The Evolving Landscape of Artificial Intelligence
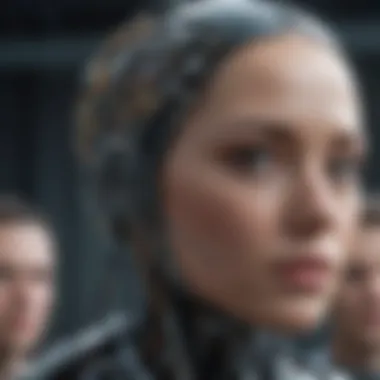
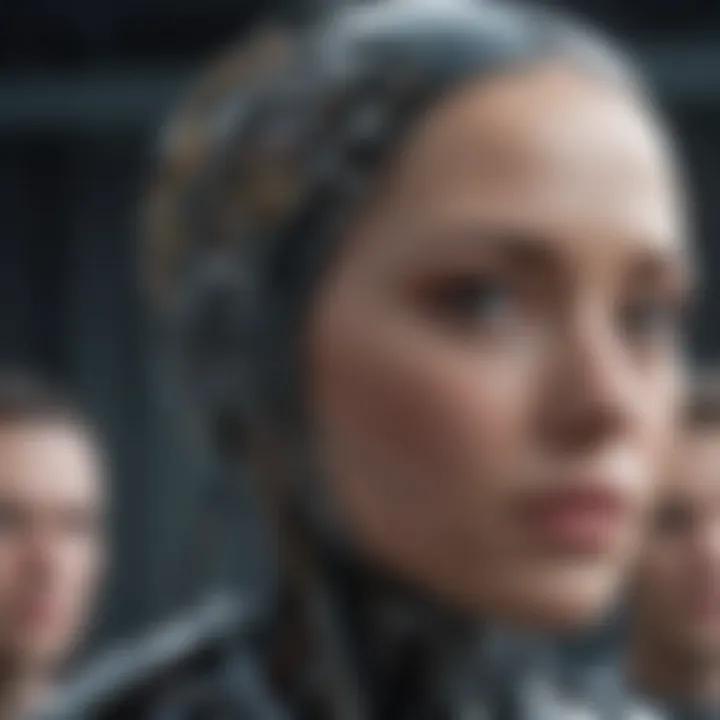
Intro
Artificial intelligence (AI) is no longer just a futuristic concept seen in science fiction movies. It has morphed into a powerful entity that shapes our daily lives, from recommendations on streaming platforms to sophisticated data analytics in healthcare. Understanding this technological marvel requires us to peel back its layers, examining its history, advancements, and future prospects.
In recent years, we’ve seen AI evolve significantly. It is no longer confined to academic research; it has permeated various sectors and presented itself in numerous forms, including machine learning, natural language processing, and robotics. But what really sets AI apart is its multifaceted nature. It integrates theories of computer science, mathematics, psychology, and ethics, creating a rich tapestry of knowledge and application.
Delving into AI's journey means grappling with complex topics while remaining mindful of the practical implications for society. There’s a wealth of information to explore, including key findings in research, the significance of these discoveries, and the essential concepts underpinning AI technology. The objective of this article is to streamline our understanding of AI by weaving these elements together, catering to students, researchers, educators, and professionals alike.
Key Research Findings
As we embark on this exploration, let’s first highlight the pivotal research findings that have marked the trajectory of AI's development. Numerous breakthroughs have emerged in recent years, significantly impacting various fields.
- Overview of Recent Discoveries:
- Significance of Findings in the Field:These research developments aren't just technical feats; they signify a shift in how machines interact with the world. AI now approaches tasks that were once exclusively human domains, raising important questions about labor, ethics, and human-computer collaboration.
- One of the most striking developments has been the evolution of deep learning algorithms. Networks like convolutional neural networks have revolutionized image recognition, enhancing accuracy rates in fields ranging from security systems to retail analytics.
- Natural language processing, particularly through advancements in transformer models like OpenAI's GPT series, has empowered machines to understand and generate human-like text. Such strides provide practical applications in customer service, content creation, and more.
"The advancements in AI have not only presented opportunities for innovation but also ethical imperatives that we must navigate with vigilance."
Breakdown of Complex Concepts
To better grasp the significance of AI’s journey, it’s essential to simplify some advanced theories underpinning these technological innovations. Many terms and concepts can be daunting, but breaking them down can illuminate their relevance.
- Simplification of Advanced Theories:The concept of machine learning, for instance, revolves around the idea that systems can learn from data and improve over time without human intervention. By understanding how algorithms are designed to recognize patterns, we can appreciate their application in forecasting trends in finance or personalizing user experiences in digital platforms.
- Visual Aids and Infographics:Utilizing visual representations can further clarify complex ideas. Infographics illustrating the differences between supervised and unsupervised learning allow for a straightforward understanding of these methodologies.
With AI firmly anchored in today's world, understanding its roots and trajectories becomes essential for anyone wishing to engage meaningfully with this dynamic field.
Understanding Artificial Intelligence
The concept of artificial intelligence has transformed from a novel idea into a powerful force reshaping various sectors of society. Understanding artificial intelligence is paramount in grasping how it impacts daily life, business practices, healthcare, and other fields. By delving into AI, we gain insights into its capabilities and limitations, equipping ourselves to navigate a world increasingly influenced by intelligent systems.
Defining Artificial Intelligence
Artificial intelligence refers to the simulation of human intelligence in machines programmed to think and learn like humans. These systems can process information, solve problems, and sometimes even understand language. More than just algorithms, AI encapsulates a range of technologies that work together to analyze data, recognize patterns, and make decisions. Clearly defining AI helps to distinguish it from simpler computational processes, illustrating its complexity and depth.
The Historical Context
Early Theories
The early theories behind artificial intelligence laid the groundwork for the development of modern AI. Thinkers like Alan Turing and John McCarthy sparked initial debates on whether machines could think. This pivotal question led to defining intelligence in a way that transcends biological constructs. A key character of early theories is their ambitious nature, pushing the boundaries of technology and thought. Their unique feature lies in the radical proposition that machines can be made to possess intelligence analogous to that of humans, a concept that has invited both praise and skepticism. While these theories have not all materialized as expected, they have steadfastly guided the path of future AI research.
Key Milestones
Key milestones in the history of AI serve as guideposts that highlight critical advancements in technology. From the creation of the first neural network in the 1950s to the victory of IBM's Deep Blue over chess champion Garry Kasparov in 1997, these breakthroughs showcase the evolving capabilities of machines. A significant attribute of these milestones is their recurrence, often unveiling new horizons in problem-solving. Their uniqueness stems from showing real-world applications that bolster public interest and investment in AI technologies, fueling a continuous cycle of innovation. However, each leap forward often brings its own set of challenges and ethical dilemmas, demanding responsible development.
Emergence of Machine Learning
The emergence of machine learning marked a substantial shift in artificial intelligence. Unlike traditional programming methods, where rules were explicitly defined, machine learning allows computers to learn from data and improve their performance over time. This characteristic makes it a vital choice for this article, as it underscores how modern AI systems adapt and evolve. The distinct feature of machine learning is its ability to find patterns in vast datasets, leading to predictions and insights that were previously unimaginable. However, one must be cautious, as relying too heavily on automated learning algorithms can pose risks, particularly if those algorithms reflect existing biases.
Core Concepts of AI
Algorithms and Data Structures
Algorithms and data structures form the backbone of artificial intelligence. Without efficient algorithms to process information and suitable data structures to store it, AI would struggle to function. An important characteristic of this core concept is its systematic approach, allowing for the breakdown of problems into manageable parts. This structure is beneficial in ensuring that AI applications run smoothly, providing consistent results across various use cases. However, this can become a double-edged sword; overly complex algorithms can complicate the programming process and lead to unforeseen consequences in AI behavior.
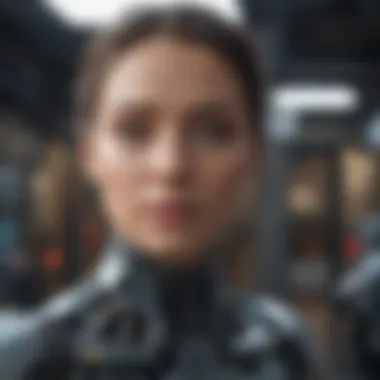
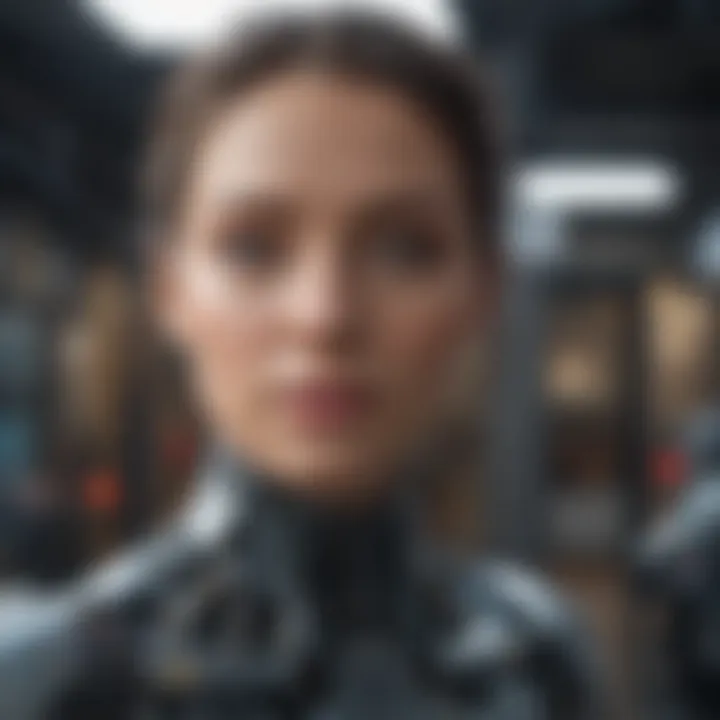
Neural Networks
Neural networks mimic the workings of the human brain, comprising layers of interconnected nodes that process information. Their configuration allows them to capture intricate relationships in data, making them ideal for tasks such as image recognition and natural language processing. The defining factor of neural networks is their depth and ability to learn from vast amounts of data. This makes them an appealing choice for implementing AI in real-world applications. On the downside, these networks can become computationally intensive, requiring significant resources to train effectively.
Natural Language Processing
Natural language processing is a fascinating branch of AI that focuses on the interaction between computers and humans through natural language. Its significance lies in the ability of machines to understand and respond to human speech or text, making technology more accessible and user-friendly. A key characteristic of NLP is its versatility; it is used in applications from chatbots to translation services. However, challenges remain in deciphering context, nuance, and cultural references, which can lead to misunderstandings or miscommunications in AI outcomes.
This comprehensive exploration of AI lays the groundwork for understanding its multifaceted journey and its implications for the future.
Technological Advances
The realm of artificial intelligence is undergoing rapid transformation, fueled by significant technological advances. Understanding the elements that contribute to this progress offers crucial insights into AI's growing relevance in a variety of fields. These developments range from sophistications in hardware to innovations in algorithms, creating an enriching landscape for applications that can enhance or even revolutionize our daily lives. Importantly, examining these advancements is not just about the technology itself but also about the broader implications they hold for society, ethics, and future interactions between humans and AI.
Recent Breakthroughs in AI
In recent years, we’ve witnessed breakthroughs in AI that stand out due to their impact and scope. One significant stride is the development of transformer architectures, which have dramatically improved natural language processing capabilities. Systems like GPT (Generative Pre-trained Transformer) have transformed human-AI interaction by enabling machines to understand and generate human-like text. This is just one thread in the rich tapestry of AI advancements that are pushing the boundaries of what's possible.
AI in Everyday Life
Smart Assistants
Smart assistants such as Amazon's Alexa or Apple's Siri have started to weave themselves into the fabric of our everyday routines. One of the most exciting aspects of these assistants is their ability to respond to natural language commands, making technology feel more approachable. The key characteristic of smart assistants is their personalizability, allowing them to learn from user interactions. However, privacy concerns surround these conveniences, raising important questions about data security.
Personalized Recommendations
Personalized recommendation systems, evident in platforms like Netflix or Spotify, craft tailored experiences for users based on their behavior and preferences. This noteworthy feature increases user engagement significantly. By analyzing user behavior, these systems can suggest content that might not be immediately obvious to the consumer. While beneficial, there is a potential downside: the risk of creating echo chambers where users are only exposed to a narrow band of content.
Automation in Various Industries
Automation is sweeping across various industries, from manufacturing to finance. One standout aspect of this transition is the shift from basic task automation to more complex decision-making processes. The key characteristic of automation lies in its capacity to enhance efficiency and reduce operational costs. However, the unique feature can also lead to job displacement, creating a critical need for dialogue around worker retraining and societal impact as machines assume roles traditionally held by humans.
The Role of Big Data
Data Collection Methods
Data collection methods are pivotal in advances in AI. Techniques such as web scraping and IoT sensors gather vast quantities of data from diverse sources, enriching AI models. The benefit is clear: a more comprehensive dataset leads to more effective model training, enhancing accuracy. Yet, challenges arise regarding user consent and data privacy, making it essential to strike a balance between innovation and ethical considerations.
Data Processing Techniques
Data processing techniques, like distributed computing and parallel processing, allow for the analysis of big data sets at unprecedented speeds. This is a game changer for AI development, as faster processing enables real-time decision-making abilities. These key characteristics make data processing not only a technical necessity but also a competitive advantage in many sectors. On the flip side, the complexity and cost of these techniques can be a hurdle for smaller organizations attempting to leverage AI.
Impact on AI Performance
The impact that data quality and quantity have on AI performance cannot be overstated. High-quality data improves model training, which in turn leads to more capable AI systems. As models become more adept, the potential applications expand further. However, reliance on poor-quality data can severely hinder AI's effectiveness, highlighting the need for diligent data management practices.
"Quality data is at the heart of effective AI; without it, systems may unravel right before our eyes."
In summary, the exploration of technological advances underscores the pivotal role these developments play in shaping the future of artificial intelligence. As our understanding deepens, so does the awareness of the responsibilities that accompany such power.
AI in Science and Research
The realm of artificial intelligence in science and research is a bustling playground where innovation meets exploration. It represents a treasure trove of possibilities, harnessing computational power for breakthroughs that were previously confined to the realm of imagination. Embracing AI means embracing the future of scientific discovery, where the intricacies of life, mathematics, and physical phenomena are decoded and understood at an unprecedented rate.
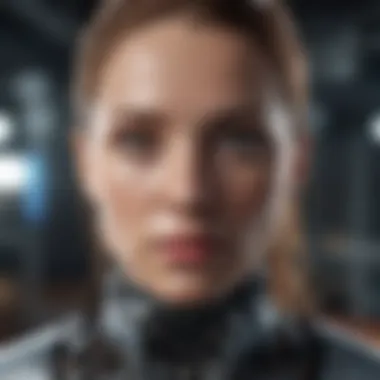
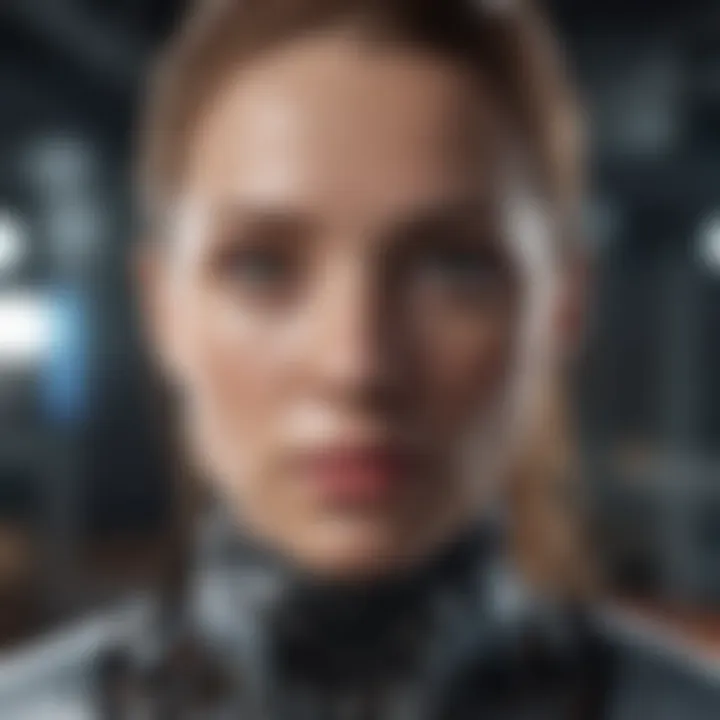
AI Applications in Life Sciences
Genomics
Genomics is at the forefront of AI's application in life sciences. It focuses on using AI to analyze vast amounts of genetic data. One standout feature of genomics is its capability to map human genomes, leading to personalized medicine, where treatments can be tailored to an individual's genetic makeup.
This is a brilliant match for AI since it can process complex data sets quickly and efficiently, providing insights that doctors and researchers traditionally struggled to uncover. The downside, however, is the need for comprehensively annotated data and the potential for misinterpretation in the analysis phase, which can lead to less than optimal treatment outcomes.
Drug Discovery
The drug discovery process, traditionally a lengthy and costly endeavor, has seen a shift with the introduction of AI technologies. By leveraging machine learning models to predict how drugs will interact with biological systems, researchers can now identify viable candidates more rapidly than before. This approach drastically reduces the time taken to develop new pharmaceuticals, which is particularly crucial during health crises like pandemics. However, while AI can streamline initial discoveries, the real-world testing of these drugs remains a meticulous process, often fraught with unexpected results.
Medical Diagnostics
In the medical field, AI’s contribution to diagnostics is becoming increasingly pivotal. From image recognition in radiology to predictive analytics in pathology, AI assists clinicians in identifying diseases early and accurately. One distinctive feature of AI-enabled diagnostics is its ability to analyze patterns in data that may not be visible to the naked eye. This technology not only augments human judgment but can sometimes surpass it, but it does raise concerns about over-reliance on algorithms and the potential blurring of ethical lines in decision-making.
AI and Mathematics
Modeling Complex Systems
Modeling complex systems is a fascinating application of AI in mathematics. This approach allows researchers to simulate phenomena ranging from climate change to biological processes. With the ability to create intricate models, scientists can predict behaviors and outcomes under various conditions. The key characteristic of this technique is its power to reveal interdependencies between components of a system, thus aiding in strategic decision-making. Yet, the accuracy of these models depends heavily on the quality of the data input, and poor data can lead to misleading conclusions.
Statistical Analysis
Statistical analysis has embraced AI to enhance traditional methods. With AI, analysts can sift through mountains of data to identify trends and correlations at breakneck speeds. A prominent aspect is its ability to adapt and improve, learning from new datasets over time to refine its predictions. However, black-box models can make it hard to decipher how these conclusions are drawn, which raises questions regarding the transparency and reproducibility of results.
Optimizing Algorithms
In optimizing algorithms, AI plays a critical role in refining processes across various domains, including logistics and data operations. It enables researchers and companies to find solutions more efficiently by continuously adjusting parameters to achieve the best outcomes. This feature of adaptive optimization means operations can be fine-tuned on-the-fly to respond instantly to changing conditions. The downside? An increasingly complex web of interdependencies can arise, making troubleshooting problematic when things don’t go as planned.
Physics Meets AI
Quantum Computing
Quantum computing represents a groundbreaking frontier in AI, offering the potential to solve problems that were previously thought insurmountable. Its core characteristic is its use of quantum bits, which can exist in multiple states simultaneously, dramatically increasing processing power. It is an exciting part of the discussion around AI because it invites unique algorithms that harness quantum mechanics principles. However, the technology is still in its infancy, and creating stable quantum computers is a well-known challenge, limiting its current practical impact.
Predictive Models
AI-driven predictive models in physics are changing the way researchers approach theory and experimentation. By simulating different physical scenarios, scientists can foresee outcomes more accurately than ever before. The unique feature of these models lies in their capacity to learn from historical data, enhancing predictive power with each iteration. But, just like any model, there’s a risk involved; the assumptions made can skew results, leading to inaccuracies if not properly validated against experimental data.
Simulating Physical Phenomena
The ability to simulate physical phenomena using AI has revolutionized research in various fields, from meteorology to astrophysics. AI models can handle vast datasets, replicating complex interactions in ways traditional methods sometimes cannot. This thorough understanding helps in testing hypotheses and understanding the underlying principles governing physical laws. However, the massive datasets required can be challenging to manage and analyze effectively, sometimes overwhelming traditional computational resources.
"AI's role in science and research is not just a tool but a vital catalyst for knowledge expansion, facilitating understanding in complex areas."
As we can see, AI's multifaceted journey in science and research continues to yield remarkable insights and innovations, dramatically shaping the future landscape of human knowledge.
Ethical Considerations in AI
In an age where artificial intelligence permeates various aspects of life, understanding the ethical considerations surrounding it is essential. Not only does AI shape industries, but it also impacts lives in profound ways. The repercussions of AI decisions can lead to significant societal shifts, hence it is necessary to reflect on the ethical implications. \n \nOne of the core benefits of addressing ethical considerations is that it fosters trust between the technology and its users. When individuals feel that AI systems operate within a framework of fairness and accountability, they are more likely to embrace these innovations. This leads to more widespread acceptance of AI in everyday life. Let's dissect the delicate balance of morality in AI by diving into the nitty-gritty of its development, potential biases, and future risks. \n \n### The Ethics of AI Development
Developing artificial intelligence raises some pretty sticky questions about what it means to act ethically. The main concern is whether the creators of AI are doing their due diligence to ensure that their technologies operate in a just manner. Transparency plays a pivotal role here. When companies give insight into their algorithms and models, they create a layer of accountability that can influence public perception positively. \n \nAnother important point is the notion of responsibility. Developers must consider the long-term implications of their work. For instance, if an AI system perpetuates stereotypes or misinformation, who is held accountable? This opens up a broader conversation about the need for ethical standards that govern AI technologies and their applications. \n \n### Bias and Fairness in AI Systems
This section is a strong reminder that even the most sophisticated AI systems can harbor biases. \n \n#### Sources of Bias
Bias can creep in through various channels, from the data used to train the algorithms to the very design choices made by developers. For example, if an AI model is trained on data that lacks diversity, its outputs will likely reflect those limitations. This is a key characteristic that developers need to address thoroughly. One notable source includes historical bias, where past prejudices are unconsciously integrated into the AI systems. This necessitates a closer examination of the data sets being utilized to train these systems. The unique feature of this issue is that it not only affects the outcomes but also undermines the credibility of AI technologies themselves. \n \n#### Impact on Decision-Making
The bias in AI can have dire consequences on decision-making processes in crucial areas, including hiring, lending, and criminal justice. Biased AI can lead to unfair treatment of individuals or groups, impacting lives and opportunities. A significant characteristic of this issue is its ripple effect. Decisions made by one biased AI system can influence multiple facets of society, amplifying existing inequalities. This becomes a double-edged sword; while AI has the potential to drive efficiency, if unchecked, it can lead to significant discrimination, raising alarm bells about ethical standards in AI development. \n \n#### Mitigation Strategies
To combat bias, organizations must adopt various strategies that provide a more equitable framework for AI systems. One effective approach is to implement diverse data sets during the training phase. This creates a robust model that reflects a wider range of perspectives. Additionally, regular audits of AI systems can help identify and mitigate bias actively. A unique feature of these strategies is their adaptability; they can be tailored to fit various contexts, enriching the discussion around fairness in AI. While these strategies aren’t a perfect fix, they represent a move towards better accountability in AI frameworks. \n \n### Future of AI: Opportunities and Risks
While AI holds promise, it presents significant challenges that cannot be ignored. Understanding the future landscape is crucial for preparing for potential shifts. \n \n#### Job Displacement Concerns
As AI continues to advance, one dilemma that surfaces is job displacement. Automation has a knack for outpacing the traditional workforce, leading to fears that robots might take over various roles. An overarching characteristic of this concern is its relevance to society as a whole. The displacement might not affect everyone equally, exacerbating existing social inequalities. This point is particularly notable, as it raises questions about the workforce's adaptability and the need for upskilling. \n \n#### AI Governance Frameworks
As AI technologies become more integrated into the fabric of society, establishing governance frameworks becomes vital. These structures can oversee the ethical deployment of AI, ensuring accountability and mitigations are in place. A defining feature is their focus on creating standardized guidelines that can align with technological advances. While these frameworks aim to provide a safety net, they may encounter pushback from organizations eager to capitalize on AI without restrictions, leading to an ongoing tug-of-war between innovation and governance. \n \n#### Long-term Societal Impacts
The continued evolution of AI brings questions about its long-term effects on society. The degree to which AI reshapes socio-economic structures, human interaction, and ethical norms remains to be seen. A key characteristic of this uncertainty is its enormity; the implications could range from how we work to our perceptions of privacy. Identifying potential risks, such as increased surveillance or decision-making centralization, is essential in preparing society for a future where AI plays an ever-growing role. \n \nIn essence, addressing the ethical considerations of AI is not merely an academic exercise, but a necessity for ensuring that technology serves humanity in a fair, just, and responsible manner.
Future Directions for AI Research
The field of artificial intelligence is constantly evolving, with new avenues of exploration emerging as technology advances. Understanding the future directions of AI research is crucial, as it highlights the potential benefits, challenges, and considerations that will shape AI's role in society. Notably, this pursuit fosters interdisciplinary collaboration, facilitates human partnerships, and emphasizes the importance of education in AI resilience.
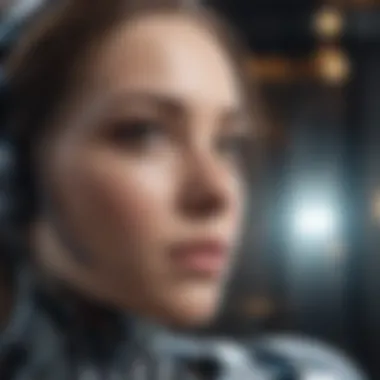
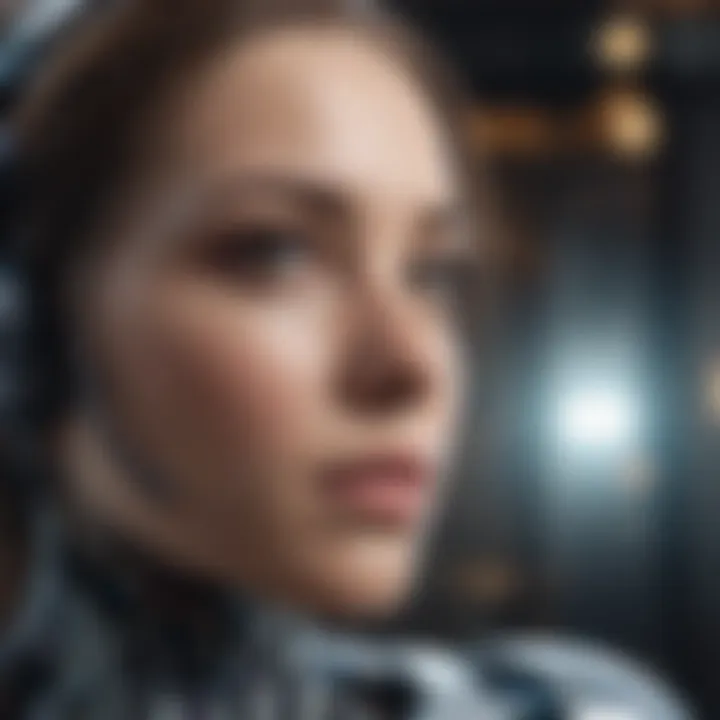
Interdisciplinary Approaches
Integrating AI with Other Fields
Integrating AI with diverse fields represents a cornerstone for fostering innovation. This approach embodies the principle that AI can enhance almost any discipline, from healthcare to environmental science. For instance, utilizing AI in environmental research can optimize energy consumption and tackle climate change more effectively. Its key characteristic lies in the ability to bring together varied expertise—from data scientists to biologists—creating a rich tapestry of knowledge. This multidisciplinary collaboration serves as a beneficial choice, for it enhances the research scope and outcomes.
Incorporating AI into healthcare has unique advantages. It can help predict patient outcomes based on historical data, allowing for proactive healthcare measures. However, this integration also has challenges. Considerations around data privacy and the potential for algorithmic bias must be addressed. The risks involve misinterpretation of the data or reliance on flawed patterns that could ultimately affect patient care.
Collaborative Research Models
Collaboration is becoming increasingly essential in AI research. Collaborative research models facilitate partnerships among universities, corporations, and government agencies, creating a nexus for knowledge sharing. The key characteristic of these models is their ability to pool resources and skills, generating more impactful outcomes than isolated efforts. This synergy is popular in this article context as it encourages diverse perspectives in tackling complex AI challenges.
A unique feature of these models is the pooling of funding and research talent to maximize output. This has advantages, such as accelerated innovation and resource efficiency. On the flip side, coordinating efforts across different entities can lead to friction and inefficiencies if communication is not effectively streamlined.
Global Perspectives
The future of AI also involves embracing global perspectives, promoting cross-border collaborations and sharing of insights. This characteristic is important as AI challenges and opportunities extend beyond national borders. A global approach allows researchers to tackle issues such as climate change or pandemic response through shared knowledge and resources, reflecting the interplay between local specificity and global necessity.
A unique feature of adopting global perspectives lies in the diversity of ideas and solutions that emerge from varied cultural contexts. While this adds richness, it can also create hurdles due to differing regulatory frameworks and ethical standards. Balancing these differences is crucial for coherent progress in AI.
AI and Human Collaboration
Augmenting Human Capabilities
Human-AI collaboration is growing in significance. Augmenting human capabilities through AI allows for enhanced problem-solving, where machines act as supportive tools rather than replacements. The key aspect here is that AI can handle vast data analysis quicker than a human could, providing insights that improve decision-making processes. This dynamic is beneficial because it enables humans to focus on strategic thinking rather than rote tasks.
A unique feature of this kind of collaboration is how it leverages human intuition and creativity alongside AI analytics. Advantages include increased efficiency and novel solutions to long-standing problems. However, there's the risk that over-reliance on AI could lead to skill degradation, where humans might lose their proficiency in essential tasks.
Cooperative Algorithms
Cooperative algorithms represent another layer of human-AI collaboration. These algorithms allow for task-sharing between human intelligence and AI systems, facilitating a cooperative decision-making landscape. This characteristic makes cooperation more smooth, as AI can adapt in real time to human inputs, thus improving outcomes. Such frameworks are a popular choice in this article as they exemplify the shared partnership between human insight and machine efficiency.
A unique feature is that these algorithms can evolve with feedback. While they encourage a more natural interaction style, there's a challenge: ensuring that cooperative algorithms learn correctly without perpetuating biases.
Shared Decision-Making
Shared decision-making takes collaboration a step further by blending AI’s computational prowess with human judgment. This grouping creates complex systems in which AI contributes data-driven recommendations while humans apply situational awareness. The key characteristic of this engagement is its capacity to benefit from both human emotion and AI logic.
This feature is particularly advantageous in sectors where nuance is critical, like healthcare or law. Yet, fostering this balance presents a drawback—humans may struggle with trusting AI recommendations completely, leading to hesitations that can impede the decision-making process.
Educating the Next Generation
Curriculum Development
As we look ahead, developing educational curriculum for AI is essential. The focus here is on integrating AI literacy at various educational levels. Its key characteristic lies in molding a workforce capable of navigating an AI-centric landscape. This choice fosters understanding and prepares future leaders to harness AI's potential effectively.
Unique features involve creating hands-on learning experiences that connect theory with practice. The challenge is ensuring that updates in a fast-paced field like AI are kept within educational frameworks to avoid obsolescence.
Practical Training
Practical training is vital for preparing individuals for a career in AI. This aspect emphasizes experiential learning through internships or real-world project collaborations. Its importance cannot be overstated as it bridges the gap between theoretical knowledge and practical application.
In this article, practical training is a favorable aspect because it provides students with networking opportunities and enhances employability. The downside, however, is that not all students may have access to valuable training experiences, widening the educational gap.
Ethics in AI Education
Incorporating ethics into AI education ensures that future professionals understand the social and ethical implications of their work. This characteristic is necessary to equip learners with the moral compass needed within a rapidly evolving technological landscape. Making ethics a prominent feature of AI curricula is beneficial to encourage responsible AI development and deployment.
The uniqueness comes from creating a framework for discussions on accountability and transparency in technology. This aspect has advantages in establishing a principled approach to AI, although it requires a careful balance so that ethical teachings don’t become dogmatic or stifle innovation.
As we venture into the future, the multidimensional exploration of AI holds the promise of transforming not just technology, but society as a whole. The cross-pollination of ideas across disciplines, the collaboration between humans and machines, and a well-grounded educational approach will shape the AI landscape of tomorrow.