The Impact of Artificial Intelligence on Daily Life
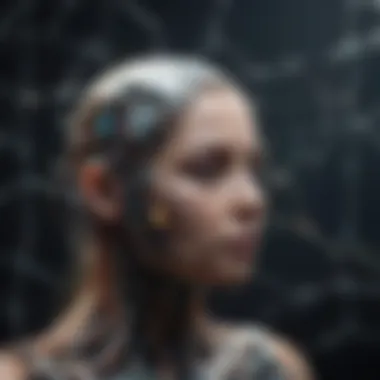
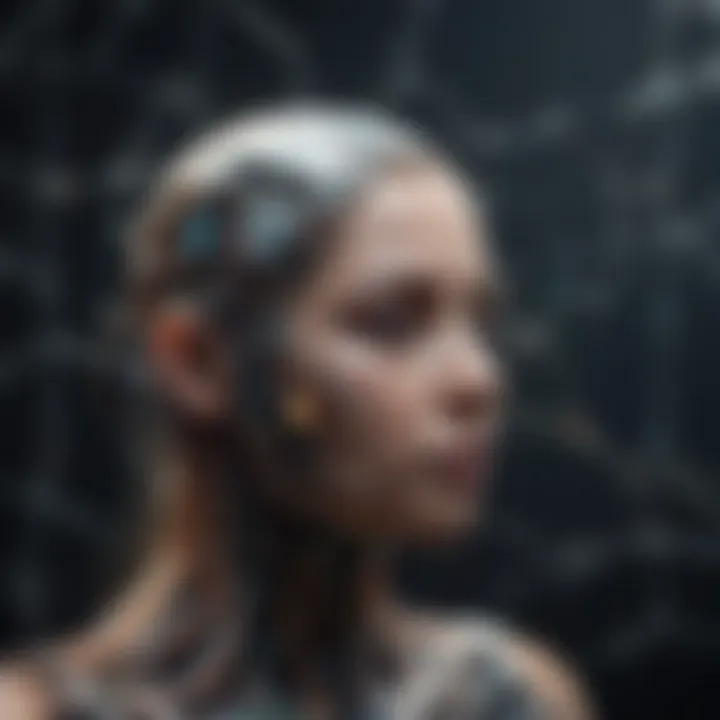
Intro
Artificial Intelligence (AI) does not simply reside in the realm of science fiction anymore. It penetrates our everyday lives, altering how we interact with technology, make decisions, and conduct business. From virtual assistants like Siri and Alexa to sophisticated algorithms that drive recommendations on platforms like Netflix, AI's presence is unmistakable. Often, the uninitiated see it as a black box – a mysterious entity capable of doing wonders. However, we aim to peel back the layers and shed some light on its real-world applications, challenges, and implications for the future.
With the acceleration of AI technology in the last few years, industries have begun to recognize its transformative potential. It's not just about automating tasks; it's about enhancing the value and efficiency of various processes. The healthcare, finance, education, and manufacturing sectors are just a few examples where AI is making a significant impact.
This article focuses on unpacking the myriad ways AI is reshaping the fabric of different industries and the very essence of daily life. As we navigate through each segment, we'll analyze recent research findings, simplify complex concepts, and discuss how AI's influence might evolve.
Let's embark on this journey to discover the intricacies of AI and its burgeoning role in our world.
Understanding Artificial Intelligence
Artificial intelligence has emerged as a vital facet of modern life, permeating various sectors and shaping interactions in ways previously thought unimaginable. The comprehension of AI is more than just understanding a complex algorithm or a machine learning model. It comprises grasping its societal implications, ethical considerations, and transformative potential across industries. Understanding AI lays a foundation that is critical for embracing its advantages while being cognizant of its limitations. This section serves as a guide that leads the reader through essential aspects of AI, its historical background, and various types to paint a comprehensive picture of its role in our everyday dealings.
Defining AI
Artificial intelligence can be comprehensively defined as the capability of a machine to imitate intelligent human behavior. At the heart of this definition lies the pursuit of replicating cognitive functions such as learning, reasoning, and problem-solving. AI is not a single entity but a collection of technologies that work together to mimic cognitive functions. This broad definition indicates that AI is not merely about robots or complex computer programs; it encompasses a vast range of applications from natural language processing to machine vision.
Historical Context
Early developments in AI
The early developments in AI date back to the mid-20th century, when pioneers like Alan Turing and John McCarthy laid the groundwork by theorizing about machines that could think. One key characteristic of early AI was its theoretical nature; it mainly existed in academic papers and hypothetical discussions rather than practical applications. This foundational period allowed for essential ideas about machine intelligence to flourish, leading to what would become known as the Turing Test, a pivotal concept in evaluating a machine's ability to exhibit intelligent behavior. The benefit of understanding these early developments helps contextualize how far technology has come and the ongoing pursuit of creating machines that can think and learn.
Key milestones in AI history
As AI evolved, certain milestones can be identified as critical turning points in its development, such as the creation of the first neural networks in the 1950s or IBM's Deep Blue defeating world chess champion Garry Kasparov in 1997. Each of these milestones has contributed to the ongoing conversation about AI's capabilities and limitations. A notable feature of these milestones is how they showcase AI not just as a tool but as a formidable opponent in intellectual tasks. By exploring these pivotal moments in AI history, we gain insights into the persistent questions surrounding its potential and ethical ramifications.
Types of AI
Strong vs. Weak AI
When discussing types of AI, a fundamental distinction is made between strong AI and weak AI. Strong AI, which aims to create machines that genuinely understand and reason, is more of a philosophical concept than practical reality today. Weak AI, on the other hand, refers to systems designed to perform a specific task without genuine understanding. This distinction is crucial for recognizing how certain AI applications function in our current landscape and assists in setting realistic expectations regarding AI's capabilities. The unique feature of weak AI is its practical utility in applications like voice assistants or recommendation algorithms, making it a popular choice across various industries.
Supervised vs. Unsupervised Learning
Another important categorization within AI is the separation between supervised and unsupervised learning. Supervised learning involves training a model on labeled data, where the outcome is known. In contrast, unsupervised learning relies on finding hidden patterns in data without pre-existing labels. This differentiation is significant in determining how AI systems are developed and trained. Each approach presents its own set of advantages and challenges; supervised learning often yields precise outcomes in areas like image recognition, while unsupervised learning can unveil hidden structures in data, which might prove invaluable for large datasets. Both techniques highlight AI's versatility and adaptability, allowing for a range of applications from customer segmentation to sales forecasting.
"Understanding AI is not just understanding machines; it’s about understanding the future of human interaction with technology."
This section lays the groundwork for appreciating the intricacies of AI, emphasizing that as technology progresses, so too must our understanding of its various implications in day-to-day life. By exploring how AI is defined, historical landmarks that shaped it, and the different types of AI, we set the stage for deeper discussions in the sections that follow.
AI in Healthcare
The healthcare sector is undergoing a seismic shift as artificial intelligence continues to integrate into various processes, reshaping patient care, diagnostics, and research. By harnessing massive amounts of data, AI systems pave the way for more efficient and accurate medical practices. As we delve into this topic, it becomes clear that AI not only enhances operational efficiencies but also drives significant improvements in patient outcomes. The emphasis on precision, speed, and adaptability solidifies AI's role as a pivotal element in the modern healthcare landscape.
Diagnostic Systems
Image recognition in diagnostics
Image recognition stands at the forefront of revolutionizing diagnostic systems. This technique involves the use of advanced algorithms that can analyze medical images, such as X-rays, MRIs, and CT scans. One of the key characteristics of image recognition in diagnostics is its high accuracy and consistency in identifying anomalies. The technology leverages deep learning methods, allowing it to learn from vast datasets and improve over time.
The unique feature of image recognition is its ability to minimize human error, optimizing the diagnostic process especially in conditions where even a seasoned professional might overlook critical details. However, while the benefits are substantial, it's crucial to address the challenges of dependence on technology and the necessity for human oversight. Human intuition and expertise still play a fundamentally important role in the diagnostic journey.
Predictive analytics in patient outcomes
Predictive analytics takes advantage of data and statistical algorithms to forecast patient outcomes, reshaping the way healthcare professionals approach treatment plans. The vital aspect here is the ability to analyze historical data and trends, which allows for informed decision-making. For instance, predictive models can indicate which patients are at risk of developing certain health conditions, making this a game changer in preventative care strategies.
One of the standout features of predictive analytics is its capacity for personalization. Being able to tailor interventions based on individual patient data means providing care that is not just reactive but proactive. There are drawbacks, though; the reliance on accurate data and the ethical concerns surrounding patient privacy can complicate its application. Nonetheless, the insights offered by predictive analytics are invaluable for enhancing patient care and operational efficiencies.
Personalized Medicine
Tailoring treatment plans
Tailoring treatment plans has emerged as a fundamental practice within personalized medicine. This approach focuses on the individual characteristics of each patient—such as genetics, lifestyle, and preferences—to determine the most effective treatment protocols. One of the distinguishing characteristics of this strategy is its adaptability; treatments are adjusted based on real-time data and responses, leading to higher success rates.
The unique feature of tailoring treatment plans lies in its ability to move away from the one-size-fits-all methodology. Patients receive care specifically designed for their unique situations, which can significantly enhance trust and satisfaction. However, this personalized method does require comprehensive data collection and analysis, raising concerns about data security and patient consent.
AI in genetic research
Artificial intelligence is also making waves in the realm of genetic research. Its introduction has accelerated the analysis of genetic data, allowing for deeper insights into genetic disorders and polygenic traits. A key aspect of AI in genetic research is its efficiency; it can process and interpret vast datasets at speeds unattainable by traditional methods, making it a indispensable tool in deciphering the complexities of the human genome.
One particularly beneficial feature is the ability to identify correlations between genetic markers and diseases. This paves the way for new treatment avenues and preventive measures. However, as promising as it seems, AI’s application in genetic research comes with challenges, particularly concerning ethical considerations and the potential for misinterpretation of data. Navigating these waters carefully will determine how AI reshapes the future of genetic medicine.
AI in Finance
Artificial intelligence is making waves in the finance sector, bringing a transformative influence to how transactions, trading, and investments are managed. In an industry where every second counts, AI provides the agility needed to process vast quantities of data in real time. The financial landscape is replete with complexities, and integrating AI into finance helps streamline operations, enhance security, and improve decision-making processes. A major allure of implementing AI in finance lies not only in efficiency but also in its potential to identify patterns and trends that humans might overlook.
Algorithmic Trading
Data analysis for trading strategies
Data analysis for trading strategies is a critical pillar of algorithmic trading. This technique employs algorithms that sift through massive datasets, identifying trends and predicting market movements with remarkable precision. By leveraging historical data, traders can construct and backtest strategies, refining them to minimize risk and maximize returns. A key characteristic of this approach is its ability to operate 24/7 without the fatigue that can impair human traders in high-pressure environments.
The primary benefit of data analysis in trading is that it quickly adapts to changing market conditions. Instead of relying on gut feeling or intuition, traders base their decisions on solid evidence extracted from the data. Nonetheless, there are unique features that come with this. On the downside, reliance on data can lead to overfitting—where a model becomes too tailored to past data, rendering it ineffective in new conditions.
Market prediction models
Market prediction models utilize sophisticated AI techniques to forecast future price movements based on historical trends and various indicators. These models incorporate elements from econometrics and machine learning, thus providing insights into potential future performance. By analyzing market sentiment, economic indicators, and trading volumes, traders can make educated predictions about fluctuations in prices.
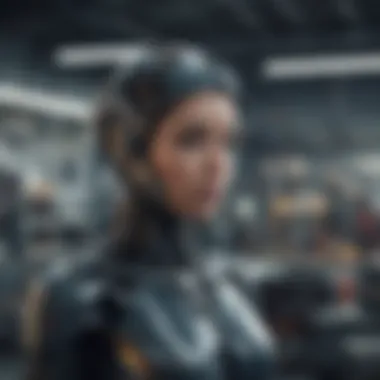
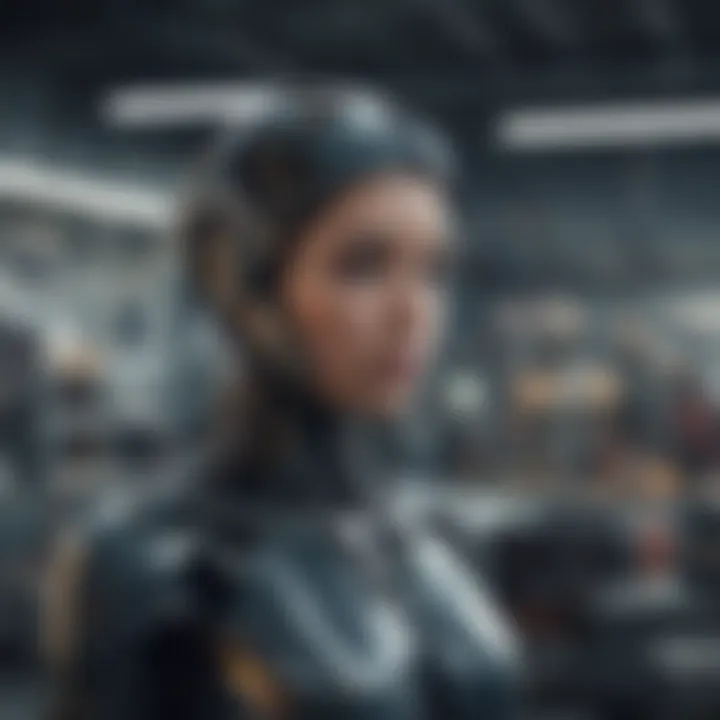
What makes these models beneficial is the incorporation of big data analytics, which offers a broader scope for identifying risks and trends. As more financial transactions go digital, the algorithms can harness rich datasets to improve accuracy. However, the unique challenge with market prediction models is that markets are inherently unpredictable.
Fraud Detection
AI-driven transaction monitoring
AI-driven transaction monitoring is revolutionizing how financial institutions combat fraud. By using machine learning models that continuously learn from transaction data, AI algorithms can detect unusual patterns or activities that indicate fraud. This system operates almost in real-time, ensuring swift action can be taken to prevent suspicious transactions from being processed.
The foremost advantage of this model is its ability to analyze multiple signals at once, something human analysts might struggle to accomplish. Yet, a unique feature is the false positives—transactions that may be flagged incorrectly. This not only annoys customers but can also result in lost revenue. It’s a delicate balance between vigilance and customer experience.
Anomaly detection techniques
Anomaly detection techniques enhance fraud detection by identifying deviations from established norms within transaction data. These algorithms comb through data to find outliers that could suggest fraudulent activity or operational issues. One of the strengths of anomaly detection is its versatility across different types of transactions, allowing it to be tailored for various banking systems or environments where fraud might occur.
However, like any method, it's not without its drawbacks. Advanced fraudsters can sometimes exploit knowledge of how detection systems work. Thus, it's a continual game of cat-and-mouse between financial institutions and criminals. Moreover, if the algorithms are not regularly updated, they can become less effective over time as fraudulent methods evolve.
The use of AI in finance is both a promise and a challenge, requiring constant adaptation and vigilance.
By harnessing the power of AI in finance, organizations can realize unprecedented levels of efficiency and security, enhancing their overall capability in detecting and mitigating risks. Navigating the complexities, however, requires a tactical approach to implementation and continuous improvement.
AI in Transportation
Artificial intelligence has left a profound imprint on transportation, significantly influencing how we navigate our world. From self-driving cars to intelligent traffic management systems, AI is a driving force that enhances safety, efficiency, and sustainability. The incorporation of AI in transportation isn't merely about automation; it's about redefining the very essence of our mobility.
Autonomous Vehicles
Sensor technologies
Sensor technologies are the backbone of autonomous vehicles. These sensors, such as LiDAR (Light Detection and Ranging) and cameras, collect data about the vehicle's surroundings. The ability to detect obstacles, interpret traffic signs, and recognize pedestrian movement makes these technologies essential. A key characteristic is their ability to function in real-time, processing vast amounts of information swiftly.
This is crucial because any delays in data processing could lead to accidents. Moreover, sensor technologies enable vehicles to share information with each other and with traffic management systems, creating a networked approach to transportation. However, they also come with challenges, such as high costs and the need for continuous maintenance, which can limit their appeal in some scenarios.
Impact on urban development
The impact of autonomous vehicles extends beyond personal transport to urban development at large. One significant aspect is that they could actually reduce the need for parking spaces. With self-driving cars, it’s feasible for vehicles to drop off passengers and park themselves away from crowded areas, leading to more green spaces and less congestion in city centers.
This creates an exciting opportunity for urban planners to rethink the layout of cities, focusing on pedestrian-friendly designs and sustainable infrastructure. A unique aspect of this is the potential for cities to become more livable and less car-centric. Yet, there’s the flip side; infrastructure must evolve to accommodate this technology, requiring heavy investment and long-term planning that could prove challenging for many municipalities.
Traffic Management Systems
Real-time data adaptation
Real-time data adaptation is one of the cornerstones of effective traffic management systems powered by AI. By using data from various sources, including traffic cameras and GPS, these systems can adapt to changing traffic conditions instantaneously. This capability allows for improved traffic flow and safety on roads.
For instance, if there’s an accident or a sudden congestion, the system can quickly reroute vehicles, thereby minimizing delays. The standout feature here is the efficiency in decision-making that AI brings to the table. However, the challenge lies in ensuring data accuracy and managing the sheer volume of information generated daily, which requires robust systems and technologies.
Reducing congestion through AI
Reducing congestion through AI-driven systems is not merely a buzzword; it’s a vital goal for cities worldwide. AI tools are designed to analyze traffic patterns and predict peak times, enabling better traffic signal timing and route planning. The key characteristic of this approach is its predictive capability, which allows for proactive rather than reactive traffic management.
For example, cities that employ AI might notice a reduction in rush hour delays, much to the relief of commuters. A unique aspect of such systems is their potential to decrease carbon emissions by optimizing traffic flow. However, the hurdles remain, especially regarding privacy concerns tied to data collection and dependency on technology that could fail due to various factors like software bugs or cyber-attacks.
"AI in transportation promises safer roads and smoother commutes, but we must navigate the complexities that come with it."
AI in Agriculture
The integration of artificial intelligence in agriculture marks a transformative shift in how food is produced and managed globally. This chapter focuses on the multifaceted impact AI has within the agricultural sector, addressing essential elements that showcase its benefits while also delving into considerations that farmers and stakeholders need to keep in mind. AI enhances crop yields, optimizes resource utilization, and ultimately contributes to a more sustainable food production system, crucial for meeting the demands of a growing population.
Precision Farming
Precision farming stands as a beacon for modern agriculture, representing a tech-savvy approach to farming. It utilizes AI tools like drones and sensors to gather data that can lead to better decision-making and improved crop outcomes. The essence of precision farming lies in its ability to monitor and manage the variability in crops, resulting in a plethora of benefits for the environment and the economy.
Use of Drones and Sensors
Drones and sensors have become pivotal in precision farming. These technology-driven tools collect real-time data from fields, making pest and nutrient management much more efficient. The hallmark of drones is their capability to cover large areas quickly, delivering aerial imagery that helps in assessing crop health.
This makes the use of drones an appealing option for farmers looking to gain a competitive edge. The unique feature of these drones is their ability to provide high-resolution imagery and real-time analysis, enabling proactive measures rather than reactive ones.
However, while the benefits are substantial, there are also challenges involved such as initial costs and the steep learning curve associated with operating the technology.
Optimizing Resource Usage
AI provides the tools to optimize resource usage, a crucial element for sustainable farming. Through data collected from various inputs, farmers can make informed decisions about when and how much water, fertilizers, and pesticides to apply.
The key characteristic of resource optimization is its potential to reduce waste while maximizing yield. This is particularly beneficial in areas where water scarcity is a pressing issue. Unique in its approach, this system analyzes soil health, weather data, and crop requirements to advocate for targeted application, hence enhancing overall efficiency.
Yet, this requires an upfront investment in technology and can lead to reliance on data accuracy. Misinterpretations can have serious repercussions on crop yield.
Crop Monitoring
The role of AI in crop monitoring has expanded dramatically, enabling farmers to predict outcomes and make adjustments in real-time. This aspect of AI encompasses various methodologies, each looking to improve the quality and quantity of agricultural output.
Predictive Analytics for Yield Forecasting
Predictive analytics has carved a niche in yield forecasting. By utilizing patterns from previous harvests and current environmental conditions, AI systems can forecast crop yields with impressive accuracy. This characteristic is vital for farmers in their planning and supply chain management.
The unique feature of predictive analytics is its capability to process vast amounts of data quickly. This leads to timely insights that guide planting and harvesting schedules. While the advantages are clear in terms of efficiency and planning, reliance on algorithms could sideline the importance of local knowledge and experience that farmers often possess.
AI in Pest Management
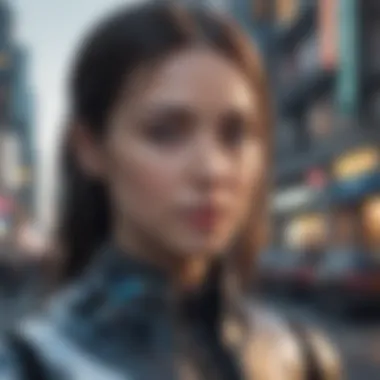
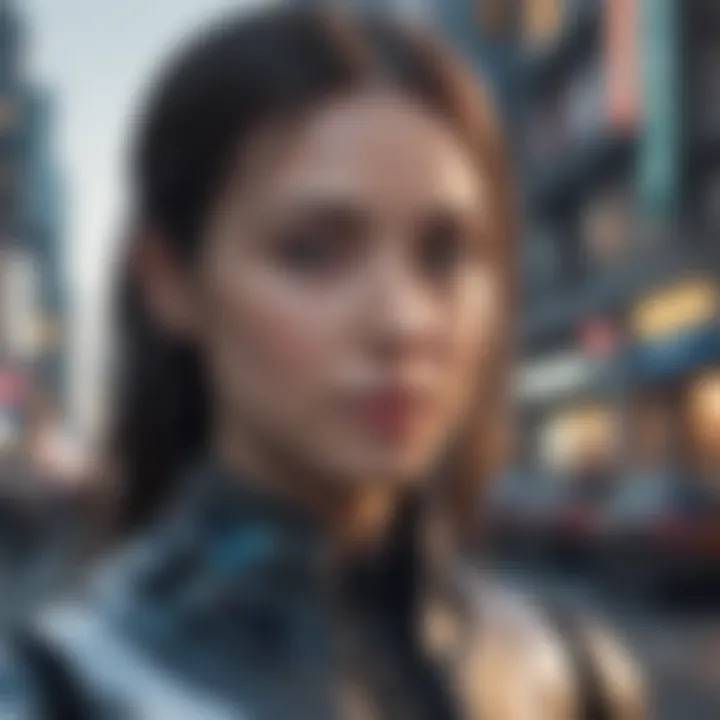
Using AI for pest management offers significant enhancements to traditional practices. This involves monitoring pest populations and identifying potential outbreaks through advanced imaging and machine learning algorithms.
The core characteristic of AI in pest management lies in its ability to adapt and learn from the data it gathers over time. This places it as a favorable choice for farmers looking to minimize chemical usage while maximining effectiveness. A unique opportunity provided by this technology is the potential for integrated pest management solutions that align with sustainable practices.
Nevertheless, there are challenges like dependency on technology and concerns about managing pest resistance that need careful consideration.
"AI in agriculture will not replace farmers; it will empower them, enabling smarter practices for a more sustainable future."
In summary, AI's role in agriculture is both expansive and essential. From drones aiding in crop health assessments to predictive analytics ensuring higher yields, the potential benefits are significant. The landscape of farming is undeniably changing, and with it comes the promise of a more efficient and sustainable approach to feeding the world.
AI in Retail
The retail sector is undergoing a seismic shift fueled by advancements in AI technology. In today's highly competitive market, the application of artificial intelligence is not just a luxury but a necessity for retailers wishing to enhance their operations and customer experience. From optimizing inventory management to personalizing shopper interactions, AI plays a crucial role in driving efficiencies and engagement that ultimately increase sales and customer loyalty. In exploring AI's role in retail, one sees not only its transformative power but also the challenges and considerations that come with it.
Customer Experience Enhancement
Personalized shopping experiences
Personalized shopping experiences stand at the forefront of retail innovations powered by AI. This approach leverages customer data—like purchasing history, browsing behavior, and demographic information—to tailor product recommendations and marketing strategies. The key characteristic of this method is its ability to make customers feel valued and understood, which translates to higher satisfaction and loyalty.
A unique feature of personalized shopping is the dynamic adjustment of offers based on real-time data. This not only enhances the customer’s journey but also increases the likelihood of purchases. However, there are challenges; for instance, the risk of data privacy issues can lead customers to feel unsettled about the way their information is being used.
In summary, personalized shopping experiences offered by AI can result in profound benefits, such as improved customer engagement and increased sales, while demands for ethical data usage present a significant consideration.
Chatbots and virtual assistants
Chatbots and virtual assistants are revolutionizing customer service in retail by providing immediate responses to customer inquiries and guiding them through purchases without needing human intervention. One remarkable characteristic of these AI tools is their availability 24/7—a feature that modern consumers, who often operate on busy schedules, find invaluable.
The unique ability of chatbots to learn from interactions allows them to improve over time, offering more accurate and helpful responses. This adaptability makes them a popular choice for retailers aiming to streamline customer service and improve efficiency. Yet, relying solely on AI can sometimes lead to frustration if the bots misinterpret customer requests or lack the ability to handle complex issues, highlighting a potential disadvantage in high-stakes situations.
Thus, while chatbots and virtual assistants enhance customer experience by providing instant support and encouraging engagement, balancing their use with human oversight is critical to maintain high service quality.
Inventory Management
Predictive analytics in stock management
Predictive analytics in stock management utilizes historical sales data and market trends to forecast future product demand. This capability can significantly aid retailers in making informed decisions regarding stock levels and ordering schedules. The main advantage here is that it helps to reduce instances of overstocking or stockouts, both of which can negatively impact revenue.
A noteworthy feature of predictive analytics is its ability to analyze vast amounts of data quickly and accurately. This makes it an attractive choice for retailers looking to optimize their operations. However, limitations exist, such as the reliance on data quality. Poor data can lead to erroneous predictions and misguided decisions, emphasizing the importance of data accuracy and cleanliness.
In essence, predictive analytics offers great potential to refine stock management practices, though retailers must navigate the complexities of data handling to truly reap its benefits.
Automating supply chain processes
Automating supply chain processes involves using AI tools to streamline various logistics and operational tasks. This encompasses everything from inventory tracking and shipment scheduling to order fulfillment. The notable advantage of automation in this context is efficiency; it reduces the need for manual intervention and minimizes human error, enabling faster, more reliable operations.
The unique aspect of automating these processes is the real-time information flow it facilitates, allowing for quick adaptations to supply chain issues as they arise. While the efficiency gains are substantial, one must consider the potential downsides, such as the upfront costs associated with implementing such technology and the need for ongoing maintenance.
Therefore, while automating supply chain processes can significantly boost operational efficiency and reliability for retailers, the initial investment and management requirements are factors that must be carefully weighed.
"AI in retail isn't just about selling more products; it's about creating a customer-centric experience that builds loyalty and trust."
AI in Education
Artificial Intelligence is making ripples in the educational landscape, changing how knowledge is imparted and absorbed. The incorporation of AI in education provides insights that can revolutionize the learning experience. Students, educators, and academic institutions can benefit from tailored learning environments, making education far more personalized and efficient.
Intelligent Tutoring Systems
One of the most significant features of AI in education is the development of Intelligent Tutoring Systems (ITS). These systems offer tailored instruction to students based on individual needs and learning styles. Unlike traditional classroom setups, ITS adapts in real-time to the learner’s performance, creating an environment that is both supportive and stimulating.
Adaptive learning techniques
Adaptive learning techniques sit at the heart of Intelligent Tutoring Systems. By using algorithms to analyze the responses and engagement levels of students, these systems modify the difficulty and subject matter instantaneously.
- Key characteristic: The ability to adjust content based on the learner's performance.
- Why it's beneficial: It allows students to progress at their own pace, which is crucial for effective learning. Each learner’s journey can be unique, which is often difficult to achieve in conventional settings.
- Unique feature: These systems can incorporate various learning mediums—videos, quizzes, and interactive content—to cater to different learning preferences.
The advantages include heightened engagement and improved retention of information. On the downside, however, it sometimes lacks the human element in teaching, which can be vital for certain students who require social interaction.
Assessing student performance
Another critical aspect of AI's role in education is assessing student performance effectively. AI can provide detailed analytics that goes beyond simple grades. It digs into patterns of performance, engagement, and even emotional responses to learning content.
- Key characteristic: In-depth data analysis that includes various metrics.
- Why it's beneficial: Educators can identify struggling students early on and provide timely interventions. A clear picture of individual strengths and weaknesses helps in crafting tailored support.
- Unique feature: Predictive analytics can inform instructors about potential future difficulties based on observed trends in a student's performance.
While detailed assessment provides insights for immediate actions, it may also lead to stress and anxiety for students who feel constantly evaluated. Striking a balance remains a challenge.
AI in Administrative Tasks
AI's impact on educational institutions extends beyond classrooms; it significantly streamlines administrative tasks. By automating mundane tasks, it frees up time for educators to focus on what matters most—teaching and engaging with students.
Streamlining admissions processes
AI is instrumental in simplifying admissions processes. Automated systems can sieve through applications and help in spoting candidates who fit well with the institution's ethos and requirements.
- Key characteristic: Efficient sorting of applications.
- Why it's beneficial: Institutions can save several hours since manual processing can be laborious and prone to errors. The focus shifts to evaluating candidates more thoughtfully.
- Unique feature: AI can also analyze the historical success of past applicants to inform and improve future admissions criteria.
This not only accelerates admissions but also enhances the quality of candidates admitted. However, it raises questions about fairness and transparency in selection.
Data analysis for resource allocation
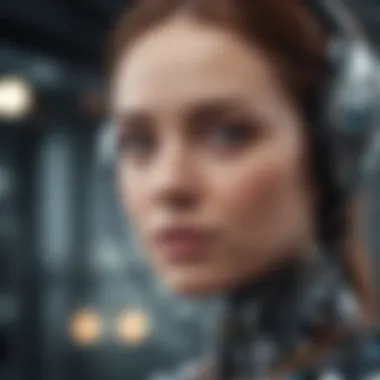
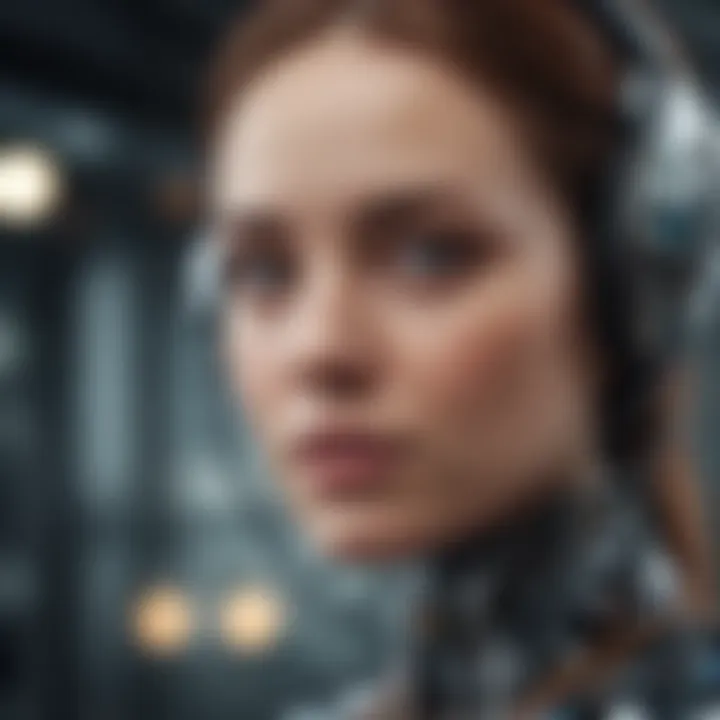
Lastly, data analysis for resource allocation is yet another area where AI shines. Institutions can utilize algorithms to study usage patterns and allocate resources—be it faculty, classrooms, or technology—more efficiently.
- Key characteristic: Utilization of comprehensive datasets to inform decisions.
- Why it's beneficial: Strategic planning becomes much more effective, resulting in better educational outcomes and optimized expenditure.
- Unique feature: Powerful forecasting tools can predict future enrollment trends, helping institutions prepare for their academic needs ahead of time.
However, relying solely on data might overlook the nuances of human behavior, and institutions must consider qualitative insights for comprehensive planning.
In summary, AI's involvement in education offers transformative possibilities, with new systems improving both learning experiences and administrative efficiency. While these technologies come with challenges, the potential benefits make them an exciting frontier for educators and students alike.
Ethical Considerations
In the rapidly evolving landscape of artificial intelligence, ethical considerations take center stage. They serve as a compass, guiding both developers and users to navigate the complexities that arise from the integration of AI into daily life. Understanding the implications of AI not only helps establish guidelines for responsible use but also fosters trust among society. As AI systems become more ingrained in our lives—from healthcare to finance—the ethical questions surrounding their deployment must be thoroughly examined.
Bias and Fairness
Recognizing biases in AI algorithms
At the heart of AI applications lies algorithms, which can often inherit biases from the data they learn from. Recognizing these biases is crucial because they can perpetuate stereotypes and lead to unequal treatment across different demographics. For instance, if a hiring algorithm is trained primarily on resumes from successful employees in a particular group, it may unintentionally favor candidates from that same background while disadvantaging others. The key characteristic of recognizing biases is its role in ensuring fairness, which is indispensable for public acceptance of AI technology. It highlights the unique feature of a more inclusive approach, drawing from diverse datasets to mitigate biases.
The advantages of acknowledging bias come with the opportunity to improve AI models, promoting fairness in decision-making and fostering a more equitable society. However, the downside might include resistance from organizations unwilling to alter existing practices or invest in bias mitigation solutions.
Impact of AI decisions on society
Every output that an AI system generates can ripple through society in unpredictable ways. The impact of AI decisions on society cannot be overstated; it shapes public perception, policy, and the very fabric of social interaction. A fundamental element of this impact is accountability. Who is responsible when an AI makes a mistake?
Recognizing the influence that AI possesses encourages developers to adhere to ethical practices, ensuring that the consequences are foreseeable and manageable. The unique feature here lies in how organizations that prioritize ethical AI can enhance their reputation and build trust with their stakeholders. However, overlooking these considerations might lead to disastrous societal consequences, ranging from misinformation to reinforcement of discriminatory practices.
Privacy and Security
Data usage concerns
As AI systems often require large volumes of data to function, data usage concerns emerge prominently in ethical discussions. Not only do individuals worry about how their personal information is used, but they also question the transparency of AI processes. This aspect of privacy is vital since it drives public trust in these technologies. Protecting user data means prioritizing ethical data collection and usage, which can significantly influence how AI systems gain acceptance in various sectors.
Privately held data can be incredibly sensitive, and improper handling can lead to severe breaches of trust, making organizations rethink their data strategies. The upside of addressing data usage concerns includes fostering a culture of accountability; however, it requires organizations to implement robust data governance frameworks, which can be both time-consuming and costly.
Regulations and compliance
Compliance with regulations surrounding AI is not just a legal obligation; it's also a certainty of public trust. Regulations and compliance are paramount in guiding ethical AI development, facilitating fair practices while ensuring users' rights are protected. This aspect of ethical considerations plays a vital role in shaping industry standards and expectations. Regulations can propel organizations to adopt more thoughtful processes in AI development.
The unique feature of rigorous compliance is its power to instill confidence in consumers. A disciplined approach to ethical guidelines can distinguish brands in a crowded marketplace. However, navigating the labyrinth of regulations could be a double-edged sword, potentially imposing costs and slowing innovation if not managed thoughtfully.
"Ethical AI is not just a technology issue; it's a societal one that demands ongoing dialogue and action."
Future Trends in AI
The landscape of artificial intelligence is in a near-constant state of flux, with advancements paving the way for transformative changes across multiple sectors. Understanding Future Trends in AI is crucial to grasp how these technologies could reshape everything from day-to-day tasks to global economies. By focusing on elements like the evolving nature of the workforce and the innovations within AI technologies, we can see both the opportunities and challenges that lie ahead.
AI and the Workforce
Automation and employment dynamics
At the cusp of a new era, automation has emerged as a key feature shaping employment dynamics. As AI systems become increasingly integrated into workplaces, they offer a fascinating blend of efficiency and cost savings for businesses. One noteworthy characteristic of automation is its potential to handle mundane and repetitive tasks, which can free up human workers to engage in more complex and creative pursuits. In this context, it’s not merely about replacing jobs; rather, it offers a chance to redefine job roles.
However, the integration of automation prompts a range of concerns. Many feel apprehensive that this shift could lead to job displacement, particularly in sectors reliant on routine tasks. An advantage of automation lies in its scalability; organizations can ramp up or scale back operations seamlessly, responding to market changes more fluidly. Yet, the challenge remains that not all workers can easily transition into roles that demand higher skill sets or creative thinking. Understanding both sides—opportunity and risk—is essential.
Skill requirements for future jobs
In light of these advancements, the skill requirements for future jobs are shifting dramatically. The most pressing aspect here is the need for workers to adapt to a rapidly-changing environment. With AI increasingly embedded in various professions, workers are now required to possess a mix of technical and soft skills. Critical thinking, adaptability, and an understanding of AI and data analytics are becoming essential traits.
This evolution in skill sets is beneficial as it encourages lifelong learning and offers growth opportunities across industries. It pushes educational institutions to revamp their curricula, preparing students for a future where jobs may not even exist yet. However, a unique challenge arises: many older workers might find themselves at a disadvantage given the speed at which these changes unfold. Access to reskilling resources becomes an important consideration, making it a potential barrier for some individuals.
Advancements in AI Technology
Continual learning approaches
One significant advancement in AI technology is the development of continual learning approaches. Unlike traditional models that require retraining from scratch, continual learning allows AI systems to adapt by learning incrementally from new data. This means they can retain previously learned information while integrating new inputs. Such flexibility is vital for applications in rapidly changing environments, like financial markets or online retail.
The key characteristic here is the ability to evolve with time. This is a beneficial feature as it reduces the computational resources needed for re-training, making AI deployment more efficient. However, continual learning comes with its own set of challenges, such as overcoming issues like catastrophic forgetting, where new information can unintentionally erase older knowledge. Navigating these complexities is essential for realizing the full potential of AI systems.
Interdisciplinary collaborations
Lastly, the charm of interdisciplinary collaborations is gaining recognition in AI advancements. By fostering partnerships across different fields—like computer science, sociology, psychology, and even the arts—innovators can develop more holistic AI solutions. The key characteristic of this trend is its capacity to integrate diverse perspectives, which can lead to more inclusive and effective AI applications.
This kind of collaboration is incredibly advantageous as it fosters a rich tapestry of ideas, promoting innovation that might not emerge in isolated silos. However, the challenge lies in effectively managing these collaborations; aligning goals across diverse teams can be a daunting task. Successful interdisciplinary efforts can lead to groundbreaking solutions that fundamentally change societal norms and behaviors.
End
In reflecting on the journey through the multifaceted realm of artificial intelligence, it becomes increasingly apparent that AI’s impact touches every corner of modern life. The concluding section serves not only to synthesize the insights shared but also to underscore the significance of understanding AI’s evolving role in our day-to-day activities and critical sectors.
Recap of AI's Impact
Artificial intelligence has fundamentally altered the way we interact with technology. From predicting patient outcomes in healthcare to shaping personalized experiences in retail, the algorithms behind AI serve as the backbone of these advancements. The quicksilver pace at which AI integrates into diverse industries generates benefits like increased efficiency, greater accuracy in decision-making, and enhanced user satisfaction. These facets create a ripple effect—changing not just business operations but also governmental procedures, educational methodologies, and even agricultural practices.
Looking Ahead
Potential Challenges and Opportunities
As we gaze into the horizon, the landscape of AI holds both challenges and opportunities that demand our attention. One of the most pressing aspects is the ethical implications surrounding algorithmic decision-making. While AI can streamline workflows, relying on it without proper transparency could foster an environment where biases seep into critical decisions, affecting everyday lives. However, this challenge presents a golden opportunity for researchers and policymakers to innovate. Fostering responsible AI practices can engender trust among users, which is invaluable. The unique feature of integrating ethical considerations into AI design could lead to more robust frameworks, establishing AI as a tool that serves society rather than undermines it.
The Continuous Evolution of AI
The landscape of AI is not static; it’s in a state of continual evolution. This characteristic is what makes AI a particularly compelling subject across varied sectors. The adaptable nature of AI opens the door to new possibilities as it learns and evolves from its interactions with users. Such developments mean that AI systems are not just one-size-fits-all solutions; rather, they can grow and improve over time. This adaptability signifies an opportunity for deeper customization in applications, making them more effective and aligned with user needs. However, with this evolution comes responsibility. There’s a risk that as AI becomes more sophisticated, its misuse could also become more pronounced. Ensuring that growth does not outpace ethical considerations remains a paramount task for all stakeholders involved.
"The true exercise of intelligence lies not in mere computational power but in advancing our societal frameworks responsibly alongside these technologies."
In summary, as we wind down this exploration of AI, the ongoing dialogue surrounding its implications—both positive and negative—will remain crucial. The evolution of artificial intelligence is a journey filled with potential and pitfalls. Embracing the challenges while harnessing the opportunities will pave the way for a future where AI is a collaborative force, counterbalancing its impacts on humanity.