Melanie Mitchell's Impact on Artificial Intelligence
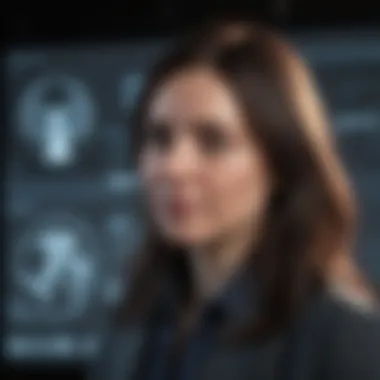
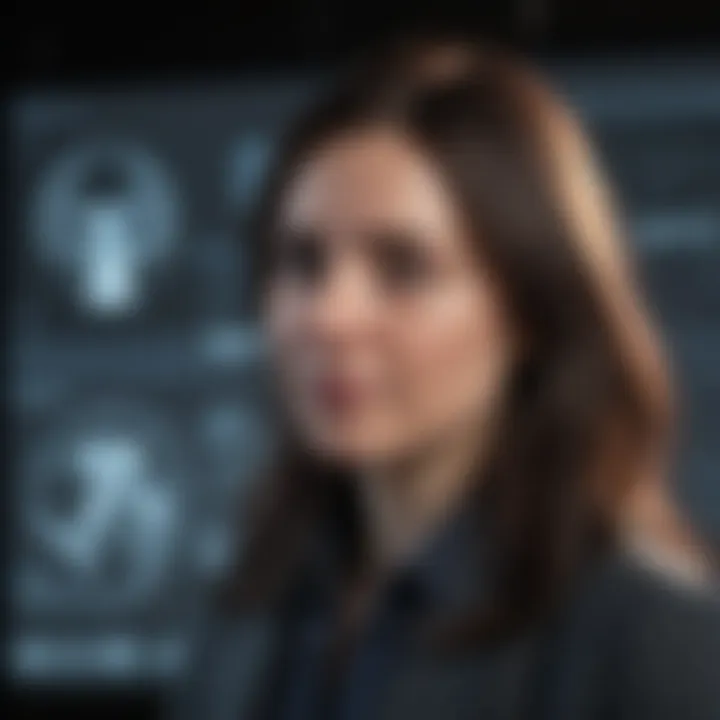
Intro
Melanie Mitchell’s work in artificial intelligence offers a fascinating lens into the evolving landscape of intelligent systems. Her research challenges prevailing assumptions while introducing powerful frameworks that push the boundaries of what we understand about machine learning and intelligence. By delving into her contributions, we can grasp not just the intricacies of her theories like emergent behavior and genetic algorithms, but also how they fit into the larger AI puzzle.
In this exploration, we aim to shine a light on the key facets of Mitchell’s research, shedding light on both its applications and implications. Each segment will tackle relevant issues that arise in the modern study of AI while incorporating Mitchell’s unique perspective, recognized for its clarity and depth. The hope is that learners, educators, and professionals alike will find value in this comprehensive narrative.
Key Research Findings
Overview of Recent Discoveries
Melanie Mitchell has uncovered a wealth of information regarding the foundations and evolution of intelligent systems. Her significant strides in understanding genetic algorithms and their capacity for adaptation and optimization stand at the forefront of her research. For instance, her work raises intriguing questions about how these algorithms can enable machines to evolve solutions over generations, akin to Darwinian evolution.
Moreover, Mitchell’s interest in emergent behaviors highlights how complex systems can produce surprising patterns and capabilities from relatively simple rules. This notion resonates across a wide range of disciplines, including biology, computer science, and even economics.
In a recent study, she examined how simulated agents demonstrated cooperative behaviors despite individual self-interest, illuminating principles fundamental to social and economic systems.
Significance of Findings in the Field
The implications of Mitchell’s discoveries are profound. By exposing limitations in conventional approaches to AI, she invites researchers to reconsider assumptions in the field. For example, she emphasizes that machine learning techniques, while powerful, may not generalize well in novel situations. This realization has far-reaching effects on how AI systems are developed and implemented in practical scenarios.
Moreover, her critique of deep learning presents a call for more interpretable and robust AI systems. It encourages a shift toward understanding not just how to build smarter machines, but also how to ensure their decisions are explainable and consistent with human values.
"The best way to predict the future is to create it."
—Peter Drucker
This quote perfectly encapsulates the vision that Mitchell promotes through her work. Rather than falling into the trap of treating AI as an ultimate black box, she urges the community to engage in a thought process that brings clarity to automated decision-making and evolves AI into a tool for human benefit.
Breakdown of Complex Concepts
Simplification of Advanced Theories
Mitchell’s approach makes complex ideas accessible. She navigates through theories and concepts that tend to bog down both newcomers and seasoned experts. The key is her ability to distill convoluted academic jargon into straightforward explanations that can resonate with a broader audience. For instance, her elucidation on genetic algorithms explains how these systems can organically evolve solutions similar to natural selection, thus making it easier for students and non-specialists to grasp.
Visual Aids and Infographics
Accompanying her written work, Mitchell often employs visual aids that enhance understanding. Diagrams illustrating the phases of genetic algorithms help demystify the process of selection, crossover, and mutation. Likewise, her graphical representations of emergent behaviors in simulated agents allow observers to visualize interactions, making the underlying math feel less intimidating. Utilizing such tools fosters a deeper appreciation of AI among varied audiences, positioning her work as a crucial resource in teaching complex AI concepts.
In summary, Melanie Mitchell’s contributions to artificial intelligence are not just about her findings, but about reshaping how we interpret and interact with intelligent systems. This balance of depth and clarity makes her research indispensable to both enthusiasts and professionals looking to navigate the complex realm of AI.
Prelude to Melanie Mitchell
Melanie Mitchell represents an enlightening force in the realm of artificial intelligence. Her work is not only significant due to her groundbreaking research but also because she challenges the conventional understandings of intelligence itself. As the discipline of AI grapples with its potential—and its limitations—Mitchell’s insights offer a fresh perspective, one that emphasizes complexity and emergent behavior in intelligent systems.
This section aims to lay a firm foundation, shedding light on who Melanie Mitchell is and why her contributions are crucial to AI research. Readers will discover her academic roots, her pivotal career moments, and how these experiences shaped her views on the intricacies of artificial intelligence. By understanding her background and milestones, one gains a deeper appreciation for the implications of her research within the broader context of AI development.
Background and Education
Melanie Mitchell’s journey began in the academic laboratories, where her curiosity and intellect flourished. She earned her bachelor's degree in Mathematics from the University of California, Berkeley, followed by her master’s degree in Computer Science from the same institution. What shapes a thinker is often their foundational knowledge, and Mitchell's immersion in these fields laid a strong bedrock for her future theories.
Later, she continued her academic pursuits at the University of Michigan, obtaining a PhD in Computer Science. Her doctoral dissertation focused on genetic algorithms, a theme that would echo throughout her career. This academic background provided not just technical expertise but also critical insights into problem-solving approaches that are unique to complex systems.
The breadth and depth of her education illustrate the importance of interdisciplinary knowledge in AI—a theme she often emphasizes in her discussions.
Career Milestones
Mitchell's career is a tapestry woven with notable achievements that speak volumes about her impact on the field. One of her significant milestones was her role at the Santa Fe Institute, where she pondered over the intersections of genetics and computation. It's here, amidst a think-tank of brilliant minds, that she honed her views on complex adaptive systems.
Another highlight was her tenure at Portland State University, where she not only conducted research but also shaped the next generation of computer scientists through her teaching.
Throughout the years, she has authored several influential publications, including her acclaimed book, Complexity: A Guided Tour, which delves into the principles driving complex systems from an interdisciplinary lens. Her works regularly focus on the limitations of traditional AI paradigms and advocate for a more nuanced understanding of intelligence through the lens of complexity science.
These milestones paint a portrait of a researcher who consistently pushes boundaries, prompting both colleagues and students to re-evaluate their assumptions about machine learning and intelligence.
“To understand intelligence, we must first understand complexity,” Melanie Mitchell often states, capturing the essence of her work and its broader implications.”
Foundations of Artificial Intelligence
Foundational concepts in artificial intelligence (AI) are critical for understanding the intricate landscape that Melanie Mitchell navigates. These basics are not just theoretical musings but the very tools that drive innovation within the field. They shape the aspirations of what AI can ultimately achieve. Understanding these elements helps demystify the perception of AI, making the subject accessible to a broader audience.
This article offers a lens through which we can appreciate and dissect the fundamental building blocks that underpin AI. Concepts like machine learning, algorithms, and system complexities are essential for both enthusiasts and industry veterans. Through this exploration, readers will see how Mitchell’s work aligns with and challenges these foundational principles.
Defining Artificial Intelligence
Artificial intelligence is often described as the capability of a machine to imitate intelligent human behavior. However, this simplistic view can obscure the multifaceted nature of the field. Essentially, AI encompasses systems designed to perform tasks that, in the past, required human intervention. These tasks might include recognizing speech, making decisions, or identifying images.
The diversity in definitions shines a light on the varying expectations people hold about AI. Some see it as mere automation, while others view it as a pathway toward enriching human capabilities. The realization that AI is a spectrum—with some systems exhibiting narrow intelligence and others aiming for general intelligence—brings clarity to discussions about its impact.
"AI is not just a set of tools; it’s a new paradigm that challenges our traditional understandings of intelligence itself."
Key Historical Developments
The evolution of artificial intelligence can be traced through a series of pivotal moments that laid the groundwork for modern advancements. It all began in the mid-20th century with the concept of machine learning. Early systems were rule-based, relying on predefined logic to operate. This approach was revolutionary yet limited, stunting advancements until new paradigms emerged.
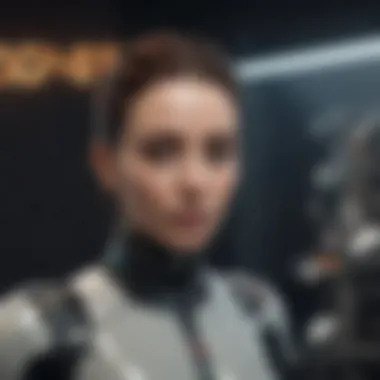
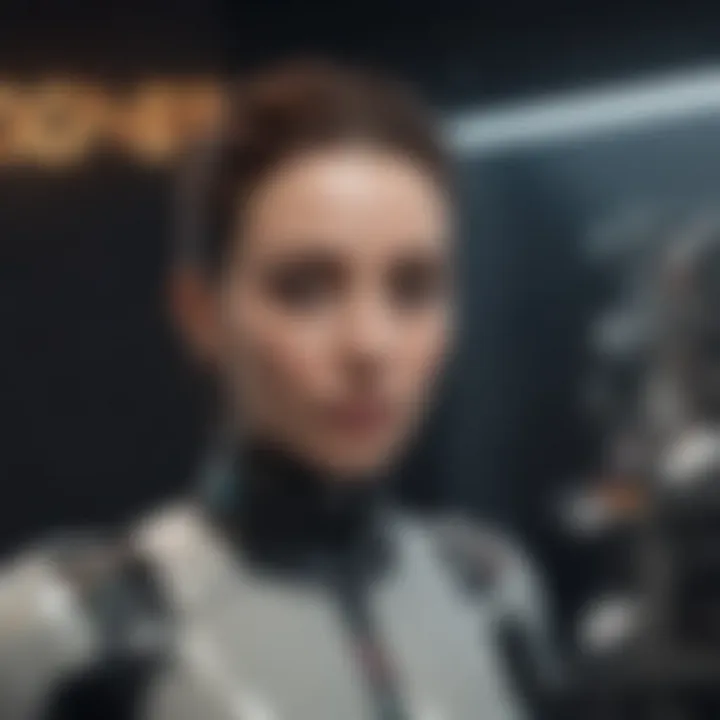
In the 1950s, key figures like John McCarthy and Alan Turing began to shape the conversation around AI. Turing's seminal paper on computable numbers introduced the idea of machines that could learn from data, a radical shift from previous thinking. Later developments in the 1980s brought forth neural networks, echoing the complexity of human brains. These networks opened doors to deep learning, allowing machines to tackle tasks that seemed insurmountable just a decade earlier.
While historical milestones serve as markers of progress, they also represent ongoing discussions in the field. Understanding where AI has come from helps in clarifying its potential future trajectories. Notably, Melanie Mitchell’s contributions have consistently reflected on these historical perspectives, using them to inform her research on complexity and emergent behavior in AI.
In summary, the foundations of artificial intelligence not only frame current debates but also guide future inquiries. With Melanie Mitchell's insights, we gain a clearer vision of the maze that AI represents, highlighting the delicate balance between developing technological prowess and addressing ethical considerations inherent in its implementation.
Mitchell's Perspective on AI
Melanie Mitchell offers a distinct viewpoint on artificial intelligence, emphasizing its essence as a study of complexity. This perspective is pivotal as it challenges conventional narratives about AI, pushing readers to appreciate the intricate systems that underlie intelligent behavior. By viewing AI through the lens of complexity science, Mitchell encourages an understanding that transcends mere programming or data processing.
Her ideas invite us to consider the implications of living systems that exhibit intelligence not just through simple algorithms but through a symphony of interactions, adaptations, and emergent patterns. This approach fosters a more nuanced dialogue regarding the future of AI technologies and the ethical dimensions they imply.
AI as a Science of Complexity
Melanie Mitchell argues that artificial intelligence is not merely a collection of advanced computational techniques, but rather a branch of complexity science. This stance highlights the interconnectivity, adaptability, and recursive behaviors intrinsic to intelligent systems. Complex systems, by nature, behave in ways that can often be unpredictable. For instance, consider an ant colony's ability to navigate complex environments in search of food. Each ant follows simple rules, yet the colony collectively engages in sophisticated problem-solving without centralized control.
In computational contexts, this perspective allows for a deeper exploration into how agents learn and adapt based on their environments. Mitchell’s work emphasizes that,
"Intelligence arises not only from algorithms but also from the complex interactions within an ecosystem of agents."
This view challenges researchers to rethink AI's foundational theories. As AI systems become more prevalent in areas such as healthcare, finance, and ecological modeling, recognizing the role of complexity in AI can lead to more robust implementations. It begs the question—how can we design systems that fully leverage these principles of complexity?
Emergent Behavior in Intelligent Systems
Emergent behavior refers to phenomena where larger entities display complexities that arise from the simple interactions of smaller parts. Mitchell’s insights shed light on how emergent behavior can inform AI research. Take, for example, traffic flow in a city: individual cars follow basic rules (stop, yield, turn), but the resulting patterns can lead to congestion or smooth traffic. This unexpected behavior isn’t inherently programmed—it arises from the system’s overall dynamics.
Mitchell contends that understanding emergent behavior is crucial for developing AI systems that mimic intelligent functions. By harnessing principles from natural systems, AI researchers can create algorithms that demonstrate flexibility and adaptability. Examples might include:
- Self-organizing systems in smart grids that adjust to real-time energy demands.
- Swarm robotics, where drones collaborate to achieve collective goals without a central command.
Highlights of Mitchell's research suggest that emergent behavior isn't just a curiosity—it has inherent applications in crafting smarter, more efficient AI solutions. As we unravel the complexities of intelligence, we are better poised to integrate these insights into practical applications. The future of AI, seen through this lens of emergence, has the potential to reshape our understanding and application of technology, minimizing inefficiencies and fostering innovative responses to complex problems.
Research Contributions
Understanding the research contributions made by Melanie Mitchell is vital for grasping the evolution and potential of artificial intelligence. Her work, particularly in genetic algorithms, has fundamentally shifted how researchers and practitioners approach problem-solving within complex systems. With an emphasis on the interplay between natural phenomena and artificial models, Mitchell has illuminated pathways that were previously obscured, providing both theoretical and practical insights.
The importance of her contributions lies not only in the depth of her understanding but also in how she effectively communicates these complex ideas. This bridges the gap between academia and the real world. By revisiting age-old problems with a fresh perspective, her research presents valuable lessons that both students and seasoned experts can learn from. Furthermore, understanding her contributions fosters a deeper conversation about the future of AI and its implications for society.
Genetic Algorithms: An Overview
Genetic algorithms (GAs) serve as a cornerstone in Melanie Mitchell's work. Drawing inspiration from the principles of natural selection, these algorithms replicate the process of evolution to tackle optimization problems. Mitchell's research here is not just academic; it’s the embodiment of how computational techniques can mimic biological processes in a meaningful way.
At its core, a genetic algorithm operates by maintaining a population of potential solutions to a given problem, much like a biological population. Over successive generations, these solutions are iteratively refined through selection, crossover, and mutation. This mimics the natural process where the fittest individuals are chosen to reproduce, with the goal of evolving solutions that efficiently address a specific task.
The adaptability of genetic algorithms makes them particularly suited for complex problems where traditional methods might struggle. For instance, in scheduling issues or resource allocation challenges, GAs can navigate vast solution spaces more effectively than deterministic algorithms. Mitchell’s work emphasizes the importance of diversity in the population, which she argues is crucial for preventing premature convergence on suboptimal solutions.
Applications of Genetic Algorithms
Where genetic algorithms shine is in their broad range of applications across different fields. Mitchell’s exploration of these applications not only showcases the versatility of her research but also underscores the practical impact of her findings. Here are some notable applications:
- Engineering Design: GAs are utilized in optimizing structures and systems, allowing engineers to experiment with various configurations to arrive at the most efficient design without excessive manual computation.
- Finance: In algorithmic trading, genetic algorithms help organizations optimize trading strategies by evolving rules based on historical data patterns, thus adapting to ever-changing markets.
- Bioinformatics: Genetic algorithms are instrumental in gene selection and sequence alignment, enabling researchers to manage and analyze vast datasets efficiently.
- Game Development: Developers use GAs to create adaptive AI that can learn from player behaviors, enhancing the gaming experience and introducing more complex opponent behaviors.
The adaptability of genetic algorithms allows them to solve problems across various domains, demonstrating the breadth of their impact.
Through her ongoing research and teachings, Melanie Mitchell continues to inspire a new generation of scientists and engineers to explore these dynamic methods. Her contributions significantly enrich the discourse on artificial intelligence, providing a laneway for future innovations.
Machine Learning Insights
Machine learning sits at the heart of artificial intelligence, playing a crucial role in driving innovation and applications across various domains. It's like the engine of a train; without it, the journey towards advanced AI solutions would stall. This section unpacks Melanie Mitchell's observations and critiques surrounding machine learning, emphasizing the nuances often overlooked in the discourse.
The Limitations of Current Systems
Despite the advances in machine learning, Mitchell argues that contemporary systems face serious limitations. One major issue is that these systems can process massive amounts of data but struggle with context and understanding. They can spit out predictions faster than a parrot can mimic human speech, yet that doesn’t mean they truly “understand” the information they handle.
"The ultimate goal of AI is understanding the world in a nuanced manner, not just pattern recognition." - Melanie Mitchell
Here are several core limitations:
- Data Dependency: Current machine learning models require extensive labeled data to produce meaningful outcomes. However, acquiring such datasets can be time-consuming and costly.
- Lack of Explainability: Many models function as black boxes, making it extremely difficult to trace how decisions are made. Understanding why a model made a particular choice can be as opaque as reading hieroglyphics.
- Static Learning: Models can become outdated swiftly. Once trained, if they’re not constantly updated with fresh data, their performance tends to dwindle.
These shortcomings highlight significant areas for growth and improvement in the field. Recognizing these limitations allows researchers to target specific issues, paving the way for future advancements.
Overfitting and Generalization
Overfitting is a complex but essential concept that Mitchell addresses with a keen insight. When a model is too finely tuned to the training data, it performs beautifully on this data but flops like a failed magician on unseen information. Essentially, it loses its ability to generalize, which is the whole point of machine learning in the first place.
Generalization is the term used to describe a model’s capacity to apply learned patterns to new, unseen data. Poor generalization reveals a fundamental flaw—a model may seem promising during tests but face an imminent failure when put in real-world scenarios.
A few points regarding overfitting include:
- Trade-off Dilemma: Striking the right balance between underfitting (too generalized) and overfitting (too specific) is a constant challenge, akin to walking a tightrope.
- Complexity of Models: More complex models often lead to overfitting. Simpler is sometimes better; a model that is easier to interpret is frequently more durable in practice.
- Regularization Techniques: Implementing methods to prevent overfitting is a common practice. Techniques such as dropout or early stopping help models remain flexible and responsive.
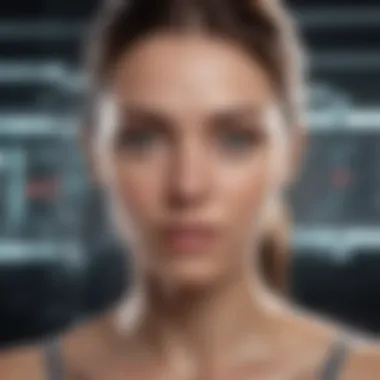
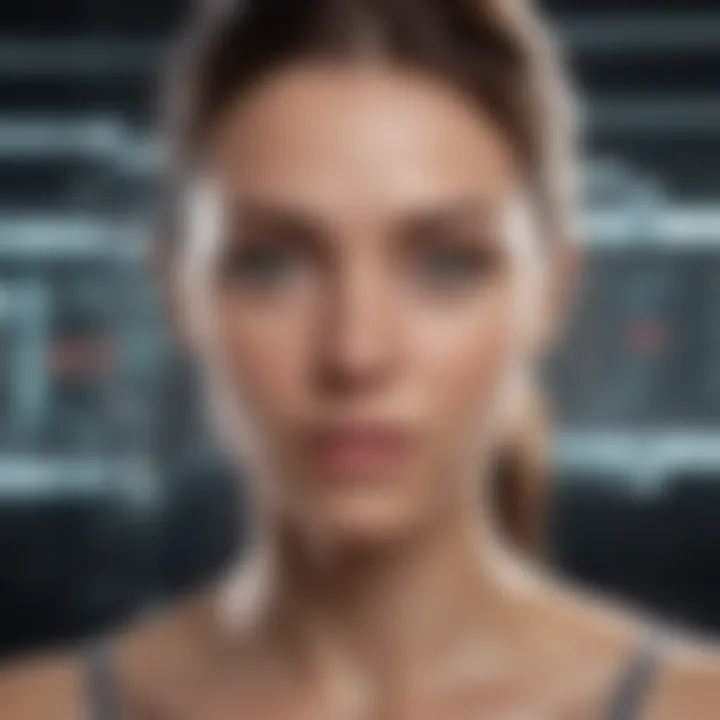
The Role of Abstraction in AI
Abstraction plays a central role in the development of artificial intelligence. It allows for simplification of complex systems, making it easier for researchers and practitioners to understand, design, and implement intelligent behaviors in machines. By stripping away unnecessary details, abstraction helps to focus on the key concepts and functionalities that drive AI performance. This is especially significant when dealing with intricate systems like neural networks or genetic algorithms that operate on vast datasets and multiple variables.
Understanding Abstraction in Complex Systems
When discussing abstraction in complex systems, it’s important to comprehend what it entails. Essentially, abstraction involves distilling complexity into manageable layers. For instance, in the realm of AI, instead of diving into the minutiae of a neuron’s behavior in a neural network, one might look at the network's overall output and how it generalizes from input data without getting bogged down in every individual component.
- Benefits of Abstraction:
- Enhanced Focus: Researchers can zoom in on critical interactions without distraction from lesser details.
- Interdisciplinary Collaboration: Different fields—like computer science, cognitive science, and linguistics—can communicate more effectively by relying on shared conceptual frameworks.
- Improved Efficiency: Algorithms can be designed with fewer computational resources, making AI applications more feasible in real-world scenarios.
Abstraction can also be represented through different models. For example, a 2D representation of a 3D object simplifies the understanding and interaction with the object at hand, illustrating how abstraction can reveal patterns without overwhelming complexity. This insight is essential as it reveals the underlying mechanisms that propel complex systems.
Mitchell's Views on Abstraction
Melanie Mitchell has articulated her perspectives on the importance of abstraction in AI through her research. She argues that abstraction is not merely a tool but a fundamental aspect of understanding intelligence itself. In her work, the idea of abstracting away irrelevant details is not just a matter of convenience; it’s a necessary step in emulating human cognitive processes.
Mitchell emphasizes that the use of abstraction can facilitate more effective problem-solving strategies in AI. She views it as a way to bridge the gap between raw data processing and intelligent decision-making. Mitchell has pointed out that
"the intricate dance of abstraction enables us to perceive the forest for the trees, capturing the essential essence of intelligent behavior."
Her insights lead to a broader understanding of how machines might think, not merely as a replication of human thought processes, but as a novel mechanism that operates differently yet effectively within a structured abstract framework.
By integrating abstraction into AI research, Melanie Mitchell pushes the boundaries of what is perceived as intelligence, encouraging future researchers to explore abstract representations in ways that go beyond surface-level understanding.
Theoretical Implications
The realm of artificial intelligence is intimately tied to theoretical frameworks that shape how researchers and practitioners approach problems related to intelligent systems. Melanie Mitchell’s work not only advances our understanding of AI but also raises important theoretical questions about the nature of intelligence and complexity.
At its core, the theoretical implications of Mitchell's research suggest that AI is much more than mere automation of tasks. It touches on philosophical and cognitive considerations, examining how systems can exhibit behaviors that resemble human intelligence in unpredictable ways.
Complex Systems Theory
Complex systems theory posits that systems made of many interconnected parts can exhibit behaviors that aren’t predictable solely from the properties of the individual parts. This idea aligns closely with Mitchell’s perspective on artificial intelligence. By viewing AI systems through the lens of complexity, we can start to appreciate the emergent behaviors that arise when myriad elements interact in intricate ways.
Consider biological ecosystems, where the behavior of an entire system cannot be fully understood by examining only its components, such as animals or plants. Similarly, Mitchell’s exploration of genetic algorithms and neural networks emphasizes the importance of holistic analysis in AI research. This approach highlights several advantages:
- Interconnectivity: Understanding how parts interact can lead to better system designs.
- Robustness: Systems designed with complexity in mind tend to be more resilient to failures.
- Adaptability: These systems can evolve and learn from their environments, much as organisms do in nature.
This perspective fosters a deeper comprehension of how artificial systems operate, encouraging the development of algorithms that embrace complexity rather than shy away from it.
The Future of AI Research
Looking ahead, the intersection of complex systems theory and artificial intelligence presents promising pathways for future research. As technological advancements unfold, Mitchell's insights remind us that understanding AI requires more than just improved computational power—it necessitates profound theoretical investigations into how intelligence emerges from chaos.
With a focal point on interdisciplinary research, the future could unveil interdisciplinary collaborations that marry insights from fields like biology, physics, and sociology with AI research. Some anticipated trends in this direction include:
- Bio-inspired Algorithms: Drawing from processes in nature could radically enhance the efficiency and effectiveness of AI systems.
- Ethics in Complexity: As AI systems become more complex, ethical considerations will respond to emerging responsibilities of these systems.
- Adaptive Learning Systems: Future AI may leverage real-time data to adapt and evolve consistently, mirroring nature’s own processes.
Overall, Mitchell’s work prompts a rethinking of established paradigms in AI research. By emphasizing the theoretical implications of complexity, we are better equipped to draft a roadmap for the next generation of intelligent systems.
"In the heart of complexity, we find not just challenges but also the keys to future innovations." - Melanie Mitchell
By engaging critically with concepts of emergent behavior and complexity, researchers can forge a path that goes beyond technological optimization, pushing the boundaries of what AI can achieve in society.
Ethical Considerations
The ethical considerations in artificial intelligence are paramount. As technology evolves at lightning speed, the implications of AI on society cannot be overlooked. In this discussion, we will explore the essential aspects of ethics in AI, shedding light on why they are critical in guiding the direction of research and application.
First and foremost, ethical considerations help in addressing substantial moral questions surrounding AI. With systems getting increasingly autonomous, one fears the potential consequences of decisions made by algorithms. Who is accountable when an AI makes a mistake? The answer to such queries shapes policies that govern the deployment of AI technologies. Moreover, these policies ensure that AI benefits the public rather than aggravating disparities or biases that may already exist.
"Ethics is not about knowing what to do, it's about engaging with the implications of what we do."
Benefits of considering ethics in AI include fostering trust among users, promoting transparency in AI systems, and protecting user privacy. By integrating ethical frameworks into their innovations, organizations can mitigate risks linked to biases in data, safeguard personal information, and ultimately, instill confidence in their AI implementations.
Specific Elements to Consider in AI Ethics:
- Fairness: Ensuring that AI systems treat all users equitably without discrimination.
- Transparency: Making the functioning of algorithms understandable to stakeholders.
- Privacy: Protecting users' personal data from misuse or unauthorized access.
- Accountability: Defining responsibility in cases of AI failure or adverse outcomes.
- Sustainability: Assessing the long-term impact of AI on society and the environment.
In summary, understanding ethical considerations in AI is crucial for responsible development and deployment. They not only mitigate risks but also encourage a culture of fairness and accountability that must accompany technological advancement.
Understanding AI Ethics
AI ethics encompasses a broad range of principles and values that guide the development and implementation of artificial intelligence technologies. This domain is often intertwined with broader discussions around human rights, data privacy, and societal impact. Essentially, the goal here is to ensure that AI serves the collective good while minimizing harm.
At the core, ethical AI recognizes the potential for both positive and negative outcomes. Technologies can enhance productivity, foster innovation, and lead to breakthroughs that benefit humanity. However, they can also exacerbate existing inequalities, invade privacy, or make decisions that lack human empathy.
AI ethics usually aligns with principles set forth by various organizations such as the IEEE and the European Commission. These frameworks stress the importance of:
- Human-centric design: Keeping users at the heart of AI development.
- Inclusivity: Engaging diverse stakeholders in AI discussions.
- Bias mitigation: Addressing potential prejudices in data and algorithms.
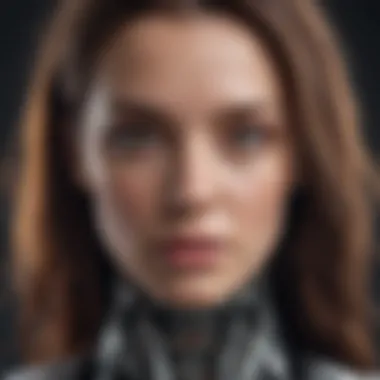
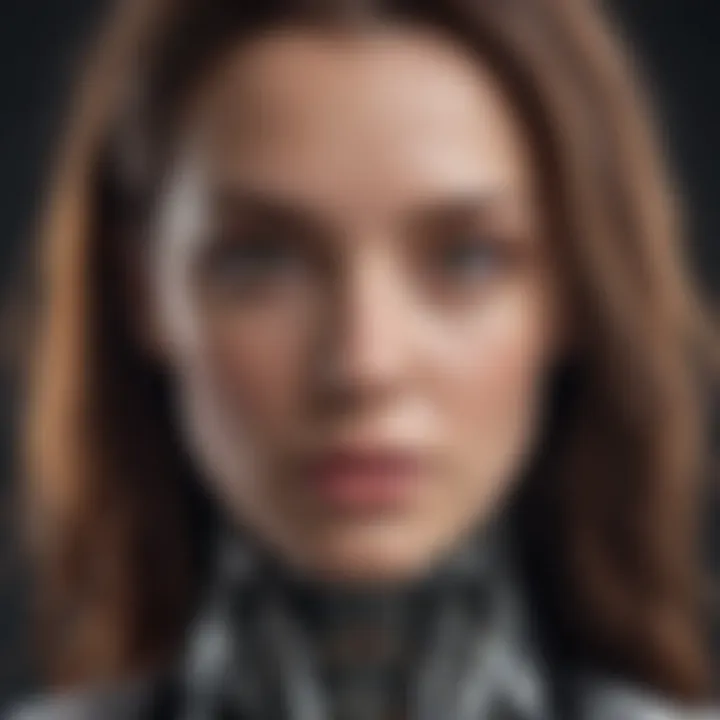
Additionally, fostering interdisciplinary collaborations among technologists, ethicists, and policymakers can lead to well-rounded approaches in shaping the future of AI.
Mitchell's Ethical Stance
Melanie Mitchell has consistently highlighted the essential role of ethics in AI development. Her work does not shy away from the importance of scrutinizing how intelligent systems are developed and the potential consequences they wield. She asserts that while AI can emulate human-like behaviors, it cannot replace the ethical and moral frameworks that guide human choices.
Mitchell often emphasizes the need to expand our understanding of intelligence, not just as a functional attribute but as something deeply intertwined with ethical considerations.
Her perspectives invite a conversation about why we need to think critically about what it means for machines to learn from human data. Are we replicating our biases when we design these systems? Is it ethical to train machines on datasets with historical prejudices?
As research advances, Mitchell advocates for continuous engagement between technologists and ethicists, asserting that ethical deliberations cannot be an afterthought. They need to be integrated throughout the lifecycle of AI development. Her work inspires a more conscious approach to AI, prompting professionals to ask not just if they can do something with AI, but whether they should.
Public Engagement and Education
In today's fast-paced technological landscape, understanding artificial intelligence is becoming as crucial as literacy or numeracy. Melanie Mitchell emphasizes the importance of public engagement in AI, recognizing that a well-informed public can facilitate more responsible advancements in the field. As AI technologies rapidly evolve, fostering a dialogue around their implications is not just beneficial; it’s essential. This ensures that societal values are mirrored in the development and integration of AI systems.
One primary element of public engagement is clear communication. Mitchell advocates for demystifying AI, breaking it down into concepts that even those outside technical fields can grasp. This involves translating complex ideas into relatable terms, thereby encouraging broader participation in discussions about AI governance and ethics.
There are considerable benefits to enhancing public understanding of AI:
- Informed Decision-Making: A populace educated in AI can make better choices regarding policy and technology adoption.
- Ethical Development: When citizens are aware of AI’s potential impacts, they can hold organizations accountable, ensuring ethical considerations aren’t brushed aside in the name of innovation.
- Innovation Feedback: Engaging the public creates avenues for diverse perspectives, which can lead to innovative ideas and solutions that might not emerge in isolated research circles.
Mitchell’s approach suggests that engagement should not merely aim to educate, but to inspire action and curiosity regarding the future of technology.
"The greatest challenge is not the code, but the culture surrounding it."
Communicating AI Concepts
Effective communication of AI concepts goes beyond mere knowledge transfer. It involves artfully weaving narratives around how AI affects daily life and societal structures. Mitchell's work highlights the use of metaphors and analogies as powerful tools in making AI relatable. For instance, comparing AI decision-making processes to human reasoning can create a bridge between expert knowledge and layperson understanding.
Moreover, diverse media formats—such as podcasts, videos, and interactive workshops—can significantly enhance engagement. Using a multi-channel approach allows for reaching wider audiences. Educators and researchers ought to consider their target demographics and tailor communication strategies that resonate with them.
Outreach Programs in AI Education
Outreach programs play a pivotal role in educating the next generation about AI. Programs at various levels, from elementary schools to universities, are vital in weaving AI literacy into the fabric of our education system.
Mitchell has been a staunch advocate for integrating AI education into the curriculum, emphasizing:
- Hands-On Learning: Building simple AI models or participating in robotics competitions can ignite interest in the subject.
- Interdisciplinary Connections: AI is not just about computer science; it intersects with ethics, sociology, and the arts. Outreach programs can showcase these connections, appealing to a broader range of students.
- Collaboration with Community Partners: Partnering with industry professionals can provide students with mentorship opportunities and real-world insights.
Through such initiatives, students not only gain knowledge but also develop critical thinking skills essential for navigating a world increasingly shaped by artificial intelligence. Mitchell’s insights urge us to think beyond technical training and focus on fostering a culture of curiosity and ethical awareness surrounding AI.
Future Directions
As we look at the horizon of artificial intelligence, it becomes crystal clear that the future direction of AI research and development is crucial not just for academics, but for society as a whole. The ideas and contributions of key figures like Melanie Mitchell serve as the bedrock upon which we can build advanced systems that are smarter, more efficient, and ethically responsible. In this section, we will delve into anticipated trends and the initiatives that can guide researchers and practitioners in navigating the complex landscape of artificial intelligence.
Anticipated Trends in AI
The field of AI is constantly evolving, but some trends seem to be gaining traction and could define the next wave of innovations. Here are a few of the expected developments:
- Increased focus on common sense reasoning: As systems become more sophisticated, they need to also understand the subtleties of human life. Common sense reasoning will enable machines to process information in a more human-like way.
- Greater integration of AI with other technologies: This includes the interplay between AI and the Internet of Things, which can create smarter environments that adapt to users' needs in real-time.
- Ethical AI frameworks: As AI systems get embedded deeper into public and private life, there's a noticeable shift toward developing robust ethical frameworks. This could guide how AI is applied in sensitive areas like healthcare and justice.
"The future of AI is not just about creating smarter machines; it's about creating machines that are morally accountable and socially beneficial."
– Melanie Mitchell
Research Initiatives and Collaborations
Collaboration and cross-disciplinary research are increasingly viewed as essential for tackling complex AI challenges. Here are some initiatives that show promise:
- Partnerships between academia and industry: These collaborations can foster a vibrant exchange of ideas and practical applications. For instance, partnerships like those seen at institutions such as MIT and Stanford help marry theory with real-world needs.
- Involvement in open-source projects: Engaging with open-source communities not only democratizes access to AI research but also accelerates innovation through collective contributions.
- Collaborative research centers: Initiatives like the Partnership on AI bring together a wide array of stakeholders, from technology firms to non-profits, aiming to tackle ethical concerns and advance the responsible use of AI.
The future of AI offers an exciting landscape shaped by ongoing research initiatives, collaborations, and anticipated trends. As we move forward, embracing these developments will be critical to advancing our understanding and application of AI, ensuring that these technologies work in harmony with the complexities of human life.
Closure
The exploration of Melanie Mitchell's work in artificial intelligence illuminates not just her individual contributions but also the intricate tapestry of the field as a whole. Understanding her impact allows us to appreciate the nuances of AI development, particularly in how complex systems operate and evolve. This examination draws attention to areas such as genetic algorithms and machine learning, showcasing the pivotal role these elements play in modern AI.
Summarizing Mitchell's Impact
Melanie Mitchell’s influence stretches across several domains within AI research. One of the standout aspects of her work is the way she integrates concepts from biology, particularly through genetic algorithms, to enhance computational problem-solving. For instance, her book, "Complexity and the Art of Public Speaking," unpacks how emergent behaviors can be understood through simple rules, leading to intricate phenomena, akin to how ant colonies or bird flocks behave.
Furthermore, Mitchell continuously emphasizes the importance of learning from setbacks. Her analyses highlight that understanding failure is as critical as understanding success in AI systems. This perspective is crucial, especially as many current AI models stumble over basic generalization tasks, and her insistence on recognizing these gaps reshapes how researchers approach problem-solving.
"The challenge isn’t just to create smarter algorithms, but to understand the details behind their limitations and potential," reflects Mitchell’s philosophy, underscoring that a comprehensive grasp of both strengths and weaknesses is essential for future development.
Ultimately, Mitchell has carved out a distinctive niche where interdisciplinary knowledge can flourish, inviting others to consider the biological underpinnings of intelligence alongside computational approaches.
The Future of AI Inspired by her Work
Looking ahead, the implications of Melanie Mitchell’s insights are profound for the future of AI research. As we continue to grapple with the inherent complexities of intelligent systems, her emphasis on abstraction and complex systems theory offers a roadmap. Holistic approaches model behaviors that can serve as foundations for designing algorithms, which in turn foster cooperative interactions within systems.
Also, there’s growing recognition of the need for AI to learn in ways that mirror human cognitive processes—combining data, experiences, and environmental cues. Her work suggests that future research initiatives ought to focus on refining these adaptive learning mechanisms.
- Emphasizing Interdisciplinary Research: By bridging disciplines, researchers can pool insights from various fields to address the multifaceted issues present in AI.
- Encouraging Robustness over Perfection: Rather than chasing the ultimate algorithm, future work should center on creating systems that adapt and thrive amid uncertainties and challenges.
In the grand schema, Mitchell's contributions act as both a mirror and a beacon—they reflect the current state of AI while guiding future inquiries into how we define and design intelligence. The path forward is not solely marked by technological advancement but also by a deeper understanding of the principles that govern intelligent behavior, informed by her rich body of work.
Thus, Melanie Mitchell’s influence will continue to inspire a new generation of researchers and practitioners who aim for a balanced exploration of AI's depths and heights.