Exploring the Synergy of AI and Quantum Computing
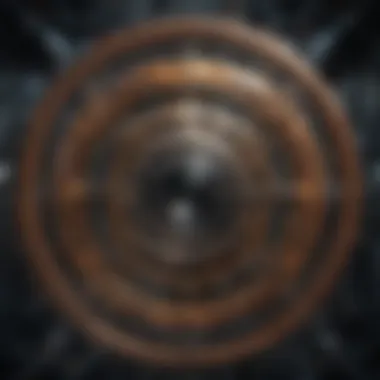
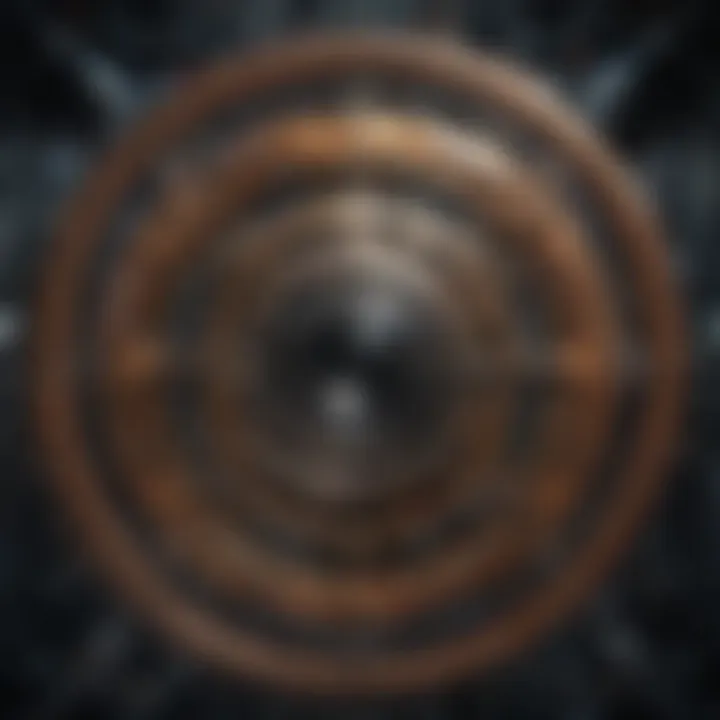
Intro
The fields of artificial intelligence (AI) and quantum computing are advancing at an unprecedented pace. Researchers and developers are beginning to explore how these two domains might converge. This intersection holds the potential to create systems that could revolutionize industries such as pharmaceuticals, finance, and materials science. Understanding the foundational elements of AI and quantum computing is crucial to appreciating their combined capabilities.
Key Research Findings
Overview of Recent Discoveries
Recent studies demonstrate promising results in the integration of quantum computing algorithms with machine learning techniques. For instance, quantum algorithms like the Quantum Approximate Optimization Algorithm (QAOA) and Quantum Support Vector Machines (QSVM) are showing improved performance in solving complex problems that are often intractable for classical computers. This synergy is evident in the exploration of large datasets, where quantum speed-ups can significantly enhance computational efficiency.
Significance of Findings in the Field
These advancements are reshaping our approach to data analysis, modeling, and simulation. The significance lies in the unique computational advantages that quantum systems can bring to AI tasks. By leveraging phenomena like superposition and entanglement, quantum computers can process information in ways that classical systems cannot. For example, in drug discovery, the combined power of AI and quantum computing could lead to the identification of new compounds at an unprecedented scale.
"The convergence of AI and quantum computing is not merely an incremental improvement; it represents a paradigm shift in how we approach complex problem-solving."
Breakdown of Complex Concepts
Simplification of Advanced Theories
To better understand this intersection, it is essential to break down complex theories. AI primarily involves algorithms that enable machines to learn from data. These algorithms can be classified into supervised, unsupervised, and reinforcement learning. Quantum computing, on the other hand, relies on quantum bits or qubits, which can represent multiple states simultaneously, providing a significant advantage in processing capacity.
Visual Aids and Infographics
Using infographics can aid in visualizing data flows between traditional and quantum computing systems. For example:
- Classical AI Model: Involves linear computations, where one state follows another in a sequence.
- Quantum AI Model: Utilizes quantum entanglement to link states, allowing for simultaneous processing of multiple paths.
This distinction highlights how quantum models can exponentially enhance the efficiency of AI algorithms, opening new avenues for innovation.
End
In summary, the intersection of AI and quantum computing is an area ripe for exploration and innovation. The discoveries made so far suggest a powerful partnership that could redefine problem-solving across various sectors. Understanding the core concepts and their implications will pave the way for future advancements that hold promise for both technology and society.
Prelims
By merging AI's exceptional data processing and learning capabilities with the computational power of quantum mechanics, organizations can unleash a new wave of possibilities. AI can benefit from quantum computing's ability to analyze vast datasets at inconceivable speeds. This synergy promises to enhance efficiencies, improve decision-making processes, and foster groundbreaking innovations.
However, while the potential is significant, there are various considerations to navigate in this journey. Ethical implications, data security, and the current limitations in quantum hardware are just a few factors that must be addressed. Moreover, understanding these technologies deeply is crucial for leveraging their full potential.
Through this article, we aim to provide a thorough examination of these technologies and their interrelationship, delving into foundational aspects, current applications, potential challenges, and future implications.
"The future will be shaped by the interplay between AI and Quantum Computing, ultimately redefining how we solve complex problems."
Defining Artificial Intelligence
Artificial Intelligence, often abbreviated as AI, refers to the simulation of human intelligence processes by machines, particularly computer systems. These processes include learning, reasoning, problem-solving, perception, and language understanding. The primary goal of AI is to create systems capable of performing tasks that typically require human cognitive abilities.
AI can be classified into two broad categories: narrow AI and general AI. Narrow AI refers to systems designed to handle specific tasks, while general AI encompasses machines that possess the ability to understand and learn any intellectual task a human can perform.
Applications of AI are already prevalent in many sectors, including healthcare, finance, transportation, and communication. Tools like natural language processing, image recognition, and predictive analytics are all examples of how AI is being utilized to improve efficiency and productivity.
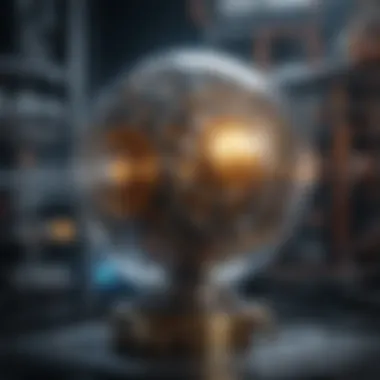
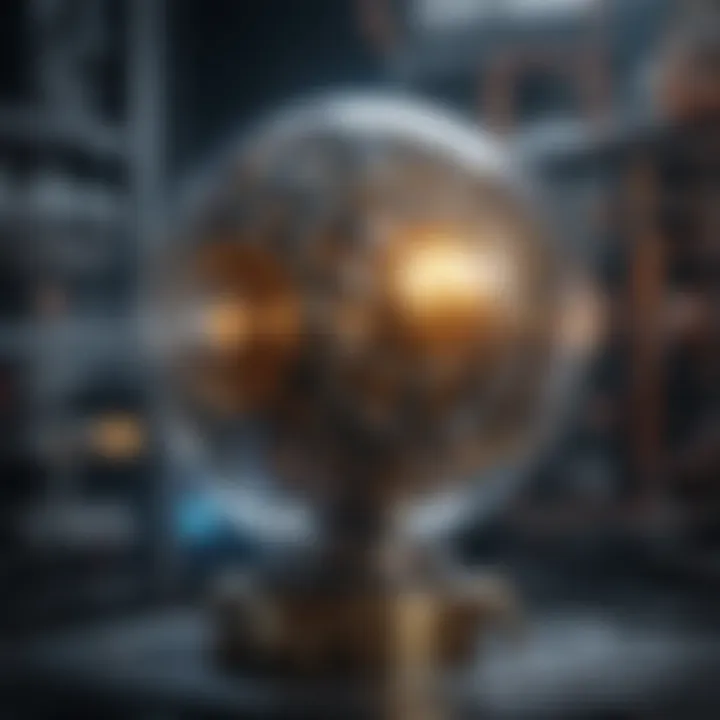
Understanding Quantum Computing
Quantum computing is a revolutionary approach to computation that utilizes the principles of quantum mechanics. Unlike classical computers, which use bits as the smallest unit of data, quantum computers use quantum bits or qubits. Qubits have the unique ability to exist in multiple states simultaneously, thanks to a property known as superposition. This allows quantum computers to process information in ways that classical computers cannot.
Another key concept in quantum computing is entanglement. When qubits become entangled, the state of one qubit becomes dependent on the state of another, no matter how far apart they are. This characteristic can potentially lead to unprecedented levels of processing power, enabling quantum systems to solve complex problems much faster than their classical counterparts.
Current research in quantum computing is centered on developing practical applications, ranging from cryptography to optimization problems.
Interrelationship Between AI and Quantum Computing
The interaction between AI and quantum computing is an area of significant interest and research. AI can benefit from quantum computing's capabilities in various ways. For example, quantum algorithms can optimize machine learning models, enabling faster training times and improved accuracy. This is particularly valuable in fields that require analyzing large datasets or complex simulations.
Conversely, AI can also aid quantum computing. Machine learning techniques can help interpret quantum data and develop new quantum algorithms. By learning from past quantum experiments, AI systems could inform better decision-making processes for future research and development.
The integration of these two fields could lead to breakthroughs that were previously unimaginable. As research evolves, we expect to see more cross-disciplinary collaborations that maximize the potential of both technologies.
The Fundamentals of Artificial Intelligence
Understanding the fundamentals of artificial intelligence is crucial to appreciate the implications and opportunities that arise when integrating this technology with quantum computing. AI serves as the backbone for numerous modern applications, from virtual assistants to autonomous vehicles. Without a solid grasp of its core principles, the synergy between AI and quantum computing may remain elusive to many.
Key Concepts of AI
Artificial intelligence revolves around the development of algorithms and systems that can perform tasks typically requiring human intellect. Key concepts include:
- Machine learning: A subset of AI where systems learn from data, improving performance over time.
- Natural language processing: The ability of machines to understand and respond to human language.
- Computer vision: Enabling machines to interpret and make decisions based on visual inputs.
Understanding these concepts is fundamental for recognizing how AI can evolve with advances in quantum computing.
Machine Learning and Deep Learning
Machine learning is a pivotal area in AI that focuses on enabling machines to learn from data without explicit programming. It utilizes statistical techniques to enable machines to improve at tasks through experience. Deep learning, a further specialization of machine learning, employs neural networks with multiple layers. These networks can model complex patterns and representations in data.
For instance, in image recognition tasks, a deep learning model can identify features such as edges and textures, progressing through layers to recognize complex objects like faces or animals. Its capacity to process vast amounts of unstructured data efficiently makes it particularly suited for many real-world applications.
Applications of AI in Various Sectors
AI's versatility allows it to be implemented across diverse sectors. Key applications include:
- Healthcare: AI algorithms assist in diagnosing diseases, predicting patient outcomes, and personalizing treatment plans.
- Finance: In finance, AI helps in fraud detection, algorithmic trading, and personalized banking services.
- Manufacturing: Robotics powered by AI streamline production processes, improving efficiency and reducing errors.
- Transportation: Self-driving cars leverage AI to make real-time driving decisions, aiming to reduce accidents and traffic congestion.
These applications underscore the transformative power of AI. Coupled with quantum computing's capabilities, the potential advancements could be significant, enabling even more sophisticated models and solutions to complex problems.
The Principles of Quantum Computing
Quantum computing represents a groundbreaking shift in how we process information. Its significance in the realm of technology cannot be overstated. At the heart of quantum computing are several key concepts that differentiate it from classical computing. Understanding these elements is crucial for grasping the potential benefits and applications of this emerging technology.
Quantum Bits and Superposition
In classical computing, the basic unit of data is the bit, representing a state of either 0 or 1. Quantum computing utilizes quantum bits, or qubits. Qubits can exist in multiple states at once, a phenomenon known as superposition. This ability allows quantum computers to perform many calculations simultaneously. The implications of this are substantial. With superposition, a quantum computer can process information at a rate fundamentally beyond that of classical computers.
- Key Features of Qubits:
- Representation: Unlike a bit, a qubit can represent 0 and 1 at the same time.
- Entanglement: This unique property allows qubits that are entangled to be correlated with each other irrespective of distance.
- Interference: Quantum algorithms make use of interference patterns to enhance the probability of correct outcomes while minimizing incorrect ones.

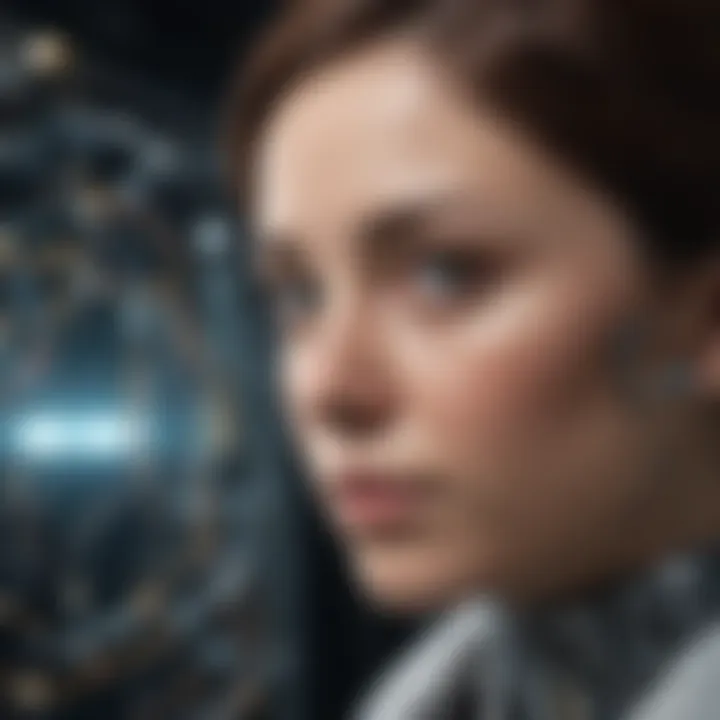
With these characteristics, quantum computing offers a more robust framework for solving complex problems, particularly in areas like cryptography and optimization.
Entanglement and Quantum Gates
Entanglement is another crucial principle of quantum computing. When two qubits become entangled, the state of one qubit becomes dependent on the state of another, regardless of the distance separating them. This interconnectedness is vital for quantum communication and is a key feature that makes quantum algorithms powerful.
In addition to entanglement, quantum gates serve as the operational tools in a quantum circuit. They manipulate the state of qubits through various quantum operations. These gates function similarly to classical logic gates but take advantage of quantum mechanics to allow for a greater degree of complexity in computations. Below are examples of quantum gates:
- Pauli-X Gate: Acts similar to a classical NOT gate, flipping the qubit's state.
- Hadamard Gate: Places a qubit in a state of superposition, enabling multiple calculations at once.
- CNOT Gate: A controlled gate that entangles two qubits, serving as a fundamental building block for quantum algorithms.
Potential Applications of Quantum Computing
The convergence of quantum computing with various sectors could lead to revolutionary advancements. Some potential applications include:
- Pharmaceutical Research: Quantum computing can model complex molecules much faster than classical computers, significantly accelerating drug discovery.
- Optimization Problems: Industries such as logistics and finance can optimize routes and investment strategies efficiently using quantum algorithms.
- Cryptography: Quantum computing could break many of the cryptographic systems currently in use, necessitating new forms of secure communication.
"Quantum computing has the potential to solve problems that are currently considered intractable for classical computers."
These applications highlight not only the power of quantum computing but also its transformational potential across technology, science, and even social frameworks.
Current State of AI-Quantum Computing Integration
The integration of artificial intelligence and quantum computing represents a pivotal evolution in computational capabilities. This section explores the contemporary state of this synergy, underscoring the significance of research advancements, strategic company initiatives, and collaborative efforts in the field. Together, these elements provide insight into how they may reshape technology and industry.
Research Developments
Research in AI and quantum computing is rapidly expanding. Various academic institutions and laboratories are exploring how quantum algorithms can enhance algorithms traditionally driven by AI. Recently, initiatives have surfaced aimed at improving machine learning techniques utilizing quantum circuits. For instance, researchers have focused on developing quantum support vector machines and quantum neural networks. These innovations aim to increase computational efficiency, allowing for the processing of vast datasets at unprecedented speeds. Moreover, publications in peer-reviewed journals are beginning to address how such integrations can lead to breakthroughs in fields like cryptography, drug discovery, and complex systems modeling.
The potential for quantum computing to solve problems deemed intractable for classical systems may reshape not only AI but also entire industries.
Company Initiatives
Corporate interest in the integration of AI and quantum computing is growing. Tech giants like IBM and Google have dedicated resources to explore this convergence. IBMβs Quantum Experience platform, for example, allows users to experiment with quantum algorithms and integrate them with AI tools. Similarly, Googleβs Quantum AI efforts seek to develop practical applications that use quantum processors to enhance machine learning capabilities. These initiatives illustrate how industry leaders view this integration as a pathway to competitive advantage, prioritizing investments that enhance research and product development in quantum-enhanced AI systems.
- Investment trends highlight several areas:
- AI optimization of quantum algorithms.
- Development of hybrid models combining classical and quantum computing.
- Focus on scalable quantum computing architectures to support AI.
Collaborations in the Field
Collaboration between various stakeholders is crucial for pushing the boundaries of AI and quantum computing. Joint efforts between academia and industry are yielding fruitful results. Many universities partner with technology companies to drive innovation. For instance, partnerships between institutions like MIT and corporations such as Microsoft have produced notable advancements in quantum machine learning.
"Integrating multi-disciplinary perspectives accelerates progress in understanding the implications of combining AI and quantum technology."
The global landscape is seeing similar collaborations as researchers and engineers converge to brainstorm solutions, share ideas, and solve complex challenges. This ecosystem encourages knowledge sharing that propels technology forward and addresses the diverse applications and implications of this integration.
In summary, the current state of AI-quantum computing integration encompasses pivotal research advancements, industry initiatives, and collaborations driving innovation. The alignment of these components is vital for harnessing the vast potential of both technologies.
Challenges of AI and Quantum Computing Collaboration
The collaboration between artificial intelligence (AI) and quantum computing holds great potential. Yet, this synergy is not without its challenges. Understanding these obstacles helps navigate the journey towards effective integration. There are several dimensions to consider: technical barriers, ethical implications, and the skills gap.
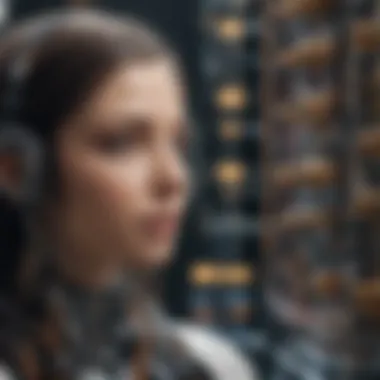
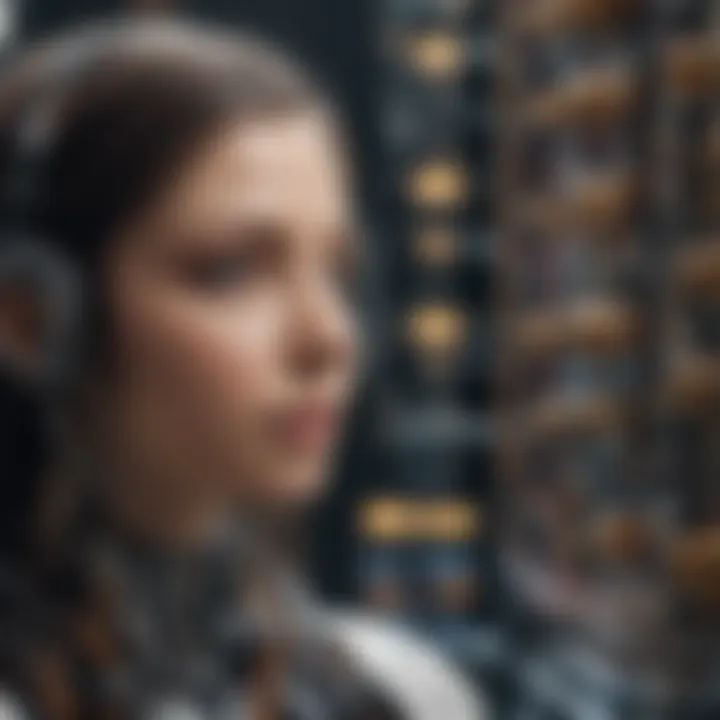
Technical Barriers
One of the primary technical barriers is the lack of robust quantum infrastructure. Quantum hardware is still in an experimental phase. Most companies developing AI need reliable quantum computers for their algorithms. The existing quantum systems, such as those from IBM and Google, often lack the necessary scalability and error correction capabilities. This limitation reduces their effectiveness in solving complex AI problems.
Moreover, the algorithms that AI practitioners typically use do not seamlessly transition to quantum environments. Most machine learning algorithms were designed with classical computers in mind. Adapting these algorithms for quantum computing demands a significant shift in approach and often a considerable level of expertise. This presents a significant technical challenge for researchers.
Ethical Considerations and Risks
The intersection of AI and quantum computing raises important ethical considerations. With great power comes great responsibility. AI's capability to analyze and process vast amounts of data can lead to privacy infringements. Meanwhile, quantum computing may amplify this issue due to its processing speed and ability to break current encryption methods.
There is also the risk that quantum-enhanced AI could contribute to biased decision-making. If the underlying data is skewed, the results may propagate existing inequalities. Thus, both fields must address transparency and fairness, ensuring that equitable practices are in place as they develop new technologies.
The Skills Gap
A significant hurdle in the interplay of AI and quantum computing is the skills gap. Professionals are needed who understand both fields thoroughly. The intricacies of quantum mechanics combined with AI's complexity create a steep learning curve.
- Educational programs must evolve to bridge this gap. Universities should develop interdisciplinary curricula that combine quantum physics, computer science, and AI to produce adept graduates.
- Industry leaders can also take initiatives. They should invest in training programs to upskill existing employees, making sure they are familiar with both domains.
In summary, while the collaboration of AI and quantum computing offers many benefits, the challenges are considerable. Addressing technical barriers, ethical concerns, and the skills gap is essential for the successful integration of these transformative technologies.
Future Prospects of AI and Quantum Computing
The future prospects of AI and quantum computing represent a critical juncture in technological evolution. As these fields converge, the implications extend beyond computational efficiency; they may redefine our approach to complex problem-solving and data processing. A deeper understanding of these prospects is essential for students, researchers, educators, and professionals who aim to stay ahead in this rapidly changing landscape.
Predictions for AI Advancements
Advancements in AI are projected to follow a trajectory shaped by the integration with quantum computing. Predictive algorithms will harness quantum processing power to analyze vast datasets much quicker than classical systems. This may lead to innovations in natural language processing, image recognition, and real-time data analytics. By utilizing quantum states, AI can achieve higher accuracy in predictions, especially in fields such as healthcare, finance, and autonomous vehicles. Expect significant developments in the precision of generative models and enhanced capabilities in machine learning frameworks. The collaboration will likely lead to truly intelligent systems that can reason, learn, and adapt in unprecedented ways.
The Transformational Potential of Quantum Computing
Quantum computing holds transformational power across various sectors. Its ability to perform many calculations simultaneously enables solutions to problems deemed infeasible for classical computers. Industries like drug discovery and cryptography will benefit immensely. Quantum algorithms can simulate molecular structures far more efficiently, leading to breakthroughs in pharmaceuticals. Furthermore, the financial sector may see enhancements in risk analysis and portfolio optimization thanks to quantum algorithms that can process complicated calculations quickly. It becomes clear that the potential for integration with AI can pave the way for entirely new paradigms in several critical areas of society.
Societal Impacts of the Integration
The merging of AI and quantum computing can lead to profound societal impacts. As algorithms become more powerful, there is a promise for solving pressing global challenges such as climate change, energy management, and disease control.
However, the implications are not purely beneficial. Ethical concerns arise regarding job displacement and privacy issues, especially in data-sensitive fields. As machines become increasingly capable, the necessity for a framework to govern their use becomes urgent. Educational systems will need to adapt to prepare the workforce for the demands of a quantum-augmented future.
"The integration of AI and quantum computing will not only advance technological frontiers but will also challenge our ethical frameworks and societal norms."
In summary, the future prospects of AI and quantum computing present a landscape rich with opportunities and challenges. Understanding the dynamics between these technologies is crucial for navigating the complexities ahead.
Finale
In synthesizing the various aspects of artificial intelligence and quantum computing, the conclusion serves as a crucial element in encapsulating the broader themes discussed in this article. This segment draws attention to the transformative potential that emerges from the convergence of these two fields. As understanding deepens regarding their interrelationship, it becomes evident that the implications are far-reaching and multifaceted.
Summary of Key Points
- Interconnected Technologies: Both AI and quantum computing stand at the forefront of technological evolution. Their integration could lead to advancements that were previously unattainable.
- Current Developments: Research and initiatives demonstrate a growing interest in combining these technologies. Companies are investing resources to explore practical applications.
- Challenges Ahead: There are notable barriers, including technical hurdles and ethical considerations, that must be addressed to foster meaningful collaboration between AI and quantum computing.
- Future Prospects: Predictions suggest significant advancements and societal impacts that could arise from the successful synergy of AI and quantum computing.
Final Thoughts on the Convergence of Technologies
The confluence of artificial intelligence and quantum computing not only represents a significant leap in computational power but also poses profound questions and considerations. While the benefits are promising, such as enhanced problem-solving capabilities and novel applications across numerous sectors, it becomes crucial to approach the integration with caution.
Ensuring that ethical frameworks are established is imperative to fully unlock the potential. Furthermore, stakeholders must prioritize addressing the skills gap to effectively harness the full capabilities of both technologies. By doing so, we can navigate the complexities of this convergence, ensuring it leads towards a constructive and beneficial future for technology and society as a whole.
"The future of technology lies in understanding its convergence and realizing its potential to solve critical challenges."
The integration of AI and quantum computing exemplifies the dynamic nature of technological advancement. Careful consideration and intentional actions can catalyze a new era of innovation that reshapes industries and improves human experiences.