AI Shape Recognition: Concepts and Future Impacts
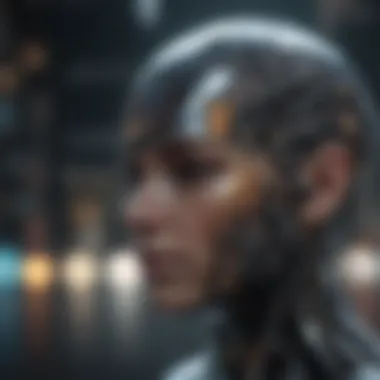
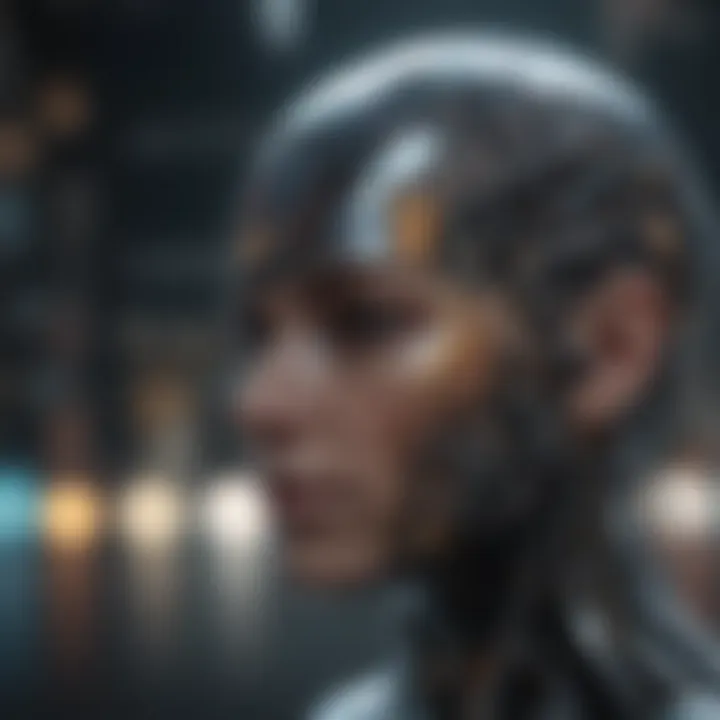
Intro
In the rapidly evolving landscape of artificial intelligence, shape recognition stands out as a crucial element that enhances our understanding of visual data. At its core, AI shape recognition involves the ability of machines to identify and analyze various shapes in different contexts, providing insights that can be applied across diverse fields.
The significance of this technology isn't just academic; its applications are far-reaching, touching industries from healthcare to autonomous vehicles. As researchers and engineers push the boundaries of what machines can perceive, a host of questions regarding the implications and challenges of these technologies arise, setting the stage for a deeper exploration of AI shape recognition.
To effectively navigate the complexities of this field, we shall first delve into the key research findings that outline recent discoveries and innovations. This groundwork will lay the foundation for understanding advanced theories, paving the way for discussions on practical applications and future challenges, all while ensuring the content remains accessible for a broad audience.
Prolusion to AI Shape Recognition
Artificial Intelligence (AI) shape recognition is a fascinating domain that melds computer science, machine learning, and visual perception. This area holds a significant place in today's data-driven world, where the ability of machines to interpret shapes can lead to groundbreaking applications across numerous sectors. The growing reliance on digital interfaces necessitates an understanding of how AI systems dissect visual cues, which plays a crucial role in enhancing user experiences and operational efficiency.
As we navigate through this section, we will not only define AI shape recognition but also delve into its evolution. Understanding these fundamental concepts is pivotal for fostering a comprehensive grasp of its broader implications on society and industry. The spotlight will be on the methodologies by which machines gain insights from visual data, particularly the effectiveness and limitations that accompany these processes.
Defining AI Shape Recognition
AI shape recognition refers to the capability of algorithms and machines to identify, analyze, and categorize shapes within digital images or physical environments. This process involves the extraction of relevant features from the shapes, facilitating classification tasks. It’s a pivotal technology underpinning critical applications in areas like autonomous driving, healthcare imaging, and robotics.
The essence of AI shape recognition lies in its ability to transform static visual input into actionable insights. For instance, when a self-driving car detects a pedestrian, it must accurately interpret the shape of the person against the backdrop of a dynamic and complex environment. This requires sophisticated models that can handle and analyze context, lighting, and various distortions. As technology barrels ahead, the accuracy and reliability of shape recognition systems enhance, marking significant milestones towards advanced AI capabilities.
Historical Context and Development
The roots of AI shape recognition can be traced back to the early days of computer vision and pattern recognition in the 1960s and 1970s. Initially, researchers grappled with the challenges posed by limited computational power and rudimentary algorithms. Yet, despite these early hurdles, the field saw significant advancements as technology progressed.
In the 1980s and 1990s, researchers began to explore more complex algorithms, drawing inspiration from biological systems and cognitive processes. These early efforts set the stage for modern approaches that benefit from machine learning and deep learning advancements. The integration of neural networks, particularly convolutional neural networks, marked a turning point that dramatically improved the effectiveness and efficiency of shape recognition tasks.
The development of more sophisticated training datasets and the exploration of various shape representation models have further propelled this field. Now, algorithms can learn from vast amounts of visual data, continuously refining their capabilities to recognize and classify shapes across diverse scenarios.
As we reflect on this evolution, it’s clear that AI shape recognition is not just a technological novelty; it serves as a cornerstone for many applications that have the potential to reshape industries and redefine human-machine interactions.
Fundamentals of Shape Recognition
The exploration of shape recognition lies at the heart of understanding how machines interpret visual data. This section examines the foundational elements that shape recognition systems utilize. Grasping these fundamentals is essential for anyone interested in building, applying, or researching this technology. Here’s a closer look at the landscape of shape representation and the algorithms that have brought it to life.
Shape Representation Models
Geometric Models
Geometric models focus on the mathematical properties of shapes. These models are predominantly concerned with defining figures based on their geometric attributes. For instance, a geometric model might describe a circle based on its radius or a rectangle based on its length and width. The key characteristic of geometric models is their precision in defining shapes through measurable parameters. This makes them a popular choice in applications where exact shape recognition is critical such as CAD systems or engineering simulations.
One unique feature of geometric models is their simplicity; they can easily represent simple and regular shapes. However, when it comes to more complex or irregular shapes, these models can become limited. While they can handle simple curves and lines well, their effectiveness wanes significantly with more intricate patterns. Thus, while they excel in many areas, they may not always provide a comprehensive understanding of shapes encountered in real-world scenarios.
Topological Models
Topological models shift focus from the specific measurements of shapes to their inherent topological properties. This means they analyze how shapes are connected, regardless of their actual sizes or distances. The most intriguing aspect of these models is their ability to capture the characteristics of shapes that remain unchanged even when they undergo deformations. This notable characteristic makes topological models exceptionally useful in contexts where shape modification happens, like in biology or material science.
One distinct advantage of topological models is their robustness against noise and distortions in data, which is often a challenge in practical applications. However, a disadvantage can be their abstract nature, which might not be as intuitive in certain contexts, particularly when precise measurements or detailed analyses are required.
Parametric and Non-Parametric Models
Parametric and non-parametric models provide two different approaches to shape representation. Parametric models use a fixed set of parameters to describe shapes and exhibit a defined structure. For example, a parametric model could define a shape based on its control points and a set of equations. This core advantage lies in their efficiency and reduced computational cost in specific scenarios.
On the other hand, non-parametric models do not depend on parameters and learn from data distribution. They’re often considered more flexible, adapting readily to the nuances of various shapes without being confined by a defined structure. Their primary advantage is their capability to represent a larger variety of shapes with unpredictability. However, they may require more data and robust learning mechanisms, highlighting a trade-off between flexibility and efficiency.
Algorithms for Shape Recognition
Algorithms for shape recognition serve as the backbone for interpreting visual data. They enable machines to learn from shapes and discern patterns that are essential in various industries. Understanding the types of algorithms available is critical for selecting the right approach for specific applications.
Feature-Based Techniques
Feature-based techniques extract key attributes from shapes, allowing algorithms to recognize and classify these shapes based on their distinctive features. The predominant characteristic of these techniques is their reliance on features such as edges, corners, and gradients. These features serve as the building blocks for identifying shapes and their relationships. This makes them a favorable choice in situations where the identification of specific traits is paramount, such as in medical imaging or facial recognition technology.
However, while their emphasis on features can improve accuracy, they may struggle with complex shapes that lack clear distinguishing traits, generating a limitation that needs to be addressed in advanced applications.
Template Matching
Template matching is another widely used algorithm that involves comparing an input shape with a pre-defined template. The vital strength of this method lies in its simple implementation and effectiveness when dealing with known shapes. Its notable characteristic is the ability to provide high accuracy with clear and defined patterns, making it advantageous in applications such as character recognition and industrial automation.
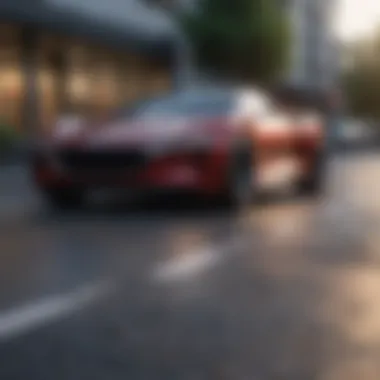
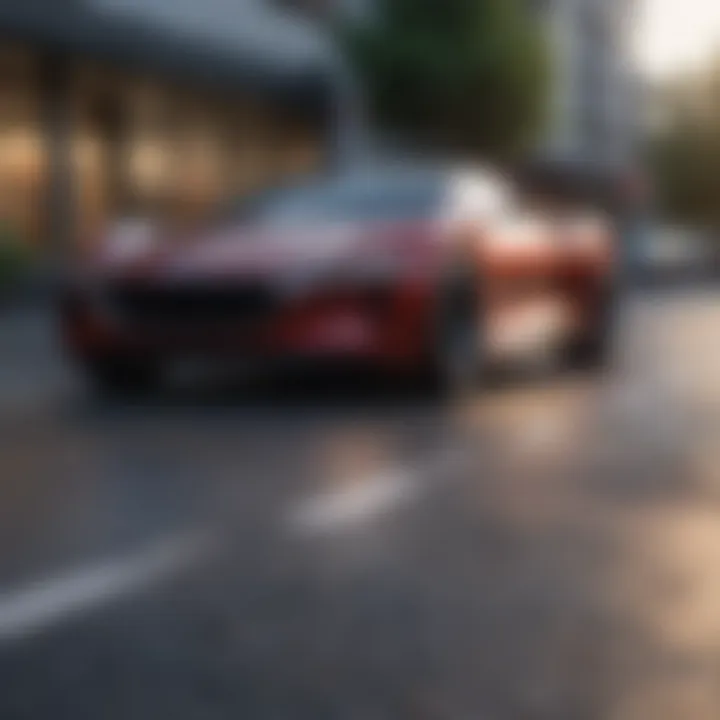
Nonetheless, one limitation of template matching is its reliance on an exhaustive number of templates to cover varied appearances of shapes, which can quickly become modal intensive. Moreover, it may face challenges in noisy environments where shapes become altereed due to distortions.
Deep Learning Approaches
Deep learning approaches utilize neural networks to recognize shapes by discerning complex patterns within large sets of images. The key characteristic of these approaches is their ability to automatically extract features from raw data, eliminating manual feature extraction. This makes them incredibly versatile and beneficial for many modern applications such as self-driving cars or facial emotion recognition systems.
While deep learning techniques offer extensive potential in automated shape recognition, they also pose challenges, primarily requiring vast amounts of data for training. In addition, computational demands can be high, leading to efficiency concerns in scenarios where resources are limited.
"Understanding the fundamentals of shape recognition equips you with essential insights to navigate the complexities of how machines interpret visual information."
In summary, the fundamentals of shape recognition encompass critical elements like shape representation and algorithms, providing a rich context for understanding how this technology functions and is applied. Grasping these concepts sets the stage for delving deeper into the technological advances and applications of AI shape recognition.
Technological Advances in Shape Recognition
In the realm of artificial intelligence, the topic of technological advances in shape recognition is crucial. It serves as the backbone for interpreting and processing visual information, which is foundational for various applications in healthcare, automation, and beyond. The incorporation of novel technologies and methodologies not only enhances precision but also broadens the scope of what can be achieved. From identifying patterns in medical imagery to navigating complex environments autonomously, the advancements in AI shape recognition provide significant benefits across different sectors.
Machine Learning Innovations
Supervised Learning
Supervised learning is a cornerstone of machine learning that involves training a model on a labeled dataset. Here, each input data point corresponds to an output, allowing the machine to learn from its errors and make better predictions over time. In the context of shape recognition, this approach excels because it typically yields high accuracy when sufficient labeled data is available.
Key characteristic: One of the most notable features of supervised learning is its dependency on labeled data. While this can be a double-edged sword, it usually results in models that are well-tuned to recognize specific shapes or features accurately.
Unique feature: The prime advantage of supervised learning is its ability to generalize from the training data to unseen shapes, ensuring effective applications in real-world scenarios. However, one drawback is that it can be resource-intensive; collecting and labeling data is no small feat.
Unsupervised Learning
In contrast, unsupervised learning deals with unlabeled data, seeking to find hidden patterns or groupings without external guidance. This learning style is particularly useful when labeled data is scarce or expensive to obtain, making it a significant approach in the domain of shape recognition.
Key characteristic: The essence of unsupervised learning lies in its capability to deduce the inherent structure of data without pre-existing labels. This can lead to discovering new categories or traits in shapes that were previously overlooked.
Unique feature: One major advantage is its flexibility; researchers can explore the data more freely without the constraints of labels. On the downside, it may take longer to fully comprehend the results since interpretation depends heavily on the algorithms employed.
Reinforcement Learning
Reinforcement learning takes a different approach, focusing on the idea of learning through trial and error. Here, an agent learns to achieve specific goals by interacting with its environment and receiving feedback in the form of rewards or penalties.
Key characteristic: The standout feature of reinforcement learning is its ability to adapt dynamically; the agent improves its performance through repeated exposure, making it particularly beneficial in complex environments like real-time shape recognition tasks.
Unique feature: The unique aspect of this method is its emphasis on decision-making processes, which empowers systems to make optimal choices in uncertain situations. Yet, it can also be computationally expensive and time-consuming due to the need for extensive simulation and testing.
Neural Networks and Shape Recognition
Convolutional Neural Networks (CNNs)
Convolutional neural networks, or CNNs, have revolutionized the field of shape recognition. They are specifically designed to process pixel data and are highly effective at capturing spatial hierarchies.
Key characteristic: The defining feature of CNNs is their ability to automatically recognize spatial hierarchies in images. By employing convolutional layers, these networks can detect edges, shapes, and more complex patterns at varying levels of abstraction.
Unique feature: An advantage of CNNs is their efficiency in handling large datasets; they tend to require less human intervention than traditional methods. A potential downside, though, is their complexity, which can make it hard to interpret results directly, posing challenges in critical applications like healthcare.
Graph Neural Networks
Graph neural networks (GNNs) represent a newer frontier in shape recognition. By focusing on the relationships between data points rather than their individual characteristics, GNNs allow for a more nuanced understanding of shapes and their components.
Key characteristic: The pivotal feature of GNNs is their capacity to model complex data structures that resemble graphs, making them ideal for recognizing shapes that are not easily captured in a grid format, such as objects with irregular features.
Unique feature: GNNs excel in tasks with relational data, providing insights that traditional approaches might miss. However, they can be computationally intensive and require specialized knowledge to implement effectively.
Transformers in Shape Recognition
Transformers have emerged as a powerful tool in AI shape recognition due to their inherent ability to process sequences of data efficiently. Initially developed for natural language processing, they have also found applications in visual recognition tasks.
Key characteristic: The unique aspect of transformers is their use of self-attention mechanisms, which allow them to weigh the significance of different parts of the input data, identifying relevant features even in complex shapes.
Unique feature: Transformer's strength lies in their versatility; they can handle various types of data inputs, which makes them adaptable. However, one downside might be the need for substantial training data to achieve optimal performance, which can limit their effectiveness in low-resource settings.
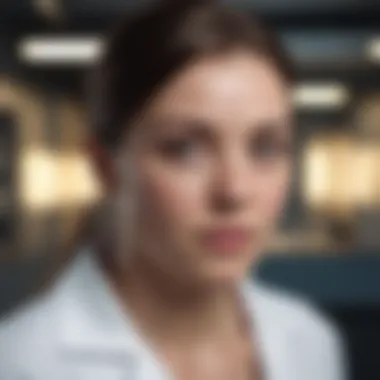
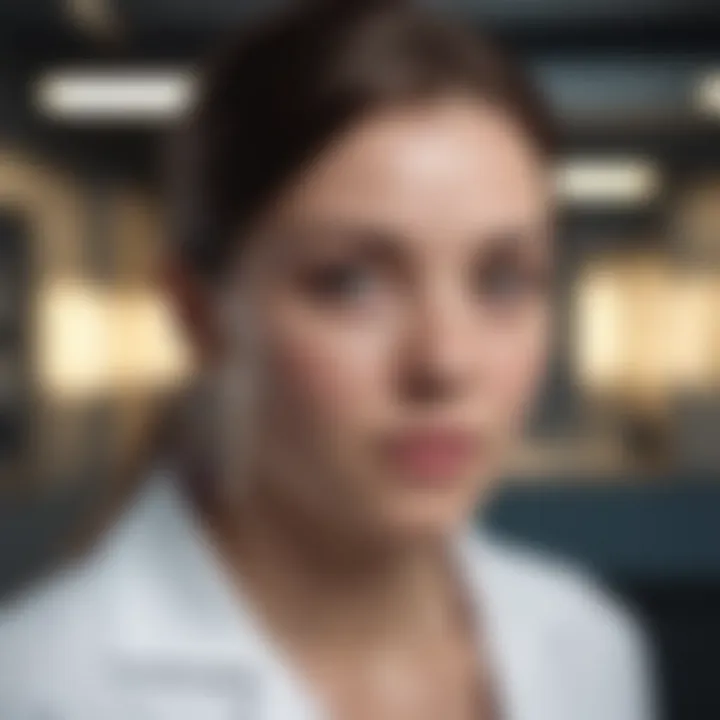
Integration of AI with Computer Vision
The integration of AI with computer vision marks a significant step forward in the capabilities of shape recognition systems. This amalgamation enables machines to interpret visual data more accurately and efficiently.
The two fields converge harmoniously, allowing innovations like real-time video analysis, augmented reality, and semi-automated decision-making in complex environments. The collaboration results in a multipronged approach, improving object detection and enhancing automated systems.
To summarize, the fusion of AI and computer vision not only elevates the potential of shape recognition but also opens doors to novel applications across different sectors, providing a pathway for continual advancement in technology.
Applications of AI Shape Recognition
The realm of AI shape recognition finds itself in a myriad of applications that span across different industries, tickling the very essence of technology and enhancing everyday experiences. As machines become increasingly adept at understanding and analyzing shapes, their relevance is felt in areas like healthcare, autonomous vehicles, and robotics. These applications not only underscore the potential of shape recognition but also pose a fascinating glimpse into a future captivated by intelligent machines.
Healthcare and Medical Imaging
Early Detection of Diseases
The role of AI shape recognition in early disease detection is nothing short of revolutionary. Its ability to analyze medical images and pinpoint anomalies allows for faster diagnoses, which can be pivotal in treating conditions like cancer. With algorithms designed to recognize unusual shapes in scans, such tools become invaluable to radiologists. The key to this technique lies in its precision; it can sift through massive datasets, identifying even the slightest deviations that might escape the naked eye. However, while it holds great promise, reliance on AI raises concerns around over-diagnosis and the need for human oversight in clinical settings.
Surgical Assistance
In the operating room, AI shape recognition takes center stage as a supporting hand for surgeons. Systems equipped with this technology can project the shapes of organs and tissues onto the surgical field, providing real-time guidance. The standout feature here is the enhancement of accuracy; surgeries become less invasive and more effective. Nonetheless, this dependence on technology might lead to hesitation among some practitioners, who may fear the loss of manual skills as they become reliant on AI systems.
Patient Monitoring Systems
AI-enabled patient monitoring systems harness shape recognition to track patient movements and vital signs within healthcare facilities. These systems provide continuous feedback, allowing for timely interventions when abnormalities are detected. Such monitoring can lead to improved patient outcomes and quicker response times in emergency situations. However, integrating this technology effectively into clinical workflows requires careful planning; there are concerns regarding data overload and the need for trained personnel to interpret the results effectively.
Autonomous Vehicles
Obstacle Detection
In the chaotic world of self-driving cars, obstacle detection powered by AI shape recognition is essential. By analyzing the shapes of surrounding objects, autonomous systems can make split-second decisions to avoid collisions. The brilliance of this method lies in its adaptability; it learns from various scenarios, enhancing safety on the roads. However, the complexity of real-world environments presents a challenge; unexpected shapes can confuse algorithms, leading to potential risks.
Navigation and Mapping
Navigation systems that leverage AI shape recognition create precise maps for self-driving vehicles, which can enhance their ability to function in diverse landscapes. These systems can interpret shapes of roads, buildings, and terrains, which help vehicles navigate smoothly. An exceptional aspect here is the ability to adapt in real-time, adjusting routes according to changing conditions. Yet, this reliance on constant data input raises concerns around privacy and the implications of data collection on individuals.
Traffic Analysis
Traffic analysis through AI shape recognition serves as a tool to manage urban flow and design smarter cities. By understanding the shapes and movements of vehicles and pedestrians, cities can optimize traffic signals, reduce congestion, and enhance safety measures. The attractive feature of this approach is its potential to create real-time responsive systems. But, challenges arise when considering the infrastructure necessary for such systems, and the potential for data misuse remains a pressing worry.
Robotics and Automation
Industrial Automation
AI shape recognition is making its mark in industrial automation, where robotic systems use it to identify materials on production lines efficiently. The critical feature is speed; these systems can quickly distinguish between different parts, ensuring production efficiency. This advancement can lead to lower operational costs and higher productivity. However, the need for ongoing maintenance and training of staff to manage these intelligent systems cannot be overlooked, as failure to do so can lead to significant downtime.
Service Robots
Service robots, particularly those used in hospitality and retail, utilize AI shape recognition to navigate environments and interact with customers. The standout benefit is their ability to streamline operations and enhance user experience. For instance, robots can identify shapes of inventory on shelves, facilitating restocking processes. Yet, there's a balancing act between technological convenience and the potential displacement of human workers, which must be managed carefully to avoid backlash.
Precision Agriculture
In agriculture, AI shape recognition introduces precision techniques that optimize resource use and boost yields. By analyzing shapes of crops, these systems can guide farmers on when and where to apply water, fertilizers, or pesticides. The major characteristic here is data-driven decision-making, minimizing waste while maximizing output. Nevertheless, farmers may encounter hurdles related to the initial investment costs and the need for ongoing education to fully utilize these advanced systems.
The multifaceted applications of AI shape recognition illustrate not just the flexibility of the technology, but also its profound implications across various sectors.
Challenges in AI Shape Recognition
In the intricate tapestry of AI shape recognition, the challenges underscore its significance and the pivotal role it plays across various industries. Addressing these hurdles is crucial, not only for further advancements in the field but also for the practical deployment of these technologies. By understanding the problems that pervade this domain, we can better appreciate the necessity for ongoing research and innovation.
Data Quality and Quantity Issues
At the core of AI shape recognition lies the quality and quantity of data. Without robust and reliable data, even the most sophisticated algorithms can sputter rather than soar. Poor-quality data can arise from several sources, such as inadequate labeling, noise within data sets, or outdated information. When machine learning models are trained on flawed data, they can develop biases, leading to misclassifications and ineffective results.
The necessity for large datasets is another element that complicates shape recognition tasks. In real-world scenarios, acquiring a vast amount of diverse, accurately labeled data is often painstakingly difficult. For instance, training a model to recognize various human organs from medical images requires thousands, if not millions, of annotated images across many variations and conditions. More often than not, researchers resort to data augmentation techniques to artificially expand the dataset.
"Clean, abundant data is the lifeblood of effective AI. Without it, we’re just throwing darts in the dark."
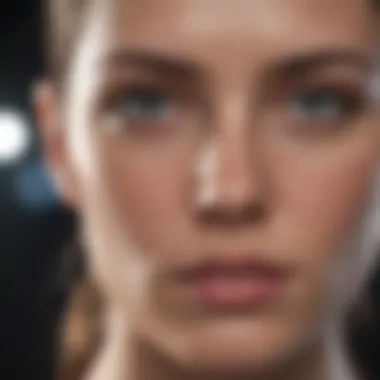
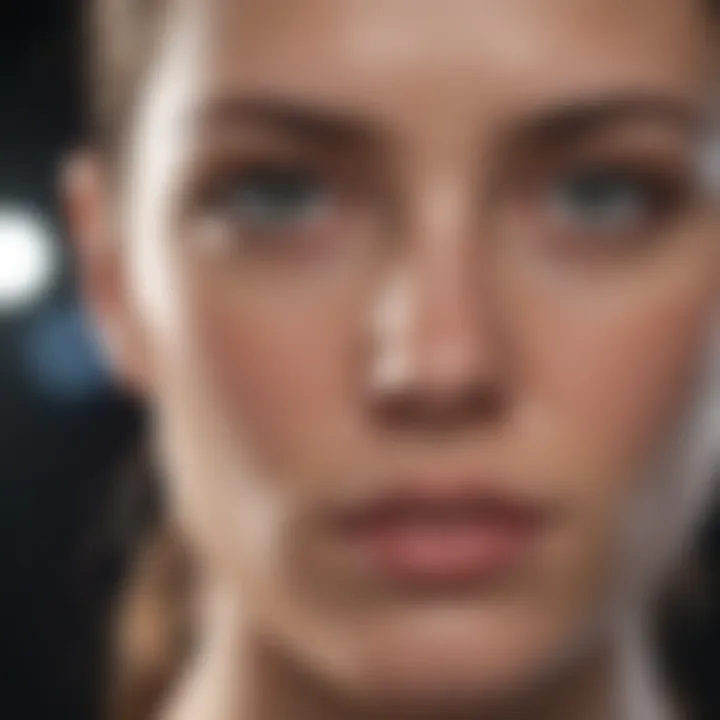
Complexity of Real-World Shapes
Moving beyond data issues, we must face the inherent complexity found in real-world shapes. In the man-made environment, shapes can be irregular and convoluted. Take, for example, furniture in a home setting: the shapes are often not just simple geometries like circles or squares, but rather intricate designs that vary from piece to piece.
Additionally, environmental conditions—lighting discrepancies, angles of view, and occlusions—further complicate the task. While algorithms can classify basic shapes under laboratory conditions, translating that ability to varied field conditions can present a hefty challenge. It’s similar to trying to read a book under a flickering light; the words may blur, making it hard to comprehend. The greater the complexity in shapes, the more advanced and nuanced the algorithms need to be.
Computational Limits and Efficiency
Computational limits also play a significant role in the efficacy of AI shape recognition systems. As shapes grow increasingly complex and datasets larger, the demand for processing power rises. This creates bottlenecks, especially in real-time applications, where swift analysis is paramount.
To compound matters, not every organization has access to cutting-edge hardware. Many smaller enterprises grapple with limited resources, grappling with outdated systems that slow down processing and increase operational costs. An efficient algorithm that can perform well under low computational costs is not just a luxury; it's a necessity for practical applications in various sectors, from healthcare to autonomous driving.
Moreover, energy consumption is another consideration. As we push the boundaries of shape recognition technology, we must balance accuracy with sustainability. Developing algorithms that are not only efficient but also environmentally friendly will be a critical aspect moving forward.
The Future of AI Shape Recognition
The future of AI shape recognition emerges as a pivotal topic in understanding both the development of this technology and its broader implications across various sectors. As we look to the horizon, it becomes clear that advancements in this field not only influence how machines interpret visual stimuli but also fundamentally reshape industries, social dynamics, and ethical considerations surrounding technology.
Trends in Research and Development
In recent years, there has been a noticeable upturn in research dedicated to AI shape recognition, with several trends indicating where this technology is headed. Researchers are delving deeper into enhancing existing algorithms, improving accuracy, and expanding the range of shapes and complexities that AI can recognize. One of the prominent trends is the integration of hybrid models that combine different machine learning techniques to create a more robust system. These models often draw from neural networks and traditional computational geometry, balancing the strengths of each method.
Furthermore, there's a trending emphasis on real-time recognition capabilities. This shift can be particularly beneficial for applications like autonomous driving, where understanding shapes and their relative movements quickly can mean the difference between safety and accidents. The move towards mobile AI solutions is also notable, as researchers strive to bring shape recognition capabilities to devices with limited computational resources, such as smartphones.
Ethical Considerations
As with any rapidly advancing technology, ethical considerations loom large over the future of AI shape recognition. The conversations around bias and fairness in AI echo louder than ever, reminding us that while these advancements offer tremendous potential, they also carry significant responsibilities.
Bias and Fairness
The concept of bias in AI systems, particularly in shape recognition, is particularly salient. Bias can manifest in various forms, often affecting how different shapes are recognized across demographics. For instance, if a dataset predominantly features one type of shape or skewed representations of cultures, the AI might perform poorly on underrepresented images. This concern is essential in fields like law enforcement or hiring processes, where decisions based on AI shape recognition can lead to unfair treatment. Ensuring fairness involves diverse datasets that better represent the variety of shapes and contexts present in the world, thus allowing the AI to recognize shapes impartially.
The unique feature of focusing on bias and fairness is its potential to create trust between technology and society. A fair AI can build confidence in automated systems, making it less likely to face backlash or skepticism from users.
Privacy Concerns
Alongside fairness, privacy concerns pose another significant challenge. With the ongoing collection of visual data for training AI models, questions arise about what happens to personal data. Ensuring user privacy while allowing these systems to learn is a balancing act that requires transparency and strict data governance measures.
A notable aspect of privacy is the need for informed consent when data is collected, especially in sensitive areas such as healthcare.
Implementing privacy by design as a unique feature during the development of AI systems can help mitigate risks. By embedding privacy measures from the onset, developers can address potential issues proactively, safeguarding user information while still advancing technological capabilities.
Potential Impact on Industries
The ramifications of AI shape recognition systems will resonate across various sectors, including healthcare, automotive, and entertainment. The potential for revolutionary changes to workplaces and consumer experiences cannot be understated.
Transforming Workplaces
AI shape recognition is set to transform workplaces, especially in manufacturing and logistics. The technology can streamline operations, such as inventory management or quality assurance by enabling machines to identify and classify shapes of products on the assembly line. This can significantly reduce errors and lead to heightened efficiency.
In this regard, embracing AI shape recognition may prove to be a double-edged sword for employees. While it can free workers from repetitive tasks, there's also the fear of job displacement, prompting significant discussions about the future of work in an automated landscape.
Influencing Consumer Experiences
The potential influence of AI shape recognition on consumer experiences is both exciting and daunting. Retailers could use the technology to better understand customer behavior by analyzing physical movements within stores or online. Enhanced customer experiences could be tailor-made by predicting needs based on shape recognition patterns. For instance, virtual try-ons fueled by shape recognition could revolutionize how people shop, allowing seamless fitting experiences without physical trials.
Yet, the very technology that promises personal experiences could infringe on privacy. Users could find themselves navigating a landscape where their movements are tracked, raising discomfort and legal questions about data ownership and usage.
In summary, the future of AI shape recognition encompasses both great promise and notable challenges. While the technology is still in its formative years, the trends in research and development, ethical considerations, and potential impacts on industries serve as critical focal points. Understanding these aspects will not only enhance the discourse around AI shape recognition but also guide its evolution in responsible and beneficial ways.
Closure
Drawing the curtain on AI shape recognition reveals a multifaceted and vital discipline within the realm of artificial intelligence. This field's significance extends beyond mere technological curiosity; it plays a major role in various sectors including healthcare, autonomous driving, and robotics. First and foremost, understanding how AI systems can discern shapes opens the door to numerous benefits, such as increased efficiency and precision in areas way too complex for humans to handle alone. By analyzing visual data, these systems not only enhance functionality but also improve user experience in countless applications.
Summary of Key Points
Let's take a moment to consolidate the major takeaways from our exploration:
- Defining and Understanding: AI shape recognition involves the ability of machines to identify and interpret shapes. A solid comprehension of this technology can significantly improve AI's practical impacts.
- Technological Advances: Innovations in machine learning, especially neural networks, have propelled shape recognition into new territories, affecting its efficiency and accuracy in real-time applications.
- Applications Across Sectors: Enhancements in healthcare and autonomous driving showcase how this technology transforms industries, providing revolutionary changes with substantial implications.
- Challenges Ahead: Despite the advancements, challenges such as data quality and the complexity of real-world shapes continue to pose hurdles that require ongoing research.
- Future Directions: As this field continues to evolve, understanding ethical implications becomes equally important. We must consider how biases may creep into algorithms and influence results in potentially harmful ways.
Final Thoughts on AI Shape Recognition
As we stand on the brink of further advancements in AI shape recognition, it is clear that we are just scratching the surface. The potential of this technology is vast and exciting, but it requires a careful and informed approach to navigate the challenges that accompany its applications. Just as a sculptor chisels away stone to reveal a masterpiece, researchers and developers must refine shape recognition algorithms to ensure precision, fairness, and efficiency.