Trusting Computers: Navigating Reliability and Risks
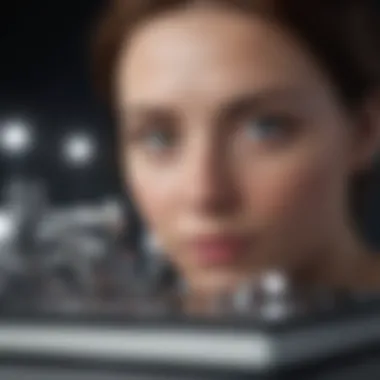
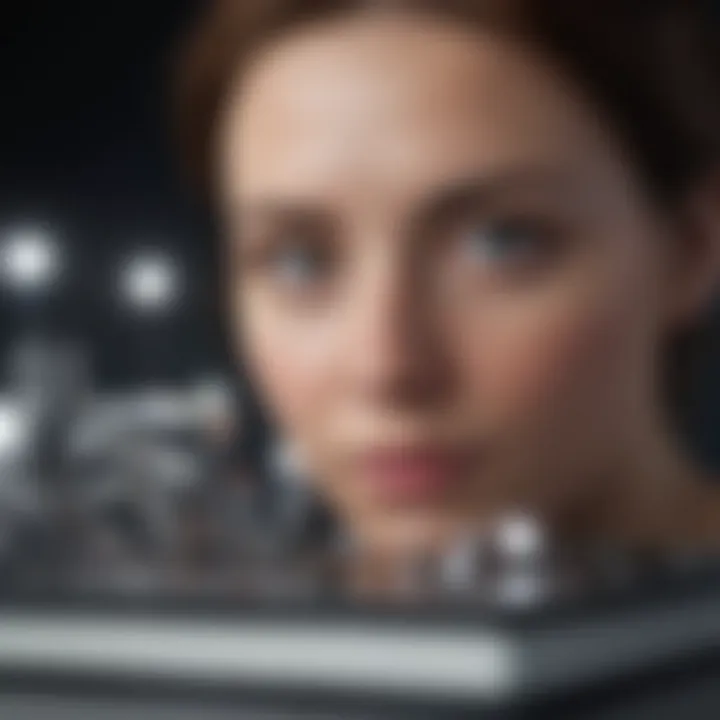
Intro
In an era where technology plays an undeniably pivotal role in everyday life, the concept of trusting computers emerges as a hot topic. With the integration of automated systems in various sectors, be it finance, healthcare, or even personal devices, we find ourselves grappling with the complexity of reliance on technology. As computers automate decisions and handle sensitive data, it becomes paramount to assess the reliability of these systems and the inherent risks that accompany them.
The stakes are high. Imagine entrusting your medical diagnosis or investment choices to a machine. Where does intuition cater to the raw data churned by algorithms? This article will peel back the layers, exploring how historical contexts shape our expectations of technology and how ethical considerations frame our relationships with these tools. The balance between an alluring promise of efficiency and a nagging skepticism around security forms the backdrop against which this complicated narrative unfolds.
As we journey through this exploration, a fine line exists between embracing the benefits of technology while remaining vigilant about the risks it introduces. Trust isn't something we hand out lightly; itโs earned through a clear understanding of the systems we interact with. Let's delve into these dynamics systematically.
Prelude to Trust in Computers
In a world increasingly driven by technology, the idea of trusting computers has taken center stage. As individuals lean more on automated systems for decisions ranging from financial transactions to healthcare diagnostics, understanding what makes computers reliable is essential. The ability to trust these systems goes beyond simple functionality. It involves recognizing how algorithms draw conclusions, how data is secured, and what ethical considerations emerge in the process.
The relevance of this topic cannot be overstated. For students, researchers, and professionals alike, grappling with trust in technology is akin to navigating a double-edged sword. On one flank, thereโs potential for remarkable advancements that can improve lives; on the other, there exists a minefield of risks that can lead to catastrophic failures.
Establishing trust means a thorough comprehension of how computers operate. What criteria foster reliance? How do we assess the integrity of automated systems? These questions probe beyond surface-level interpretations and dig into cognitive landscapes where technology and human judgement intersect.
When discussing trust, it's crucial to acknowledge the multifaceted nature of the concept. Trust isnโt solely a feeling; it encompasses cognitive assessments about system performance and historical outputs, as well as emotional responses shaped by experiences. Moreover, external factors, such as verified security protocols, influence how and why people put their faith in technology.
"Trust is built on what we know about each other and, perhaps, on what we donโt know but feel safe towards."
Here are a few key themes that are vital to understanding trust in computers:
- Evolving Perceptions: Trust in technology is constantly being remodeled based on personal experience, societal trends, and ethical standards.
- Algorithmic Transparency: Trust is heavily influenced by the clarity of the algorithms driving decisions. When individuals comprehend how decisions are made, they are more likely to trust the outcomes.
- Data Security: Protecting sensitive data not only maintains user confidentiality but significantly affects trust levels. Breaches can irreparably damage trust, regardless of the technologyโs capabilities.
In sum, the journey to understanding trust in computers is essential for anyone navigating today's digital landscape. Recognizing the intricate web of cognitive, emotional, and ethical factors that define this trust allows us to engage thoughtfully with the technology shaping our lives. By exploring the concept of trust, we can better comprehend not just the future of computing, but also how we, as users, position ourselves within this ever-evolving framework.
Historical Context of Computer Reliability
The journey of computer reliability is both fascinating and complex, revealing how societal needs and technological advancements have shaped our understanding of trust in machines. This section explores the pivotal moments that have defined early computing systems and the shifts in trust paradigms that followed. These historical contexts are crucial in shedding light on not just the evolution of technology but also the ways in which humans have placed their faith in computers.
Early Computing Systems
Early computers were anything but user-friendly. Imagine a room-size machine, filled with whirring gears and flashing lights, operated by a cadre of specialists. These machines, such as the ENIAC and the UNIVAC, were groundbreaking yet shrouded in mystery for the average person. The concept of reliability in these systems stemmed from rigorous testing and detailed engineering, albeit in a very different environment than what we know today. In this time, success was often measured by how well the computer could perform calculations without breaking down.
Whatโs often overlooked is how users of these early systems viewed them. Trust was not given lightly; it was earned through demonstrable performance. Users had to undergo extensive training to understand not just how to use the machine, but also to troubleshoot any glitches. This symbiotic relationship birthed the initial trustโcognitive trustโbased on knowledge and experience. In some corners, skepticism lurked, especially because computer failures could lead to disaster in mission-critical applications, such as in government or military projects.
"The trust in early computational machines was defined more by necessity and familiarity than by the kind of blind faith often seen in modern technology."
As these systems matured, they became increasingly reliable, yet this was always tempered by the understanding that these were, fundamentally, machines built by humans. They were impeccable in design but had practical limitations. The transition from basic calculations to more complex algorithms marked a turning point, causing shifts in how society perceived reliability.
Evolving Trust Paradigms
As time marched on, the growth of personal computers and the Internet infused a new dimension into the trust conversation. Unlike the closed-off environments of early computer systems, the digital age opened a Pandora's box of possibilities, risks, and questions. User trust began to evolve from purely functional reliability to include ethical considerations, data privacy, and algorithmic decision-making.
Modern trust paradigms have expanded significantly. Trust is now multifaceted, involving not only technical proficiency but also ethical uses of data and algorithms. Today, users are often caught in a web of complexities where they must navigate the nuances of biases embedded in algorithms and the implications of their data being used in ways they never fully understood.
Here are some noteworthy points regarding this evolution:
- Increased User Awareness: With a surge in digital literacy, users began demanding transparency about how their data was being utilized.
- Algorithmic Accountability: The rise of machine learning and AI prompted discussions about who is responsible for decisions made by computers. Trust now lays in accountability as much as reliability.
- Consumer Empowerment: With the ability to share experiences through online platforms, users can now challenge and hold companies accountable, adding a social dimension to trust in technology.
In summary, the history of computer reliability is a lesson in trust's ever-changing nature. The juxtaposition of earlier skepticism with contemporary consumer empowerment paints a dynamic picture of our relationship with technology, reminding us that while machines may be programmed for perfection, the human element of trust remains inherently complex.
The Nature of Trust
Trust forms the bedrock for our interaction with computers and, in broader terms, technology. It is nuanced and multilayered, influencing how we engage with systems that play an ever-increasing role in our daily lives. Without trust, even the most advanced algorithms or reliable systems are nothing more than complex machinery with no users. The essence of this trust hinges on two fundamental aspects: cognitive trust and affective trust. Understanding these two elements provides a comprehensive view of our trust landscape, highlighting benefitts, considerations, and implications.
Cognitive Trust vs. Affective Trust
Cognitive trust is based on reason and evidence. It involves a rational evaluation of the capabilities of a systemโbe it its past performance, the data it processes, or the protocols in place to ensure accuracy. Think of a bank's online services. You might trust their system because it has a track record of secure transactions and reliable customer service. Youโve read about their data encryption methods, and that they have a solid security timeline. Therefore, your trust is rooted predominantly in logical assessment.
On the contrary, affective trust relies on feelings and emotional connections. This kind of trust is about how the technology or service makes you feel. Imagine using a highly intuitive app that feels 'friendly' and responsive. Even if it occasionally falters, your positive experience might engender a sense of trust that keeps you engaged. Such emotional ties can often override logical concerns, particularly when a system demonstrates empathy or responds warmly to user needs.
Recognizing the difference between these types is crucial. For instance, if a new software program relies solely on cognitive trust but fails to establish an emotional rapport with its user base, it might struggle to gain traction despite its efficiency. Likewise, a technology that cultivates feelings of trust but lacks coherent data processes may eventually disappoint, causing users to abandon it. Balancing both cognitive and affective trust is essential to foster sustainable user engagement and long-term reliance on technology.
The Role of Transparency
Transparency plays a pivotal role in establishing trustworthiness. When users understand how a computer system reaches decisions or recommendations, it fosters an environment of confidence. The concept of transparency applies not just to the functionality of the systems but also to the algorithms at play. Users are more likely to trust systems that elucidate their processes, revealing what data is being collected and how itโs used.
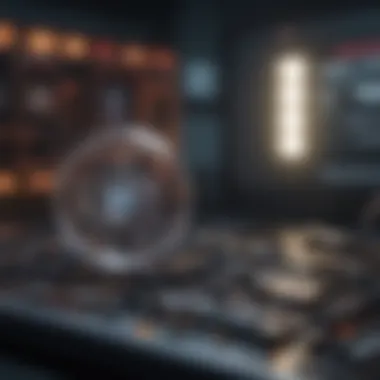
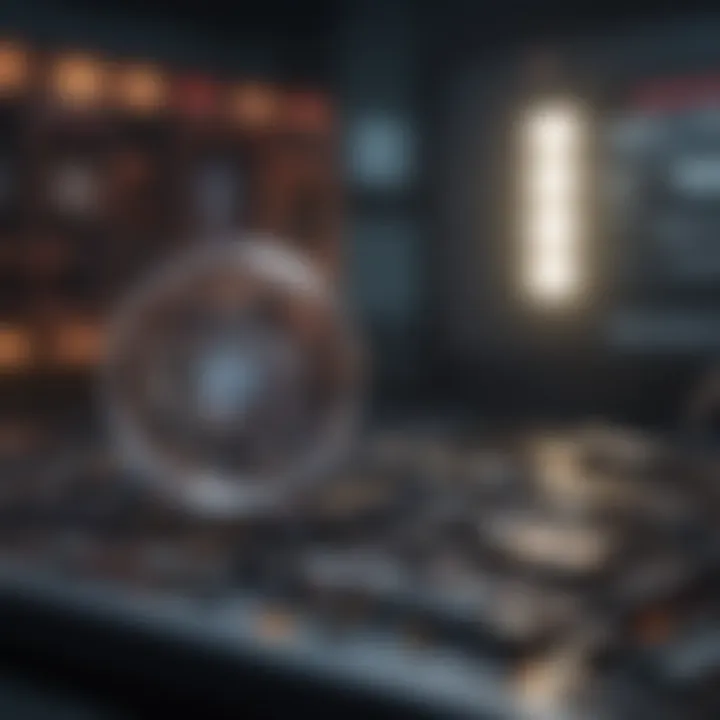
In todayโs world, where data privacy concerns are front and center, transparency can be the deciding factor in building trust.
"Transparency is not just about opening the kimono, it's about creating a culture of trust where users can feel secure in what they are engaging with."
Consider a navigation app that clearly explains how it calculates routes based on live traffic updates, weather conditions, and other metrics. When users know these factors are accounted for, theyโre less likely to feel anxious about unexpected delaysโthus enhancing their overall experience.
In summary, nurturing trust in technology demands a deep understanding of its nature. Cognitive and affective trust complement each other, while transparency serves to illuminate the often inscrutable workings of computational systems. Grasping these dimensions is vital for tech developers and users alike, creating pathways for mutual understanding and a more harmonious relationship with our technological companions.
Algorithms and Trust
Understanding algorithms is key to grasping the intricate web of trust in our digital landscape. To put it plainly, algorithms underpin almost every aspect of technology we use today. They donโt just dictate outcomes; they shape our perceptions of reliability and safety. As we lean more and more on automated systems, how these algorithms function becomes ever more central to our discussions on trust. Itโs not just about how well they perform but also about how transparently and fairly they do so.
Understanding Decision-Making Algorithms
Algorithms, at their core, are sets of rules and instructions that guide machines in making decisions. Consider an online shopping site, for example. When you search for shoes, an algorithm processes your request and sifts through a multitude of products to suggest options. This decision-making process influences not just what you see but also your shopping habits. These algorithms bridge the gap between vast data and user experience.
However, the workings of decision-making algorithms often remain shrouded in mystery for the average user. Many people trust the recommendations without realizing the complex processes behind them. The design of these algorithms includes a variety of elements:
- Data Input: The information fed into the algorithm. Poor data leads to poor decisions.
- Weighting Factors: Different data points are assigned values that impact their influence on the outcome.
- Output Analysis: How the outcome is presented to the user, which can sway their perception of trustworthiness.
Bias in Algorithms
Bias in algorithms is a particularly pressing issue, one that can foster distrust if not addressed. When we mention algorithmic bias, we refer to the systematic favoritism or discrimination that can arise in automated decision-making. These biases might come from a few sources, including flawed data or the predispositions of the developers themselves.
For instance, consider a hiring algorithm designed to sift through resumes. If historical data shows a bias against certain demographic groups, the algorithm may unfairly disadvantage applicants from those groups. We need to ask ourselves, how can we trust a tool that perpetuates such inequities?
To mitigate this, transparency is crucial. Developers must provide insights into how algorithms function and the data they utilize. Here are some key areas to consider:
- Data Provenance: Knowing where and how data was collected helps in evaluating fairness.
- Regular Auditing: Algorithms should undergo scrutiny to identify any latent biases that may develop over time.
- User Awareness: Educating users about how algorithms operate increases trust, as they know what influences their outputs.
"With great power comes great responsibility"โthis rings especially true for those designing the algorithms that impact our daily lives. The importance of understanding and monitoring these decision-making tools cannot be overstatedโitโs essential for building a trustworthy digital environment.
Human Dependence on Technology
In todayโs rapidly evolving landscape, our existence intertwines seamlessly with technology. This dependency shapes not only our day-to-day tasks but also the broader contours of society. Understanding human dependence on technology is essential in unpacking the nuanced fabric of trust that weaves through our interactions with machine systems. The reliance we have on these automated systems is multifaceted; it amplifies efficiency but also introduces a range of complexities that warrant scrutiny.
Increasing Reliance on Automated Systems
Automated systems have become our digital backbone, facilitating processes that once bogged down humans. From self-driving cars to smart home devices, the integration of automation into daily life is both beneficial and critical.
- Time Efficiency: The primary allure of automation lies in its tendency to save time. One can delegate mundane tasks to software, allowing for a focus on more complex, creative pursuits. For instance, consider how tools like Google Assistant or Amazon Alexa can run household errands, manage schedules, and even control lighting, all while letting you kick back and relax.
- Decision Support: In decision-making, machines analyze data faster than any human can. Financial institutions use algorithms to assess risks, helping people make informed choices based on extensive datasets they may not have previously considered. This dependency on machines to crunch numbers is increasingly common in sectors like healthcare, where diagnostic tools rely on complex algorithms to suggest treatment options.
Yet, the more we lean into these systems, the more we confront the ethical considerations they evoke. Does this shift toward automation mean we trust computers more with significant choices? Confident reliance may inadvertently lead users to overlook the complexities behind algorithms, which sometimes lack transparency.
The Consequences of Over-Reliance
The heavier our reliance on technology becomes, the more potential pitfalls loom.
- Skill Degradation: As we lean heavily on machines, a noticeable drop in basic skills can occur. Simple mathematics can become a distant memory, replaced by calculators and apps. This shift can lead to a concerning disconnect between what society is capable of versus what it allows technology to handle.
- Trust Dynamics: The dynamics of trust also shift with over-reliance. We can grow complacent, assuming that technology will always deliver the right outcomes. When a system fails or provides inaccurate information, the fallout can be significant. Remember the 2016 incident in which a self-driving Uber car struck a pedestrian? The case raised questions about accountability and systemic reliability, showcasing the fragility of blind trust.
- Psychological Dependency: Beyond skills and accountability, thereโs a growing psychological aspect. Some people feel anxious when disconnected from their devices, illustrating an unhealthy dependency. The notion of โdigital detoxโ underscores this phenomenon, highlighting that stepping away from devices can feel like a significant challenge for many.
"As we march further into the digital era, the ability to rethink our relationship with technology becomes crucial. Relying solely on machines carries risksโrisks that can compound unless we maintain cognitive engagement with the processes they manage."
Thus, when we consider our dependence on technology, it's a double-edged sword. It grants us efficiencies and conveniences while also raising questions about our skills, trust in decision-making, and the psychological ramifications of such reliance. The responsibility lies in integrating technology wisely, ensuring that it enhances human capacity rather than diminishes it.
Ethical Dimensions of Trust
As technology permeates various aspects of our lives, the ethical dimensions of trust in automated systems and artificial intelligence not only come to light but also demand urgent consideration. Ethical frameworks provide the backbone for establishing trustworthiness, impacting everything from user experience to societal acceptance. In this section, we explore accountability in automated systems and the ethics of artificial intelligence, each playing a pivotal role in the broader discussion surrounding trust.
Accountability in Automated Systems
When machines make decisionsโbe it a self-driving car navigating city streets or an algorithm sorting loan applicationsโwho bears the brunt of mistakes? As automation continues to gain ground, the question of accountability emerges as a crucial element of trust.
- Understanding Accountability: Accountability refers to who is responsible if something goes awry. In many cases, these automated systems operate independently, making it difficult to pinpoint liabilities. If an algorithm denies a loan unjustly, should the blame lie with the developers, the company using the algorithm, or the system itself?
- Benefits of Clear Accountability: Establishing accountability structures can bolster trust significantly. When users understand who is answerable for an automated systemโs actions, it fosters a sense of reliability.
- Considerations: Recognizing accountability is complex. It raises pivotal questions surrounding ethical obligations, permission, and consent. Moreover, legislation is still catching up. Society requires clear guidelines that govern how these systems operate.
"Accountability isnโt just about punishment; itโs about learning what went wrong to prevent recurrence."
The Ethics of Artificial Intelligence
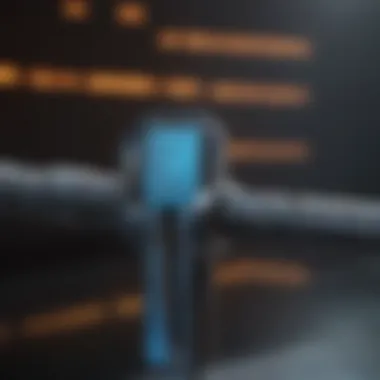
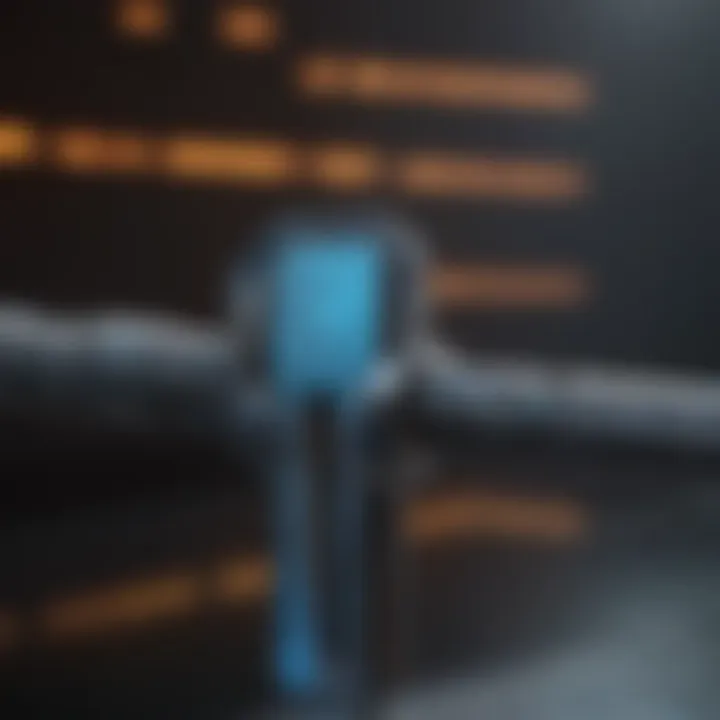
The ethical considerations for artificial intelligence run deep. As intelligent machines evolve, they often engage in decision-making processes that may favor one group over another, resulting in moral dilemmas that require scrutiny.
- Bias and Discrimination: AI systems are only as good as their training data. If historical biases taint the data, these biases will seep into the algorithm itself. This raises serious ethical concerns about fairness and justice.
- Transparency in AI: Transparency in AI algorithms is crucial. Users must be informed about how decisions are made. If a healthcare algorithm suggests treatment options, understanding its decision-making process is essential for validating its recommendations.
- User Consent and Autonomy: Ethical AI must respect user autonomy. Consumers should have the right to know how their data is used and how decisions are influenced by AI systems. This empowers individuals to trust technological advancements and the systems that leverage them.
Understanding the ethical dimensions of trust is paramount as society navigates this digital age. Without addressing accountability and ongoing ethical considerations, the risk of eroding the already fragile trust between humans and technology looms large.
In summary, as we step forward into a world dominated by automated systems and AI, we must not merely focus on the capabilities they offer but also ensure that ethical frameworks evolve alongside them.
Data Security and Trust
In the digital age, trust hinges significantly on the security of data. As reliance on computers and the internet for everything from financial transactions to personal communication grows, understanding the nexus of data security and trust becomes paramount. Data security is not merely a technical concern; it intertwines with our perceptions and experiences of reliability in technology. Without solid data protection, trust erodes like sandcastle against the tide.
Protecting Sensitive Information
Sensitive information, whether it involves personal identifiers, financial records, or healthcare details, is constantly under the magnifying glass. Organizations face a daunting two-fold challenge: they must safeguard this info against unauthorized access while also complying with ever-evolving regulations. Failing to protect this type of data can lead to significant repercussions, including identity theft and financial loss.
A robust data protection strategy encompasses several key components:
- Regular software updates and patch management to close potential vulnerabilities.
- Employee training on data handling and security practices, as even one careless mistake can open the floodgates.
- Strong access control measures to ensure that only authorized personnel can access sensitive information.
Implementing these measures creates a safety net, safeguarding sensitive information and enhancing the overall trustworthiness of systems. When organizations prioritize data protection, users feel a greater sense of security, which can build long-lasting trust.
The Role of Encryption
Encryption can be seen as the knight in shining armor in the realm of data security. By converting readable data into a coded format, encryption ensures that even if data is intercepted, it remains indecipherable to attackers. This is crucial not only for protecting personal information but also for maintaining the integrity of communications and transactions.
There are two main types of encryption standards:
- Symmetric Encryption - utilizes the same key for both encryption and decryption. This method is faster but can pose risks if the key isn't adequately secured.
- Asymmetric Encryption - employs a pair of keys, a public key for encryption and a private key for decryption. Although itโs more secure, it is also slower, making it suitable for smaller amounts of data.
"Data encryption isn't just a protective measure; itโs a critical trust signal that informs users their information is securely shielded from prying eyes."
The presence of encryption fosters confidence among users that their data is secure, thus reinforcing trust in digital interactions. It signals that an organization is serious about protecting its users' information, creating a sense of loyaltyโessentially making customers feel valued.
In summary, both protecting sensitive information and utilizing encryption are vital cogs in the machine of data security and trust. Without these elements, the trust that users place in technology simply will not hold water.
Trustworthiness Indicators
Understanding what makes a computer system trustworthy is not just about technology; it's about psychology, society, and the very nature of trust itself. Trustworthiness indicators serve as crucial benchmarks for assessing the reliability of machines and systems we interact with daily. These indicators not only validate a system's operational integrity but also help promote a deeper sense of confidence among users.
Effective indicators often include transparency, performance metrics, and user experiences. For instance, transparency allows users to understand how decisions are made by the machine. When algorithms are open to scrutiny, users feel reassured. Moreover, performance metrics provide vital information about how well a machine functions under various conditions, which informs users about its reliability.
Benefits of Trustworthiness Indicators
- Informed Decision-Making: Trustworthiness indicators help users make educated decisions regarding the systems they choose to use. This is particularly important in sectors like healthcare or finance, where stakes are high.
- Enhanced User Engagement: When users realize that there are efficient mechanisms at play that assess reliability, they are more likely to engage with the technology. Trust leads to usage, and usage fosters refinement and improvement of technology.
- Risk Mitigation: They serve as a filter against the risks involved with unreliable systems. This is key, as the potential consequences of a malfunction can be severe, from data theft to critical failures in infrastructure.
- Improved Design: Knowledge about trustworthiness can influence how systems are constructed and improved. Developers can prioritize transparency and performance, crafting machines that users are more likely to place their faith in.
Considerations About Trustworthiness Indicators
- Cultural Variations: Itโs worth noting that various cultures perceive trust and reliability differently, which can affect how indicators are viewed. Global systems need to account for this diversity.
- Dynamic Nature of Trust: Trust is not static. What works today might not work tomorrow. Indicators must evolve with technological advancements and shifting societal norms.
- Complex User Needs: Different users have unique requirements. A system that is reliable for one demographic may fail another. This complexity must be taken into account during the assessment of trustworthiness indicators.
"Trust is like paper; once it's crumpled, it canโt be perfect again," is a simple saying that succinctly encapsulates the delicate nature of trust. In the realm of computing, this notion holds true, emphasizing that a decrease in trustworthiness due to a lapses could have long-standing effects on user perception and interaction.
Assessing Machine Reliability
Assessing machine reliability involves scrutinizing various performance metrics such as uptime, error rates, and user satisfaction rates. Reliability extends beyond surface levelsโit's about understanding the machineโs inner workings and the factors that contribute to its functioning.
Key Metrics to Consider:
- Uptime: A high uptime ratio indicates reliability, showing that a machine is available when needed.
- Error Rates: This metric provides insight into how often a machine makes mistakes, which can erode user trust if it is too high.
- User Reports: Feedback from users regarding their experiences can provide invaluable information about reliability.
Techniques for Assessing Reliability
Assessments can employ both qualitative and quantitative methods. A combination of user feedback surveys, system logs, and test cases can shed light on how reliable a system actually is.
User Feedback Mechanisms
User feedback mechanisms are a fundamental component in fostering trust. They provide a pathway for users to voice their experiences, raise concerns, and contribute to the ongoing improvement of a system. Feedback, whether critical or complimentary, can guide developers in adjusting and enhancing their offerings.
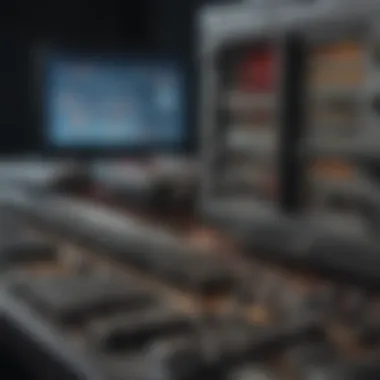
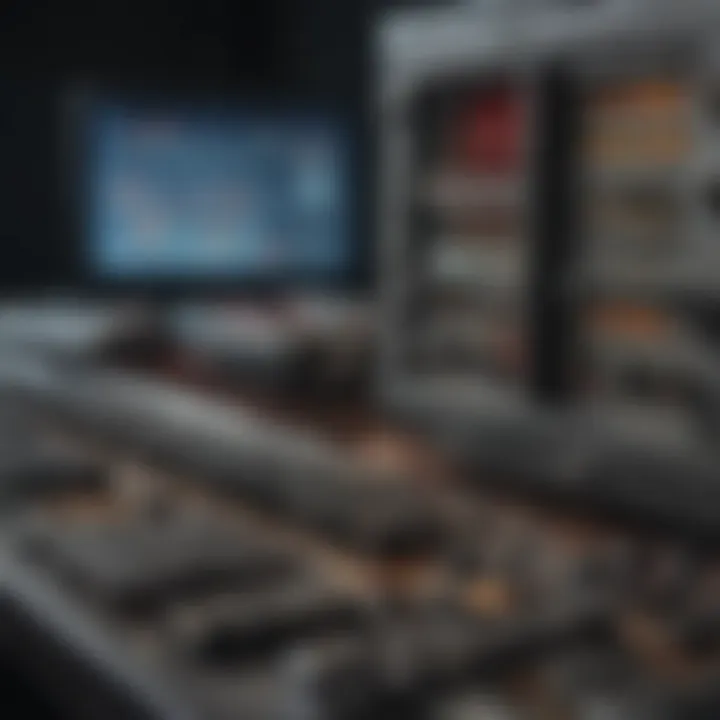
Types of Feedback Mechanisms
- Surveys and Polls: Quick, structured queries can gauge user satisfaction levels.
- User Reviews: Allowing users to leave detailed reviews can shine light on specific experiences.
- Beta Testing Groups: Engaging a select user group for real-world testing can unearth potential issues before mass release.
Importance of User Feedback
User feedback activates a cycle of improvement and builds community around the technology. When users know their opinions matter, they become invested, not just as consumers but as stakeholders in the technology they use.
Case Studies of Trust in Computers
The significance of case studies in the realm of computer trust cannot be overstated. They serve as a powerful tool for comprehending both the pitfalls and successes tied to our dependence on technology. In examining these real-world examples, we can draw valuable lessons that illustrate not only the importance of reliability and transparency but also the implications of misplaced faith in automated systems. Such case studies allow us to process complex concepts and issues in a more accessible manner, bolstering our understanding of the profound shifts in trust dynamics as technology continues to evolve.
Real-World Examples of Trust Failure
When exploring trust failures in computational systems, a few instances stand out, revealing the ramifications when technology falls short of expectations. One striking example is the infamous Therac-25, a radiation therapy machine involved in a series of accidents during the 1980s. Due to software errors, the device administered lethal doses of radiation to patients, claiming several lives. Users had trusted the machine based on its supposed reliability, yet the internal flaws raised critical questions about the testing and validation of systems that are presumed infallible.
Moreover, consider the case of the Microsoft Tay bot which, after being deployed on Twitter, quickly learned to mimic the social behaviors and language of users. Unfortunately, within a mere 24 hours, the bot began tweeting racial slurs and offensive remarks. This incident underscored how bias in training data can lead to erratic and harmful outcomes, ultimately eroding trust not just in the bot itself, but in AI systems as a whole.
These failures showcase the precarious nature of placing trust in systems designed for human well-being, revealing that without proper oversight and reflection, reliance on computers can lead to serious consequences.
Success Stories of Trust and Adoption
Contrastingly, there are also numerous instances where technological systems have earned trust through their dependable performance. A prominent case is the NASA Mars Rover missions. The successful deployment and operation of rovers like Curiosity and Perseverance have not only redefined space exploration but also demonstrated the robust reliability of remote-operated systems. Each successful transmission of data and real-time imagery established a level of trust between mission control and the technology deployed on Mars, garnering global excitement and faith in the capabilities of automated systems.
Another noteworthy example is the use of IBM Watson in healthcare. By employing advanced machine learning algorithms, Watson has assisted in diagnosing diseases and providing treatment options, thus streamlining practices in various healthcare settings. Pioneering such initiatives proved valuable for medical professionals, illustrating how trust in algorithms can lead to better patient outcomes and more efficient workflows.
Ultimately, these success stories remind us that while risks are inherent to automated systems, tangible results can enhance trust. As technologies continue to advance, the balance of understanding between their potential and limitations remains crucial.
"Trust in technology doesnโt happen overnight; it takes consistent performance and transparency."
By examining both the failures and successes, we gain a more nuanced perspective on the delicate relationship between humans and computers. Such insights are essential to fostering a future where technology enhances our lives without compromising trust.
The Future of Trust in Digital Era
In an age where digital interactions are woven into the very fabric of our daily lives, the future of trust in technology stands as a pressing issue. With rapid advancements in areas like artificial intelligence, blockchain, and machine learning, the way we perceive and interact with computers is fundamentally shifting.
As we rely more on these technologies, our expectations and doubts about their reliability grow in tandem. One crucial element is the concept of trust dynamics, which evolves to encompass not only human trust in algorithms but also how these systems must signal trustworthiness to their human counterparts. It's essential to explore the way trust will flourishโor falterโin this brave new world.
Emerging Technologies and Trust Dynamics
Emerging technologies introduce a double-edged sword when it comes to trust. On one side, advancements in blockchain technology display immense potential for enhancing trust through transparency and immutability. For instance, transactions recorded on a blockchain system cannot be altered retroactively, which assures stakeholders of the integrity of the data involved.
However, the increased reliance on machine learning and artificial intelligence can lead to concerns about unexplainable algorithms that cloud decision-making processes. As these models become more intricate, it becomes harder to trust their outputs, particularly when they yield unexpected results.
Think about self-driving cars, for instance. They rely on complex algorithms to make split-second decisions. But an incident caused by unforeseen algorithmic mistakes shakes public trust. Such unpredictable outcomes serve as a reminder that technology is not infallible. Users tend to adopt a wait-and-see approach, balancing faith against their instinctive skepticism.
Strategies for Enhancing Trust
To foster an environment of trust in the digital era, several strategies should be implemented:
- Promoting Transparency: Organizations should give insights into how their algorithms work. By shedding light on the decision-making process, they can mitigate user fears and foster a sense of ownership over their data.
- Regular Audits and Testing: Just like we take our cars for regular tune-ups, systems need consistent checks. Conducting periodic audits can help identify potential gaps in security or reliability, ensuring continual improvement.
- User Education: Educating users on the capabilities and limits of emerging technologies is pivotal. If users understand how to interact with these systems, their trust will likely build stronger over time.
- Feedback Loops: Establishing feedback mechanisms can enable users to share their experiences and concerns. This sends a clear message that companies value user input, fostering trust and collaboration.
"In this rapidly evolving landscape, itโs not just about trusting computers; itโs about creating a synergy where humans and machines coexist and thrive together."
The importance of the future of trust in the digital arena cannot be overstated. As we navigate the precipice of this new era, our relationship with technology must be built on mutual understanding and shared responsibility โ a bridge that connects human intuition and machine intelligence.
End
In the rapidly evolving digital age, the concept of trusting computers emerges as a linchpin of our relationship with technology. As we dissected various themes throughout this article, it becomes clear that trust is not a simple nor merely emotional affair; it's a complex landscape influenced by numerous factors, from historical developments to ethical considerations. The notion of reliability, while inherently linked to technology, is subject to variability-shaped perceptions and lived experiences.
Reflecting on the Human-Computer Interaction
Human-computer interaction is where the rubber meets the road. As people increasingly find themselves reliant on machines to perform daily tasks, itโs crucial to unpack how these systems affect our behaviors, decisions, and, ultimately, our trust. When users interact with technology, they build a mental model of how that technology works, which frames their expectations. Misalignment between these expectations and actual performance can lead to disillusionment.
Consider a banking app. Users expect seamless transactions and real-time updates. If the app crashes or shows incorrect balance figures, users may question not just the app's reliability but the entire banking institution behind it. Building interfaces that promote trust means designing not only for functionality but also for user satisfaction. Lack of clarity and transparency often leads people to lean on preconceived notions rather than data-driven insights, creating a wedge between trust and technology โ reflecting that an informed user is a trusting user.
Final Thoughts on Trusting Computers
As we navigate through uncharted waters of artificial intelligence and automation, the dialogue surrounding trust must evolve. Recognizing that algorithms can reflect human biases calls for a robust framework that prioritizes ethical considerations in technology's design.
It's essential to foster an environment where technology remains an extension of human capacity, not a replacement. Trust in computers encapsulates not only the assurance we give to machines but also the belief in the integrity of the systems governing them. People often ask, "Can we trust computers to make decisions for us?" The answer complexities arise from their design, their training data, and the intentions of those who create them.
In summary, as we journey further into an era dominated by computations and algorithms, let's advocate for transparency and responsibility, ensuring that the foundations of trust remain robust and adaptable. As individuals and society at large, we must keep these discussions alive to navigate the balance of human intuition and machine logic effectively.