Advancements in AI Chip Technology: A Detailed Review
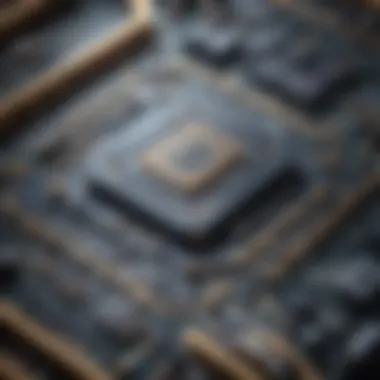
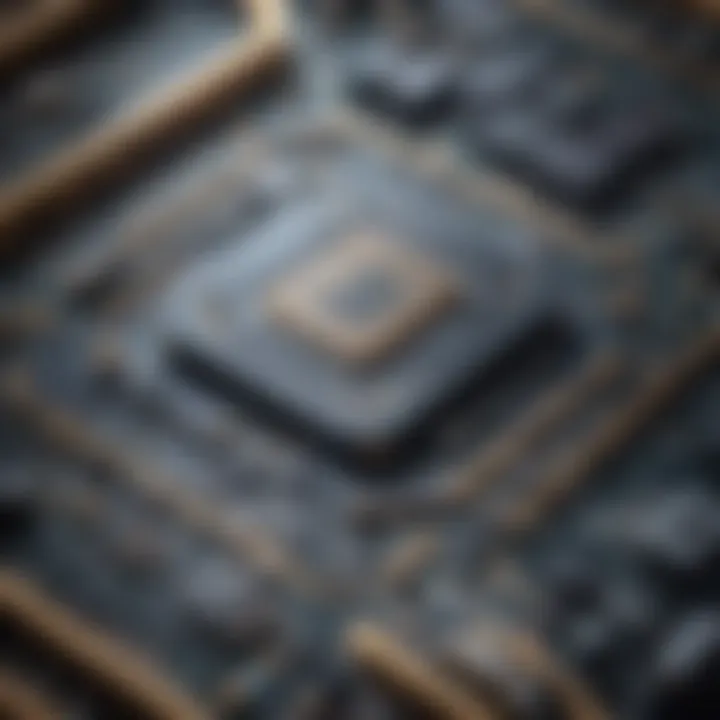
Intro
In the landscape of technology, few advancements have garnered as much interest as the evolution of AI chip technology. These chips serve as the backbone for artificial intelligence applications, driving progress in an array of sectors from healthcare to finance, automotive to entertainment. As we peel back the layers, it becomes evident that AI chips are not just simple processors; they embody complex architectures and designs that enhance computational performance in ways previously thought impossible.
This article sets the stage for an extensive exploration of AI chip technology. The significance of AI chips in the modern computational ecosystem cannot be overstated, as they hold the potential to reshape industries and influence everyday life. The upcoming sections will provide an overview of recent advancements, breaking down intricate concepts and elucidating their relevance in both academic and practical contexts.
From innovative designs to persistent challenges, we aim to offer a cohesive understanding of the journey AI chips have undertaken. Letโs dive into this fascinating world, discussing key research findings that highlight the trajectory of AI in computation.
Intro to AI Chip Technology
Artificial Intelligence (AI) chip technology is a cornerstone of modern computing, forging ahead in a world increasingly driven by data and machine learning. Its significance cannot be underestimated, as it serves not only as the backbone for AI algorithms but also as a primary enabler of rapid advancements across various sectors.
The importance of AI chips goes beyond speed and processing power. These specialized chips, designed to optimize performance for AI tasks, do more than crunch numbers; they empower applications ranging from natural language processing to complex image recognition. Understanding this technology paves the way to grasp the broader implications of AI in consumer electronics, automotive technology, healthcare solutions, and much more.
In this article, the goal is to provide an in-depth look at the evolution, architectural designs, and real-world applications of AI chip technology. Weโll explore its historical trajectory, how it has shaped computational advancements, and the challenges it faces in contemporary tech landscapes. This comprehensive analysis aims to offer valuable insights for students, educators, and professionals, connecting the dots between cutting-edge technology and its practical implications.
Definition and Importance
AI chips, also referred to as artificial intelligence accelerators, are built specifically to manage the formidable computational demands of AI workloads. Unlike traditional processors like CPUs, these chips are honed for particular tasks, maximizing efficiency and speedโthink drag-racing an electric car against a standard sedan. With evolving workloads in AI applications, these chips have become critical. The versatility they bring to computational tasks in areas like computer vision and data analysis makes them indispensable for those serious about leveraging AI effectively.
"AI is remarkably powerfulโbut it needs the right engine to harness its potential efficiently."
The importance of AI chip technology spans various sectors, impacting everything from daily consumer products to advanced industrial applications. Their proficiency in handling vast datasets allows industries to innovate and propel their services into new realms of capability.
Historical Context
The journey of AI chip development is as intriguing as the advancements themselves. It dates back to the 1960s when the concept of neural networks began to take root. Early attempts to fabricate chips dedicated to AI were rudimentary and lacked the necessary computing power.
Timeline of Key Developments:
- 1960s: Birth of the concept of neural networks.
- 1980s: The introduction of parallel processing begins to influence chip design.
- 1990s: Increase in interest towards application-specific designs, such as ASICs (Application-Specific Integrated Circuits).
- 2010s: Deep learning unlocks new frontiers in AI, leading to the rise of GPUs as a preferred choice for AI workloads.
- 2020s: Emergence of dedicated AI chips like Googleโs Tensor Processing Units and various products from startups aiming to carve niches in the market.
As we trace this history, it's evident that while traditional chips laid the groundwork for computing, the introduction of AI chips has marked a significant pivot. Their ability to handle specialized tasks has redefined whatโs possible in machine learning and artificial intelligence, enabling breakthroughs that were once considered science fiction.
Understanding these layersโthe definition and the historical evolutionโsets a strong foundation for delving deeper into fundamental concepts and innovations in AI chip technology.
Fundamental Concepts of Chip Design
The realm of chip design underpins the extraordinary development we've witnessed in AI chip technology. Understanding the fundamental concepts is like pulling back the curtain on an intricate machinery that powers our AI solutions. It encompasses the essential elements that dictate the performance, efficiency, and adaptability of these chips, shedding light on why they're indispensable in todayโs tech landscape.
Basic Architecture of AI Chips
At the core of any AI chip lies its architecture, which is fundamentally its blueprint. The design can influence everything from computation speed to power consumption. Each architecture has unique principles; for example, a von Neumann architecture focuses on a straightforward read-fetch-execute cycle, while a more recent development like the neuromorphic architecture mimics the human brain by allowing simultaneous processing across multiple nodes. This diversity in architectures means that depending on the task at hand, certain designs will always stand out as more efficient.
Types of AI Chips
The landscape of AI chips can broadly recognize three types:
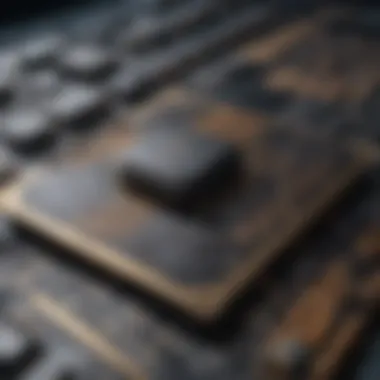
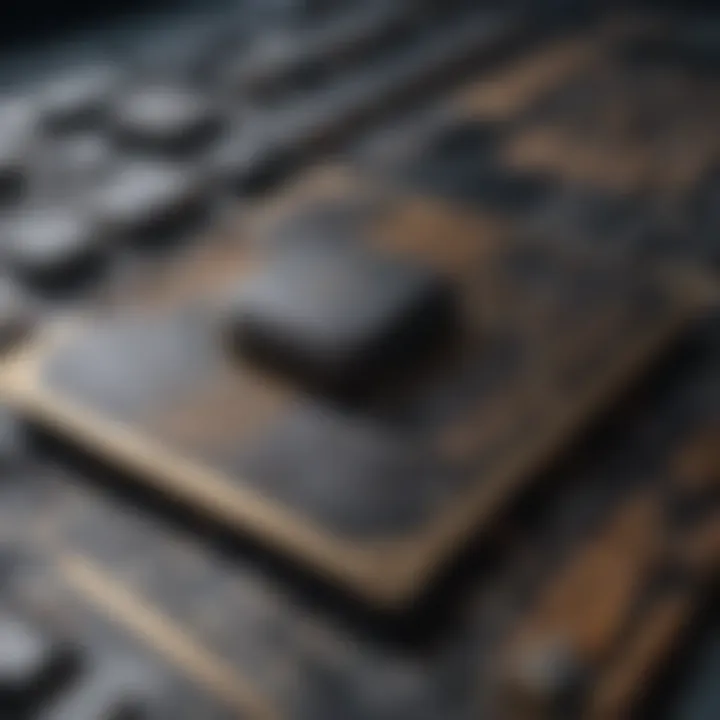
- Central Processing Units (CPUs)
- Graphics Processing Units (GPUs)
- Tensors and Application-Specific Integrated Circuits (ASICs)
- CPUs are often described as the workhorse of computing. Their flexibility allows them to perform a variety of operations but isn't always optimized for tasks that require parallel processing, which is crucial in AI tasks. They excel in executing sequential instructions and are hugely versatile, making them a popular choice in traditional computing environments. However, as AI computations become more demanding, CPUs sometimes struggle to keep pace with specialized chips designed for heavy lifting in artificial intelligence.
- On the other hand, GPUs are built for parallel processing. Originally designed for rendering graphics, they have become central to AI due to their ability to handle large blocks of data simultaneously. This characteristic makes GPUs advantageous for machine learning, where many calculations occur concurrently. They greatly enhance performance in training models, though they can be more expensive and energy-intensive than CPUs in some scenarios.
- Tensors emerge as a more niche type of chip. Specifically tailored for deep learning applications, they serve as dedicated hardware optimized for tensor operations. ASICs, while somewhat related, are unique in that they are specially designed for a particular application, often leading to extraordinary efficiencies in specific tasks. These chips can offer impressive performance metrics and lower power consumption, but their design can come with significant upfront costs and a longer development time, making them a more prominent investment for organizations aiming for specialization.
Key Technologies in Chip Manufacturing
Chip manufacturing has rapidly evolved, integrating cutting-edge technologies that transcend traditional methods:
- Nanotechnology
- Quantum Computing
- Machine Learning Optimization Techniques
- The injection of nanotechnology in chip production has transformed the field. It facilitates the design of smaller, faster, and more powerful chips by enabling transistors to shrink down to nanometer scales. This leads to reduced energy consumption and enhanced performance. However, with the complexities of scaling fabrication processes and increasing production costs, the manufacturers must tread carefully as they push the boundaries of what is physically and economically feasible.
- Quantum computing stands at the forefront of technological potential, likely to shake the foundations of chip design. It operates on principles vastly different from classical computing, allowing for computations that were previously thought impossible. The crux of quantum computing lies in its qubits, which can exist in multiple states simultaneously. However, the technological challenges, such as error correction and qubit coherence, present hurdles that need attention before it becomes mainstream.
- Finally, integrating machine learning into chip design enhances efficiencies in production and operation. Techniques like reinforcement learning enable adaptive manufacturing processes that fine-tune the chips for optimal performance. While this approach can yield great results, the complexity and evolving understanding of machine learning algorithms must be thoroughly navigated to realize these benefits.
Recent Innovations in AI Chip Technology
The realm of AI chip technology is experiencing a seismic shift, with innovations fueling advancements that go beyond mere computational power. These innovations are crucial, as they directly influence various aspects of artificial intelligence, from processing speeds to energy efficiency. Understanding the top-notch developments in hardware and the fresh entries in the market is essential for grasping the future potential of AI.
Notable Developments in Hardware
The pace of hardware development in AI chips has skyrocketed recently. Companies are introducing architectures engineered not just for performance but for specific tasks. One of the standout products is the NVIDIA A100 Tensor Core GPU, designed for data centers. This chip utilizes the Ampere architecture, which offers enhanced training performance and efficiency. These chips can tackle complex AI workloads like natural language processing and image recognition much faster than previous generations.
In addition, Google's Tensor Processing Units (TPUs) have made strides by boosting AI model training times. Optimized for machine learning tasks, TPUs provide the computing power needed to advance sophisticated algorithms. The growing adoption of these specialized chips has propelled AI applications across fields such as healthcare and autonomous driving, enabling process workflows that previously took days or even weeks to accomplish in a matter of hours.
- Key Innovations in AI Chip Hardware:
- Increased parallel processing capabilities
- Enhanced reduced precision arithmetic for faster computations
- Integration of energy-efficient features to cut power consumption
"The recent advancements in hardware reveal how strategically designed chips can influence AI capabilities dramatically, paving the road to autonomous systems and real-time data analysis."
As devices grow increasingly intelligent, manufacturers are responding with chips that support edge computing. For instance, companies like Intel have developed the Intel Movidius line, specifically aimed at processing data on-device instead of in the cloud. This shift not only streamlines operations but also provides lower latency solutions essential for applications in smart cities and IoT.
Emerging Companies and Startups
While established tech giants remain key players in AI chip technology, startups are asserting their footprint. One such company is Cerebras Systems, which produces the Cerebras Wafer Scale Engine. This engine is the largest chip ever made, providing unparalleled performance for AI workloads. Their approach challenges traditional chip design by rethinking scale and architecture, making it possible to reduce training times substantially.
Another startup, Graphcore, introduced its Intelligent Processing Unit (IPU), focused on machine learning tasks. With its unique architecture, it allows researchers and organizations to trial models at speeds that were unthinkable with older hardware. Innovations from these newcomers illustrate that the landscape is ripe for disruption, showing that fresh ideas can keep pace with or even outweigh established technologies.
- Noteworthy Startups in AI Chip Technology:
- Cerebras Systems: Specializes in ultra-large chips targeting complex workloads.
- Graphcore: Focuses on unique processing units tailored for machine learning.
- SambaNova Systems: Innovates in dataflow architecture, enhancing efficiency in AI tasks.
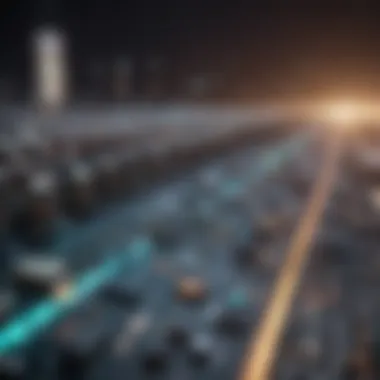
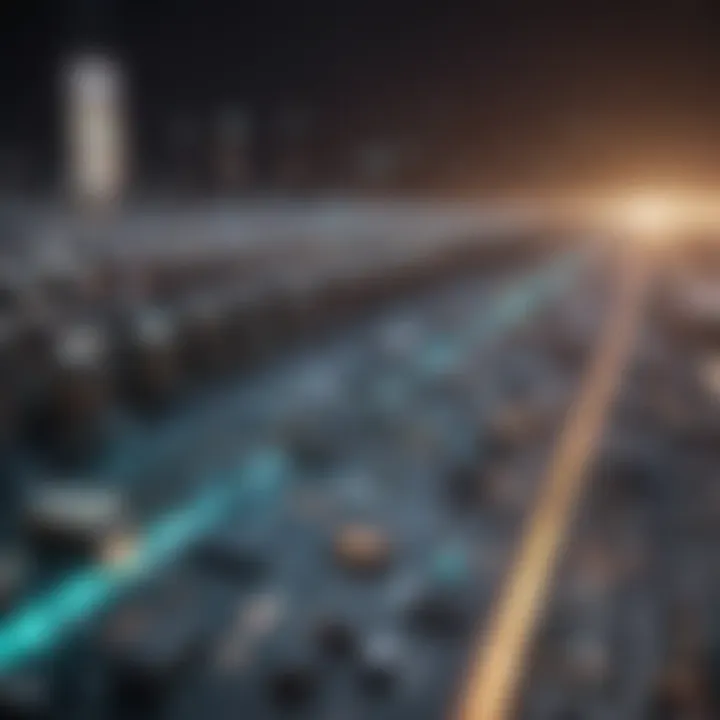
As we witness these advancements, it becomes increasingly clear that the future of AI chip technology will not solely rely on legacy manufacturers. Instead, a mix of innovation-driven startups and established entities is likely to shape the next chapter for AI applications across sectors. Understanding these trends offers valuable insights for students and professionals alike.
Applications Across Various Sectors
The impact of AI chip technology stretches far beyond mere computational prowess; it is at the core of groundbreaking initiatives across numerous sectors. Businesses and industries are harnessing the power of these chips to streamline operations, enhance decision-making processes, and deliver innovative products and services. The significance of these applications cannot be overstated, as they often lead to efficiencies that transform existing paradigms and create new opportunities.
Healthcare
In the healthcare sector, AI chips have become pivotal in advancing diagnostic capabilities and personalized medicine. They support complex algorithms that analyze vast amounts of medical data, enabling quicker and more accurate diagnoses. For instance, AI-powered imaging tools can detect anomalies such as tumors in scans with remarkable precision, often outperforming traditional methods.
Additionally, these chips drive wearable health devices that monitor vital signs continuously. They gather data that can be sent to healthcare professionals in real-time, facilitating proactive medical responses. This shift towards more data-driven patient care not only improves outcomes but also aids in preventative medicine, thus attributing further value to the healthcare system.
Automotive Industry
The automotive sector is experiencing a revolution, courtesy of AI chip technology. High-performance chips are at the heart of advanced driver-assistance systems (ADAS) and autonomous vehicles. They process data from numerous sensors and cameras in a matter of milliseconds, allowing cars to make informed decisions about speed, direction, and safety maneuvers.
Moreover, AI chips enable predictive maintenance by analyzing performance data, predicting potential failures before they occur. This approach not only enhances safety but reduces downtime, creating a smoother experience for users. The trend towards electric vehicles also benefits from AI chips, as they optimize battery management and energy efficiency, proving their versatility in modern transportation.
Financial Services
In financial services, the introduction of AI chips has led to a seismic shift in data analysis and risk management. These chips allow institutions to process transactions and market trends at unprecedented speeds. Machine learning algorithms can detect fraudulent activities in real-time, significantly reducing the financial impact of cyber threats.
Investment firms are also leveraging AI chips to enhance algorithmic trading strategies. By analyzing market data faster than any human could, AI-powered systems can react to changes in the market instantaneously, maximizing returns for traders. The ability to forecast market trends more accurately has made AI chips indispensable tools in this sector.
Smart Cities and IoT
AI chip technology is essential in the development of smart cities and the Internet of Things (IoT). These chips are embedded in infrastructure that optimizes everything from traffic signals to waste management systems. By analyzing data from various sources in real-time, they help to improve urban planning and city operations.
For example, traffic management systems equipped with AI chips can adapt to changing traffic patterns, easing congestion and improving travel times. Smart IoT devices, ranging from environmental sensors to smart grids, rely on powerful processing capabilities of AI chips to maintain efficiency and connectivity. In a world that is ever more densely populated, the efficiency offered by these technologies is not just beneficialโitโs critical for sustainable urban development.
"The future of AI chip technology is not just about speed, but smart applications that can enhance daily life and improve efficiency across all sectors."
The utilization of AI chips in various sectors exemplifies the transformative power of technology. As industries continue to explore and expand their use of this technology, the potential for innovation and enhancement in productivity seems boundless.
Challenges Facing AI Chip Technology
The realm of AI chip technology is not without its thorns. While advancements in this field are promising, they are accompanied by a set of challenges that can hinder progress and implementation. Understanding these challenges is essential for anyone deeply invested in the area of artificial intelligence, whether they are students, researchers, or industry professionals.
Technical Limitations
As AI chip technology continues its rapid progression, technical limitations pose a significant bottleneck. One prime concern is the current manufacturing capabilities that often cannot keep up with the escalating complexity of AI algorithms. For instance, deep learning models require colossal amounts of data and computational power; however, the chips we rely on often fall short in delivering the speed and efficiency needed. Performance can lag due to heating issues where higher processing demands lead to increased energy consumption and overheating.
Moreover, architectures like Graphics Processing Units (GPUs) and Tensor Processing Units (TPUs) have their own set of drawbacks. While they are well-designed for floating-point operations, they sometimes struggle with integer computations essential for many neural network models. As a result, we find ourselves caught in a race between chip innovation and the requirements of ever-evolving algorithms.
"Technological evolution, much akin to a marathon, requires not just speed but also endurance in addressing its challenges."
Economic and Supply Chain Constraints
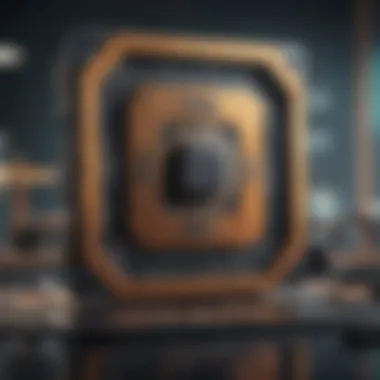
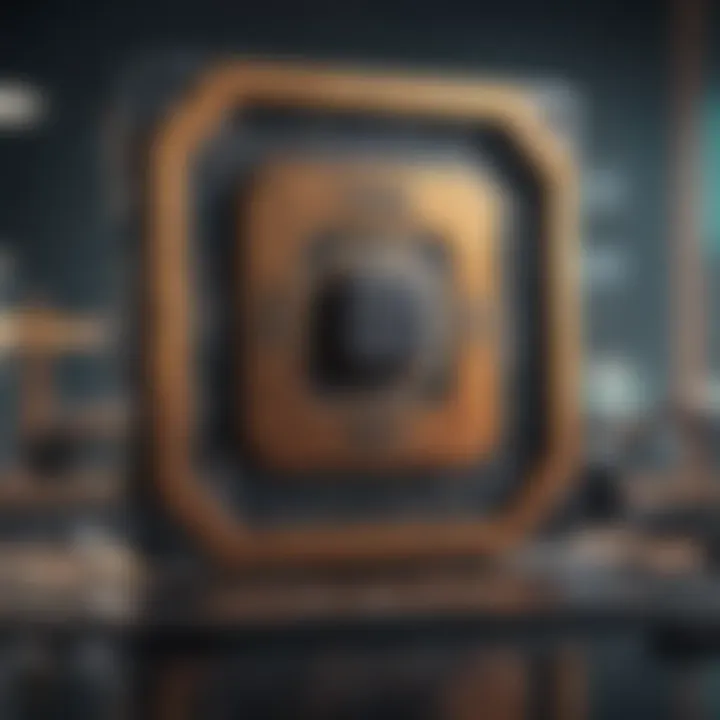
The economic landscape surrounding AI chip technology is fraught with challenges that can disrupt the supply chain. The semiconductor industry has been shaken by waves of shortages that have hampered production rates across various sectors. As demand skyrockets, supply chains have been put under immense pressure, leading to delays and increased costs. High-quality chip manufacturing relies on raw materials that aren't always easily accessible.
Investment in new fabrication facilities, known as fabs, requires immense financial backing, not to mention the time and resources needed to train skilled workers to operate these complex factories. Additionally, geopolitical factors can influence supply chains unpredictably, sometimes with debilitating effects for companies that rely on foreign partnerships.
- Some notable constraints include:
- Resource Scarcity: Key materials such as silicon and rare earth elements are constantly at risk of supply shortages.
- Global Competition: Countries are vying for technological supremacy, affecting market stability and resource allocation.
Ethical Concerns and Security Issues
The development and deployment of AI chips carry ethical implications that we must address. One primary area of concern revolves around the potential for biased algorithms. If AI chips are designed and programmed with inherent biases, the consequences can be grave, affecting lives in areas like hiring practices or law enforcement. Moreover, transparency becomes a challenge; if we cannot understand how a chip processes data, how can we trust its outcomes?
Security issues are equally pressing. The more integrated AI technologies become in our daily lives, the more potential vulnerabilities arise. Hackers may exploit weaknesses within AI chip frameworks, leading to data breaches or misuse of sensitive information. Given these concerns, regulators and stakeholders must engage in rigorous discussions about best practices and ethical guidelines to safeguard our future.
In summary, while AI chip technology holds remarkable potential, its advancement is fraught with challenges that require careful navigation. Addressing these issues is not merely an engineering problem; it is an interdisciplinary effort that will shape the trajectory of AI's role in our society.
Future Prospects of AI Chip Technology
The future of AI chip technology is brimming with potential and ripe for exploration. As the demand for faster, more efficient, and specialized computing rises, several key advancements are anticipated to shape the landscape of AI processing. In this section, we grapple with various predicted trends that will define the development of AI chips and discuss the necessity of adaptive policies to keep pace with these technologies.
Predicted Trends in Development
As we look ahead, it becomes apparent that the chip industry is poised for significant innovations. Here are notable trends on the horizon:
- Increased Integration: Chips are expected to combine multiple functionalities, compressing various processing units into single packages. This means faster data processing, using less energy without sacrificing performance.
- Focus on Energy Efficiency: With energy costs being a growing concern, research is likely to emphasize developing chips that consume less power. While still achieving high performance, these chips could revolutionize mobile devices and large-scale data centers alike.
- Neuro-Inspired Architectures: The design of future AI chips may closely mimic the human brain's neural processes. This could improve your ability to process complex tasks such as natural language understanding and sensory processing, guiding us toward smarter AI systems.
- 3D Chip Stacking: This technology stacks layers of chips vertically, increasing bandwidth and reducing latency without expanding the physical footprint. With advancements in thermal management, 3D stacking will provide a game-changing edge in performance.
- Enhanced Security Features: With rising concerns over vulnerabilities, creators may integrate sophisticated security features directly into the chip design. This includes hardware-based encryption, fostering a secure environment for processing sensitive data, which in turn boosts user trust.
"As AI systems become more integrated into daily life, the call for robust security in chip design will grow louder."
The Role of Policy in Technology Evolution
Policies play an instrumental role in shaping the progress of technology, particularly in AI chip development. Interestingly, balancing innovation with regulation becomes crucial for several reasons:
- Setting Ethical Standards: As AI grows, so does the potential for misuse. Itโs critical to establish guidelines that govern how these chips are utilized, ensuring they serve humanity positively while discouraging malicious applications.
- Promoting Research and Development: Government incentives may motivate companies to invest in AI chip technology. This could include grants or tax breaks for research, ensuring that the industry remains competitive on the global stage.
- Facilitating Collaboration: Policies can also foster partnerships between academic institutions, industry leaders, and governmental bodies, creating a collaborative atmosphere that brings fresh ideas and innovations to the table.
- Addressing Environmental Impact: As AI chip technologies advance, policies aimed at minimizing ecological footprints of chip manufacturing processes could lead to greener alternatives, balancing advancements with sustainability considerations.
- Navigating Supply Chain Challenges: Given that the industry does faced limitations due to economic factors, government intervention might be necessary for overcoming barriers in global supply chains, ensuring that resources are available for chip production.
As we navigate these future prospects, it becomes clear that both the predicted advancements in technology and the policies that will guide them are interdependent facets of a wider landscape. Embracing these changes lays the groundwork for a future where AI serves not just as a tool, but as a foundational contributor to societal progress.
Epilogue
The conclusion of this article underscores the pivotal role that advancements in AI chip technology play in contemporary computing landscapes. As we are ramping up our reliance on artificial intelligence, understanding the nuances of these chips becomes ever more critical. The digital realm has matured beyond its nascent stages, thus, AI chips are not just supporting actors; they are spearheading a revolution.
Summary of Insights
In summary, the exploration required us to sift through a myriad of developments, from the complex architectures of AI chips to their applications across various sectors, including healthcare and finance. We saw how innovative hardware has shifted the landscape, particularly with emerging startups fostering an ecosystem that embraces creativity and competition. The challenges posed, whether they be technical or ethical in nature, are ever-present. Yet, they are not barriers but rather stepping stones that hold the potential for transformative solutions.
- AI Chips and Architecture: Understanding different types of chips and their designs is crucial. The central processing units provide versatility, whereas graphics and tensor processing units focus on speed and efficiency.
- Industry Applications: From diagnostics in healthcare to innovative financial algorithms, the application scope is vast.
- Challenges and Opportunities: The sector faces hurdles, but each challenge pushes for more innovative thinking, enabling a brighter future in AI and computation.
Implications for Future Research
Looking forward, there are significant implications for future research within AI chip technology. The trajectory suggests not just a race for higher performance but a search for optimal efficiency and cost-effectiveness as well. Key areas for investigation include:
- Ethical AI: As technology races forward, there exists an acute need for research into ethical implications to ensure that AI development aligns with societal values.
- Supply Chain Sustainability: With the complexities in manufacturing, future research could focus on creating more sustainable supply chains that safeguard against shortages.
- Integration of Quantum Computing: The intersection of AI chips with quantum computing presents fertile ground for exploration, aiming to foster a new era of computation.
In essence, the importance of continued study in this field cannot be overstated. With the evolving landscape of AI, the requirement for superior, efficient, and ethically developed chips stands stronger than ever. The collective insights gathered from this analysis can serve as a valuable foundation for students, researchers, and industry professionals aiming to carve out a place in this rapidly evolving domain.