AI Continuous Learning: Revolutionizing Knowledge Acquisition
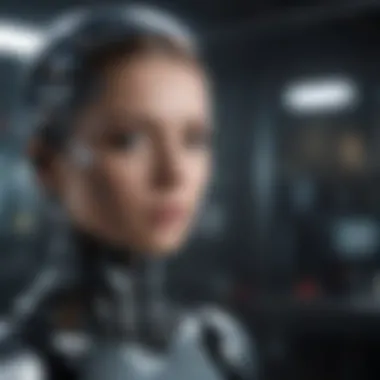
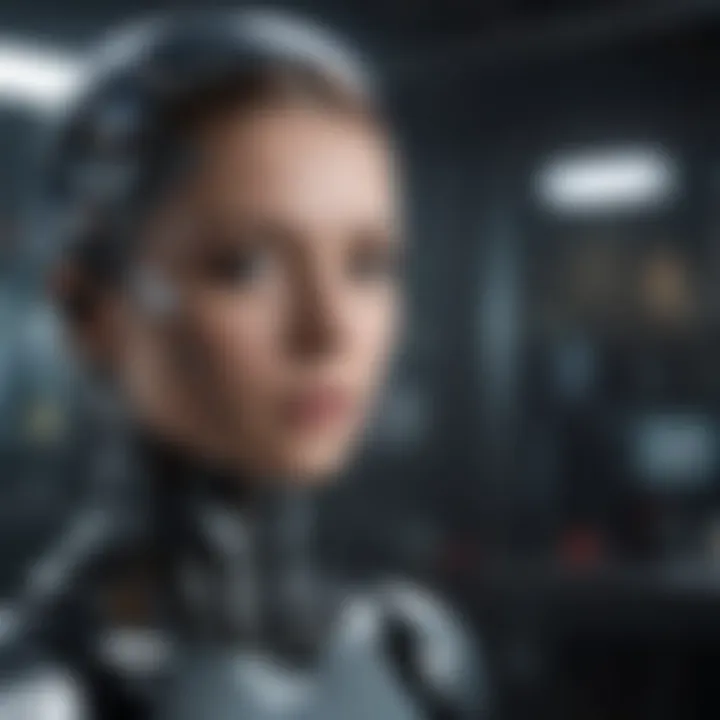
Intro
Artificial Intelligence (AI) has seen rapid advancements in recent years, with continuous learning emerging as a cornerstone of its evolution. Continuous learning refers to the ability of AI systems to learn from new information and experiences without the need for complete retraining. This adaptive quality is vital for dealing with the ever-changing landscape of data in various domains. In this article, we will explore key findings related to AI continuous learning, delve into its mechanisms, identify its challenges, and discuss its broad applications.
Key Research Findings
Overview of Recent Discoveries
Recent studies have focused on various techniques that enable AI systems to perform continuous learning efficiently. These techniques include:
- Online Learning: Systems can update their models incrementally as new data arrives, rather than employing batch learning methods.
- Transfer Learning: This approach involves leveraging knowledge gained in one domain to facilitate learning in another, making the process faster and more efficient.
- Meta-Learning: Also known as "learning to learn," this technique aims to optimize the learning process by enabling AI systems to adapt their strategies based on prior experiences.
These discoveries showcase how AI can achieve ongoing learning in practical scenarios, minimizing the downtime often required for retraining.
Significance of Findings in the Field
The ability to continuously learn transforms how AI integrates into real-world applications. This represents a significant evolution in the capabilities of AI systems, providing several benefits:
- Enhanced Flexibility: Continuous learning enables AI to adjust more readily to new situations and data.
- Improved Efficiency: Ongoing learning can help reduce the resources and time needed for training models, allowing for quicker deployment and real-time updates.
- Better Decision Making: As AI systems learn continuously, they can improve their performance and accuracy, leading to more informed outcomes in applications ranging from healthcare to finance.
Continuous learning is reshaping the paradigms of AI development and application.
Breakdown of Complex Concepts
Simplification of Advanced Theories
To understand continuous learning deeply, it is essential to break down its core components. The primary concepts include:
- Feedback Mechanisms: These allow systems to learn from their successes and failures, improving over time.
- Data Integration: The challenge of merging new data with existing knowledge bases in a coherent manner.
- Model Adaptation: How models can adjust to new patterns while retaining important information from previous experiences.
These elements combine to create a robust continuous learning system capable of adapting dynamically to the external environment.
Visual Aids and Infographics
Visual aids can enhance understanding of continuous learning processes, showing how data flows through AI systems and how models update based on new information. Diagrams illustrating the transition from traditional learning methods to continuous learning frameworks can be particularly informative.
By highlighting the flow of data and the mechanisms at play, visual representations can simplify the complexities of AI continuous learning for researchers, educators, and practitioners alike.
Understanding Artificial Intelligence
The importance of understanding artificial intelligence (AI) is central for exploring the nuances of AI continuous learning. AI represents a significant technological advancement with the potential to reshape various fields including healthcare, finance, and education. However, without a clear comprehension of what AI is and how it functions, discussions on continuous learning can quickly become muddled. Definitions and principles empower researchers, educators, and professionals to navigate the complexities of AI with precision.
Understanding AI involves recognizing its core components, namely machine learning, natural language processing, and computer vision. Each of these elements provides a building block that contributes to the holistic capabilities of AI systems. Furthermore, it fosters a sense of responsibility in terms of how we develop, implement, and govern AI technologies. Better comprehension leads to informed choices that can mitigate risks associated with AI misuse.
Definition and Scope
Artificial intelligence can be defined as the simulation of human intelligence in machines that are programmed to think and learn like humans. The scope of AI includes multiple subfields such as machine learning, deep learning, and neural networks. AI continuously evolves, expanding into areas like robotics and autonomous systems. This evolution highlights the necessity to define and refine the scope continually, ensuring alignment with ever-changing technological and societal dynamics.
Separating AI systems into different categories can also aid in clarification. These categories include narrow AI, which is designed for specific tasks, and general AI, which aims to understand and reason across a broader scope. Continuous learning falls primarily within the narrow AI category but offers significant enhancements to knowledge acquisition through adaptive learning processes.
Historical Context
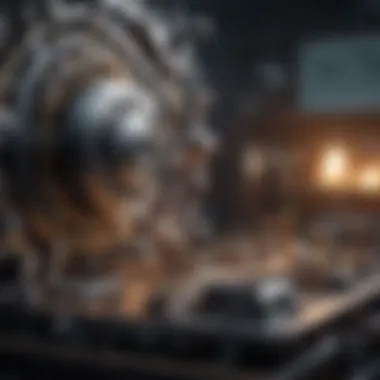
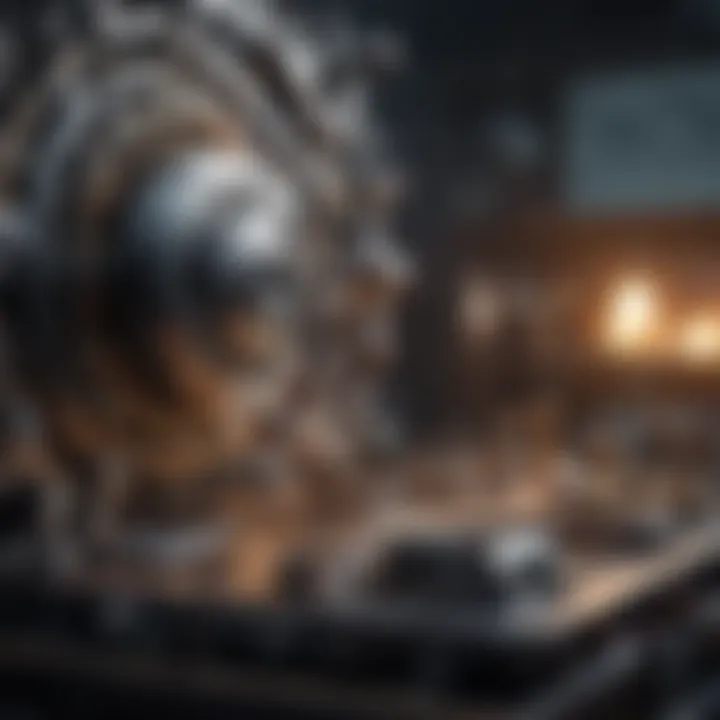
The journey of artificial intelligence has been characterized by various milestones. Beginning in the mid-20th century, AI was conceptualized as a means to replicate human cognitive functions. Initial endeavors focused on symbolic reasoning, emphasizing logic and rules. However, the limitations of these early models became evident, leading to the development of statistical methods in the 1980s and 1990s.
The advent of more substantial computational power and large datasets introduced machine learning approaches, transforming the landscape of AI. As algorithms matured, AI witnessed a renaissance in the 21st century, driven by advancements in deep learning and increased interest in practical applications. Today, AI's ability to continuously learn and adapt from real-time data marks a new chapter in its history, providing exciting opportunities for innovation.
In summary, understanding artificial intelligence is crucial for contextualizing AI continuous learning. With a solid foundation in definitions and historical developments, readers can appreciate the interconnections and implications of continuous learning in transforming knowledge acquisition.
The Principles of Continuous Learning
Continuous learning is not merely an academic concept; it stands as a fundamental principle that underpins the evolution of artificial intelligence. In the context of AI, continuous learning refers to the capability of systems to assimilate new data and improve performance over time without reverting to initial states. This principle is pivotal as it shapes how AI adapts to dynamic environments, enhances efficiency, and ultimately improves decision-making processes.
Key Components
The key components of continuous learning in AI involve different methodologies and frameworks that enable effective learning from an unceasing stream of data. These components include:
- Lifelong Learning: AI systems are designed to learn incrementally, acquiring knowledge over time while retaining previous information. This helps to build a richer knowledge base without starting from scratch.
- Incremental Learning: Unlike traditional batch learning, incremental learning allows systems to assimilate data as it arrives, leading to reduced latency in learning cycles.
- Feedback Mechanisms: Continuous learning hinges on mechanisms that facilitate feedback. This includes both positive reinforcement for desired outcomes and adjustments based on errors or mispredictions.
- Adaptive Algorithms: These are pivotal in continuous learning, allowing for adjustments to be made in response to new data trends and requirements.
Mechanisms of Learning
Understanding the mechanisms that drive continuous learning is essential for grasping its significance in AI applications. The mechanisms include:
- Real-Time Data Processing: Continuous learning systems rely on algorithms that can process data in real-time, making it possible to adapt promptly to new information.
- Transfer Learning: This mechanism involves leveraging knowledge acquired from one task to improve the learning of another. It minimizes redundancy and accelerates the learning curve.
- Self-Supervised Learning: In this approach, the system generates its own labels from the data, facilitating learning without the need for extensive annotation by humans.
- Neural Networks: Deep learning models often utilize architectures that support continuous learning, making them adept at adapting to latent patterns within data while preserving essential information from previous stages.
In short, the principles of continuous learning form the backbone of adaptive AI systems, enabling them to evolve and improve become more robust over time.
The exploration of these components and mechanisms reveals the greater potential of AI in diverse fields, particularly as the demand for intelligent systems that continuously learn and adapt becomes increasingly pronounced. An in-depth understanding of this principle not only benefits researchers but also serves educators and professionals striving for innovation in their respective domains.
Contrasting Traditional Learning Models
Understanding the differences between traditional learning models and continuous learning is crucial for grasping the impact of AI continuous learning on knowledge acquisition. Traditional learning often relies on static datasets, where models are trained with a fixed set of information before deployment. This approach lacks the adaptability required for todayβs data-driven environments.
Batch Learning vs. Continuous Learning
Batch learning refers to the training of models on a large set of data all at once. Once the model is trained, it is then used for inference without incorporating new data until the next training cycle. This can lead to challenges when the data environment changes dynamically. For example, if a weather prediction model is trained on historical data but not updated regularly, its predictions might become inaccurate as new weather patterns emerge.
In contrast, continuous learning allows models to adapt and learn incrementally from new data. This approach makes the system more resilient and capable of providing relevant outputs in real time. As a result, continuous learning systems can manage the ever-changing flow of data without significant downtimes for retraining. Moreover, models can refine their predictions based on the latest information, which is essential in fields such as finance or healthcare where real-time decision-making may significantly impact outcomes.
Continuous learning can be valuable not only in updating data but also in enriching the model with diverse knowledge sources. The ability to learn continuously aligns with how humans accumulate knowledge and adapt to their surroundings, providing a more natural and effective mechanism of learning.
Advantages of Continuous Learning
The momentum of continuous learning brings several advantages compared to traditional methods. These advantages include:
- Adaptability: Continuous learning models can adjust to new information and emerging trends.
- Efficiency: Instead of retraining from scratch, these models update their existing knowledge base, saving time and computational resources.
- Improved Accuracy: These systems can provide more accurate results by constantly refining their understandings based on recent data.
- Reduced Risk of Obsolescence: By integrating new knowledge regularly, continuous learning reduces the risk of becoming outdated, enhancing relevancy in rapidly evolving fields.
In summary, the comparative analysis of traditional learning models and continuous learning highlights a shift necessary for the future of AI development. The former is static and confined, while the latter offers the dynamic capabilities demanded by modern applications. As understanding of these distinctions deepens, it becomes clear that embracing continuous learning methods will shape more intelligent and responsive AI systems.
Challenges in Implementing Continuous Learning
Continuous learning in AI presents numerous challenges and considerations that must be addressed if we are to harness its transformative potential effectively. As AI systems are integrated more deeply into various sectors, understanding these challenges becomes critical. Not only do they influence the performance of AI models, but they also impact the overall reliability and ethical implications involved in AI deployment. Navigating these complexities is essential for both practitioners and organizations looking to utilize AI continuous learning effectively.
Data Management Issues
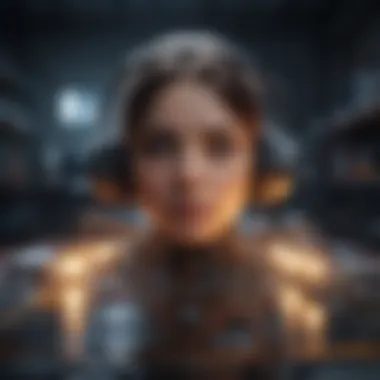
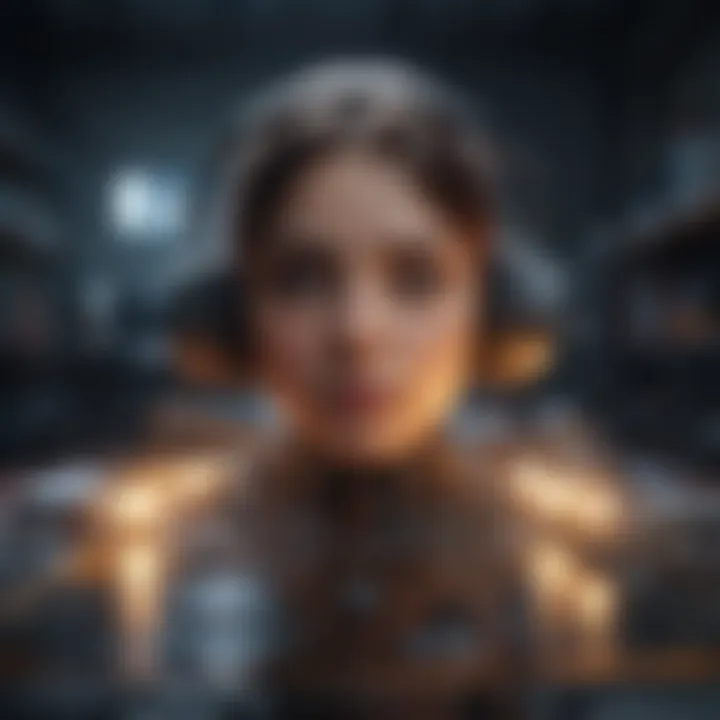
One of the foremost challenges related to continuous learning is data management. For AI models to learn continuously, they need access to a constant stream of data. This data must not only be diverse and comprehensive, but it should also be cleaned and pre-processed effectively. The sheer volume of data generated on a daily basis can lead to several problems:
- Integration: Merging data from different sources can prove difficult, as discrepancies may arise.
- Quality: Data quality must be assured to avoid biases that could skew learning outcomes.
- Storage: As models continuously learn, the storage requirements increase, necessitating robust data architecture.
These issues require well-thought-out strategies to manage data efficiently. Organizations need develop best practices around data governance to tackle these challenges up front.
Model Degradation and Catastrophic Forgetting
A significant concern in continuous learning paradigms is the issue of model degradation. As new information is incorporated, existing knowledge can be overshadowed, leading to a phenomenon commonly referred to as catastrophic forgetting. This can severely impair the reliability of an AI model, as it may lose previously learned behaviors that are still relevant.
Addressing this problem often involves:
- Regularization techniques that help maintain a balance between old and new information.
- Memory-enhanced architectures that allow models to retain valuable past experiences while acquiring new knowledge.
Fostering a modelβs ability to learn without compromising essential skills or knowledge remains a pressing challenge in the field. Solutions to this problem are still evolving, and research is ongoing.
Ethical and Security Concerns
As with any technology, ethical and security concerns are paramount when implementing continuous learning in AI systems. The continuous nature of learning introduces unique risks. For instance, the reliance on newly generated data can lead to unintended consequences, such as:
- Bias propagation: If new data introduces biases, the model may inadvertently exacerbate these issues.
- Privacy violations: Learning from sensitive data without adequate safeguards can lead to breaches of confidentiality.
- Accountability: As models evolve, tracing back decisions to a specific data point becomes increasingly complex, raising questions about accountability.
Organizations must implement strict ethical standards and security protocols to mitigate these risks. Developing transparent models that can explain their learning processes and decisions is essential in fostering trust in AI systems.
Continuous learning is a double-edged sword, offering immense potential while also presenting significant challenges that must be navigated with care.
The challenges in implementing continuous learning are significant yet surmountable with robust frameworks and innovative solutions. By understanding and addressing these issues, practitioners can enhance the reliability and effectiveness of AI technologies.
Applications of AI Continuous Learning
AI continuous learning has a vast array of applications that significantly enhance knowledge acquisition across various fields. As artificial intelligence evolves, its ability to adapt to new information without requiring complete retraining becomes increasingly valuable. This capacity not only increases efficiency but also leads to deeper insights and improved decision-making processes in real-world scenarios. To understand its impact effectively, we will explore key areas of application, including healthcare innovations, autonomous systems, and recommendation systems.
Healthcare Innovations
In healthcare, AI continuous learning transforms patient care and operational efficiency. Machine learning models can analyze patient data continuously, learning from new case studies, outcomes, and treatment protocols. This ongoing learning process means that healthcare providers can offer personalized treatment plans that adapt to individual patient needs over time.
For example, AI systems can monitor a patientβs vital signs and adapt their analysis based on daily data collection. If a patient shows unusual symptoms, the AI can modify its algorithms to prioritize specific risk factors or potential conditions. This allows healthcare professionals to respond more quickly to emergent health issues, potentially saving lives.
The benefits of applying continuous learning in healthcare are numerous:
- Improved Diagnostics: Machines become increasingly adept at diagnosing conditions through pattern recognition, leading to earlier interventions.
- Resource Allocation: Continuous learning systems optimize the use of resources in hospitals, ensuring that staff and equipment are deployed most effectively.
- Research and Development: AI can help speed up medical research by continuously integrating new findings into existing databases, enabling quicker advancements in treatments and medication developments.
Autonomous Systems
Autonomous systems, such as self-driving cars and drones, benefit immensely from AI continuous learning. These systems rely on real-time data processing and decision-making to navigate complex environments. Continuous learning allows them to incorporate new experiences and situations into their operational frameworks, increasing their reliability and safety.
For instance, a self-driving vehicle uses data from previous trips to enhance its travel route decisions. It learns about traffic patterns, prefers certain paths, and identifies obstacles. As it collects more data, it can make better predictions and adjustments on the road, improving passenger safety and efficiency over time.
Significant advantages include:
- Safety Enhancements: Continuous learning systems can identify and react to potential hazards faster by recognizing patterns in driver behavior or road conditions.
- Operational Efficiency: These systems can continually optimize their routes, reducing fuel consumption and travel times.
- Adaptability: Autonomous technologies can adjust to localized driving rules and conditions based on the region, proving crucial for deployment in different countries.
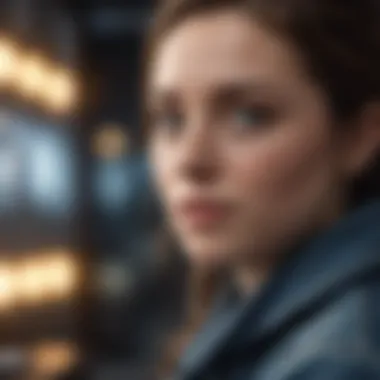
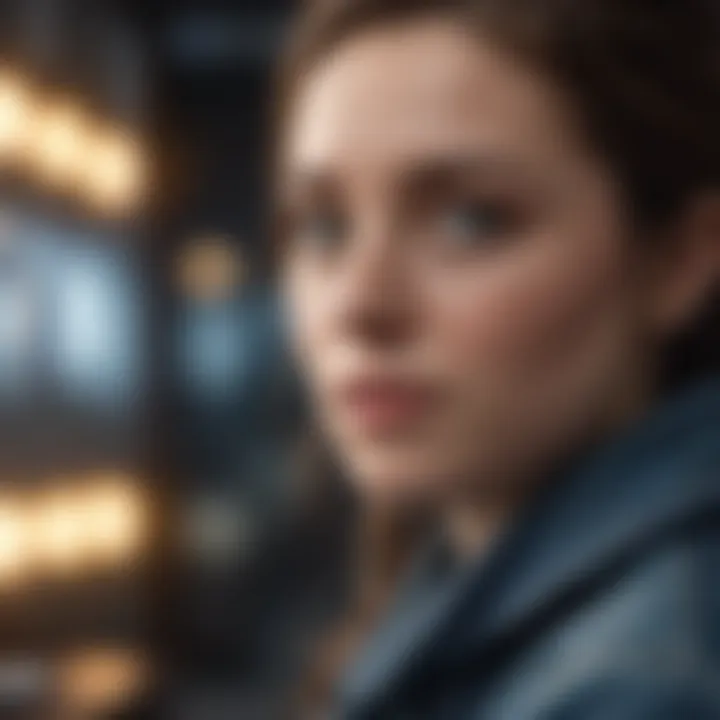
Recommendation Systems
Recommendation systems, widely used by platforms like Netflix and Amazon, enhance user experience through continuous learning methodologies. These systems analyze user behavior and preferences, evolving their suggestions based on new data points. As more feedback is gathered from users, the recommendations become increasingly relevant and tailored.
For instance, Netflixβs recommendation algorithm uses continuous learning to refine its suggestions based on user interactions. If a user starts watching a new genre, the system can quickly adapt its recommendations, introducing similar content that may pique the viewerβs interest.
The benefits of continuous learning in recommendation systems include:
- Personalization: Users receive suggestions tailored specifically to their tastes, improving satisfaction and engagement rates.
- Dynamic Learning: The system adapts not only to individual preferences but also to shifts in global trends or consumer behavior quickly.
- Increased Engagement: By continuously improving the relevance of recommendations, platforms can keep users engaged longer and encourage subscriptions.
In conclusion, the applications of AI continuous learning across various domains demonstrate its transformative potential. From enhancing healthcare expertise to optimizing travel through autonomous systems and creating personalized experiences in digital platforms, continuous learning in AI is not only about efficiency but about providing improved, data-driven responses to complex human needs.
Future Directions of AI Continuous Learning
The exploration of future directions in AI continuous learning is crucial for understanding how these technologies will shape knowledge acquisition and usage. With the rapid pace of innovation in artificial intelligence, staying abreast of emerging trends becomes paramount. Continuous learning systems possess the unique ability to evolve over time, adapting seamlessly to new information and challenges. This feature enhances their relevance and applicability across various fields, ensuring that they remain effective tools for knowledge management and decision making.
Research Trends and Innovations
Research in AI continuous learning is steering towards novel methodologies and innovative practices. Here are several emerging trends worth noting:
- Meta-Learning: Techniques that enable machines to learn how to learn are gaining traction. This approach allows AI systems to optimize their learning processes based on past experiences.
- Federated Learning: This method enhances data privacy and security by enabling models to learn across decentralized devices, without needing to centralize sensitive data.
- Self-Supervised Learning: This area focuses on deriving supervisory signals from the input data itself, allowing models to learn from unlabeled data. It reduces the reliance on large labeled datasets, which is often a bottleneck in traditional learning.
- Online Learning: This involves continuously updating AI models as new data arrives. It contrasts sharply with traditional batch learning, promoting flexibility and responsiveness.
Research indicates that these innovations can lead to more robust and adaptable AI systems, which are essential for handling the complexities of real-world scenarios.
Integration with Other Technologies
The integration of continuous learning with other emerging technologies can further enhance its capabilities. This synergy is visible in several areas:
- Internet of Things (IoT): Devices equipped with continuous learning algorithms can analyze data collected in real-time from numerous sensors. This integration can help optimize processes in industries like manufacturing, transportation, and smart cities.
- Blockchain: Combining AI continuous learning with blockchain can strengthen data integrity. AI can analyze transaction patterns while blockchain secures the data from unauthorized changes.
- Natural Language Processing (NLP): By merging continuous learning with NLP, systems can improve their ability to understand context and user intent over time, leading to more refined human-computer interactions.
The fusion of AI continuous learning with other technologies offers a path toward developing smart systems that can learn, adapt, and respond to the dynamic environment effectively.
The End: The Impact of Continuous Learning in AI
The discussion around AI continuous learning is vital to understanding the future implications of artificial intelligence. It signifies a shift from traditional learning paradigms toward a model where AI systems remain enabled to learn continuously. This is not only about improving performance but also about making AI adaptable, efficient, and aligned with real-world dynamics. Continuous learning broadens the scope of AI applications, enhancing decision-making processes and user experiences across multiple sectors.
This conclusion emphasizes the value of integrating continuous learning into AI systems. By enabling machines to learn from real-time data, businesses can respond promptly to changes within their respective environments. For several industries, this capability translates into significant competitive advantages. It fosters innovation, supports informed decision-making, and cultivates the ability to keep pace with rapidly evolving information landscapes.
Summarizing Key Insights
Several key insights emerge from the discussion on AI continuous learning:
- Adaptability: Continuous learning allows AI systems to adapt to new information and changes in their environment swiftly. This capability is crucial in fields like healthcare and finance, where keeping up with trends is necessary for effective outcomes.
- Efficiency in Training: Unlike traditional models that require extensive retraining, continuous learning focuses on incremental updates. This results in reduced computational costs and time-saving, enabling organizations to allocate resources more efficiently.
- Enhanced User Engagement: Applications like recommendation systems benefit from continuous learning, leading to more personalized experiences for users. Adaptive learning models fine-tune suggestions based on evolving user preferences.
"The ability of AI to continuously learn not only offers a pathway for improved accuracy but also creates solutions tailored to specific needs and conditions."
This synthesis highlights the transformative potential AI continuous learning presents to knowledge acquisition, necessitating further exploration of its capabilities and challenges.
Final Thoughts on Future Potential
The journey of AI continuous learning is still in its early stages. However, its future holds remarkable potential. As AI systems become more sophisticated, we anticipate greater integration with other advanced technologies, such as the Internet of Things (IoT) and blockchain. These synergies can enable richer and more informed decision-making frameworks, particularly in sectors like smart cities and automated logistics.
Furthermore, research continues to unveil new methodologies that address the challenges of continuous learning, such as data privacy and security issues. Ensuring robust ethical frameworks around data is imperative as systems gain access to sensitive information.
Continuous learning is likely to evolve within a context that respects privacy while fostering innovation. The balance between leveraging data for enhanced learning and ensuring rigorous ethical safeguards will play a crucial role in shaping the future trajectory of AI development.
In summary, AI continuous learning not only revolutionizes how machines process information but also cultivates a more adaptive and responsive approach to knowledge acquisition. As this field advances, it is poised to redefine our relationship with technology, paving the way for unprecedented growth and understanding.