Artificial Intelligence's Impact on Scientific Research

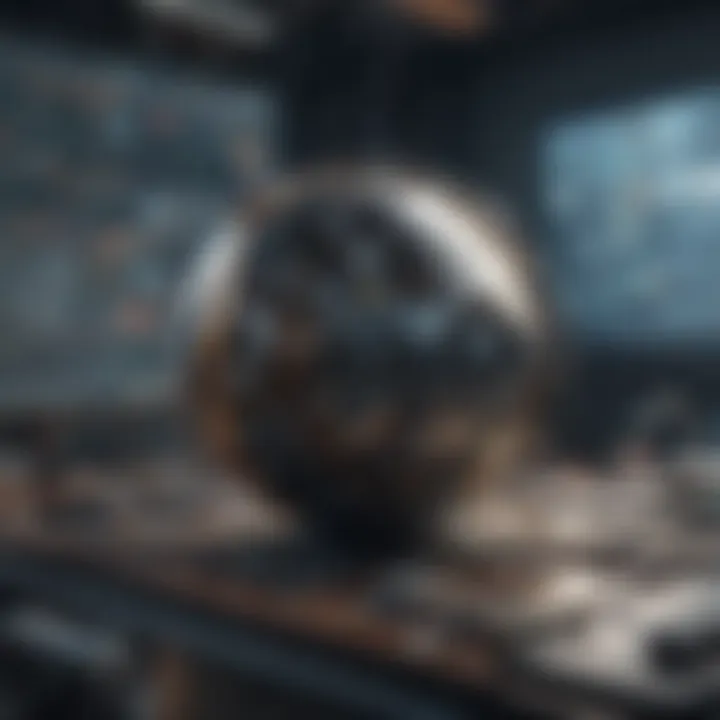
Intro
In today's rapidly evolving scientific landscape, the role of artificial intelligence (AI) cannot be overstated. Across various domains, from mathematics to life sciences, AI is not just a tool; it has become a critical participant in shaping research and enhancing our understanding of complex phenomena. This integration prompts essential questions about not only the benefits but also the ethical considerations tied to the increasing impact of AI in scientific discourses.
As AI systems become more prevalent in scientific research, their ability to analyze vast datasets and identify patterns is revolutionizing the way scientists approach problems. This article will delve into the key discoveries made possible through AI, simplifying complex theories, and addressing potential ethical dilemmas, providing a robust overview of how AI transforms modern science.
Key Research Findings
Overview of Recent Discoveries
The last few years have seen a surge in groundbreaking research facilitated by AI. For instance, in the realm of drug discovery, AI algorithms can analyze chemical compounds much faster than traditional methods, drastically shortening the time frame necessary for developing new medications. A prominent example includes the work by Atomwise, which utilizes deep learning to predict how different molecules will interact with one another, thereby accelerating drug screening processes. These advancements highlight AI’s potential to solve some of healthcare's pressing challenges.
Moreover, in the field of astrophysics, AI has been instrumental in analyzing the deluge of data generated by telescopes. Algorithms specifically designed to detect gravitational waves have led to new discoveries, including the identification of previously unseen cosmic events. These instances exemplify how integrating AI into scientific methodologies not only enhances efficiency but also expands the frontiers of human understanding.
Significance of Findings in the Field
The findings stemming from AI research indicate a drastic shift in how scientific inquiries are approached. By automating tedious tasks such as data collection and analysis, researchers can redirect their efforts towards more theoretical aspects of their work.
- Greater efficiency: AI significantly reduces the time it would take for scientists to sift through data, thereby expediting the research timeline.
- Enhanced accuracy: With machine learning models becoming increasingly sophisticated, the likelihood of human error decreases, leading to more reliable outcomes.
- Innovative methodologies: The introduction of AI into research fosters novel ways of thinking, prompting scientists to tackle challenges that previously seemed insurmountable.
"The synergy between AI and scientific research may well define the next era of discovery, transforming the methodologies we once took for granted."
Breakdown of Complex Concepts
Simplification of Advanced Theories
Many scientific concepts can be daunting, often leaving students and even seasoned researchers scratching their heads. AI's ability to distill complex theories into more digestible forms opens the door for broader understanding across various audiences. For instance, think about quantum mechanics. Once, only a handful of elite physicists grappled with its nuances, but with AI tools capable of simulating quantum systems, educational materials can become less abstract and more tangible, providing visuals that bolster comprehension.
Visual Aids and Infographics
Integrating AI into scientific communication isn’t just about making elite knowledge accessible; it often involves the creation of relevant visual aids and infographics. These tools not only clarify intricate details but also engage an audience that may not have an extensive background in science. Studies show that individuals often retain information better when presented graphically.
In this context, researchers can leverage apps to illustrate data trends or utilize AI-driven platforms that generate real-time infographics from ongoing studies, fostering a deeper understanding of scientific narratives.
As we navigate through the ensuing sections of this article, we will delve deeper into each aspect of AI's role in modern scientific discourse, providing both a comprehensive guide and critical insights into its implications. From the benefits to the ethical considerations, we aim to paint a complete picture of how these technologies are not merely additions but integral parts of the scientific community.
Prelims to Artificial Intelligence
Artificial Intelligence (AI) is not just another buzzword; rather, it represents a seismic shift in how scientific discussions are reshaping themselves in modern contexts. As we delve into the various scientific realms, the integration of AI becomes clear. It is setting new standards for methodology, enhancing data analysis, and opening doors to innovative research outcomes.
For students, researchers, and professionals alike, understanding AI is crucial for keeping pace with advancements that are molding our approaches to complex problems. This discussion isn't merely theoretical; it's about real-world implications and how AI stands at the forefront of scientific inquiry. The scope of this exploration encompasses various fields where AI applications are not only beneficial but transformative.
Defining Artificial Intelligence
At its core, Artificial Intelligence can be defined as the simulation of human intelligence in machines programmed to think and learn. This definition encompasses various dimensions such as natural language processing, machine learning, and robotics. By mimicking cognitive functions, AI systems can perform tasks ranging from simple pattern recognition to complicated decision-making processes.
The essence of AI lies in its ability to learn from data—transforming mere numbers and data points into actionable insights. One common way to visualize this is by thinking of AI as a highly sophisticated tool that helps researchers unearth patterns in large datasets which would have otherwise gone unnoticed. For instance, a study published in Nature illustrated how AI could analyze genetic data to identify mutations associated with certain diseases.
Historical Context of AI Development
Understanding the historical backdrop of AI development paints a clearer picture of its current impact. The roots of AI can be traced back to the 1950s with pioneers like Alan Turing and John McCarthy exploring the possibilities of machines performing intelligent tasks. Turing proposed the famous "Turing Test," providing a framework for evaluating a machine's ability to exhibit intelligent behavior.
As the decades rolled on, AI saw its share of ups and downs, often described through the lens of cycles commonly known as AI winters and springs. During the optimistic periods—solar springs—enthusiasts invested heavily, leading to breakthroughs in expert systems and neural networks. Conversely, when expectations were not met, funding dried up, leading to the so-called winters.
However, recent advancements in computational power, access to large datasets, and improved algorithms have breathed new life into the field. Today, AI is embedded in everyday applications, driving innovation not just in technology, but profoundly influencing sectors like healthcare, economics, and environmental science. The historical arc of AI development showcases its capacity to evolve, adapt, and redefine itself in various scientific discourses.
AI in Mathematics
The integration of Artificial Intelligence in mathematics serves as a pivotal component of modern scientific inquiry. AI augments traditional mathematical techniques, bringing forth new methodologies and perspectives. This doesn’t merely enhance efficiency; it transforms the way mathematical problems are approached and solved, allowing researchers and educators alike to unlock complex concepts more readily. The dialogue between AI and mathematics is a pragmatic one, where tools and algorithms manifest in myriad forms, driving innovations across various mathematical disciplines.
Algorithmic Advances and Theorem Proving
The impact of AI on algorithmic developments is profound. Traditional theorem proving often involves painstaking manual work, requiring an intricate understanding of logical frameworks. With the advent of AI, researchers have found themselves equipped with automated theorem provers, like Coq and Lean, which can process vast amounts of logical implications at a bewildering speed. These algorithms are not just a set of rules; they are dynamic tools that adapt and learn from previous proofs and data.
For instance, a recent advancement is the integration of neural networks within theorem proving. Neural-based methods can assist in generating proofs faster and more efficiently, learning optimal strategies through reinforcement. Such techniques reduce time spent on tedious tasks, allowing mathematicians to focus on creative problem-solving rather than rote computations.
Moreover, the accessibility of these tools has democratized the field. Complex mathematical ideas are no longer confined to elite circles; high school students, hobbyists, and non-experts can now engage in serious mathematical discourse with the help of AI-driven applications.
Data-Driven Approaches to Mathematical Research
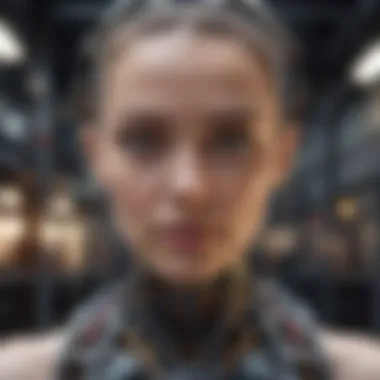
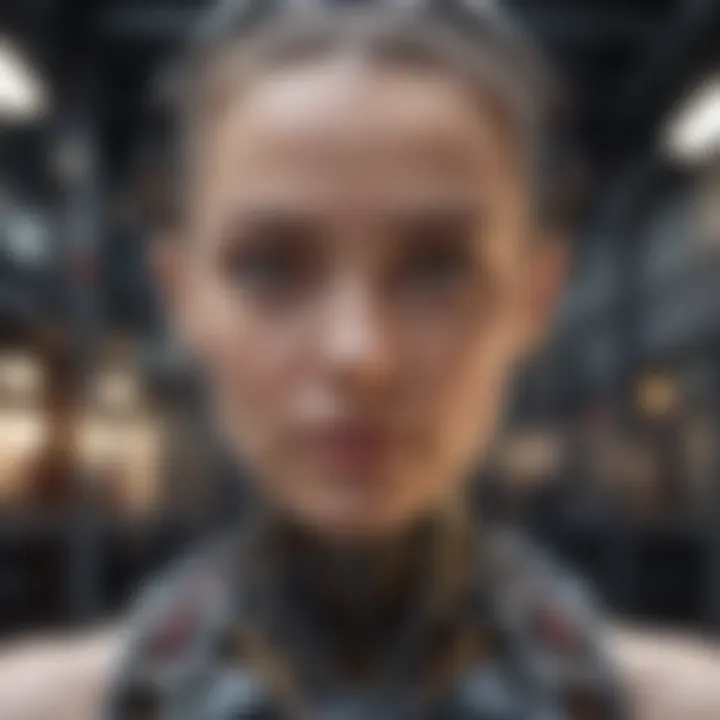
In contemporary mathematics, data has become a valuable currency. AI's data-driven approaches offer robust frameworks capable of processing large datasets to identify patterns, draw correlations, and even formulate conjectures. For instance, researchers can apply machine learning to sieve through astronomical data, obtaining insights that would otherwise elude human analysis alone.
Here are some noteworthy aspects of this trend:
- Pattern Recognition: AI algorithms excel at finding hidden patterns across massive datasets, which can lead to the discovery of new mathematical relationships.
- Predictive Analysis: By employing statistical models, AI can forecast trends and outcomes in various fields, thereby informing mathematical theories.
- Exploratory Data Analysis: AI tools can simplify complex datasets, presenting findings in more digestible formats, enabling easier interpretation and discussion among researchers.
AI is reshaping how mathematical research is conducted, steering the focus toward interdisciplinary collaboration, where mathematicians often find themselves working in tandem with data scientists and domain experts. This collaborative spirit enhances knowledge sharing and leads to innovative approaches to addressing multifaceted problems.
"AI has transformed mathematics into a more inclusive field, where insights and creativity can flow from every corner of the globe, not just academia's traditional strongholds."
Engaging with AI in mathematics goes beyond technical advancement; it heralds a new era in which interdisciplinary approaches become commonplace, blurring lines between fields and fostering a richer dialogue about scientific discovery.
AI in Physics
Artificial intelligence plays a pivotal role in advancing the field of physics by providing new methodologies and insights that were previously unattainable. The integration of AI technology has sparked significant improvements in research capabilities, enhancing the precision and efficiency of both theoretical and experimental physics. As researchers harness the power of AI, it shifts the dynamics of physics research, creating a landscape where complex problems can be tackled more effectively than ever before.
Modeling Complex Systems with AI
Modeling complex systems in physics—such as climate patterns, astrophysical phenomena, or even quantum interactions—has always been a Herculean task. Traditional modeling often relies on simplifying assumptions that can obscure important details. Here, AI stands as a champion, allowing physicists to develop models that capture the intricate details of these systems without oversimplifying the underlying physics.
AI techniques, particularly machine learning algorithms, excel at pattern recognition. By training algorithms on vast datasets, researchers can identify correlations and insights that are usually invisible to human researchers. This may include detecting subtle variations in cosmic microwave background radiation or analyzing chaotic fluid dynamics. The adaptability of AI models means they can improve continuously as more data becomes available, ensuring that they remain relevant and accurate.
For example, deep learning frameworks are now being implemented in climate modeling to predict weather patterns more effectively. Instead of relying solely on physical equations, AI-enhanced models learn from historical data and make predictions accordingly, which can lead to better forecasting and more efficient resource management.
AI's ability to handle and analyz complex datasets serves as a catalyst for advancing research frontiers, paving the way for innovative breakthroughs in understanding the universe.
AI in Particle Physics Research
The complexities of particle physics research demand precision and computational power that traditional methods often struggle to meet. AI has become a game changer in this domain, particularly in analyzing data produced by particle colliders like the Large Hadron Collider (LHC).
AI contributes significantly in several key areas:
- Data Analysis and Classification: The proliferation of data generated during particle collisions presents a challenge. AI algorithms can sift through petabytes of data at lightning speed, classifying and analyzing outcomes in a way that would take humans years to accomplish.
- Anomaly Detection: Anomalies in data may indicate new particles or unforeseen physical phenomena. AI systems can identify these anomalies in real-time, allowing physicists to adjust experiments and explore new theories without delay.
- Predictive Modeling: By modeling the interactions among various particles and predicting decay processes, AI helps researchers focus their efforts on the most promising areas of inquiry. This capability can accelerate the validation of theoretical frameworks and enhance discoveries.
"AI techniques not only enhance the speed of analysis but also open avenues for discoveries that were once thought impossible."
The use of AI in particle physics has already led to notable discoveries, including the identification of the Higgs boson. As the field evolves, machine learning techniques are expected to play an even more substantial role, assisting researchers in unveiling the mysteries of our universe with increasing clarity and efficiency.
AI in Computer Science
The role of artificial intelligence in computer science is not just significant; it's central to shaping how we think about technology today. With rapid advancements, AI has become a key player in optimizing processes, solving complex problems, and improving efficiency across various applications. It acts as a powerful tool that enhances the capabilities of computer scientists and engineers alike. By integrating AI technologies, developers can streamline workflows, reduce the likelihood of human error, and ultimately pave the way for innovative software solutions.
Machine Learning Algorithms and Their Impact
Machine learning algorithms represent one of the most powerful subsets of AI. These algorithms enable computers to learn from data, identify patterns, and make decisions with minimal human intervention. One of the biggest advantages of machine learning is its capacity to analyze vast datasets—something that would take humans a considerable amount of time.
For instance, in predictive analytics, machine learning algorithms can forecast trends based on historical data, which is invaluable for businesses making strategic decisions. They are utilized in natural language processing, computer vision, and even in personalized recommendations used by platforms like Netflix and Amazon.
Moreover, the training process of these algorithms can dramatically improve their performance over time. Consider the following benefits:
- Scalability: As datasets grow, machine learning systems can adapt and improve without significant reprogramming.
- Automation: By automating repetitive tasks, these algorithms free up human resources to focus on more complex issues.
- Accuracy: Advanced algorithms continuously learn and evolve, enhancing their predictive capabilities.
However, this power comes with responsibilities. Developers need to be mindful of issues like data bias and privacy concerns, as these can have far-reaching consequences. The critical takeaway is that while machine learning offers exceptional opportunities, ethical considerations must remain at the forefront of its application.
AI in Software Development and Testing
Artificial intelligence has profoundly transformed the software development cycle, impacting both the creation and maintenance of software products. One of the pivotal aspects is AI-driven testing tools that enhance software quality and speed up development timelines.
AI in testing not only automates mundane tasks but also smartly identifies bugs and optimizes processes based on data-driven insights. Unlike traditional manual testing, AI algorithms can learn from past testing sessions, predicting where potential failures may occur in the future.
A few notable advantages of AI in this segment include:
- Faster Time to Market: AI can significantly decrease the development lifecycle, allowing software to be deployed more swiftly.
- Enhanced Precision: AI systems can execute tests with a high degree of accuracy, catching errors that may go unnoticed in manual testing.
- Predictive Maintenance: By analyzing patterns from previous software performance, AI can anticipate future issues, leading to proactive solutions rather than reactive fixes.
Given these transformative effects, professionals in computer science must leverage AI effectively while remaining mindful of the changing landscape of technology. It’s crucial to adapt to these innovations continually, gaining insights from how AI reshapes the realm of software development and testing.
"AI is not just a tool; it's a revolution that has the potential to change every aspect of our tech-driven lives."
Embracing the changes brought on by AI in computer science is not only advisable, it may soon become necessary for staying competitive and relevant in today's market.
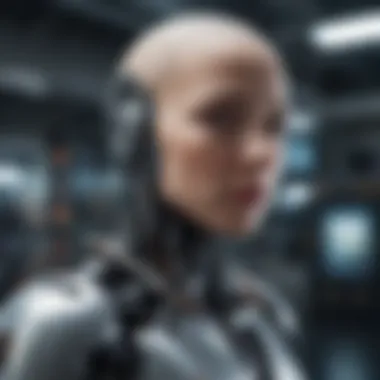
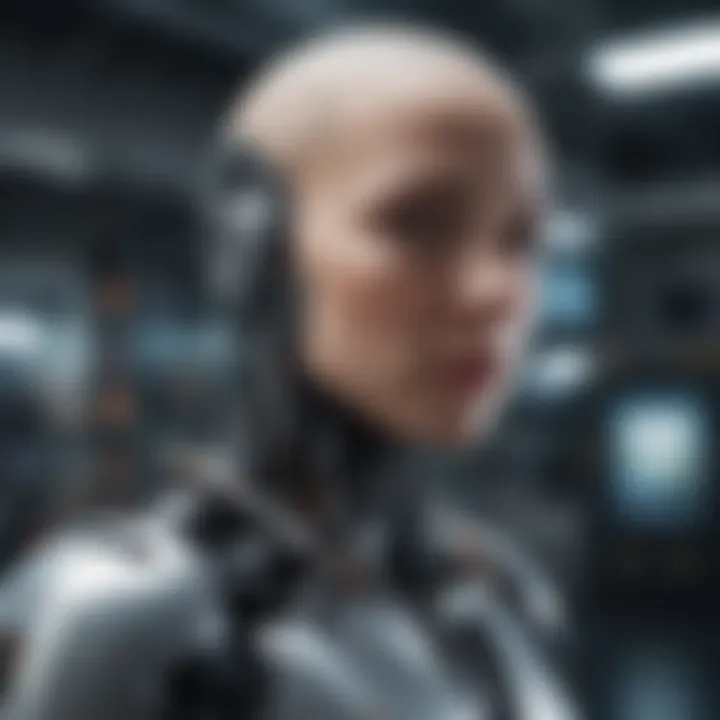
AI in Life Sciences
The infusion of artificial intelligence into the life sciences marks a watershed moment in scientific exploration. From genomics to drug development, AI redefines our capabilities, making once-daunting tasks far more manageable. The blend of computational power with biological data not only accelerates processes but also unlocks new avenues for innovation.
AI in Genomics and Biotechnology
The realm of genomics has become a fertile ground for the application of AI technologies. By harnessing vast datasets generated through sequencing, AI algorithms can analyze complex genetic information at an unprecedented scale. This computational prowess enables researchers to identify genetic mutations that contribute to diseases more effectively. For instance, machine learning models can sift through thousands of genetic variations, pinpointing potential candidates linked with conditions like cancer or rare genetic disorders.
Moreover, AI is pivotal in personalizing medicine. Algorithms that analyze genomic data can help tailor treatments to individual patients, vastly improving therapeutic outcomes. A prime example is how AI enhances the understanding of pharmacogenomics, where a person’s genetic makeup influences their response to drugs.
AI not only expedites data analysis but also aids in predicting outcomes of genetic interventions, transforming research into focused, actionable insights.
Applications of AI in Drug Discovery and Development
The journey from drug discovery to market implementation is notoriously intricate, involving substantial investment and time. AI provides a unique advantage by streamlining this process, minimizing the risks and resources required for successful outcomes.
In drug discovery, AI algorithms can analyze existing compounds and predict their effectiveness against specific biological targets. Techniques such as deep learning empower scientists to forecast how various chemical structures will behave in biological systems. This predictive capability helps prioritize candidates that are more likely to succeed in clinical trials.
Additionally, AI enhances screening processes. Traditionally, hundreds of thousands of compounds might be assessed for a single target, a process that is both labor-intensive and slow. With AI, high-throughput screening becomes feasible. Algorithms can quickly evaluate and optimize drug candidates based on simulations and historical data, leading to more focused testing phases.
The drug development stage is equally transformed through AI. By predicting potential side effects and therapeutic interactions in silico, researchers can modify drug designs before actual testing, saving time and reducing the rate of failure in later trial phases.
Overall, AI's integration into life sciences represents a formidable leap forward. It not only accelerates our understanding of life at the molecular level but also reshapes our approaches to health and medicine.
Transformative Effects of AI on Research Methodologies
The integration of artificial intelligence in research methodologies marks a pivotal shift in how scientific inquiry is conducted across various disciplines. As the capabilities of AI evolve, they enable researchers to approach questions with unprecedented rigor and efficiency. This transformation is not merely a trend; rather, it reflects a paradigm shift in the research landscape.
AI's influence can be particularly noted in two key areas: enhanced data analysis and visualization and collaboration between AI and human researchers. Together, these elements streamline the research process and foster innovation.
Enhancing Data Analysis and Visualization
In the world of research, data is the gold that fuels inquiry. However, the sheer volume of data generated today is staggering. The ability to extract meaningful insights from this data can significantly affect the outcomes of scientific research. AI plays a crucial role here through advanced algorithms that analyze massive datasets at lightning speed, identifying patterns and correlations that might go unnoticed by human analysts.
Consider the case of climate science. Researchers rely on vast data from satellite imagery, weather patterns, and atmospheric readings. Traditional methods of analysis are often slow and laborious. Yet, when AI tools, like TensorFlow or PyTorch, are employed, they can sift through petabytes of data, providing visualizations that are not only clear but predictive of future trends.
- Key benefits of AI in data analysis include:
- Speed: AI algorithms process data much faster than conventional methods.
- Accuracy: These tools minimize human errors, leading to more reliable results.
- Insights: They uncover hidden relationships within data, aiding hypothesis formulation.
Visualizations, enhanced by AI, can transform complex datasets into intuitive graphical representations. This aids in communicating findings effectively to both scientific and public audiences. For instance, in genomics, AI-driven software can create 3D visualizations of molecular structures, allowing researchers to easily manipulate and analyze complex interactions.
Collaborative AI and Human Researchers
The relationship between human researchers and AI is evolving from mere tool usage to a collaborative partnership. Rather than purely taking a backseat, human scientists are increasingly engaging with AI systems that can complement their expertise.
AI can assist researchers in various tasks such as literature review, identifying relevant studies, and even formulating new research questions. This collaborative dynamic helps scientists to focus on strategic aspects of their research rather than getting bogged down in routine tasks.
"AI is not replacing researchers; rather, it is acting as a powerful ally in the quest for knowledge."
- Benefits of collaboration include:
- Workforce productivity: Researchers can dedicate more time to designing experiments and interpreting results.
- Interdisciplinary innovation: Teams from different fields can combine their efforts with AI capabilities, leading to novel outcomes.
- Broader impact: AI-assisted research has the potential to accelerate scientific breakthroughs in areas like medicine and environmental science.
In summary, the transformative effects of AI on research methodologies are profound. By enhancing data analysis and visualization, as well as fostering collaboration between AI and human researchers, AI is reshaping how research is conducted. These advancements not only improve the quality and efficiency of scientific inquiries but also pave the way for groundbreaking discoveries.
Ethics and AI in Science
As artificial intelligence systems continue to permeate various scientific domains, the emphasis on ethics in AI applications becomes imperative. Understanding how these technologies affect society, research, and the environment is not just a practical necessity but a moral obligation. Ethical considerations in AI are not only about addressing potential risks but also about ensuring that AI technologies are leveraged for the greater good. This section speaks to the heart of AI’s role in modern scientific discourses, highlighting the balance between innovation and ethical responsibility.
Navigating Ethical Challenges in AI Applications
The landscape of AI presents nuanced ethical challenges that researchers and developers grapple with regularly. One of the pivotal challenges is bias in algorithms, where the data used to train AI systems may inadvertently reflect existing societal biases. This could lead to skewed results that perpetuate stereotypes or provide inaccurate conclusions. For instance, if an AI system trained on historical data is implemented in medical research, it could potentially favor certain demographics over others, risking the health of underrepresented groups.
"Ethical AI involves not just compliance, but a genuine commitment to fairness and accountability."
Additionally, there’s the issue of data privacy. With vast amounts of data being collected and processed, the handling of sensitive information is crucial. Researchers must ensure that data usage respects participant privacy and complies with regulations like GDPR. Transparency is also a key component; stakeholders should understand how AI systems operate and make decisions.
Another significant ethical dilemma revolves around autonomy and decision-making. As AI takes on roles ranging from advisory to directive, it’s essential to delineate the final decision-making authority. Who is responsible if an AI system makes a harmful recommendation? Cushions against accountability must be built into systems to safeguard the interests of all parties involved.
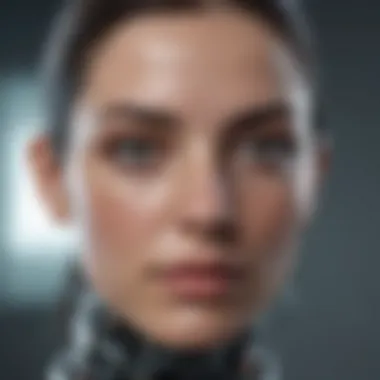
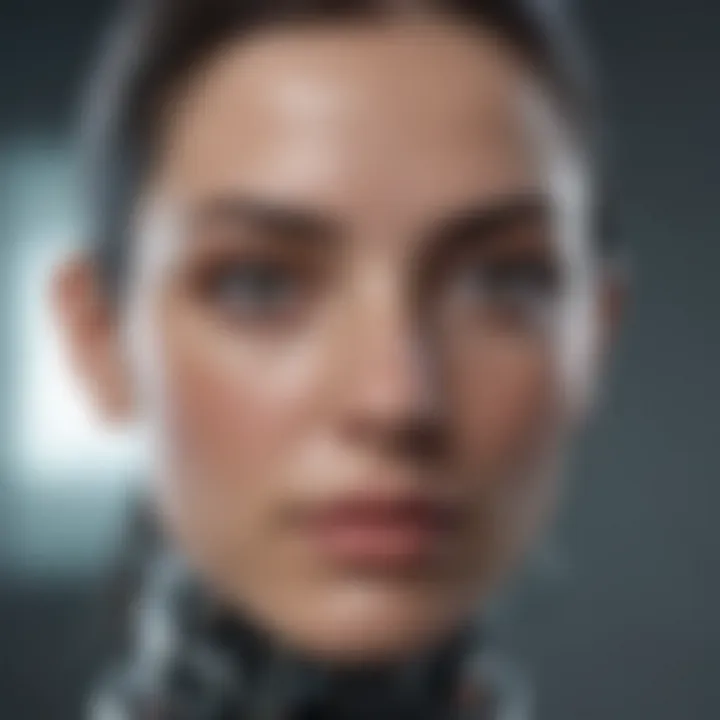
The Impact of AI on Scientific Integrity
AI profoundly affects the integrity of scientific research and knowledge production. While AI can enhance the speed and efficacy of research, it brings forth legitimate concerns about replicability and verification of findings. The ability for AI to analyze vast data sets may lead to conclusions that lack human oversight. If scientists overly rely on AI-generated insights without thorough scrutiny, the integrity of scientific findings could be jeopardized.
Moreover, there is a potential for manufacturing research outcomes. Automated systems could be exploited to produce favorable results, which can lead to misconduct. This creates an environment where scientific integrity is at risk, making it essential to maintain rigorous ethical standards.
Therefore, fostering an environment of transparency and reproducibility is crucial. Publishing the methodologies used in AI systems and openly discussing their limitations can help maintain trust in scientific inquiry. Peer reviews should expand to include assessments of AI tools employed in research, scrutinizing them just as thoroughly as experimental methods.
In summary, navigating the ethics of AI in science requires vigilance, commitment, and proactive measures across the board to ensure that advancements do not come at the cost of ethical integrity. As we advance, the interplay between ethics and technology must remain a central focus in discussions surrounding scientific research.
Public Perception of AI in Science
The integration of artificial intelligence (AI) into various scientific landscapes isn't just a technical or academic topic; it is gaining traction as a social phenomenon too. When we discuss AI’s role in the scientific realm, it's crucial to address how the general public views these advancements. This section highlights the importance of public perception, which can shape funding, policy, and even the direction of research. A positive perception can foster widespread support for innovation, while skepticism might lead to resistance or regulatory challenges, ultimately affecting research outcomes.
Understanding Public Attitudes Towards AI Technologies
Public attitudes toward AI technologies can run the gamut from enthusiastic support to outright wariness. Factors influencing these attitudes include media portrayal, individual experiences, and cultural contexts. Surveys often reveal that while many recognize the benefits that AI brings to science—like faster data analysis and more precise simulations—a significant portion remains uneasy about its implications.
Some common sentiments include:
- Optimism: Many people hold a belief that AI will lead to breakthrough discoveries and solutions for pressing global issues, such as climate change and disease control.
- Concerns about Ethics: A lingering question exists around whether AI can be trusted, particularly in sensitive areas like genetic research and patient data in healthcare.
- Fear of Job Displacement: The idea that AI could automate tasks traditionally performed by humans creates anxiety regarding employment in various sectors, including scientific research.
"Public concerns can serve as a double-edged sword; while they may highlight valid issues, they might also stifle innovation through excessive caution."
Engaging the public in meaningful dialogues about these concerns is vital for fostering a healthier relationship between society and emergent AI-based scientific practices.
The Role of Education in Shaping Perception
Education is one of the most effective tools for shaping public perception about AI in science. When the public is equipped with knowledge about the functions and limitations of AI technologies, misunderstandings can decrease, leading to more informed opinions.
Here are some effective approaches where education plays a key role:
- Curriculum Development: Integrating AI education into school and university curriculums can demystify the subject. As students learn about AI's strengths and weaknesses, they become more equipped to discuss and assess AI technologies critically.
- Public Workshops: Community-driven workshops that focus on AI literacy can open doors for discussions about benefits and ethical dilemmas, allowing participants to voice concerns and seek answers in a collaborative environment.
- Accessible Resources: Platforms like en.wikipedia.org and britannica.com house valuable information that helps bridge the knowledge gap. Ensuring that such resources are easy to understand encourages broader engagement.
As society continues to grapple with the complexities surrounding AI in science, education will serve as the cornerstone for fostering an informed populace, paving the way for a more nuanced understanding of this transformative technology.
Future Directions of AI in Scientific Research
Artificial intelligence continues to evolve at a rapid pace. Its integration into scientific research opens new avenues and shapes future directions that are worth exploring. These directions have profound implications for how researchers approach problems, analyze data, and collaborate across disciplines. This section sheds light on the importance of anticipating developments in AI technologies and fostering interdisciplinary collaborations.
Anticipated Developments in AI Technologies
With the relentless progress in AI, it's crucial to keep an eye on upcoming innovations. These advancements can potentially alter key aspects of scientific inquiry. One significant area of focus is the development of enhanced machine learning models. With deeper neural networks becoming more accessible, researchers can expect:
- Improved predictive analytics: AI will refine its ability to accurately model complex systems, enabling researchers to forecast outcomes more reliably.
- Integration of explainable AI: As the demand for transparency increases, the focus will shift toward creating AI systems that can provide explanations for their predictions. A better understanding of AI decision-making processes is essential in maintaining trust within the scientific community.
- Quantum computing influence: As quantum computing matures, its synergy with AI could lead to breakthroughs, particularly in fields like materials science and biochemistry, enabling researchers to simulate molecular interactions with unmatched precision.
While these advancements hold great promise, they also come with challenges. Maintaining data privacy, dealing with biases in algorithms, and ensuring AI systems are ethically designed are ongoing considerations.
Emerging Interdisciplinary Collaborations
In a world where knowledge is increasingly specialized, interdisciplinary collaborations become essential for growth. The emergence of AI has stirred up this phenomenon, encouraging experts in disparate fields to join forces. These collaborative efforts are set to harness AI power effectively. Here are a few key aspects to consider:
- Cross-pollination of ideas: By bringing together different disciplines, researchers can integrate various perspectives—mathematicians, biologists, and computer scientists working side-by-side. This mix can lead to innovative solutions that wouldn't surface in isolation.
- Creation of hybrid teams: When scientists from diverse fields team up with AI specialists, they can tackle complex, multidisciplinary challenges. For example, biomedical researchers collaborating with data scientists can better analyze genomic data and uncover patterns in diseases.
- Interdisciplinary educational programs: As the demand for expertise in AI shapes the job market, educational institutions are beginning to design curricula that incorporate AI across various scientific disciplines. These programs will ensure that the next generation of researchers is well-equipped to leverage AI in their pursuits.
Collaboration in research fosters a culture of collective intelligence. When experts unite, they stand to achieve more than what their individual domains could yield alone.
Ending
In synthesizing the myriad ways in which artificial intelligence has woven itself into the fabric of modern scientific discourses, several critical points emerge. AI's presence is not merely a passing trend but a profound evolution in the way research is conducted across various disciplines. It markedly enhances our capacity for data analysis, enabling researchers to sift through voluminous datasets with unprecedented speed and accuracy. This rapid processing is significant as it can lead to innovations that may have taken years or decades using traditional methods.
Summarizing Key Insights on AI Integration
The integration of artificial intelligence into scientific methodology unveils key insights that shape our understanding of its transformative role. Firstly, AI facilitates enhanced collaboration among diverse research fields.
- Interdisciplinary Approach: AI obliterates the boundaries that traditionally segmented disciplines, promoting holistic solutions to complex problems. For instance, the intersection of AI with genomics and biotechnology has led to breakthroughs in personalized medicine.
- Efficiency in Research: Tasks that once demanded a considerable amount of time, like data collection and initial analysis, can now be automated. This reshapes the workflow of scientists, letting them focus on hypothesis generation and creative thinking.
- Accessibility of Techniques: The use of AI democratizes research methodologies, making advanced analytical tools available to institutions with limited resources. This shift fosters innovation in settings that might otherwise struggle to keep pace with advancements.
"AI not only accelerates the pace of discovery but also broadens the horizon for scientific inquiry, reminding us of the intricate connections within and between disciplines."
Final Thoughts on the Future of AI in Science
Looking towards the future, the role of artificial intelligence in science appears to be positioned for significant growth. As algorithms become increasingly sophisticated and capable of learning from broader datasets, they will likely contribute to deeper insights and enhanced accuracy in predictions.
The potential for AI to reshape scientific landscapes includes:
- Advancements in Predictive Modeling: Improved models in climate science, healthcare, and even economics stand to better inform policy decisions and foster sustainability.
- Ethics and Responsibility: With great power comes great responsibility. The ethical considerations surrounding AI's application in science will continue to evolve, necessitating continuous dialogue among researchers, ethicists, and policymakers.
- Global Collaboration: The need for global cooperation in AI research is paramount. Collaborative projects can address universal challenges like climate change and pandemics, leveraging diverse expertise.