Current AI Capabilities: An In-Depth Exploration
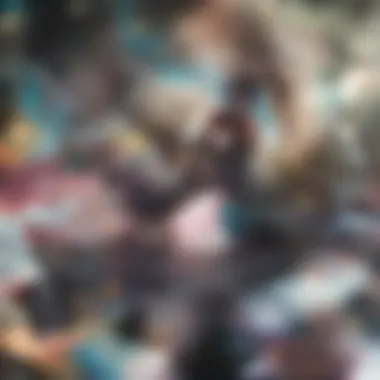
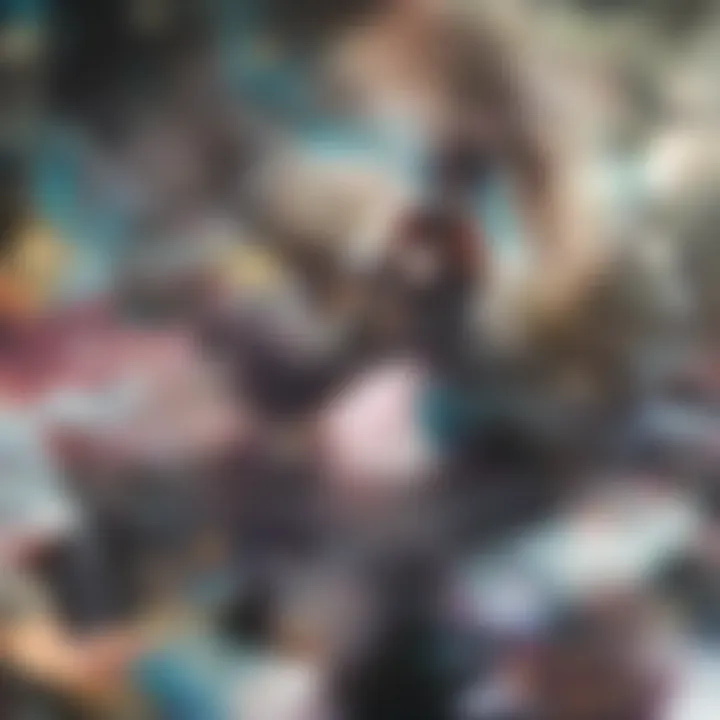
Intro
Artificial intelligence is not just a concept of the future; it is a powerful force transforming various industries today. The capabilities of AI have advanced significantly, touching aspects of everyday life. Organizations are harnessing these technologies, aiming for increased efficiency and innovation. From machine learning enhancing data analysis to natural language processing improving human-computer interaction, there is so much to explore.
This article investigates the dimensions of current AI capabilities. We will delve into methodologies, applications, and the interdisciplinary challenges they trigger. By examining recent findings and simplifying complex concepts, our goal is to provide a thorough understanding of how AI shapes our modern world.
Key Research Findings
Overview of Recent Discoveries
Recent advancements in AI reveal remarkable capability enhancements across various domains. Significant discoveries include:
- Improved machine learning techniques that allow faster data processing and predictive analytics.
- Breakthroughs in natural language processing enabling more human-like interactions through chatbots and voice assistants, like Google Assistant and Amazon Alexa.
- The rise of computer vision, which is facilitating new applications in sectors like healthcare, automotive tech, and retail.
These advancements not only heighten operational effectiveness but also create entirely new avenues of exploration within research and industry sectors.
Significance of Findings in the Field
The implications of these findings stretch beyond technical prowess. They contribute to:
- Informed Decision-Making: Organizations are utilizing AI-driven insights to make data-backed decisions. This is crucial in fields such as finance and healthcare.
- Innovation in Products and Services: Companies are redesigning offerings, integrating AI to improve user experiences.
- Ethical Discussions: With the rise of AI capabilities, discussions on privacy, bias, and transparency are becoming increasingly relevant. Institutions are now challenged to create policies and frameworks addressing these ethical implications.
As these capabilities continue shifting paradigms, the relevance of responsible deployment increases.
Breakdown of Complex Concepts
Simplification of Advanced Theories
Many advanced theories in AI are complex. However, simplification allows a broader audience to grasp these ideas. For instance, consider neural networks. They mimic the human brain's structure, allowing machines to learn from data by identifying patterns.
To further illustrate:
- Artificial Neural Networks (ANN): These consist of nodes (like neurons) interconnected to process data through layers.
- Training Process: Data is fed into the model iteratively to improve accuracy.
This foundational concept illustrates how AI learns from data, making it applicable in various contexts, from image recognition to fraud detection.
Visual Aids and Infographics
Visual tools can enhance understanding. Infographics showing AI applications in sectors like health, education, and finance can be beneficial. For more detailed visual aids, consider utilizing resources from platforms like Wikipedia, which often provide clear illustrations on complex topics.
"AI has the power to unlock numerous possibilities. However, understanding its capabilities and implications is vital to harnessing its true potential."
Foreword to Current AI Capabilities
Artificial Intelligence (AI) has permeated various aspects of our lives, transforming industries and altering how we engage with technology. Understanding the current capabilities of AI is essential for appreciating its influence on modern society. This article will focus on several critical elements, including the methodologies that underpin AI, the wide range of applications, and the ethical considerations that arise.
In today's fast-paced world, AI represents a paradigm shift in technology. It promises enhanced efficiency, accuracy, and new insights across sectors like healthcare, finance, and transportation. Thus, establishing a clear definition of AI will provide context for its significance.
- Understanding AI's relevance: A solid grasp of what AI entails helps elucidate how it functions and its potential benefits.
- Exploring methodologies: Different AI approaches, like machine learning and natural language processing, provide insight into the technology's development and application.
- Consideration of ethical concerns: As industries increasingly rely on AI, awareness of ethical issues like bias and privacy becomes crucial.
Evaluating AI capabilities is not just an academic exercise but a necessity as we navigate future challenges and opportunities.
Defining Artificial Intelligence
Artificial intelligence refers to the simulation of human intelligence processes by machines, particularly computer systems. This includes learning, reasoning, and self-correction. AI operates under various methodologies that structure its functionality.
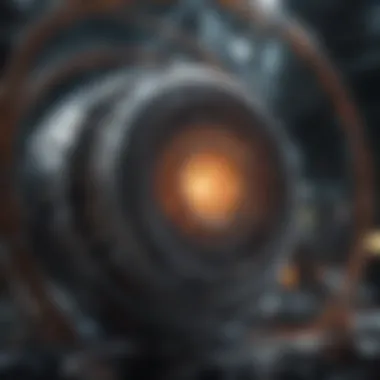
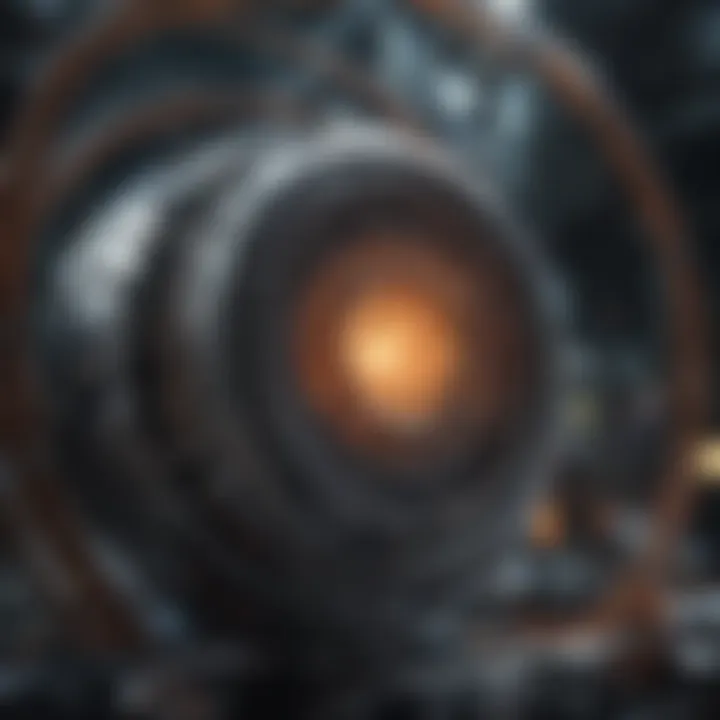
While AI systems can perform specific tasks better than humans, they lack self-awareness and consciousness. Key components of AI include:
- Machine Learning: Computational methods allowing systems to learn and improve with experience.
- Natural Language Processing: Techniques that enable computers to understand and respond to human language.
- Robotics: The branch of AI that deals with robots and their ability to perform tasks autonomously.
Defining AI correctly sets the stage for understanding its practical applications. It highlights the differences between machines mimicking intelligent behavior and true human cognition.
Historical Context of AI Development
The journey of AI began in the mid-20th century when researchers first aimed to replicate human thought processes in machines. The term "artificial intelligence" was coined during the Dartmouth Conference in 1956, which is often considered the birth of AI as a field.
Over the decades, AI development has experienced cycles of optimism and disenchantment. Early AI models were primarily driven by rule-based systems. Data-driven approaches gained prominence later, especially with advances in computer processing power.
Key milestones include:
- Expert Systems (1960s-1980s): Early AI applications relying on programmed knowledge.
- Machine Learning Surge (1990s-2010s): A shift from rule-based systems to statistical methods that adapt and learn from data.
- Deep Learning (2010s-): An evolution leveraging neural networks for complex problem solving.
The historical milestones in AI development provide a framework for understanding its current capabilities and future potential. Through a reflection on the past, we can better appreciate how far artificial intelligence has come and what lies ahead.
Key Methodologies in AI
The methodologies supporting artificial intelligence are the backbone of its capabilities and applications. Each method, from machine learning to natural language processing, offers distinct advantages that address specific needs across various contexts. Grasping these methodologies provides insight into the efficiencies and innovations AI brings to diverse sectors.
Machine Learning Techniques
Machine learning is one of the most prominent concepts within AI. Its approaches enable the extraction of patterns from data, facilitating predictions and decision-making. The three main types of machine learning techniques are supervised learning, unsupervised learning, and reinforcement learning.
Supervised Learning
Supervised learning focuses on learning from labeled data. This method requires a training dataset containing input-output pairs. The goal is to map inputs to outputs effectively based on this training. A key characteristic of supervised learning is its ability to provide predictions, making it a popular choice for tasks like classification and regression.
The unique feature of supervised learning lies in its reliance on the quality of the labeled data. The performance of models is directly influenced by the comprehensiveness and accuracy of training examples. The advantages include clear guidance and measurable outcomes, as predictions can be evaluated against known results. However, the dependency on labeled data can limit its application in scenarios where data is scarce or difficult to label.
Unsupervised Learning
Unsupervised learning, in contrast, deals with unlabeled data. Its main aim is to identify patterns or groupings within the data without prior knowledge of the outcomes. This method is beneficial for exploratory analysis and is widely used for clustering and association tasks.
The key characteristic of unsupervised learning is its ability to reveal hidden structures in data. This makes it useful for various applications such as market segmentation or anomaly detection. A unique feature of unsupervised learning is the potential to uncover insights that may not be immediately apparent. While it offers flexibility, the lack of labeled data can lead to challenges in evaluating the results. Additionally, without guidance, the interpretation of findings can sometimes be subjective.
Reinforcement Learning
Reinforcement learning is an approach based on the concept of agents learning to make decisions through trial and error. In this method, an agent interacts with an environment, receiving rewards or penalties based on its actions. This feedback loop is critical in shaping the agent's strategies for optimal decision-making.
A crucial characteristic of reinforcement learning is its adaptability. The agent continually learns and improves its strategies over time, making it highly beneficial for dynamic environments such as gaming and robotics. The unique aspect of this method lies in its ability to operate without a predefined dataset, learning directly from interactions. However, it requires a considerable amount of data and computation, which can be a disadvantage in resource-limited settings.
Natural Language Processing
Natural language processing (NLP) encompasses techniques that allow machines to understand and manipulate human language. Its relevance continues to grow as AI systems increasingly interact with people through text and speech. The primary methodologies in NLP include text analysis and sentiment analysis.
Text Analysis
Text analysis is the process of deriving meaningful information from text. Through various techniques, it categorizes and extracts pertinent data from large corpora. A key characteristic of text analysis is its ability to handle diverse text formats and structures. It stands out as a beneficial choice for businesses seeking insights from customer feedback or social media.
The unique feature of text analysis lies in its capability to automate the extraction of valuable information, saving time and resources. Its advantages include improved decision-making based on data-driven insights. On the downside, challenges such as ambiguity in language or context can complicate the analysis.
Sentiment Analysis
Sentiment analysis focuses specifically on understanding the emotional tone behind a body of text. It assesses opinions, emotions, and attitudes, enabling companies to gauge public sentiment about their products or services. A key characteristic of sentiment analysis is its application in real-time monitoring of social media conversations.
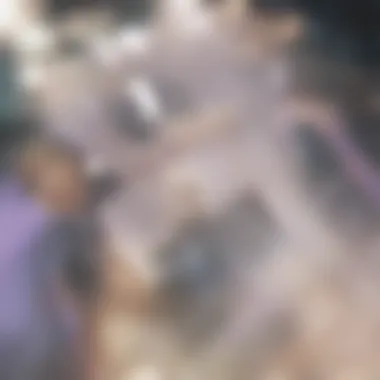
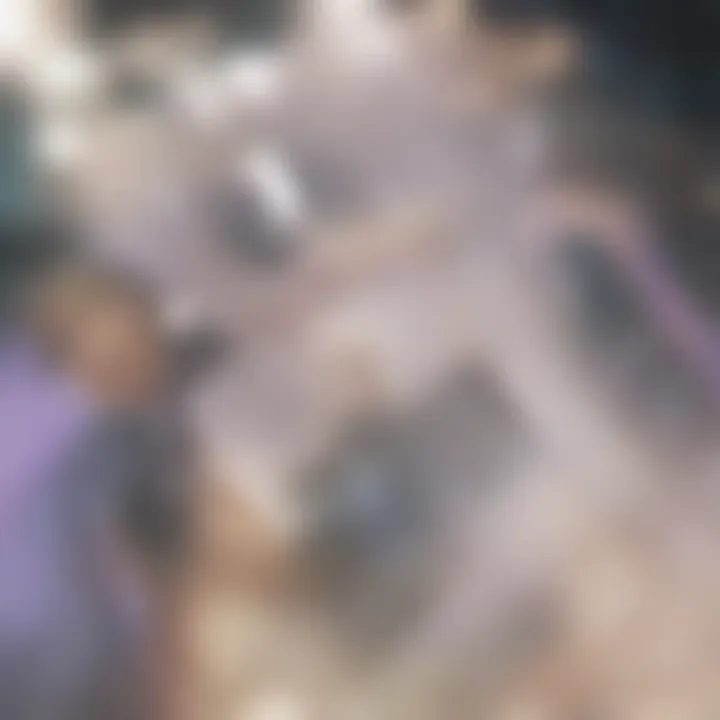
This methodology is beneficial for marketing strategies and brand reputation management. The unique aspect of sentiment analysis is its emphasis on nuance — deciphering positive, negative, or neutral sentiments. However, limitations exist in the form of sarcasm or complex expressions that may lead to misinterpretation.
"The methodologies of AI are critical to its success, offering unique approaches that cater to different tasks and industries."
Understanding these methodologies lays the groundwork for comprehending AI's capabilities and implications in various fields.
Applications of AI Technologies
Understanding Applications of AI Technologies is vital for comprehending how artificial intelligence integrates into various sectors. Each application utilizes advanced algorithms to enhance efficiency and effectiveness. These technologies can transform industries ranging from healthcare to finance, demonstrating their versatility and relevance. By exploring these practical applications, we can recognize AI's broad potential and its ability to address complex challenges, benefiting society and economy.
AI in Healthcare
Diagnostic Tools
Diagnostic tools powered by AI represent a significant advancement in the medical field. These tools harness data to assist in identifying diseases at earlier stages, which can lead to better patient outcomes. A key characteristic of these diagnostic tools is their reliance on extensive datasets of medical records and test results. This data-driven approach makes them a popular choice in modern healthcare systems.
One unique feature of these tools is their ability to learn over time, improving accuracy as they analyze more cases. The advantages are clear; quicker and more reliable diagnosis can lead to timely treatment and potentially saving lives. Nonetheless, there are challenges, such as ensuring data privacy and security, which must be addressed.
Personalized Medicine
Personalized medicine is another groundbreaking application of AI in healthcare. It aims to tailor treatment plans specifically for individual patients based on their unique genetic and environmental profiles. This approach significantly enhances the effectiveness of treatments, moving away from the traditional one-size-fits-all model. A core feature of personalized medicine is its use of predictive analytics, which allows for better identification of potential treatment responses. While this method brings numerous benefits, such as improved patient satisfaction and outcomes, it also raises ethical questions regarding data handling and access to such targeted therapies.
AI in Finance
Fraud Detection
AI technologies play a crucial role in fraud detection within the financial sector. These systems can analyze transaction patterns to identify irregularities indicative of fraud. A distinctive aspect of AI in fraud detection is its ability to adapt to evolving fraudulent techniques, making it an invaluable tool for financial institutions.
The use of machine learning models enables real-time monitoring and alerts, which allows for prompt action against suspected fraud. However, reliance on these systems can lead to concerns about false positives, potentially inconveniencing innocent customers.
Algorithmic Trading
Algorithmic trading utilizes AI algorithms to analyze market data and execute trades at optimal times. This technique can greatly reduce human error and emotion in trading decisions. A key characteristic of algorithmic trading is its speed; algorithms can react to changes in market conditions far faster than human traders. The unique feature here is the ability to process vast amounts of data quickly, allowing for more informed trading strategies. Still, there are challenges such as market unpredictability and the risk of systemic issues, underscoring the need for careful regulation.
AI in Autonomous Systems
Self-Driving Vehicles
Self-driving vehicles represent a fascinating application of AI, pushing the boundaries of transportation technology. These vehicles rely on sophisticated sensors and AI algorithms to navigate roads without human intervention. The ability of self-driving vehicles to communicate with one another and understand their environments is a key feature that promises safer driving experiences.
While there are substantial benefits, such as reduced accidents and improved traffic management, the technology also faces criticism regarding safety, regulatory concerns, and ethical dilemmas in decision-making processes.
Robotics
Robotics has embraced AI to enhance automation across multiple industries. AI-driven robots can perform tasks ranging from assembly line production to healthcare assistance. One key特徴 of robotics is flexibility, allowing machines to adapt to different tasks as needed.
The unique feature of these AI-enhanced robots is their ability to learn from their environment and improve efficiency over time. However, the downsides include job displacement concerns and the need for significant investment in infrastructure to support these systems.
Challenges and Limitations of AI
Artificial intelligence continues to evolve, yet it faces numerous challenges and limitations that impact its effectiveness across various sectors. The discussion around these challenges is crucial for understanding AI's capabilities and guiding future developments. Recognizing the ethical concerns and technical limitations allows developers and users to implement AI responsibly and effectively. This exploration will cover both ethical concerns, including bias and fairness, privacy issues, and the technical limits of data dependency and model interpretability.
Ethical Concerns
Bias and Fairness
Bias in AI systems presents a serious issue. It occurs when algorithms produce prejudiced results due to skewed training data. Such bias can further entrench social inequalities, making this a vital topic in our exploration of AI. Understanding this aspect contributes to the overall goal of fostering equitable AI solutions. The key characteristic of this bias is how AI reflects the societal values embedded in data, making it a popular and necessary focus in AI research.
One unique feature of bias in AI is its often unseen nature. Many users may not recognize bias until harmful outcomes manifest. This leads to disadvantages such as eroded trust in AI systems and potential legal ramifications. It is imperative to address these shortcomings for responsible AI deployment.
Privacy Issues
Privacy is another critical concern in the AI landscape. The collection and analysis of vast amounts of personal data can jeopardize individual privacy. This aspect plays a significant role in shaping public perception of AI technologies. A key characteristic of privacy issues is that they directly impact user trust; if individuals feel their information is at risk, they may resist using AI applications.
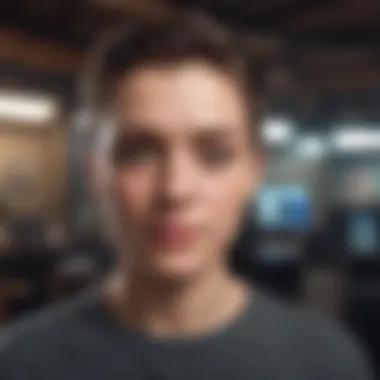
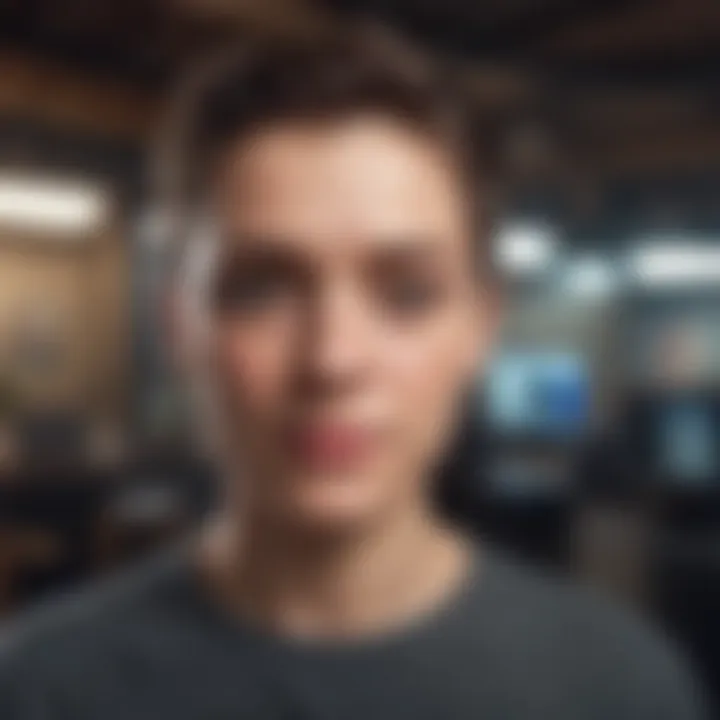
The unique feature lies in balancing data utility and user privacy. While AI models require substantial data for training, strict measures must be in place to protect individual information. The advantages include enhanced security and ethical considerations, while disadvantages consist of potential data breaches and misuse of information.
Technical Limitations
Data Dependency
Data dependency is a fundamental challenge in the AI domain. AI models rely heavily on high-quality data for training, and the absence of such data limits their functionality. This aspect significantly contributes to the overall goal of developing functional AI systems. A key characteristic of data dependency is its direct influence on model performance—inadequate or biased data leads to poor outcomes.
A unique feature of this dependency is the need for continuous data while training models. As environments change, old data can become irrelevant. The advantage of ensuring diverse data sets is improved model accuracy, while the disadvantage includes the difficulty of obtaining comprehensive datasets, especially for niche applications.
Interpretability of Models
Model interpretability presents an additional technical limitation. Many advanced AI models, like deep learning networks, function as black boxes, making their decision-making processes challenging to examine. This characteristic is crucial as it impacts accountability for AI systems. A key reason for addressing interpretation is that stakeholders need assurances about AI decisions.
One unique aspect of interpretability is its effect on user trust. When AI outputs are explainable, users can better understand the reasoning behind decisions, increasing their comfort. However, the downside includes a trade-off; more interpretable models can be less powerful or accurate compared to complex models. As AI evolves, the challenge will be finding a balance between accuracy and interpretability.
The Future of AI
The future of artificial intelligence is a topic of great significance in understanding its evolving landscape. As AI technology advances, various sectors will be influenced in profound ways. Focussing on emerging trends and societal impacts will help frame how AI will shape our lives, economies, and ethical considerations. It is crucial to analyze these aspects as they help us grasp the potential of AI and prepare for challenges that may arise.
Emerging Trends in AI Research
Explainable AI
Explainable AI refers to methods that make AI decisions understandable to humans. This is vital in creating trust between users and AI systems. By promoting transparency, stakeholders can comprehend how algorithms reach conclusions. A key characteristic of Explainable AI is that it demystifies the decision-making process of complex models.
In this article, Explainable AI is beneficial as it helps bridge the gap between technology and user comprehension. One unique feature is how it helps in sectors such as healthcare, where understanding AI's reasoning can be critical. The advantages include increased trust and improved user acceptance. However, the complexity of certain models can still pose challenges to clarity in explanation.
Generalized AI
Generalized AI strives for the development of systems that can understand and learn any intellectual task instead of being limited to specific functions. Its contribution towards cognitive versatility can radically extend the applications of AI. This includes the ability to adapt to new contexts and solve diverse problems without needing extensive retraining.
A notable characteristic of Generalized AI is its potential to surpass narrow AI capabilities. This is beneficial as it allows applications across various domains, thus broadening the impact of AI technology. The unique feature lies in its adaptability, which provides significant advantages for innovation and problem-solving. A challenge, however, is that achieving true Generalized AI remains largely theoretical, raising concerns over practicality.
Impact on Employment and Society
Job Displacement
Job displacement is a pressing issue arising from the proliferation of AI technologies. As automation increases, jobs in various sectors may become obsolete, leading to economic difficulties for affected workers. The key characteristic of this aspect lies in its immediate relevance; understanding this issue is crucial for policy-making. In this article, the discussion on job displacement is central because it highlights the need for a balanced approach to AI integration in workplaces.
One unique feature is the potential for certain job sectors, like manufacturing, to experience severe job loss. While AI offers opportunities for efficiency, it also raises substantial risks. Advantages include the possibility of creating new job types that require advanced skills, but the disadvantages encompass the lengthy transition period, which may lead to economic instability.
AI-Driven Innovations
AI-driven innovations encompass novel concepts and technologies informed by AI applications. These innovations can significantly enhance productivity, efficiency, and creativity across various sectors. A key characteristic of AI-driven innovations is their capacity for continuous improvement, which allows rapid advancements in knowledge and technology.
In this article, AI-driven innovations are essential due to their transformative potential. The unique feature of these innovations is their ability to integrate seamlessly into existing systems. They can offer significant advantages, like increased productivity and enhanced capabilities. However, there are also disadvantages, such as increased dependency on technology and the risk of cybersecurity threats.
Advancing AI technology shapes not just industry, but also social structures, requiring a careful examination of its implications.
By understanding these emerging trends and their implications, we can foster a balanced integration of AI into society, preparing for both its opportunities and challenges.
Epilogue: Evaluating Current AI Developments
In the context of this article, the conclusion serves as a crucial point to synthesize the extensive discussion on artificial intelligence. By evaluating current AI developments, we reflect on both the advancements and challenges that have emerged in recent years. This section underscores the significance of AI technologies across multiple sectors and their ongoing influence on modern society.
Our journey through the capabilities of AI has highlighted key methodologies, such as machine learning and natural language processing. These methodologies form the backbone of numerous applications that span healthcare, finance, and autonomous systems. Each application demonstrates the profound potential of AI to enhance efficiency, accuracy, and innovation.
However, it is essential to consider the challenges that come alongside these developments. Ethical concerns, such as bias and privacy issues, have become more prominent as AI technologies become more integrated into everyday life. Acknowledging these challenges is vital for responsible AI deployment.
The future of AI is closely linked to emerging trends, including explainable AI and generalized AI. These trends promise to address some of the limitations we currently face, while simultaneously pushing the boundaries of what AI can achieve. Understanding these trajectories is critical for anyone involved in AI research, application, or policy-making.
"Evaluating current AI developments allows us to appreciate both the potential benefits and the ethical complexities inherent in this technology."