Quantum Computing and AI: A New Frontier
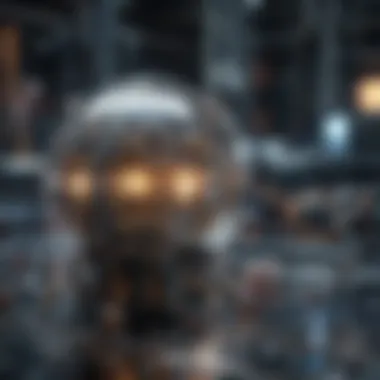
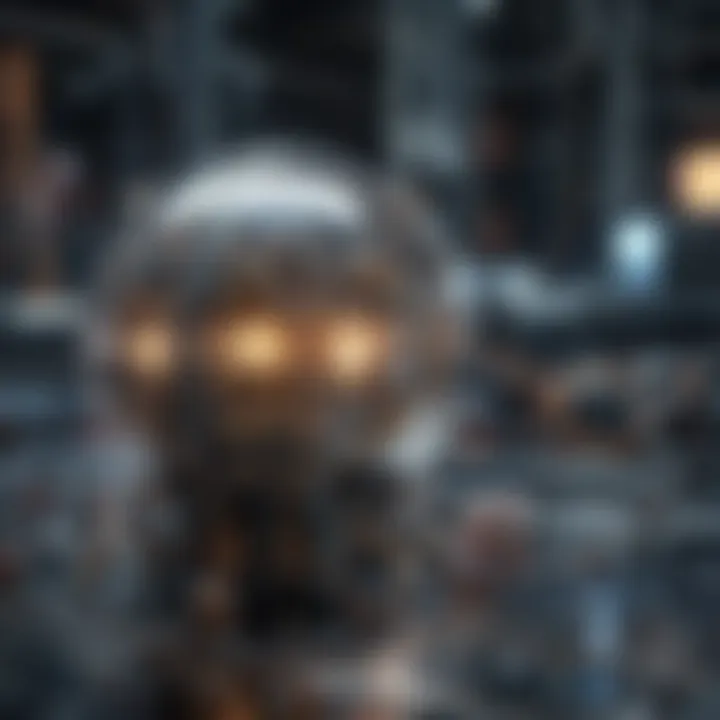
Intro
In today's rapidly evolving tech landscape, the fusion of quantum computing and artificial intelligence stands not just as an intersection of disciplines, but as a crucible for potential breakthroughs that could reshape industries. This compelling relationship between quantum mechanics and machine learning is not merely theoretical; it opens up promising avenues for enhancing data processing capabilities and improving algorithmic efficiency. By grasping the nuances between these two powerful domains, we can better appreciate their collective impact on the future of technology.
Key Research Findings
Overview of Recent Discoveries
Recent advancements in both fields have sparked a flurry of research aimed at harnessing quantum properties to bolster AI. For instance, researchers at Google and IBM have made significant strides in utilizing quantum systems to optimize machine learning tasks. These explorations delve into how quantum algorithms can potentially tackle problems such as pattern recognition and data classification with unprecedented speed. One particularly noteworthy discovery involves the quantum version of the k-means clustering algorithm, which outperforms its classical counterpart in certain scenarios, propelling researchers to investigate further.
Significance of Findings in the Field
The implications of these findings stretch far and wide. The ability of quantum systems to process complex data sets exponentially faster than traditional computers means that AI developers can create more sophisticated models that can learn and adapt at a quicker rate. This can have a transformative effect on sectors ranging from healthcare, where faster diagnoses can be achieved, to finance, where rapid anomaly detection can improve security measures. The importance of these breakthroughs cannot be emphasized enough; they could very well pave the path to quantum-enhanced efficient decision-making tools.
"In the foreseeable future, we may find ourselves leveraging quantum-enhanced AI tools to address some of humanity's most pressing challenges."
Break Down of Complex Concepts
Simplification of Advanced Theories
Understanding the interplay between quantum computing and artificial intelligence does not have to be reminiscent of diving down a rabbit hole. At its core, quantum computing utilizes qubits, which differ from classical bits. While a classical bit can be either 0 or 1, a qubit can exist in superpositions of both states. This property allows quantum computers to explore multiple possibilities simultaneously, making them potentially more powerful for certain types of computations, particularly those that can benefit from parallel processing.
In terms of AI, machine learning models can leverage the computational advantages provided by quantum systems. For example, optimization problems, which often appear in training AI models, can be solved more efficiently by quantum-enhanced algorithms. This harmonization of technologies presents an appealing proposition in both theoretical models and real-world applications.
Visual Aids and Infographics
To aid in understanding, visual representations of these concepts can be invaluable. Infographics demonstrating the differences between classical and quantum computing processes can facilitate comprehension. Diagrams illustrating how quantum entanglement works or how qubits operate in superposition would provide clarity and deepen understanding. Such resources not only illuminate the subtleties of quantum theory but also clearly connect them to practical applications within AI.
Prelude to Quantum Computing and AI
As the digital world evolves, the intersection of quantum computing and artificial intelligence (AI) emerges as a landscape ripe with possibilities. Understanding this interplay is more than just satisfying academic curiosity; it unveils the potential for transforming industries and enhancing decision-making capabilities. Quantum computing offers a radically different paradigm of processing information, leveraging the peculiarities of quantum mechanics to execute complex calculations at rates unimaginable with traditional systems. Meanwhile, AI stands on the precipice of becoming smarter and more efficient as it incorporates advancements from quantum technology.
Why This Matters
The pertinence of this topic cannot be overstated. Quantum computing has the ability to crack problems that are currently intractable for classical computers. Imagine being able to analyze vast datasets in healthcare or finance in seconds, rather than years. This could lead to breakthroughs in drug discovery or fraud detection, elevating AI's role in sectors where decision-making is both critical and time-sensitive.
The implications also stretch into addressing societal challenges, such as climate change and resource management, where AI can optimize resources and predict outcomes if powered by quantum enhancements. Exploring how these two fields complement and bolster each other may provide answers to some of the toughest puzzles modern technology faces.
"The next wave of technological advancement will come from the convergence of quantum computing and artificial intelligence, leading us into an era with transformational capabilities."
Integrated Insights
Delving into the definitions, foundations, and current trends surrounding both quantum computing and AI will illuminate their boundaries and overlap. It is essential to observe not only the theoretical aspects but also the practical applications these technologies promise. As the world leans more on AI, understanding how quantum computing can refine these algorithms is not just beneficial but necessary.
By understanding quantum mechanics' principles and how they contrast with classical computing alongside a comprehensive overview of AI's current capabilities, we begin to see a more integrated vision of the future. This narrative will shape much of the exploration that follows, where we unravel how each component feeds into and enhances the other, providing a clearer lens through which to view the future of technology.
Foundations of Quantum Computing
Understanding the foundations of quantum computing is like laying the cornerstone for a great architectural feat; without a solid base, the entire structure may wobble. Quantum computing stands apart from classical computing by employing principles from quantum mechanics, and it's vital to grasp these concepts to appreciate the full impact they can have on artificial intelligence. This section explores three key components: quantum bits, entanglement, and quantum algorithms. Each element not only contributes to building a powerful computational model but also highlights the potential benefits and considerations that come with integrating quantum technology into AI systems.
Quantum Bits and Superposition
At the heart of quantum computing lies the quantum bit, or qubit. Unlike classical bits, which exist in a state of either 0 or 1, qubits can exist in multiple states simultaneously due to a phenomenon known as superposition. Imagine spinning a coin; while in the air, it's neither heads nor tails but something in between. This capacity for being in several states at once allows quantum computers to process vast amounts of information far more efficiently than classical computers, capable of solving problems that would take years, if not centuries, on traditional systems.
"The real power of quantum computing comes from its ability to handle complex problems with quantum entanglement and superposition, transforming the way we think about computation and its applications in AI."
By leveraging superposition, quantum systems can explore multiple solutions at the same time. For machine learning tasks that require sifting through massive datasets, this means that pattern recognition and data analysis could advance at breakneck speeds. As researchers uncover more about qubits, the ability to encode, manipulate, and read these qubits will further facilitate breakthroughs in AI performance.
Entanglement and Quantum Gates
Next up is entanglement, a peculiar quantum phenomenon where the states of two or more qubits become intertwined. When qubits are entangled, the state of one immediately impacts the state of the other, no matter the distance between them. This unique property allows quantum computers to perform complex calculations with remarkable efficiency since operations on one qubit can instantaneously influence others that are entangled with it.
Moreover, quantum gates serve as the basic building blocks for quantum circuits, analogous to logic gates in classical computing. These gates manipulate qubits through various operations, such as flipping their states or creating entanglements. By strategically applying these gates, quantum algorithms can be constructed to solve problems or analyze data in ways that classical algorithms simply cannot achieve.
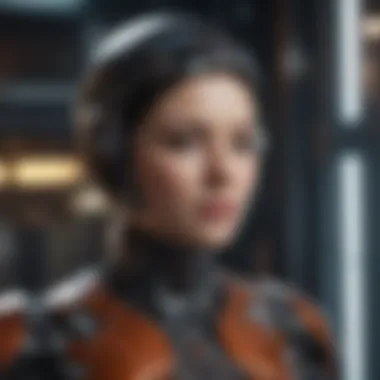
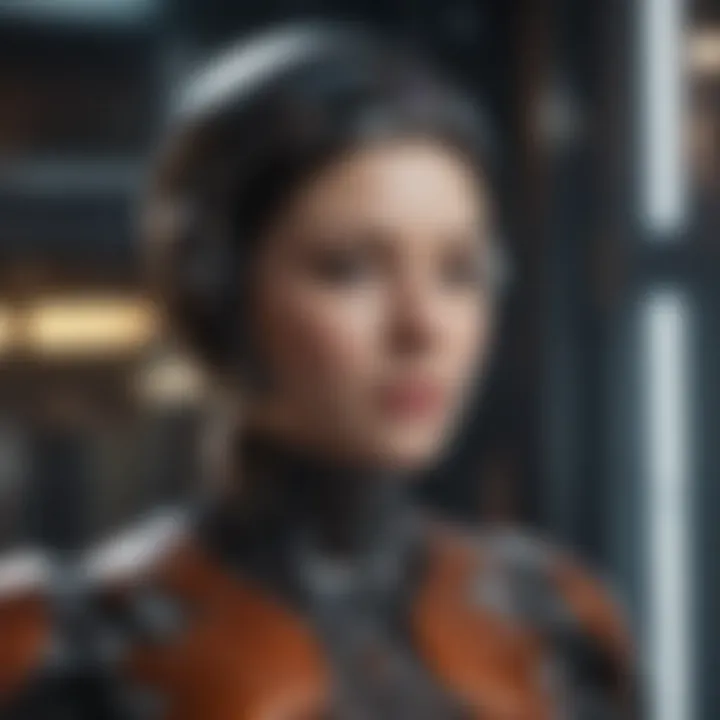
Quantum Algorithms: Shor’s and Grover’s
Now let’s delve into quantum algorithms, which are game-changers in the field. Two algorithms that stand out are Shor’s and Grover’s algorithms. Shor’s algorithm breaks down the large numbers that serve as a backbone of encryption systems, rendering classical encryption methods outdated. This has wide-reaching implications for cybersecurity and safe data sharing, which are vital for AI applications that handle sensitive information.
On the other hand, Grover’s algorithm excels at searching unsorted databases, providing a quadratic speedup over classical search algorithms. This proficiency can aid machine learning by allowing quicker access to relevant information in large datasets, enabling faster training and more accurate models.
The importance of grasping these foundational concepts cannot be overstated; as the integration of quantum computing and artificial intelligence matures, understanding these principles will be crucial for harnessing their full potential. In a nutshell, quantum bits and their unique properties, the phenomenon of entanglement, and innovative quantum algorithms form the core of what will likely revolutionize computational tasks in AI, leading to faster, more efficient, and more powerful systems.
As we forge ahead, looking deeply into the interplay between these technologies, it becomes clear that the foundations of quantum computing host a myriad of possibilities for the next generation of artificial intelligence.
AI: Machine Learning and Deep Learning
The realm of Artificial Intelligence is vast, yet at its core lies two pivotal components: Machine Learning and Deep Learning. Understanding these concepts is not just an academic exercise; it’s essential for appreciating how they synergize with quantum computing to reshape the tech landscape.
Machine Learning, in a nutshell, enables machines to learn from data. It allows algorithms to identify patterns and make decisions without human intervention. This capability drives countless applications, from recommendation systems on streaming platforms to fraud detection in banking.
Deep Learning, on the other hand, is a subset of Machine Learning that utilizes neural networks with many layers. This intricate architecture mimics human brain functions in a rudimentary way, making it particularly powerful for tasks like image and speech recognition. As you can imagine, the advancement of these technologies holds significant implications, especially when paired with the computational prowess that quantum computers can offer.
Understanding the specifics of these technologies is crucial. Here’s how they operate:
The Basics of Machine Learning
Machine Learning revolves around algorithms capable of learning from data patterns. Imagine you have a dataset containing various features - say, age, income, and purchasing habits of consumers. A machine learning model can analyze this data to predict future buying behavior based on historical information.
There are primarily three types of learning approaches:
- Supervised Learning: In this method, models learn from labeled data. For example, predicting house prices from past sales data involves knowing the actual prices as part of the training phase.
- Unsupervised Learning: Here, the model works with unlabeled data and tries to identify inherent patterns. Clustering similar customers based on shopping behavior can be done without prior knowledge of their labels.
- Reinforcement Learning: This approach involves agents that learn through trial and error, receiving rewards based on their actions. Think of it like training a dog - it learns to perform tricks when given treats.
Each of these methods contributes uniquely to AI’s capabilities, enabling machines to be more predictive and proactive.
Understanding Deep Learning
Deep Learning takes Machine Learning a step further by employing neural networks. These networks consist of numerous layers, each processing data at different levels of abstraction. For those familiar with the concept of hierarchical organization, it’s similar to breaking down complex concepts into simpler parts.
Consider the example of a facial recognition system. The first layer of a deep learning model might identify simple features like edges and shapes. Subsequent layers get progressively complex, recognizing patterns like eyes, noses, and eventually whole faces.
Key Advantages of Deep Learning:
- Feature Extraction: Unlike traditional methods that require manual feature selection, deep learning automates this process, leading to higher accuracy and efficiency.
- Large Data Handling: With vast amounts of data becoming more common, deep learning models excel at finding insights without the need for extensive data preprocessing.
- Continuous Learning: Similar to human learning, deep learning models can be fine-tuned with new data, thereby improving their efficacy over time.
In summary, the interplay between Machine Learning and Deep Learning is fundamental to the capabilities of AI. As quantum computing continues to evolve, the potential for these two areas to evolve alongside is unparalleled. They not only promise to make data-driven decision-making faster and more accurate but also transform industries as we know them.
"The future of AI doesn’t just lie in making intelligent decisions but in optimizing those processes at an unprecedented speed and accuracy."
In the subsequent sections, we will explore how quantum computing can amplify these technologies, paving the way for innovations that are still beyond our horizon.
Quantum Computing's Impact on AI
The crossroads between quantum computing and artificial intelligence offers a glimpse into a future where computational capabilities are dramatically enhanced. Traditional computing approaches have served us well, but the complexities involved in processing vast amounts of data and executing complex algorithms demand a new perspective. Enter quantum computing, which promises to revolutionize these challenges, thereby sparking conversations about its potential impact on AI technologies.
The unique attributes of quantum computing—such as superposition and entanglement—allow for the execution of tasks at unprecedented speeds and efficiencies. These capabilities have major implications for deep learning and other forms of AI, where high computing power is a must. Furthermore, as AI continues to evolve, its dependence on data increases exponentially. Quantum computing can provide the necessary boost to deal with this intensifying demand, paving the way for more sophisticated and intelligent applications in various sectors.
Enhancing Computational Power
Computational power is the proverbial 'bread and butter' for any machine learning model. The efficiency with which algorithms process data directly impacts the accuracy and reliability of AI outcomes. Quantum computers stand apart from classical machines due to their ability to perform many calculations simultaneously. This is akin to a maestro conducting an orchestra of operations, making the entire process harmonious rather than sequential.
- High-Performance Computing: Quantum computers can tackle complex tasks at remarkable speeds. Algorithms that could take weeks on classical computers might be executed in mere minutes.
- Solving NP-Hard Problems: Problems classified as NP-hard, which are notoriously difficult for classical computers, can be approached with new strategies using quantum algorithms, such as using quantum annealing.
By harnessing quantum abilities, we can imagine AI systems that learn faster, adapt better, and perform more efficiently in real time. This enhancement of computational prowess marks a significant shift in how we understand AI's capabilities.
Accelerating Data Processing and Analysis
Data is often called the "new oil," and its management is crucial for AI performance. Quantum computing offers fresh solutions for processing and analyzing data with speed that exceeds classical paradigms greatly. Just picture a highway: quantum computing allows data streams to flow freely without the roadblocks often faced in traditional systems.
A few ways quantum computing revolutionizes data management include:
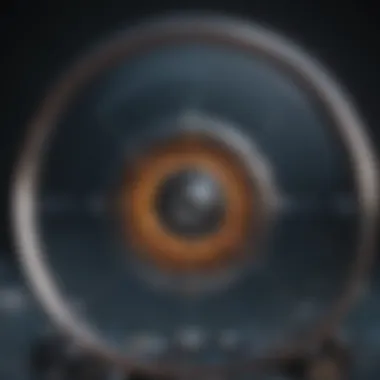
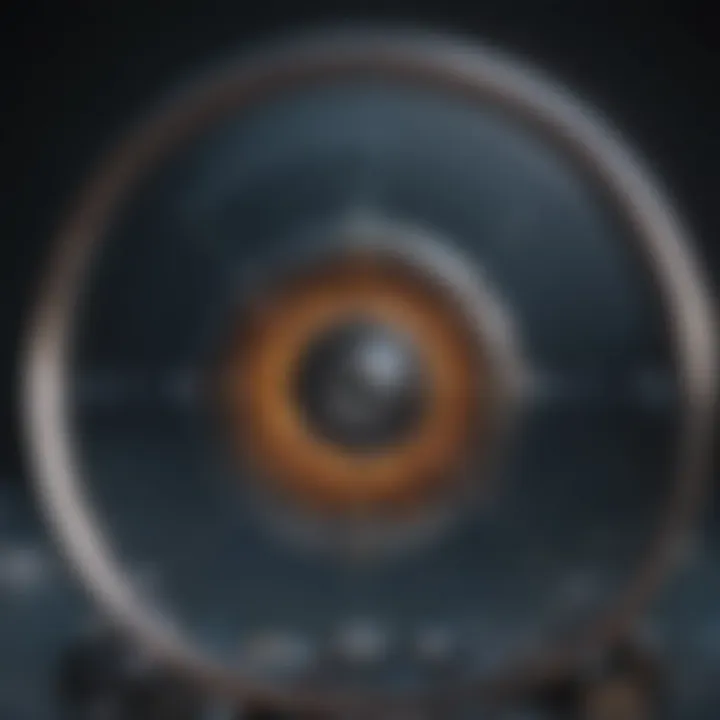
- Parallel Processing: Quantum algorithms leverage the principles of superposition, allowing them to tackle multiple pieces of data simultaneously. This capability magnifies the speed of data processing.
- Increased Pattern Recognition: Machine learning's efficiency is heavily reliant on its ability to recognize patterns. Quantum computing enhances this ability through complex statistical analyses that traditional systems struggle to perform effectively.
The combination of these features not only streamlines the processing workflow but can also turn insights into actionable information at a staggering pace. Potential applications that showcase this acceleration range from financial modeling to complex simulations in biology and chemistry, where AI is becoming indispensable.
"The fusion of quantum computing and AI holds the potential to rewrite the rules of what's possible in data processing and machine learning, blending speed with depth of analysis like never before."
In summary, the impact of quantum computing on AI is set to reshape the landscape of technology, offering paths previously unfathomable with traditional computing methods. As we navigate this exciting frontier, it's worth observing how these developments unfold and influence both existing and future AI applications.
Current Research Developments
The crossroads of quantum computing and artificial intelligence is buzzing with exciting research activities. This intersection holds promise not only for the refinement of AI but also for solving complex problems that are intractable for classical computers. It’s truly a game of chess where the pieces are constantly shifting, and understanding these movements is critical for anyone involved in the evolving tech landscape.
Quantum Machine Learning Models
At the heart of this research are quantum machine learning models. These unique models employ quantum bits or qubits, enhancing the capabilities of traditional algorithms. Here’s why this is significant:
- Speed and Efficiency: Qubits can exist in multiple states simultaneously, which allows for processing vast datasets much faster than classical bits.
- Improved Pattern Recognition: The quantum superposition principle enables the models to recognize patterns in data that would take classical systems considerably longer, if it's feasible at all.
For instance, companies like Google are exploring how quantum models can be applied to optimize not just algorithms, but also neural networks. Early findings illustrate the potential for quantum-enhanced AI in areas such as facial recognition and natural language processing, where speed and accuracy matter.
"Quantum machine learning offers new capabilities that could redefine what we expect from AI."
Hybrid Quantum-Classical Approaches
Given the uncertainties surrounding fully operational quantum systems, researchers are also turning toward hybrid quantum-classical approaches. In this setup, the strengths of both classical and quantum computing are harnessed, leveraging an existing infrastructure while paving the way for quantum advancements.
- Easier Transition: By integrating classical computing resources, organizations can gradually adapt to new quantum technologies, allowing for a smoother integration process.
- focused Problem Solving: Complex tasks can be divided, with straightforward computations handled classically, while quantum systems target the more complex portions.
For example, in pharmaceuticals, the drug discovery process can be accelerated using quantum simulations for understanding molecular interactions, while traditional machine learning processes can categorize existing data efficiently. This mixed-method approach seems promising in addressing computational challenges in various fields.
In sum, current research developments in quantum machine learning and hybrid approaches are driving a revolution in AI, showing how closely intertwined these fields are as they evolve together. Emphasizing collaboration and innovation, it's clear that the future holds a spectrum of possibilities that may very well redefine technology as we know it.
Real-World Applications
The synergy between quantum computing and artificial intelligence is not just a theoretical marvel; it is paving new avenues across various industries. Real-world applications serve as a testament to this interplay, illustrating how these advanced technologies are not merely futuristic concepts but active elements reshaping tangible sectors. The integration of quantum computing into AI phenomena helps tackle complex challenges that were previously insurmountable, and understanding its impact is crucial for students, researchers, and professionals alike.
Healthcare Innovations
In healthcare, the convergence of quantum computing and AI opens a Pandora’s box of possibilities. These technologies provide unprecedented capabilities in areas such as drug discovery, personalized medicine, and patient data management. For instance, machine learning algorithms, powered by quantum processors, can analyze molecular interactions on a scale that classical computers struggle to match. This can speed up the drug development process, allowing companies to pinpoint viable candidates much faster.
- Drug Discovery: Quantum AI can analyze vast databases of molecular structures to identify patterns and predict interactions, which is a game-changer in developing new therapies.
- Genomic Medicine: Quantum algorithms can crunch genetic data, enabling the customization of treatments based on an individual’s unique genetic makeup, enhancing the effectiveness of therapies.
Moreover, the ability to process large datasets with unparalleled speed aligns perfectly with AI's predictive capabilities, ultimately leading to better healthcare outcomes.
Finance and Optimization
The financial sector is another area where the marriage of quantum computing and AI is making waves. Financial institutions are exploring how quantum algorithms can enhance trading strategies, risk assessment, and fraud detection. By processing complex models rapidly, quantum AI enables real-time analysis of market trends, which classical systems find cumbersome.
- Algorithmic Trading: Quantum computing allows for the development of sophisticated trading algorithms that can adapt to real-time market changes, improving return on investment.
- Risk Mitigation: Quantum-enhanced AI models can simulate numerous scenarios, providing financial analysts with enhanced tools for understanding risk in volatile markets.
The optimization of investment portfolios and predicting market movements with razor-sharp accuracy represents not just an advantage but a necessity in today's fast-paced economy.
Logistics and Supply Chain Management
In the realm of logistics and supply chain management, the application of quantum computing combined with AI takes efficiency to the next level. With the growing complexity of global trade, optimizing supply chains has become a critical challenge.
- Route Optimization: Quantum algorithms can identify the most efficient routes for delivery, reducing costs and improving delivery times. This ability to analyze multiple variables simultaneously can significantly enhance the logistics planning process.
- Inventory Management: AI models, enhanced by quantum computing, are capable of predicting demands with greater accuracy, leading to optimal inventory levels and minimizing waste.
Companies that utilize these innovations are gaining a competitive edge, streamlining operations, and significantly reducing operational costs.
"The intersection of quantum computing and AI is not just a merging of technologies; it is the dawn of a new era where possibilities become realities across industries."
In sum, the real-world applications of quantum computing and AI highlight their potential to solve complex problems, drive industry growth, and improve overall efficiency. Whether in healthcare, finance, or logistics, the fusion of these technologies is resonating through various sectors, translating theory into practical solutions. Understanding these applications lays the groundwork for future innovations and integrations that can continue to shape our reality.
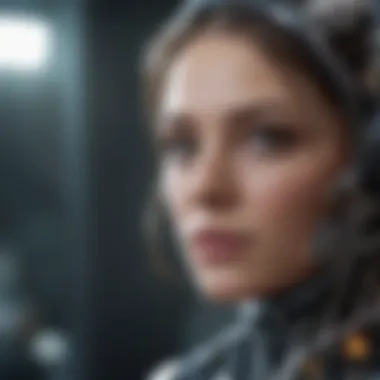
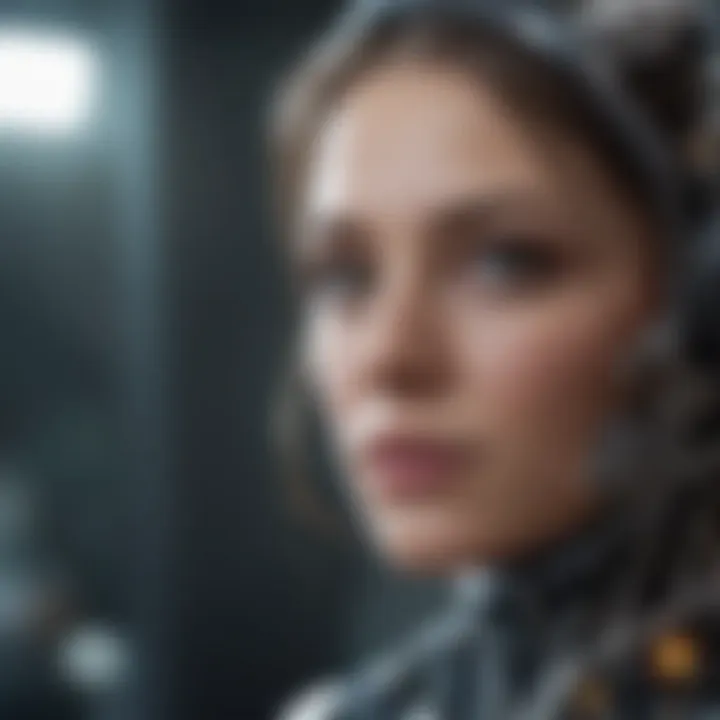
Challenges of Integration
Integrating quantum computing and artificial intelligence presents a host of challenges that require careful consideration. As both fields advance, the synergy between them promises to transformative AI technologies. Nonetheless, this interlacing of AI with quantum computing isn’t without hurdles that must be addressed to fully exploit their capabilities.
Technical Limitations of Quantum Systems
The significant technical limitations inherent in quantum systems pose a real barrier to effective integration. For starters, quantum computers are still in their developmental phase and face obstacles like error rates that can impede reliability.
Here are key highlights:
- Error Correction: Quantum bits, or qubits, can easily lose their quantum state due to environmental interference, introducing a need for complex error-correcting mechanisms.
- Limited Qubit Count: The number of qubits available in existing quantum computers often restricts the computational power needed for advanced AI tasks.
- Decoherence: Qubits can lose their coherence very quickly, which is a crucial condition for them to function correctly. The swift decoherence times limit how long qubits can perform computations before needing to be reset.
The combination of these limitations presents a formidable challenge. Researchers must innovate robust error-correction methods and increase coherence times to make quantum systems viable for AI. As of now, the potential that quantum computing could have on AI remains clouded under these technical limitations.
Scalability and Practicality Issues
Scalability is another pressing concern when looking at the practical applications of quantum-enhanced AI. Current quantum technologies, such as those the IBM Quantum Experience and Google Sycamore platforms showcase, remain highly specialized and are primarily based in research environments. This means that they’re not yet ready for widespread implementation in business or consumer applications.
Key points about scalability include:
- Infrastructure Investment: Adopting quantum solutions demands significant changes in the existing computing frameworks of businesses and requires hefty investments in infrastructure. Many companies may find it hard to justify this cost without clear short-term returns.
- Skill Gap: There exists a notable skill gap in the workforce where quantum computing knowledge is limited. This presents a barrier to organizations seeking to integrate quantum systems into existing workflows.
- Interoperability: Creating systems that can bridge classical and quantum processing is crucial. Practical solutions need to ensure that both systems can work together seamlessly, bringing forth a more accessible architecture for AI applications.
The practicality of operating at the intersection of quantum computing and AI is thus tethered to the resolve of the tech community. The ongoing research and collaboration among quantum physicists, AI experts, and computer scientists need to focus on finding innovative solutions to these scalability issues for true synergy to take shape.
The integration of quantum computing with AI stands to redefine technological advancements, but only if we can navigate the myriad challenges that lie in our path.
Future Direction and Implications
The fusion of quantum computing and artificial intelligence is not just a buzzword; it’s a pivotal point in technology that commands serious attention. As the world gravitates towards reliance on AI for various applications, the potential to enhance these systems using quantum principles transforms our outlook on computing entirely. This section will delve into the significant advantages, theoretical underpinnings, and forward-thinking concepts that can redefine the trajectory of AI.
Theoretical Advances in Quantum Algorithms
Emerging from the realms of physics and computer science, quantum algorithms offer a novel approach to data processing and problem-solving. They harness the uncanny properties of quantum bits, allowing computations to unfold in ways classical systems simply cannot fathom. One of the most promising advances is the development of quantum algorithms that can potentially outperform classical counterparts in machine learning contexts.
For instance, let’s consider quantum support vector machines and quantum neural networks. These algorithms leverage quantum superposition and entanglement, allowing them to operate in multidimensional spaces simultaneously. The result? They can sift through large datasets with more efficiency, revealing patterns that might elude traditional methods. Moreover, the ability of quantum algorithms to potentially expedite optimization problems presents a grand opportunity across numerous sectors.
"Quantum algorithms are like a hidden treasure, awaiting to revolutionize how we approach complex computational tasks."
Investing in these theoretical advancements spurs innovation and draws in researchers eager to explore uncharted territories. Not only do these algorithms promise faster computations, but they also encourage interdisciplinary collaborations, knitting together ideas from physics, computer science, and neuroscience. As we tread deeper into the quantum age, the implications of these theoretical achievements ripple through academia and industry.
Envisioning Quantum-Enhanced AI
When we ponder on the future of AI intertwined with quantum computing, it’s not just about speed; it's fundamentally about redefining intelligence itself. Imagine systems that learn and adapt at unprecedented rates due to enhanced computational power. Quantum-enhanced AI could transform industries from healthcare to finance, underpinning decision-making processes with deeper insights gained from vast datasets.
Take healthcare for instance. AI algorithms could analyze genomic data quicker and with improved accuracy, leading to tailored treatment plans. In finance, quantum-enhanced AI might analyze market trends for optimized trading strategies, potentially leading to fairer markets.
The thought of such advancements brings light to the consideration that while current AI struggles with certain complexities, quantum computing can unfasten these shackles. Quantum-enhanced AI could deliver:
- Significantly reduced time for training machine learning models
- Enhanced problem-solving capabilities in complex optimization scenarios
- Increased accuracy in predictions through more refined data analysis
As these realities inch closer, ethical considerations grow ever more vital. Who is responsible when AI, backed by quantum power, makes mistakes? How do we ensure equitable access to such technologies? The dialogue surrounding these queries will shape the fabric of our technological society.
Finale
The intersection of quantum computing and artificial intelligence is not merely a point of curiosity within the field of technology but rather a crucial chapter that lays the groundwork for the future of computation. As we find ourselves on the brink of what could be termed a technological renaissance, understanding how these two powerful forces intertwine is paramount.
Summary of Key Takeaways
In summary, several key points emerge from our exploration:
- Quantum Computing's Unique Capabilities: The distinct nature of quantum bits, superposition, and entanglement provides computational power far beyond what classical systems can deliver.
- AI Methodologies Benefit from Quantum Advancements: Various machine learning algorithms stand to gain exponentially from the enhanced processing potential offered by quantum computing.
- Real-World Applications Are Flourishing: Industries such as healthcare, finance, and logistics are beginning to embrace these technologies, showcasing their potential in real-world settings.
- Existing Challenges Remain: While the possibilities are tantalizing, a number of obstacles—technical limitations and scalability issues—must be overcome.
- Future Directions Hold Promise: The ongoing research indicates that the combination of AI with quantum computing could lead to breakthroughs that fundamentally alter how decisions are made and data is analyzed.
Final Thoughts on Quantum AI
As we gaze into the horizon of technological advancement, the relationship between quantum computing and AI presents not only challenges but also vast opportunities. It is a dynamic field where constant research and innovation are indispensable. Those looking to delve into these waters should prepare for a landscape that is ever-evolving and full of latent potential.
As we conclude, it’s worth reflecting on the potential ramifications of quantum AI on society. Will it elevate our capabilities to unprecedented heights, or will it bring about new ethical dilemmas that require scrutiny? The road ahead is winding, and as this journey unfolds, staying informed and engaged with the developments of both fields will be crucial for educators, researchers, and professionals alike.
"The greatest challenge of our time lies not in what AI can achieve, but in how we choose to integrate it with revolutionary technologies like quantum computing."
In this era of innovation, it serves us well to remain vigilant, curious, and thoughtful about the possible futures that lie ahead.