisy, model outputs may become unreliable.
Another critical aspect is the variability in interpretation among practitioners. Different users may apply the models differently based on their unique perspectives or experiences, leading to inconsistent results. Moreover, when incorporating these models into educational curriculums, teachers often face difficulty in making complex concepts relatable and understandable to students.
In summary, while Hoffman models have considerable potential, they are not without challenges. Understanding their mathematical constraints and implementation issues is essential for leveraging their power effectively in both research and educational contexts.
Future Directions in Hoffman Models Research
As we explore the realms of Hoffman models, a clear understanding of future directions shapes the trajectory of research and application. This section aims to provide insights into potential advancements and opportunities in the context of these models. Innovations and integration with various fields not only enhance the findings but also broaden the scope of understanding in mathematical and scientific communities.
Innovative Applications
The realm of innovative applications for Hoffman models is vast. Researchers are beginning to investigate how these models can be applied beyond traditional settings. For instance, interdisciplinary research can unlock new possibilities in data analysis, optimization problems, and predictive modeling. Different fields such as environmental science or economics can benefit from these models, adapting them for specific challenges.
Some potential innovative applications include:
- Complex System Analysis: Applying Hoffman models to understand interactions within complex systems can provide better forecasting capabilities.
- Real-Time Data Processing: Utilizing dynamic Hoffman models to analyze data streams can improve decision-making processes in real-time.
- Healthcare Models: Adaptation of Hoffman frameworks in medical research facilitates understanding patient care pathways and optimizing resource allocation.
The beauty of these applications lies in their flexibility. The models can evolve as new data emerges, allowing continuous refinement of hypotheses and outcomes.
Integration with Emerging Technologies
Another area ripe for exploration is the integration of Hoffman models with emerging technologies. The proliferation of computational resources and artificial intelligence presents new horizons for enhancing these models.
Important considerations regarding integration include:
- Machine Learning: Combining Hoffman models with machine learning techniques could lead to nuanced insights, enabling better interpretations of complex datasets.
- Artificial Neural Networks: These networks can synergize with Hoffman models to mimic cognitive processes, optimizing learning algorithms in various fields.
- Blockchain Technology: The robustness of Hoffman models, when integrated with blockchain, can enhance data security and integrity in sharing research findings.
By embracing these technologies, researchers can develop more robust models that address real-world problems effectively.
As we look forward to the future, the emphasis on innovation and integration suggests that Hoffman models will continue to evolve, paving the way for new discoveries and applications in science and mathematics. This direction not only highlights their relevance but also ensures that they remain integral to educational dialogues, research initiatives, and practical applications.
End
In concluding this exploration of Hoffman models, it is crucial to emphasize the multifaceted value of these frameworks across various disciplines. The synthesis of findings throughout the article highlights their core principles, types, and applications, particularly in the fields of mathematics, physics, and computer science. Understanding these models fosters deeper insights into complex scientific concepts, bridging theoretical knowledge with practical application.
Summation of Key Findings
The Hoffman models serve as a crucial tool in advanced scientific inquiry. Here are the key takeaways:
- Foundations: The models are built on strong mathematical principles, allowing for robust predictions and analyses.
- Types: Differentiation among linear, nonlinear, and dynamic models illustrates their diverse applicability.
- Applications: Real-world applications in mathematics, physics, and computer science show their usefulness in problem solving and experimental design.
- Educational Implications: These models provide significant enhancements to teaching methodologies and curriculum developments.
Thus, they represent an intersection of theory and practice that educates and drives innovation.
Implications for Future Research
Looking forward, the exploration of Hoffman models presents numerous avenues for further research. Understanding their implications can spur developments in:
- Innovative Applications: Researchers can adapt these models to new scientific challenges, especially in emerging fields like artificial intelligence and data science.
- Integration with Emerging Technologies: The potential for integration with tools like quantum computing and advanced data analytics indicates a promising direction for interdisciplinary collaboration.
- Educational Advancements: Continued research into effective teaching techniques employing these models could enhance student engagement and comprehension in STEM fields.
Overall, the ongoing evolution of Hoffman models invites both students and educators to contribute to a richer educational landscape and promote ongoing research in various scientific domains. According to Britannica, understanding such frameworks is essential for processing and interpreting complex scientific information.