Biological Intelligence vs Artificial Intelligence

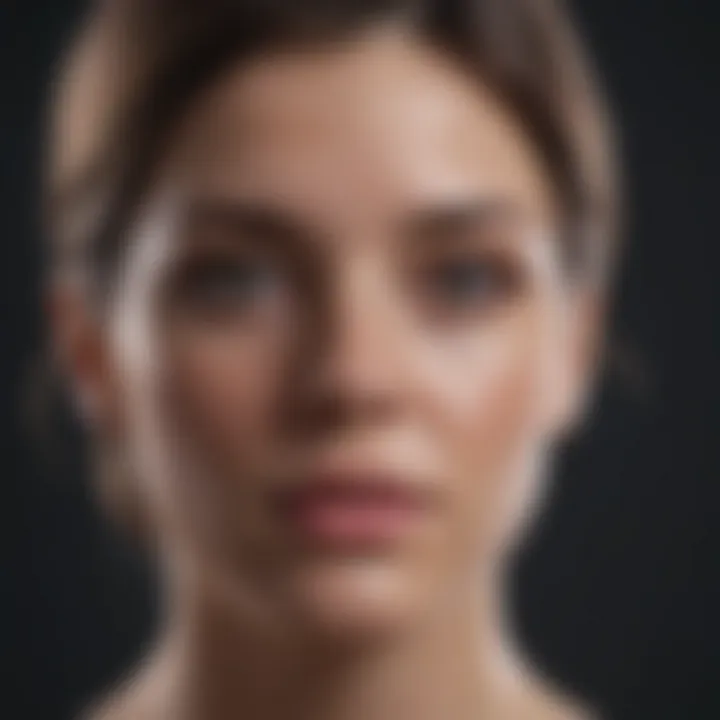
Intro
The relationship between biological intelligence and artificial intelligence is complex and multifaceted. Biological intelligence, inherent in human cognition, involves the intricate workings of the brain and neural networks. On the other hand, artificial intelligence represents a synthetic attempt to replicate or simulate aspects of human thought and reasoning using algorithms and machines. Understanding the nuances between the two can offer insights into their potential, limitations, and implications for society.
In exploring this field, it is crucial to examine how distinct yet interconnected these forms of intelligence are. The comparison often invokes questions of capability, evolution, and ethical implications, making it a relevant subject for educators, researchers, and professionals alike.
The following sections will unpack key research findings, simplifying advanced theories and their significance in our understanding of intelligence.
Prelude to Intelligence
The exploration of intelligence is fundamental to understanding both human capabilities and the evolving nature of technology. Intelligence, in its various forms, shapes our interactions, decision-making processes, and overall engagement with the world. To grasp the intricacies of biological systems versus artificial constructs, we need to define intelligence clearly and categorize the types that exist within these frameworks.
Defining Intelligence
Intelligence can be defined as the capacity to acquire and apply knowledge and skills. This encompasses a range of cognitive abilities, including reasoning, problem-solving, planning, abstract thinking, judgment, and learning. Biological intelligence refers to the innate cognitive capabilities found in living organisms, primarily seen in humans and animals. In contrast, artificial intelligence encompasses systems designed by humans to replicate or simulate these cognitive abilities.
The distinction between these two types is important. Biological intelligence has evolved over millions of years, deeply rooted in genetics and environmental influences. It combines emotion, experience, and intuition. Artificial intelligence, on the other hand, relies on algorithms, data, and predefined rules to function. Each type has a unique set of strengths and weaknesses, warranting an in-depth exploration.
Types of Intelligence
Understanding the types of intelligence is essential for analyzing their implications on society, technology, and ethics. In this section, we will explore biological and artificial intelligence, dissecting their contributions and examining their core characteristics.
Biological Intelligence
Biological intelligence is characterized by cognitive processes observed in living organisms. The key feature of biological intelligence is its adaptability. This adaptability allows individuals to learn from their environment, make decisions based on experience, and develop new skills throughout their lives.
One important aspect to note is the impact of emotions on biological intelligence. Emotions play a vital role in decision-making and learning. They influence what one remembers and how one interacts with others. This characteristic underlines the profound depth of biological intelligence, as it integrates various senses and experiences.
The advantages of biological intelligence include complex problem-solving abilities and the capacity for empathy and social interaction. However, it also comes with limitations such as cognitive biases and slower processing speeds compared to artificial systems.
Artificial Intelligence
Artificial intelligence refers to machines designed to perform tasks typically requiring human intelligence. The most striking characteristic of AI is its efficiency in processing large volumes of data. AI systems can learn patterns and make predictions at a rapid pace.
A notable feature of artificial intelligence is its ability to improve through machine learning. This allows them to adapt based on new information without explicit programming. AI excels in structured environments where data is abundant, making it a popular choice for applications in industries ranging from healthcare to finance.
However, the reliance on data can be a limitation. AI systems may fail in ambiguous situations or lack the understanding of nuanced human emotions. It's these differences that laid the groundwork for further exploration into the intersections and disparities between biological and artificial intelligence.
Understanding Biological Intelligence
Biological intelligence represents the complex suite of cognitive abilities and functions inherent to living organisms, predominantly humans. Understanding biological intelligence is crucial to differentiate it from artificial systems. This section provides insight into the mechanisms and processes that govern human cognition, learning, and emotional responses. Recognizing these elements plays a vital role in evaluating how biological intelligence can inform the development of artificial intelligence.
Cognitive Processes in Humans
Cognitive processes are the mental actions or tasks that encompass thinking, understanding, learning, and remembering. These processes allow humans to interact with their environment effectively. Key cognitive processes include perception, attention, memory, reasoning, and problem-solving.
- Perception: The ability to interpret sensory information from the environment. It is the starting point for cognitive processing as it provides the data required for sound decision-making.
- Attention: The selective focus on certain stimuli while ignoring others. This is crucial for task completion and efficient communication.
- Memory: Encompasses short-term and long-term storage of information that aids learning and influences future decisions.
- Reasoning: The ability to draw conclusions from premises or known facts. It involves critical thinking, analysis, and evaluation of information.
- Problem-Solving: The cognitive process that navigates through obstacles to reach a desired outcome. This requires not just analytical skills but also creativity.
Understanding these processes helps researchers design AI systems that mimic human cognitive functions, leading to more advanced and human-like AI interactions.
Neuroscience of Learning and Memory
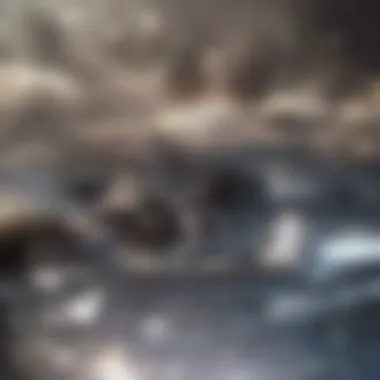
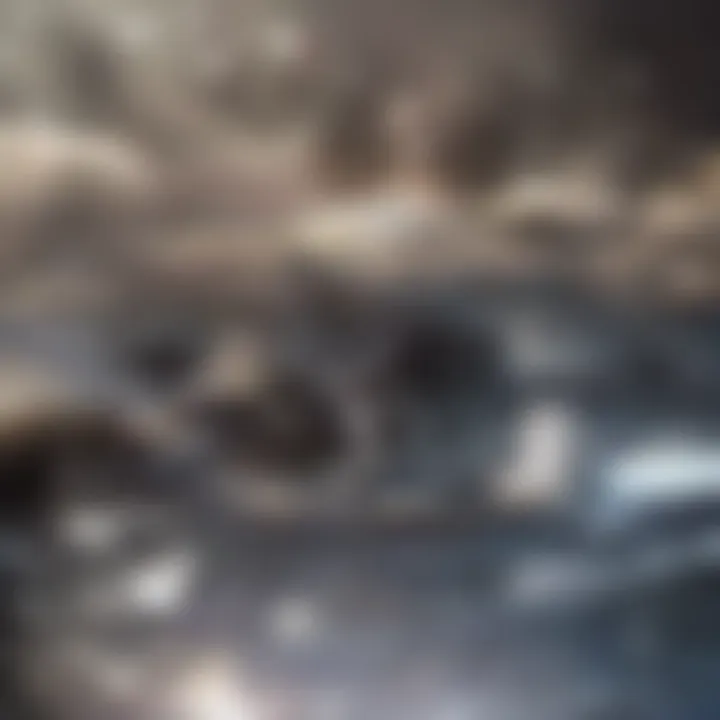
Neuroscience delves into the biological underpinnings of learning and memory, drawing connections between brain structure, function, and cognitive efficiency. Brain regions such as the hippocampus play a critical role in forming new memories, while the neocortex is vital for higher-order thinking and understanding.
- Neuroplasticity: This is the brain's capacity to reorganize itself by forming new neural connections. It is essential for learning throughout life since it allows adaptation in response to new experiences. Neuroplasticity highlights a unique feature of biological intelligence that remains a challenge for AI to replicate.
- Memory Systems: Human memory is divided into various systems, including declarative memory (facts and events) and procedural memory (skills and tasks). Each system operates through different mechanisms that are intricately tied to specific brain areas.
The exploration of these systems assists AI developers in creating algorithms that may emulate aspects of human learning and memory. By reflecting on how biological systems operate, artificial systems can become more sophisticated and efficient.
Emotions and Decision Making
Emotions significantly influence human decision-making processes. Understanding how emotions interact with cognition is vital in the discourse of intelligence. Emotions can act as motivators and modifiers of behaviors, affecting choices in complex situations.
- Emotional Intelligence: The ability to understand one’s own emotions, as well as the emotions of others, creates a framework for interpersonal interactions and effective communication. Emotional intelligence also plays a significant role in organizational decisions and conflict resolution.
- Impact on Decisions: Emotional reactions can lead to rapid choices based on gut feelings. Research indicates that emotions enhance or impair judgment, depending on the context of the situation. It is crucial for AI systems to consider emotional responses to improve user interaction.
"Emotions are embedded in human decision-making and are essential for rational thought. Understanding this interplay is fundamental for creating empathetic AI systems."
In summary, grasping the intricacies of biological intelligence through cognitive processes, the neuroscience of learning, and emotional understanding provides valuable insights. These elements not only differentiate biological intelligence from its artificial counterpart but also lay the groundwork for advancing AI technologies. This intersection of understanding will illuminate future developments in both fields.
Exploring Artificial Intelligence
Artificial Intelligence (AI) has become an integral part of contemporary technology. It encompasses various techniques and systems designed to replicate human cognitive functions. Understanding AI offers significant insights into its capabilities, limitations, and implications for society. This section elaborates on the characteristics, development, and types of AI systems that form the foundation of this technology.
Defining Artificial Intelligence
Artificial Intelligence is defined as the capability of a machine to imitate intelligent human behavior. This definition encompasses a range of cognitive functions, including problem-solving, learning, and reasoning. AI can be classified into two broad categories: narrow AI, which is designed for a specific task, and general AI, which aims to perform any intellectual task a human being can do. The prevalence of narrow AI in applications like image recognition and voice assistants illustrates its current dominance in the tech landscape.
Historical Development of AI
The journey of AI began in the mid-20th century, marked by Alan Turing's fundamental question: "Can machines think?" This query spurred early research into machine learning and logic. Over decades, AI experienced periods of optimism and setbacks known as AI winters, where progress slowed due to unrealistic expectations. However, the resurgence of interest in recent years can be attributed to significant advancements in computing power, the availability of big data, and improved algorithms. These factors have elevated AI from theoretical constructs to practical applications that impact various sectors today.
Types of AI Systems
AI systems can be categorized based on their functionality and application.
Machine Learning
Machine Learning is a cornerstone of AI development. It involves algorithms that allow computers to learn from and make predictions based on data. The key characteristic of machine learning is its ability to improve performance with experience. This adaptability makes it a popular choice in sectors such as finance and healthcare. One unique feature of machine learning is its vast range of algorithms, from supervised to unsupervised learning. However, it also carries the disadvantage of requiring large datasets, which are not always readily available.
Natural Language Processing
Natural Language Processing (NLP) focuses on the interaction between computers and humans through natural language. This field enables machines to understand, interpret, and generate human language. The key characteristic of NLP is its versatility in applications, from chatbots to translation services. NLP is beneficial for user engagement and accessibility, as it allows for more natural interactions with technology. A unique feature of NLP is its reliance on context, which can sometimes lead to misunderstandings in complex conversations.
Computer Vision
Computer Vision enables machines to interpret and make decisions based on visual data. This branch of AI has applications in diverse fields, such as security, healthcare, and automotive industries. A key characteristic of computer vision is its ability to analyze images and videos in real-time. This feature is particularly advantageous in surveillance and autonomous vehicles. However, one limitation is the susceptibility to inaccuracies in conditions like low lighting or extreme angles, which can affect system reliability.
Understanding the various facets of artificial intelligence helps to contextualize its impact on society and the future of intelligence in human and machine collaboration.
Key Differences Between Biological and Artificial Intelligence
Understanding the key differences between biological and artificial intelligence is fundamental for discerning how these two constructs operate, evolve, and interact within our world. In this section, we will explore specific mechanisms, adaptability, learning styles, as well as the limitations and challenges each form of intelligence faces. Highlighting these differences not only enriches our knowledge but is crucial in guiding future research, ethical considerations, and technological developments.
Mechanisms of Operation
Biological intelligence operates through a complex network of neurons and synapses that form the brain's architecture. This biological network is responsible for processing sensory information, coordinating motor functions, and enabling cognitive tasks such as reasoning and problem-solving. The interaction between different neurons allows for a rich tapestry of thought and experience, paving the way for creativity and emotional depth.
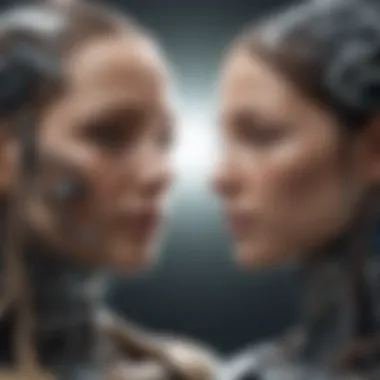
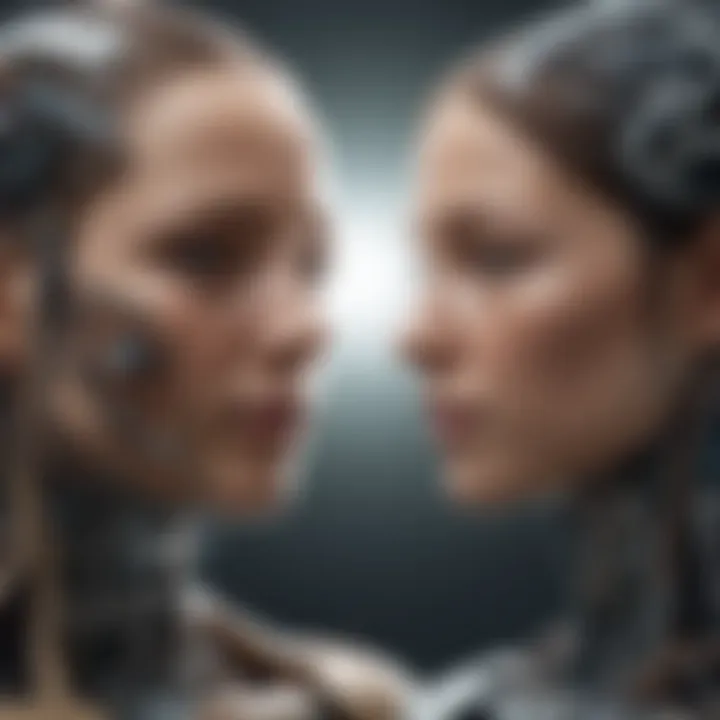
In contrast, artificial intelligence relies on algorithms and mathematical models to simulate behaviors mimicking human cognition. One common operational mechanism in AI is machine learning, which uses data to improve performance over time. This involves training models on massive datasets, allowing them to recognize patterns, make predictions, and automate tasks. An example is neural networks, which are designed to process information in a way analogous to human brains. However, the functioning of AI is fundamentally different because it lacks consciousness or subjective experiences typical of biological intelligence.
Adaptability and Learning Styles
Adaptability is another critical difference between biological and artificial intelligence. Biological organisms adapt to their environments through a combination of instinct and learned behavior. Human beings, for example, grow and learn from their experiences, allowing them to modify responses based on past outcomes. This dynamic process is not only shaped by external environments but also influenced by emotions, social interactions, and cultural contexts.
Artificial intelligence systems typically adapt through pre-defined learning algorithms. They can optimize performance by analyzing data and refining their outputs without the emotional underpinnings present in biological intelligence. For instance, a system using reinforcement learning adjusts its behavior based on received rewards and punishments. However, its adaptability is limited to specific tasks and contexts defined during its training phase. In essence, while AI can excel in narrow domains, it often struggles with transferring knowledge to unforeseen situations, unlike humans who can apply learning across diverse scenarios.
Limitations and Challenges
Both biological and artificial intelligence face unique limitations and challenges. Biological systems, although remarkably adept at processing information, are constrained by physical and cognitive boundaries. For instance, human memory is fallible, and cognitive biases can distort decision-making processes. Additionally, biological intelligence can suffer from fatigue, emotional disturbances, and various health conditions that impair performance.
On the other hand, artificial intelligence systems face challenges rooted in their design. Issues such as algorithmic bias, lack of transparency in decision-making, and dependency on quality data can lead to flawed outputs. Additionally, AI lacks the ability to understand context as deeply as humans do, which can cause errors in judgment. The reliance on large datasets for training can also lead to ethical concerns regarding privacy and your exploitation of sensitive information.
In summary, the distinctions between biological and artificial intelligence are marked by their underlying mechanisms, adaptability, and inherent limitations. Recognizing these differences is vital for advancing our understanding of both forms of intelligence and how they can be integrated to foster future innovations.
Intersections of Biological and Artificial Intelligence
The intersections between biological intelligence and artificial intelligence represent a critical area of exploration in modern technology and science. Understanding these connections helps to unveil how human-like thinking can be mirrored and possibly enhanced through technology. This section elaborates on specific elements such as neuro-inspired AI technologies, collaborative intelligence systems, and applications in technology and medicine.
Neuro-inspired AI Technologies
Neuro-inspired AI technologies take cues from the biological processes that underpin human cognition. The architecture of neural networks often reflects the principles of how the human brain operates, though simplistically. The idea behind these technologies is to develop systems that can process information more like a human would, effectively mimicking natural cognitive functions. This approach has led to tremendous advancements in areas such as deep learning and machine learning.
For example, convolutional neural networks are designed to analyze visual data similarly to how humans perceive images. The architecture utilizes layers of processing units that sequentially filter input data, enhancing the system’s ability to recognize patterns. The derivation of such technologies from biological systems makes them more efficient in specific tasks, particularly those involving complex data.
Moreover, research in this field often focuses on increasing the efficiency of these AI systems. By exploring the mechanisms of the brain’s learning processes, AI developers can design more responsive and adaptive models.
Collaborative Intelligence Systems
Collaborative intelligence systems are emerging as a promising paradigm that combines the strengths of both biological and artificial intelligence. In these systems, human intelligence collaborates with AI in various contexts, creating a symbiotic relationship that enhances overall effectiveness.
This approach enables AI to assist humans in making complex decisions, while human insights contribute emotional and ethical dimensions that AI alone cannot grasp. For instance, in environments such as healthcare, collaborative systems use AI algorithms to analyze vast datasets, while humans provide the contextual and ethical considerations when interpreting results or making decisions.
Such collaborations not only improve decision-making processes but also emphasize the importance of ethical frameworks in AI development. The role of humans in guiding AI operations becomes essential because it adds a layer of accountability and moral judgment that AI lacks.
Applications in Technology and Medicine
The applications of these intersections unfold significantly in the realms of technology and medicine. In technology, companies harness neuro-inspired designs to create more sophisticated algorithms and software that can learn, adapt, and automate repetitive tasks with minimal human intervention. Technologies such as facial recognition and voice assistants are products of blending both forms of intelligence, yielding platforms that enhance user experience.
In medicine, there is even a more profound impact. AI algorithms analyze medical data, assist in diagnostics, and predict patient outcomes, all while learning from previous cases. For example, IBM Watson Health utilizes AI to support healthcare professionals in their diagnostic processes. By efficiently analyzing vast amounts of data, it aids in delivering personalized treatment recommendations.
"The integration of biological and artificial intelligence is not merely about automation; it’s about augmenting human capabilities and improving decision-making processes."
These innovations underscore how the integration of biological and artificial intelligence has the potential to redefine industries, improving outcomes while enhancing our understanding of both human intelligence and machine learning. As we progress, the cooperation between these forms of intelligence will likely continue to evolve, opening new avenues for exploration and application.
Ethical Considerations in AI Development
The rise of artificial intelligence (AI) introduces significant ethical considerations that are crucial for its sustainable growth and acceptance in society. As AI technologies become integrated into daily life, understanding the ethical implications becomes imperative for developers, businesses, and users alike. This section will explore vital elements that must be taken into account when developing AI systems, ensuring they align with human values and societal norms.
Moral Implications of AI
The moral implications of AI encompass a variety of concerns regarding the impact these systems have on human behavior and society at large. One prominent aspect is the potential for bias in AI algorithms. Machine learning systems learn from data, which can sometimes reflect existing biases in society. If these biases are not addressed, AI systems may perpetuate and even exacerbate discrimination in areas such as hiring, law enforcement, and lending.
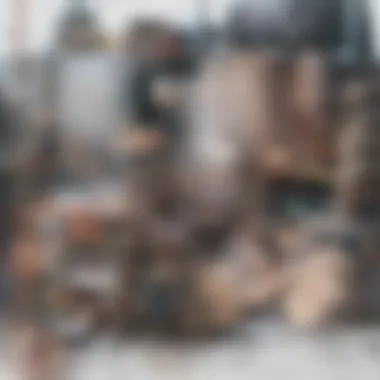
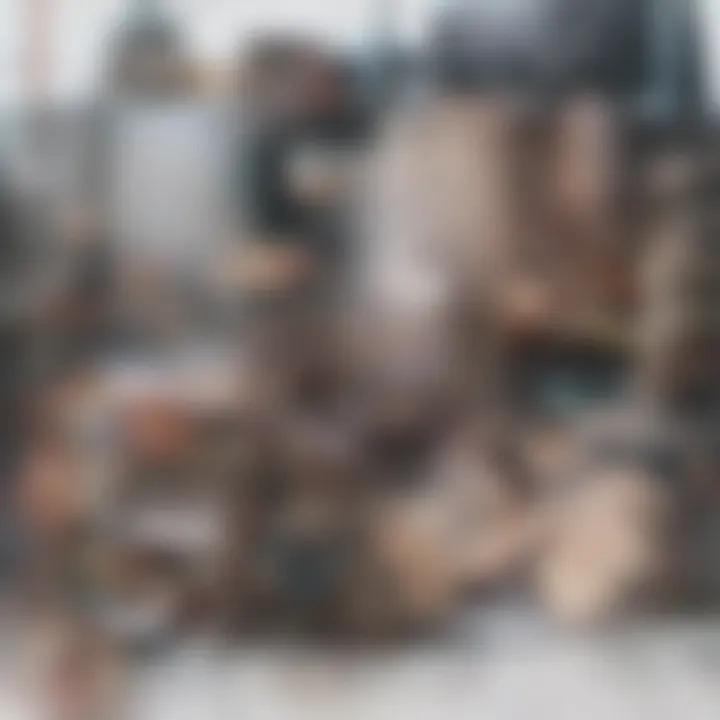
Additionally, there is a pressing concern about accountability. Who is responsible when an AI system makes a mistake or causes harm? The lack of clear responsibility can lead to significant ethical dilemmas, particularly in critical applications like autonomous vehicles or healthcare.
- Key considerations include:
- Ensuring fairness in algorithmic decision-making.
- Developing transparent AI systems where decisions can be understood and scrutinized.
- Establishing accountability mechanisms to address error or misjudgment by AI.
These moral considerations highlight the need for ethical frameworks that guide AI development and deployment. Such frameworks should not only focus on technological capability but also consider how AI aligns with ethical principles.
Privacy and Surveillance Issues
The integration of AI in surveillance technology has raised substantial privacy concerns. As organizations deploy AI for monitoring and data collection, the fine line between security and invasive surveillance often blurs. The use of facial recognition technology by governments and corporations illustrates this issue well.
There are several critical points to examine:
- Data Collection: AI systems require vast amounts of data to function effectively. This often means collecting personal information, potentially without consent or understanding of how it will be used.
- Surveillance Ethics: Continuous monitoring can lead to an erosion of personal privacy, resulting in a chilling effect on free expression and individual behavior.
- Compliance with Regulations: There is an ongoing debate about how to align AI surveillance systems with established privacy laws, such as the General Data Protection Regulation (GDPR) in Europe.
"The ethical application of AI in surveillance must not compromise individual privacy rights."
Ultimately, the challenge lies in striking a balance between leveraging AI for safety and maintaining the fundamental right to privacy. It is crucial that stakeholders engage in open dialogue and develop policies that protect individuals while harnessing the benefits of AI technology.
Future Directions in Intelligence Research
In the ongoing exploration of intelligence, the future directions of research emerge as crucial pathways leading to deeper understanding and innovative applications. This section examines the evolving landscape of intelligence, weaving together insights from both biological and artificial intelligence domains. The focus is not merely on technical advancements but also on broader implications for society and ethical considerations.
Fusion of Biological and Artificial Intelligence
The fusion of biological and artificial intelligence brings together the strengths of both realms. Biological systems, with their advanced cognitive abilities, provide inspiration for constructing more sophisticated artificial systems. Conversely, artificial intelligence tools can enhance our understanding of human brain functions by simulating cognitive processes. This intertwining is evident in neuro-inspired AI technologies, such as neuromorphic computing, which mimics the architecture and functioning of biological neural systems.
Key points include:
- Enhanced Cognitive Models: By studying how humans learn and process information, AI researchers can design systems that more closely align with natural cognitive processes.
- Augmented Human Decisions: Tools like decision support systems can assist humans in complex decision-making, benefiting fields like healthcare and finance.
- Collaborative Learning: Merging human intuition with machine precision can lead to breakthroughs in various applications, enhancing problem-solving capabilities.
The synthesis of biological insights and artificial intelligence could lead to systems that not only excel at specific tasks but also possess a more general understanding of intelligence itself.
Implications for Society
The implications of merging biological and artificial intelligence are profound. As we integrate these technologies into everyday life, there are several societal aspects to consider. First, educational frameworks may need to adapt, incorporating these new tools to better prepare students. Additionally, workplaces might evolve to include hybrid teams made of humans and intelligent systems, requiring new skills and approaches.
Key considerations involve:
- Job Displacement: As AI becomes more capable, there might be significant shifts in job markets. Understanding these changes is essential for workforce planning and policy development.
- Ethical Governance: Balancing innovation with ethical oversight becomes crucial. Policymakers must ensure that artificial systems are designed and deployed in ways that respect human rights and promote equity.
- Social Interaction: The way humans interact with technology is under transformation. As AI systems increasingly support daily activities, issues around dependency and trust may arise.
The future of intelligence research sits at the intersection of progress and responsibility. As explorations into these fused intelligences continue, society must engage thoughtfully with the potential outcomes, ensuring that advancements serve the greater good and foster sustainable development.
End
The conclusion of this article serves as a critical summation of the discussions surrounding biological intelligence and artificial intelligence. This section not only recaps the core elements presented throughout the article but also highlights the significance of their coexistence in the modern world.
Recap of Core Concepts
Throughout the article, we explored various facets of both biological and artificial intelligence. Key themes include:
- Defining Intelligence: We outlined what constitutes intelligence in both biological and artificial contexts.
- Cognitive Processes: Understanding how humans process information and memories informed our perspective on biological intelligence.
- AI Development: An overview of the historical context within which artificial intelligence has grown.
- Differences and Intersections: We analyzed the operational mechanisms, adaptability, and limitations of each form of intelligence.
- Ethics and Future Directions: The discussion included ethical concerns surrounding AI and its implications for society.
This synthesis of concepts emphasizes the complexity of intelligence, whether biological or artificial. Each type has unique strengths and weaknesses, and recognizing these can inform future research and application.
Final Thoughts on the Coexistence of Intelligence
As we look toward the future, the intersection of biological and artificial intelligence presents both opportunities and challenges. The integration of AI systems into various domains amplifies human capabilities, yet it also raises questions about ethical considerations and societal impacts.